- 1Chemnitz University of Technology, Chemnitz, Germany
- 2Faculty of Humanities, Institute for Media Research, Chemnitz University of Technology, Chemnitz, Lower Saxony, Germany
Introduction: The increasing integration of digital tools in education highlights the potential of embodied pedagogical agents. This study investigates how task-related errors and language cues from a robot influence human perception, specifically examining their impact on anthropomorphism and subsequent empathy, and whether these perceptions affect persuasion.
Methods: Thirty-nine participants interacted with a NAO robot during a quiz. Employing a 3 × 2 mixed design, we manipulated the robot’s error rate (above average, human-like, below average) between subjects and language style (humble, dominant) within subjects. We measured perceived anthropomorphism, empathy, sympathy, and persuasion. Data were analyzed using multilevel modeling to assess the relationships between manipulated variables and outcomes.
Results: Our findings indicate that human-like error rates significantly increased perceived anthropomorphism in the robot, which in turn led to higher levels of empathy and sympathy towards it. However, perceived anthropomorphism did not directly influence persuasion. Furthermore, the manipulated language styles did not show a significant direct effect on perceived anthropomorphism, empathy, sympathy, or persuasion in the main experiment, despite pretest results indicating differences in perceived personality based on language cues.
Discussion: These results have important implications for the design of embodied pedagogical agents. While strategic implementation of human-like error rates can foster empathy and enhance the perception of humanness, this alone may not directly translate to greater persuasiveness. The study highlights the complex interplay between perceived competence, likability, and empathy in human-robot interaction, particularly within educational contexts. Future research should explore these dynamics further, utilizing larger samples, diverse robot designs, and immersive environments to better understand the nuances of how errors and communication styles shape learner engagement with pedagogical agents.
Introduction
The integration of digital tools into educational environments is an accelerating trend in our rapidly evolving technological landscape. A particularly promising approach is the development of pedagogical agents–virtual entities or embodied robots designed to facilitate learning across various educational contexts. These agents often incorporate anthropomorphic features, emulating human interactions, emotions, and communication styles. This strategy leverages learners’ predisposition to engage with social cues, aiming to create a more immersive and effective educational experience (Alzubi et al., 2023). Facial expressions, body language, voice modulation, and even expressions of empathy are carefully integrated to deepen engagement (Riek et al., 2009). Moreover, pedagogical agents can provide personalized feedback, encouragement, and emotional support, potentially influencing motivation, promoting deeper conceptual understanding, and enhancing long-term knowledge retention (Hu et al., 2023).
While earlier research in human-robot interaction focused on observable human characteristics like appearance (Fink, 2012), emphasis is shifting towards understanding the cognitive abilities of these agents (Airenti, 2015). The development of emotion-recognizing technologies, such as the robot Alice, and sophisticated language models (Bommasani et al., 2022) highlight the importance of higher-order social and emotional skills. However, this increased humanization introduces new challenges.
For embodied digital technologies (EDTs) to be truly assistive in our daily lives, successful interaction within relevant contexts is essential. This necessitates seamless communication: EDTs must respond appropriately to human input, and conversely, humans must be able to recognize and interpret informational and communicative signals. External appearances, human-like movements, and modulation of the voice are important foundational elements. Yet, humanized sensory information output from EDTs represents only a small component of effective communication and interaction. The attribution of human-like qualities to agents may result in the application of stereotypes and implicit biases (Bommasani et al., 2022), potentially obscuring their true capabilities. While research and development have prioritized the concrete capabilities of embodied digital technologies (Mara et al., 2022), critical questions regarding implicit cognitive and psychological processes remain unanswered. What are the cognitive mechanisms involved in engaging with these artificial, yet increasingly human-like companions? And how do these internal psychological processes interact? Can we assume that the implicit processes mirror those occurring during human-human interactions, or is there a more nuanced dynamic present?
Addressing these questions is paramount in learning and teaching contexts, as they are inherently social-cognitive activities with outcomes substantially influenced by social skills. This dependence becomes even more significant with the introduction of autonomous social pedagogical agents. Learning and performance-relevant information is no longer merely presented but instead become embedded in a social situation. The successful transmission and internalization of information relies on the proper alignment of social cues within the learning environment. Information presented flawlessly by the agent, in terms of tone and expression, may be ineffective if the accompanying social relevance cues are misaligned with the specific situation.
To make informed design decisions about the most impactful human characteristics to incorporate into these technologies, we must first gain a deeper understanding of the cognitive processes that humans experience during social interactions with artificial helpers. This research area remains under-explored (Airenti, 2018). The present study aims to address this knowledge gap by investigating the complex interplay between implicit attribution processes, anthropomorphism, and empathy within human-robot interaction. Specifically, we explore how the perceived anthropomorphism of a robot interacts with empathy, as well as with factors relevant to learning and performance, such as personality traits, perceived similarity, sympathy, and the frequency of errors made by the agent.
Previous research suggests that robots exhibiting unpredictable behavior or frequent errors may be perceived as more relatable, even endearing, leading to increased anthropomorphization (Gideoni et al., 2022). Conversely, robots with minimal errors are often viewed as efficient tools, eliciting fewer attributions of human-like qualities (Roesler et al., 2021). This paradoxical effect underscores the dynamic interplay between perceived performance and perceived anthropomorphism, a dynamic we aim to explore further in this study. The emergence of new technologies has expanded the use of the term “anthropomorphization” beyond its traditional scientific context. In public discourse, it frequently denotes the design of technology with human-like capabilities, regardless of whether these features elicit human-like perception in users. To address potential ambiguity surrounding terminology, we distinguish between anthropomorphic design and user-driven anthropomorphization. While anthropomorphic design refers to the intentional incorporation of cues intended to be perceived as human-like into technology, anthropomorphization includes the user’s subjective perception and interpretation of these design cues as human-like. Thus, anthropomorphic design is the inclusion of cues typically (but not exclusively) perceived as human-like. Anthropomorphization, conversely, is the process of attributing human-like characteristics to non-human entities based on the interpretation of these anthropomorphic cues.
Whether a design element triggers anthropomorphization depends on whether it is interpreted by the user as an anthropomorphic cue. These cues can be consciously or unconsciously perceived as signifying human qualities and thus initiate the process of anthropomorphization. The perception of a human name (e.g., Siri, Alexa) as an anthropomorphic cue, for example, can be influenced by various moderating factors (Epley, 2018). Conversely, non-human properties can evoke the perception of human-like intentionality, meaning design elements can be perceived as anthropomorphic even if unintentionally designed as such (van Buren et al., 2016).
Furthermore, the processing of anthropomorphic cues can be either conscious and explicit or operate implicitly. Implicit processing frees up cognitive resources for tasks requiring focused attention, such as learning, performance, communication, and social interaction; implicit processing also facilitates rapid categorization of novel stimuli and promotes efficiency in decision-making. The availability of cognitive resources is particularly important when communicating and interacting, where the attribution of a theory of mind to the interaction partner is often necessary (Sidera et al., 2018). A theory of mind is a mental model of another’s mental states–their beliefs, desires, and intentions–which is a complex construct, requiring substantial information or, in the case of a non-human entity, numerous clear, unambiguous anthropomorphic cues (Bagheri, 2023). Interaction necessitate the attribution of a theory of mind to the interaction partner (Sidera et al., 2018).
When encountering anthropomorphic cues, human cognitive models are gradually augmented with anthropomorphic concepts (Epley, 2018). This expansion requires relevant and appropriate information for integration, as incongruity can impede acceptance (Bartneck et al., 2009). Clear anthropomorphic cues facilitate adaptation. The often implicit and rapid nature of anthropomorphization, even with minimal cues, means the entity can be perceived with empathy early in the interaction. Purposeful movement, for instance, can trigger motor and cognitive empathy due to perceived intentionality. Simple geometric shapes can elicit such responses (van Buren et al., 2016).
Anthropomorphization encompasses the interpretation of a cue as human-like and the subsequent attribution of human-like qualities to the entity. This attribution validates the cue as signifying human resemblance and constitutes a key process in empathy formation. Anthropomorphization concludes once this validation is complete. Anthropomorphization, then, enables empathic responses, leading to appropriate social interactions with non-human entities.
Empathy is a multi-faceted construct that can be divided into motoric empathy, emotional empathy, and cognitive empathy. Motoric empathy is the mirroring of the motor patterns of another entity. Emotional empathy is sharing a similar affective state, while cognitive empathy includes understanding the perspective and mental state of that same entity. Empathy is rooted in social-cognitive processes that rely on motion, emotion and cognition, and anthropomorphization initiates this process for non-human entities. Consistent with this multi-stage model of interaction, neuroimaging studies have revealed similar brain regions activated during both anthropomorphism and empathy. These shared neural underpinnings have been observed in children (García-Corretjer et al., 2023) and individuals with diverse neurological profiles (Li et al., 2023).
We argue that the parallels between anthropomorphic and empathic processes extend beyond shared neural activation. Both rely on mentalizing, the process of inferring mental states (e.g., thoughts, feelings, and intentions) in oneself and others (Bagheri, 2023). This process of social cognition transforms non-human entities into social actors through the attribution of human-like mental states. These attributions, however, are not always accurate and can frequently be fictional, as exemplified in media reception of real or animated characters (Luis et al., 2023). This is not limited to robots, but extends to other non-human entities, such as virtual pedagogical agents.
We posit that the ability of a cue to elicit an empathic response is determined not by the physical presence of the entity, but by its ability to stimulate cognitive empathy, affective empathy and motor empathy. Categorizing anthropomorphic cues based on their corresponding empathic subprocess suggests that cues triggering multiple subprocesses simultaneously evoke stronger empathic responses overall. Therefore, our first hypothesis is:
H1: Higher levels of anthropomorphism will lead to stronger empathic reactions toward a non-human entity.
Robots are entering human social spaces, which leads to increasing social interaction between humans and robots and therefore necessitates understanding how humans perceive and respond to robotic agents. When robots are ascribed social agency, people tend to rely on mental models of human-to-human interaction (Waytz et al., 2014). These models are often shaped by media portrayals, which frequently depict robots as flawless machines (Horstmann and Krämer, 2019). This study investigates the influence of error rate on the perception of robot animacy and likeability. While some studies suggest that robots exhibiting errors are perceived as more human-like and likeable (Arikan et al., 2023), others highlight that flawless performance is associated with increased perceptions of competence, intelligence, and functionality (Salem et al., 2015). These are characteristics that are especially important in learning and performance contexts, where error-free robots may also be perceived as more trustworthy and reliable. Interestingly, research suggests that imperfections in human-robot interaction can enhance social perception, leading to higher ratings of popularity and credibility compared to flawless counterparts (Mirnig et al., 2017). This effect is likely attributed to the increased perceived human-likeness associated with imperfection. Based on these findings, we propose the following hypothesis:
H2: As the robot’s error rate approaches the human error rate, it will be perceived as more human-like and, consequently, more likeable.
This study additionally investigates the impact of perceived robot animacy on task performance and blame attribution. While Salem et al. (2015) found no significant effect of robot error rate on user performance, contrasting results were reported by Ragni et al. (2016). Furthermore, Waytz et al. (2014) demonstrated that individuals tend to attribute greater blame to counterparts perceived as more anthropomorphic. This finding suggests that increased perceived human-likeness leads to the attribution of enhanced mental capabilities and control over actions, implying the robot possesses agency beyond pre-programmed behavior. Supporting this notion, Furlough et al. (2021) reported increased blame attribution even towards counterparts perceived as simply possessing greater autonomy. Prior research has primarily investigated user responses to robots committing errors or acting flawlessly (Mirnig et al., 2017), focusing exclusively on violations of technical and social norms. To address this gap, the present study examines the effect of content-related errors on blame attribution. Based on these findings, we propose the third hypothesis:
H3: As the robot is perceived as more human-like, greater blame will be attributed to it for task-related errors.
This study also investigates the influence of language style on the persuasive impact and perceived anthropomorphism of robots in human-robot interaction. A crucial factor in attributing blame is the perceived level of control held by each entity involved. To effectively influence user behavior, robots must possess the ability to subtly guide or modify user actions. The Media Equation Hypothesis (Reeves and Nass, 1996) posits that individuals respond similarly to social cues exhibited by robots and those displayed by other humans, a notion aligned with our theoretical assumptions about anthropomorphism and empathy. Building upon this, several studies have explored the role of persuasion, focusing particularly on psychological reactance (Ghazali et al., 2017). Reactance describes a behavioral response to perceived persuasion attempts, often manifesting as disregard for instructions or adoption of opposing behaviors to reassert autonomy (Sharon S Brehm, 1981).
Beyond social cues (e.g., head movements, facial expressions, vocalizations), language plays a crucial role in shaping perceptions and facilitating interaction with robots and embodied digital technologies. Studies show that people respond to robots’ language similarly to how they respond to human language (Reeves and Nass, 1996). High controlling language, in particular, has been linked to increased psychological reactance, with users disregarding instructions or adopting opposing behaviors to assert autonomy (Sharon S Brehm, 1981). In addition research suggests that submissive language is perceived more favorably than dominant language (Habler et al., 2019). Similarly, extraverted robots are often perceived as more socially intelligent, likable, and animate (Mileounis et al., 2015). Conversely, robots using high controlling language are perceived as less competent, sociable, trustworthy, and anthropomorphic (Ghazali et al., 2017). In the present study, the robot will not provide direct instructions but rather subtly present its suggestions to aid participants in selecting the correct answer in a quiz. Therefore, we will manipulate the robot’s language style, contrasting a dominant and a humble version. Both conditions will incorporate characteristics from various dimensions (extraversion, high controlling language, etc.), acknowledging the inherent overlap and interconnectedness of these dimensions. Given the limited and inconclusive research on the persuasive influence of (anthropomorphic) robots based on language cues, coupled with the conflicting findings regarding anthropomorphism, this study investigates the following research question.
RQ1: How do different language styles influence human-robot interaction, especially the persuasive impact and perceived anthropomorphism of robots?
The influence of perceived robot personality on human-robot interaction (HRI) is a vital research area. Personality, a fundamental aspect of human social interaction (Aly and Tapus, 2013), consists of enduring characteristics that shape thoughts, emotions, and behaviors. Cues for inferring personality include visual elements (appearance, status), verbal markers (speech, language), and nonverbal communication (gestures, body language) (Ekman et al., 1980).
Some research suggests a preference for individuals with congruent characteristics (Tapus et al., 2008). For example, an introverted person might find an introverted robot more appealing. Tapus et al. (2008) attribute this preference to the perceived ease of adapting to similar personalities, leading to increased likeability. Extroverts tend to speak loudly, rapidly, with minimal pauses, and employ informal language and positive emotion words (Dewaele and Furnham, 1999). Conversely, Nass et al. (1995) associate submissive and introverted behavior with low controlling language. Additionally, Tapus et al. (2008) found that introverted participants preferred introverted robots, supporting the hypothesis. Interestingly, perceived robot personality was also influenced by interpersonal distance, with extroverted individuals finding closer proximity less uncomfortable than introverts (Nielsen et al., 2022), a finding consistent with the importance of interpersonal distance in human social interactions (Walters et al., 2005).
However, Lee et al. (2006) reported contrasting findings, suggesting a complementary-attraction phenomenon, where participants perceived robots with opposing personalities as more intelligent, attractive, and present. This highlights the potential influence of embodiment on social perception–interaction with a humanoid robot might be perceived more similarly to human-to-human communication than interaction with a digital avatar or text-based agent (Benk et al., 2023). Woods et al. (2005) neither confirmed nor refuted either hypothesis, potentially due to the non-humanoid nature of the robot used. Their research revealed that technical experience significantly impacted perceived personality, with individuals possessing greater experience exhibiting a stronger tendency to project human-like qualities onto robots. Walters et al. (2008) emphasized the role of robot appearance, demonstrating a halo effect where aesthetically pleasing robots were evaluated more positively, suggesting that user preferences can influence perceived personality. For instance, they observed that introverted participants with low emotional stability exhibited a preference for mechanic-looking robots.
Additional studies by Mileounis et al. (2015) yielded inconclusive results regarding both the similarity-attraction and complementary-attraction hypotheses. Introverted robots were consistently perceived as more socially intelligent, emotional, intelligent, and likable than extroverted robots, regardless of participant personality. The authors attributed this to the cooperative and submissive communication style employed by the introverted robot, which was perceived more favorably than the commanding tone of the extroverted robot.
In conclusion, current research on the influence of perceived robot personality on user preferences in HRI remains inconclusive. While the impact of personality on human-robot interactions is undeniable, further research is necessary to definitively establish the nature of this relationship. This study aims to address this gap by investigating the second research question:
RQ2: How do the robot’s perceived personality and perceived similarity influence human-robot interaction, especially in collaborative task-solving scenarios?
To test the hypotheses and answer the research questions, the following laboratory experiment was carried out, in which test subjects had the opportunity to interact with different robots.
Methods
A 3 × 2 factorial mixed design was employed to investigate the hypothesized relationships. The first independent variable, error rate, consisted of three levels: above average, human-like, and below average, and was varied between-subject. The second independent variable, language style, was manipulated within-subjects, comprising two levels: humble and dominant. The dependent variables of interest were attribution of blame and likeability. Additionally, several covariates were measured, including technophobia and technophilia, participant personality, perceived robot personality, and perceived anthropomorphism.
Participants
Participants were recruited through mailing lists of Chemnitz University of Technology and social media platforms. A total of 39 individuals (51.3% female,
Materials
The study employed two NAO robots manufactured by SoftBank Robotics. These humanoid robots stand 58 cm tall and weigh 4.5 kg. Each robot possesses 25 degrees of freedom, enabling movement of the arms, legs, and head. Additionally, they are equipped with four microphones and two cameras for recognizing human movement, faces, and facilitating interaction (Kulk and Welsh, 2008). The robots were outfitted with white shells and either red or blue accents. We chose the NAO robot for this study due to its humanoid form and interactive capabilities, making it a suitable representation of an embodied pedagogical agent. Its relatively small size and non-intimidating appearance make it appropriate for interacting with diverse participant groups. Furthermore, while our study focused on physical interaction with NAO, its design and behaviors can be readily translated to virtual representations within XR learning environments, providing a bridge between physical and virtual pedagogical agents. This allows for future studies to explore similar interactions in immersive settings where the physical presence of a robot may not be feasible or desirable. A collection of quiz questions was obtained from various websites (Kratzenberg, 2020). Some questions were supplemented with answer choices. The initial pool consisted of 36 questions, which were subsequently reduced to 30 following a pretest. These 30 questions were further divided into two separate quizzes, as described in the following section.
Pretest I - error rate
Prior research has not established a consistent approach to defining and manipulating error rates in human-robot interaction studies (Mileounis et al., 2015). As this study aims to optimize robot design for enhanced anthropomorphism, the robot’s responses should closely mimic human behavior in terms of error rates. To determine appropriate error rate levels and validate the selected quiz questions, a pretest was conducted in the form of an online survey. This pretest comprised 36 questions, each with four answer choices and one correct answer (see supplementary material). The order of questions and answer options was randomized. Upon completing the quiz, participants provided demographic information (gender, age, etc.). Participants (N = 43, age range: 18–75 years, Mage = 34.7 years, SD = 14.9 years, 60.5% female) were recruited through personal contacts, social media groups, and websites. The sample included individuals from diverse backgrounds, encompassing students (n = 9), employees (n = 23), freelancers (n = 4), pensioners (n = 3), and pupils (n = 1). Educational qualifications also varied considerably, ranging from GCSE to Ph.D. This broad demographic spread suggests the calculated error rate likely approximates the actual average human error rate.
The pretest revealed a mean error rate of 43.2% (SD = 12.2%), with a range of 11%–66%. Participants answered an average of 19.9 questions correctly. One question was deemed ambiguous and excluded from analysis. Notably, no question was answered correctly or incorrectly by all participants. To select questions for the final quizzes and determine which questions NAO would answer correctly, the questions were ranked based on their error rate. Five questions were deemed too easy due to being answered correctly by over 80% of participants and were excluded. This resulted in a final selection of 30 questions.
Given the within-subjects design for the language cue manipulation, each participant would complete two quizzes, each containing 15 questions. The questions were divided to ensure an even distribution of difficulty across both quizzes. While the calculated error rate for the “human” condition closely approximates the pretest mean, the other two conditions deviate slightly, differing by approximately one and a half standard deviations. Consequently, the final error rates were established as follows: below-average (26.7%, 4 questions answered incorrectly), human-like (46.7%, 7 questions answered incorrectly), and above-average (66.7%, 10 questions answered incorrectly). When providing incorrect answers, NAO mirrored the most frequently chosen incorrect response from the pretest. To maximize the human-likeness of NAO’s responses, question selection for correct answers was also guided by error rate. Specifically, the four questions with the highest error rate in all three conditions were designated as incorrect for NAO in both quizzes. The following three questions were designated as incorrect in the human-like and above-average conditions, and so on. Errors were introduced randomly. This approach was chosen to prevent task complexity from confounding the results. The resulting answer sets for both quizzes are presented in the supplementary material.
Pretest II - language cues
To investigate the influence of language cues on perceived robot personality and user preferences, two conditions were implemented: dominant and humble. These conditions were informed by previous research on language cues associated with various personality traits (Mileounis et al., 2015). The dominant NAO embodied characteristics of dominance, extraversion (Mileounis et al., 2015), high controlling language (Roubroeks et al., 2011), authoritarianism (Trovato et al., 2017), and masculinity (Habler et al., 2019). It employed assertive language with imperatives and commands, conveying a confident and controlling demeanor (e.g., “You have to choose answer A!”). Conversely, the humble NAO utilized low controlling language (Roubroeks et al., 2011) and exhibited submissiveness, introversion (Mileounis et al., 2015), supportiveness (Trovato et al., 2017), and femininity (Habler et al., 2019). It phrased responses as suggestions and questions, using german first-person singular pronouns more frequently and incorporating words like “maybe” or “possibly”. Additionally, it emphasized the uncertainty of its responses (e.g., “I’m not sure, but maybe it’s answer A.”). Each condition employed a total of nine distinct phrases.
To evaluate the effectiveness of the language cue manipulation, a separate online pretest was conducted. Participants were recruited through personal contacts, social media groups, websites, and university mailing lists. Students from the Chemnitz University of Technology’s Institute for Media Research received course credit for their participation. A total of 200 individuals participated, with 176 completing the survey. One participant was excluded for answering the quiz questions before NAO’s response appeared. The final sample comprised N = 175 respondents (66.9% female) aged 15–76 years (Mage = 26.5 years, SD = 9.5 years). The majority were students (72%) with either a high school diploma (42.3%) or a bachelor’s degree (35.4%). Participants were randomly assigned to either the dominant (n = 92, 68.5% female, Mage = 26.6 years, SD = 8.6 years) or submissive (n = 83, 65.1% female, Mage = 26.4 years, SD = 10.4 years) language cue condition.
The language cues employed by NAO were pre-programmed and did not adapt to participant responses. This method was chosen to ensure consistent presentation of the dominant and humble language styles across all participants. While this approach may limit ecological validity, it facilitates a controlled investigation of how language style independently affects perceptions of anthropomorphism, trust, and likeability.
The pretest employed nine questions from the first quiz, corresponding to the nine distinct phrases used in each language cue condition. NAO was not present in this pre-study, but instead presented as a minimal graphical animation. NAO’s responses were determined by the answer set corresponding to the human error rate, with four incorrect answers mimicking the human error rate. To ensure a consistent initial experience for all participants, the quiz invariably began with the easiest question, which NAO answered correctly. The order of subsequent questions was randomized. Before commencing the quiz, participants were informed that NAO’s answers might not always be accurate. Five seconds after a question appeared, NAO’s response was presented within a speech bubble. Participants were allotted time to read the question and formulate their own answer. Upon completing the quiz, they received feedback on their performance, including the number of correct answers and the correct answers for all questions.
Subsequently, participants rated NAO on five dimensions corresponding to the language cue manipulation: dominance (1–5), introversion (1–5), masculinity (1–5), control (1–5), and authoritarianism (1–5). These questions were presented in a randomized order, utilizing semantic differential scales ranging from the designated extremes of each dimension. Following this, participants’ technophilia and technophobia were measured using three adapted items from the Martínez-Córcoles et al. (2017) scale. Five-point Likert scales (1 = “not at all” to 5 = “very much”) were employed. Both scales demonstrated acceptable internal consistency (technophobia: Cronbach’s
Measures
To measure the dependent variables and coviariates, validated German versions of the instruments were used where available. If there was no German version or it was not available, the items were translated into German using a classic forward-backward translation approach.
Attribution of blame
Measured using a translated two-item scale developed by Kim and Hinds (2006)
Perceived anthropomorphism
Perceived anthropomorphism was assessed using the German version of the anthropomorphism subscale of the Godspeed Questionnaire (Bartneck, 2023)
Likability
Measured with the German version of the likability scale of the Godspeed Questionnaire (Bartneck, 2023)
Personality
Personality were measured using the german ten-item version of the Big Five Inventory (Remmstedt and John, 2007)
Empathy
Empathy was assessed using the translated state empathy scale by Shen (2010)
Technophobia and technophilia
Technophobia (
Procedure
Following an initial greeting and a brief explanation of the tasks, the experiment commenced. NAO welcomed participants and offered assistance during the quiz. Its initial color (red or blue) was randomized. The first quiz consisted of 15 general knowledge questions. Participants first read and answered each question independently. They then read their answer aloud, allowing NAO to respond. Participants could subsequently decide whether to modify their initial answer. NAO’s behavior was manipulated along two dimensions: dominance (humble vs dominance, see Figure 1 for a visual representation) and error rate (above average, human-like, below average). Both conditions were randomly assigned to participants. The first question in each quiz was the easiest, and the order of subsequent questions and answers was randomized. Upon completing the first quiz, participants received feedback on the number of correct answers achieved with NAO’s assistance. They then completed questionnaires assessing attribution of blame, perceived anthropomorphism, likability, empathy, and robot personality. The order of these questionnaires was randomized.
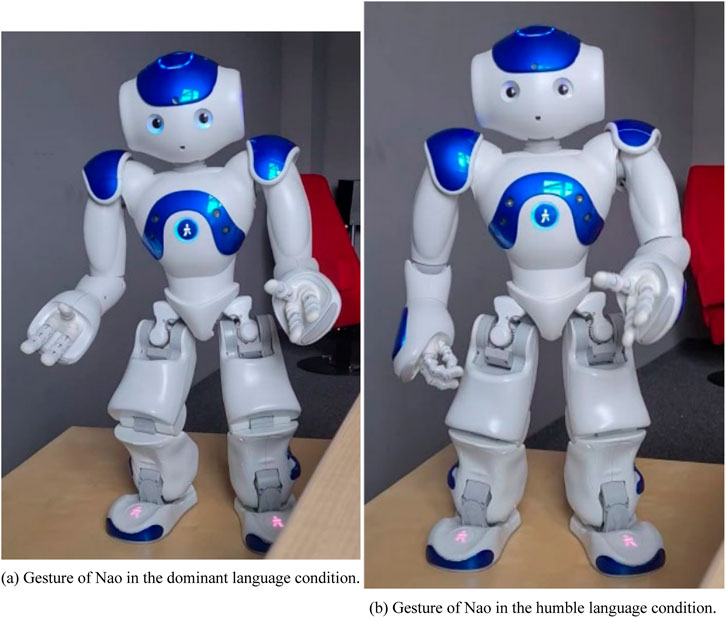
Figure 1. Gestures and Postures Emphasizing Personality Manipulation in the Nao Robot. (A) The humble condition, characterized by submissive posture and open gestures, suggesting uncertainty and approachability. (B) The dominant condition, featuring assertive posture and closed gestures, conveying confidence and control.
Following questionnaire completion, participants were introduced to a second NAO with a different color (red or blue) and personality display humble or dominant) compared to the first. Only the error rate remained constant. Participants then completed another set of 15 questions following the same procedure as the first round. After receiving feedback on their performance, they repeated the robot evaluation questionnaires. Finally, participants provided demographic information and completed questionnaires assessing technophobia and technophilia and personality factors. Participants were debriefed after the experiment and informed about the different experimental conditions, including the variations in the robot’s error rate, language style, and the purpose of these manipulations. The pre-programmed nature of the robot’s behavior and the lack of genuine emotions or intentions were emphasized to address any potential misunderstandings. As compensation for their time, participants enrolled at the Institute for Media Research could choose between two course credits, while others received 10 Euros. The complete questionnaire can be found in the supplementary material.
Data aggregation and statistical analyses
For data aggregation and statistical analyses R version 4.3.2 (R Core Team, 2024) were used. For data cleaning and aggregation specifically the tidyverse (Wickham et al., 2019) meta package in version 2.0.0 was utilized. Reliability was analysed with the help of the Psych (Revelle, 2024) package in version 2.4.1. The multilevel modeling was conducted with the help of the Lavaan (Rosseel, 2012) package in version 0.6–17. For reporting and visual data preparation, the Easystats (Lüdecke et al., 2022) meta package in version 0.7.1 was used.
For all questionnaires, scores were calculated by summing item responses and dividing by the total number of items (item mean) in relation to the length of the scale. This generated scores ranging from 0 to 1, facilitating comparison between scales of varying lengths.
To assess personality similarity, the Mahalanobis distance
Results
Reliability analysis
Our analysis of reliability showed acceptable to good reliability values for most questionnaires. The questionnaires by Martínez-Córcoles et al. (2017) resulted in
Multilevel modeling of the hypotheses
For the main analysis, two multilevel group models were employed, with the two group variables experimental error rate (below human average vs human average vs above human average) and NAOs personality via language cues (dominant vs humble). Since all three hypotheses (H1 to H3) are based on the Error Rate Condition, all three hypotheses were fitted in one model as each provides a different parameter for the model. Then, for the two research questions, another model based on the language cue condition was fitted.
Based on this, first the multilevel group model for the error rate condition was formulated and its fit evaluated. The model is depicted in Figure 2, with the first hypothesis, stating that higher levels of anthropomorphism elicit stronger empathic reactions to a non-human entity, incorporated via the regression path between the exogeneous predictor anthropomorphism and the endogenous variable empathy. The second Hypothesis, proposing that the human average condition is the condition with the highest level of anthropomorphism, leading to the highest level of sympathy, is found as the group level and the regression paths from anthropomorphism directly to sympathy. The last hypothesis stating that higher amounts of anthropomorphism will lead to higher amounts of blame for task related error, modeled as the group level and the corresponding regression paths from the Participants Error Rate to Attribution of Blame.
Robust Maximum Likelihood served as the model estimator for the analysis, with bootstraped standard errors (1,000 Iterations), based on a sample size of N = 78 data points with 3 Groups. The quality of the error condition group model was assessed using various goodness-of-fit indices, including the Model Test User Model (MTUM) and the Model Test 0-Model (MTOT). To evaluate the model’ fit compared to the empirical data, the chi-square statistic (MTUM) was computed. The non-significant result (
The subsequent analysis aimed to assess measurement invariance across groups, specifically scalar invariance. Even though invariance analysis typically seeks a high level of invariance for accurate measurement, invariance is not necessarily expected in pure measurement models (without a latent structural model) (Millsap, 2007). Instead, potential group differences in intercepts and slopes by comparing two models were investigated. The first model, termed the “free model,” allowed all parameters to be freely estimated and vary across groups. The second model, termed the “constrained model”, restricts intercepts and slopes to be the same across groups based on the entire dataset. Therefore the restricted model represents the null hypothesis (no difference in effect between the groups), while model with free parameters represent the alternative hypothesis. If the free and constrained models did not differ significantly and the constrained model fit the data well, the regression coefficients could be considered equivalent across groups, suggesting no group-level differences and negating the need for separate analyses.
The chi-square difference test revealed a significant difference between the free and constrained model (
The first hypothesis targets the proposed core and enduring interaction between anthropomorphism and empathy and postulates a significant effect of anthropomorphism on empathy, thus enabling any empathic reaction toward a non-human entity. The relevant path in the model is the path from anthropomorphism to empathy. For a core connection between two psychological processes, like the one that is proposed in the introduction, there should a) be a general effect of anthropomorphization on empathy, visible through significant path coefficients for each group in the general model, and b) the effect should be independent of the condition, meaning, although that effect can differ in strength between groups, it should never vanish. To proof for this, a model in which only the relevant path is constrained (partially constrained model), is compared to the free and fully constrained model, with the later one as Nullhypothesis. If the hypothesis holds true, the first comparison will yield a non-significant result, while the second comparison will give significant results. Table 2 shows the regression paths, separated by experimental condition. For each group, anthropomorphism was a significant predictor for empathy with a large positive effect, low:
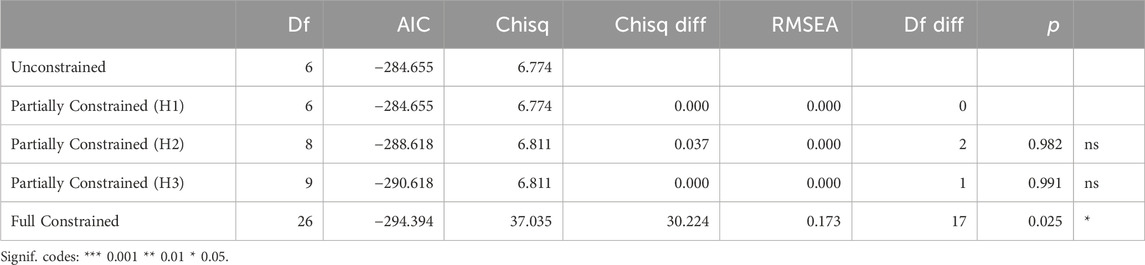
Table 3. Table of model comparison between free, partially and fully constrained models for the error rate condition.
The second hypothesis states, that for the error rate the condition with a human average error rate of the robots should show the highest levels of anthropomorphism and as a result the highest level of sympathy. The regression path from anthropomorphism to sympathy only shows a significant effect for the human average condition, low:
The third hypothesis states, that higher levels of anthropomorphism will lead to higher levels of blame towards the robot for task-related errors. The Analysis revealed a significant effect of Participants task-related errors on Attribution of Blame for the low error rate condition, low:
Multilevel modeling of the research questions
To address the open research questions (RQ1 and RQ2), a path model for the language cue condition was developed (Figure 3). RQ1 investigates how specific language cues influence the persuasive impact and perceived anthropomorphism of robots. RQ2 aims to elucidate how different personality factors modulate the perceived personality of the robot and shape interactions with these task-helping agents. The model builds upon the previously established structure used for the first model, focusing on the expected relationships between anthropomorphism, empathy, and sympathy. Initially, perceived similarity was included as a variable based on the notion that an empathic response towards an interaction partner is necessary to establish a mental model of their personality. As highlighted in the introduction, anthropomorphic cues are crucial for triggering anthropomorphization during interactions with non-human entities. Only then does an empathic response become possible. Consequently, similarity is modeled as a variable predicted by empathy and anthropomorphism, reflecting its dependence on this preceding processes. In addition, we modelled similarity as another predictor for sympathy as well as the persuasive impact. To investigate personality factors it was originally planned to include the Big-5 personality traits in the model. Due to the poor reliability of the questionnaire in this study, it was decided to not incorporate the 5 dimensions into the model. Only the covariates technophilia and technophobia were included as personality traits. While technophilia and technophobia describe behavioral, affective and attitudinal responses to modern technology in general, anthropomorphism is a more basal process not necessarily related to technology. Therefore anthropomorphism, technophilia and technophobia were modelled as exogenous variables, originally predicting similarity, sympathy and persuasion. In addition the dependent variable persuasion is likely to be predicted by similarity, anthropomorphism, sympathy and empathy.
Again Robust Maximum Likelihood served as the model estimator with 1,000 bootstrap iterations for the analysis, this time based on a sample size of N = 78 data points with 2 Groups. The quality of the language cue condition group model was assessed using the same goodness-of-fit indices as before. The MTUM showed a non-significant result (
The testing for group invariance see Table 4 revealed no significant difference between the free and constrained model (

Table 4. Table of model comparison between free and fully constrained models for the language cue condition.
To answer RQ2, as a follow up, path coefficients for the second model without group separation were calculated. Results can be seen in Table 5. The variables anthropomorphism, empathy and sympathy show the same pattern as in the first model, further cementing the hypothesis, that anthropomorphism enables an empathic response to a non-human entity. However, anthropomorphism (
Discussion
This study investigated the interplay between anthropomorphization and empathy during Human-EDT Interaction. We theorized that anthropomorphization serves as a foundational mechanism for empathy, enabling humans to respond empathically to non-human entities by allowing us to use various properties of the observed object as anthropomorphic cues. Prior research predominantly treated anthropomorphization as an outcome variable. In contrast, this study positioned it as the initial predictor within a path model.
Our findings reveal a complex relationship between these variables. H1, positing that higher anthropomorphism leads to stronger empathy, was supported. The path analyses consistently showed a positive relationship between perceived anthropomorphism and empathy across all error rate conditions. This suggests that anthropomorphic cues, regardless of the robot’s performance, can trigger empathic responses in users.
H2, which predicted increased likability with human-like error rates, received partial support. While the human-like error rate condition did elicit higher anthropomorphism than the low error rate condition, the difference in likeability was not statistically significant. This nuances the current literature (Gideoni et al., 2022) suggesting that while human-like error rates increase perceived humanness, they do not necessarily translate into greater likeability. This may be due to users evaluating a pedagogical agent primarily based on its ability to facilitate learning. If errors, even human-like ones, hinder this facilitation, then likeability may not increase. This highlights the importance of context in HRI: the same cues may have different effects depending on the specific task and the user’s goals.
Our findings align with the research by Riek et al. (2009), demonstrating that even subtle cues of human-likeness in robots can trigger empathic responses. However, unlike their study on real-time mimicry, our focus on error rates as an anthropomorphic cue revealed a different dynamic. While errors did increase perceived humanness, they also decreased likability, suggesting a complex interplay between empathy, perceived competence, and social acceptance.
The results for H3, regarding blame attribution, were also mixed. While the low error rate condition did result in less blame attributed to the robot, there was no significant difference in blame between the human-like and high error rate conditions. This suggests a threshold effect: Below a certain level of error, the robot is perceived as less responsible, potentially due to users attributing failures to external factors or programming limitations. However, beyond this threshold, even high error rates that exceed human performance do not proportionally increase blame.
This aligns with the reasoning presented by Waytz et al. (2014). Future research is warranted to definitively elucidate this effect. Additional experimental conditions (including “no errors” and “only errors”) and a wider range of error rates are recommended to achieve clearer differentiation in blame attribution patterns.
RQ1, exploring the influence of language style, did not yield the expected significant results. Despite the significant differences in perceived personality based on language cues found in our pretest, the main experiment showed no effect of language style on anthropomorphism, likability, or persuasion. This may be due to limited cues in each condition, or the scripted, non-adaptive nature of NAO’s communication. The predictability of the robot’s responses might have reduced the persuasive impact of language style (Aly and Tapus, 2013), as participants may have perceived the robot as less human-like and therefore less capable of genuine empathy. It’s also important to consider that NAO’s communication, unlike more advanced large language models (LLMs), lacks the dynamism and flexibility of human speech, potentially limiting the influence of subtle language manipulations. Furthermore, future research may consider different levels of anthropomorphism based on human likeness in physical appearance, displayed behaviour, and type of interaction, as suggested in (Fink, 2012). The limited social expression shown by NAO during the interaction, together with differences in participants’ interpretation of these cues, warrants further investigation. Similarly, following Epley (2018), it is possible that certain aspects of NAO triggered animism or personification rather than anthropomorphism, thus causing empathy to emerge without perceived human likeness. Future research should explore the complex interplay between anthropomorphism, empathy, and persuasion in more detail, potentially considering mediating factors such as trust, perceived credibility, or reactance to robotic influence, as explored by Pelau et al., (2021).
Finally, regarding RQ2, perceived similarity to the robot’s personality did not significantly predict likeability or willingness to use the robot. Technophobia, however, showed a positive relationship with perceived similarity, suggesting that individuals more apprehensive about technology might overestimate their similarity to a robot. Interestingly, empathy was the only positive predictor of persuasion, while anthropomorphism and technophilia negatively predicted it. This apparent contradiction between increased empathy and reduced persuasion with higher anthropomorphism is intriguing. It may reflect a trade-off: While increased anthropomorphism and empathy towards the robot can increase trust and liking in general, they may also increase users’ sensitivity to potential manipulation or persuasion attempts. In other words, people may feel more empathy for a human-like robot but simultaneously become warier of being persuaded by it. This further highlights the dynamic and context-dependent nature of human-robot interaction and raises the question of whether or not a reduction in trust towards highly human-like robots may be related to the uncanny valley effect.
An unexpected challenge arose during the examination of how various personality factors influenced outcomes: selected questionnaires for measuring personality traits (the Big-5 inventory) exhibited unexpectedly low reliability, rendering the collected data unsuitable for analysis. This is notable, as the Big-5 instruments are generally well-validated tools with extensive norming data. The reason for the poor reliability within this study remains unexplained but underscores potential challenges when applying even well-established instruments to novel research contexts.
Besides that, the study has several more limitations. First, the sample size (N = 39) is small, impacting the generalizability and statistical power of the findings. While the observed trends are informative, a larger and more diverse sample is needed to draw firm conclusions. A power analysis, using semPower (Moshagen and Bader, 2024) (AGFI = 0.97,
These insights into the implicit processes that shape human-robot interaction have significant implications for designing effective embodied pedagogical agents, especially within immersive XR learning environments. Our findings suggest that by carefully balancing a robot’s error rate and language style, it may be possible to optimize its perceived humanness, foster empathy, and create a more engaging and productive learning experience. Future research should explore how these dynamics translate to virtual agents in XR, where the cues that trigger anthropomorphism and empathy may differ. Additionally, the role of individual differences in learning preferences and technological affinity should be investigated to personalize agent design and optimize learning outcomes for diverse student populations. To optimize human-robot interaction, particularly in child-centered learning environments, future research should prioritize a deeper understanding of these underlying processes. Developers should be cognizant that the mere technical functionality of an EDT, while essential, is insufficient for success in a social context.
Data availability statement
The original contributions presented in the study are included in the article/supplementary material, further inquiries can be directed to the corresponding authors.
Ethics statement
The studies involving humans were approved by research ethics committee at the Faculty of Humanities, Chemnitz University of Technologies. The studies were conducted in accordance with the local legislation and institutional requirements. The participants provided their written informed consent to participate in this study.
Author contributions
OR: Conceptualization, Data curation, Formal Analysis, Investigation, Methodology, Project administration, Resources, Software, Supervision, Validation, Visualization, Writing–original draft, Writing–review and editing. SJ: Conceptualization, Software, Writing–original draft. MS: Conceptualization, Writing–review and editing. PO: Conceptualization, Funding acquisition, Methodology, Project administration, Supervision, Writing–review and editing.
Funding
The author(s) declare that financial support was received for the research, authorship, and/or publication of this article. This work is funded by the Deutsche Forschungsgemeinschaft (DFG, German Research Foundation)–Project-ID 416228727–CRC 1410.
Acknowledgments
Patricia Krabbes, Marlene Queck and Anthonia Scherf as this research is based on their research for their master thesises.
Conflict of interest
The authors declare that the study was conducted in the absence of any commercial or financial relationships that could be construed as a potential conflict of interest.
Publisher’s note
All claims expressed in this article are solely those of the authors and do not necessarily represent those of their affiliated organizations, or those of the publisher, the editors and the reviewers. Any product that may be evaluated in this article, or claim that may be made by its manufacturer, is not guaranteed or endorsed by the publisher.
References
Airenti, G. (2015). The cognitive bases of anthropomorphism: from relatedness to empathy. Int. J. Soc. Robotics 7, 117–127. doi:10.1007/s12369-014-0263-x
Airenti, G. (2018). The development of anthropomorphism in interaction: intersubjectivity, imagination, and theory of mind. Front. Psychol. 9, 2136. doi:10.3389/fpsyg.2018.02136
Aly, A., and Tapus, A. (2013). “A model for synthesizing a combined verbal and nonverbal behavior based on personality traits in human-robot interaction,” in 2013 8th ACM/IEEE International Conference on Human-Robot Interaction (HRI), Tokyo, Japan, 03-06 March 2013, 325–332. doi:10.1109/HRI.2013.6483606
Alzubi, T. M., Alzubi, J. A., Singh, A., Alzubi, O. A., and Subramanian, M. (2023). A multimodal human-computer interaction for smart learning system. Int. J. Human–Computer Interact. 0, 1–11. doi:10.1080/10447318.2023.2206758
Awang, Z. (2012). A handbook on structural equation modeling using AMOS. Malaysia: Universiti Technologi MARA Press, 83–102.
Arikan, E., Altinigne, N., Kuzgun, E., and Okan, M. (2023)). Robots be held responsible for service failure and recovery? The role of robot service provider agents’ human-likeness. J. Retail. Consumer Serv. 70, 103175. doi:10.1016/j.jretconser.2022.103175
Bagheri, E. (2023). A mini-survey on psychological pillars of empathy for social robots: self-awareness, theory of mind, and perspective taking. Int J Soc Robotics 15, 1227–1241. doi:10.1007/s12369-023-01022-z
Bartneck, C. (2023). “Godspeed questionnaire series: translations and usage,” in International handbook of behavioral health assessment. Editors C. U. Krägeloh, M. Alyami, and O. N. Medvedev (Cham: Springer International Publishing), 1–35. doi:10.1007/978-3-030-89738-3_24-1
Bartneck, C., Kulić, D., Croft, E., and Zoghbi, S. (2009). Measurement instruments for the anthropomorphism, animacy, likeability, perceived intelligence, and perceived safety of robots. Int J Soc Robotics 1, 71–81. doi:10.1007/s12369-008-0001-3
Benk, M., Kerstan, S., Wangenheim, F. v., and Ferrario, A. (2023). Two decades of empirical research on trust in ai: a bibliometric analysis and HCI research agenda. Available at: http://arxiv.org/abs/2309.09828 (Accessed October 11, 2023).
Bommasani, R., Hudson, D. A., Adeli, E., Altman, R., Arora, S., Arx, S. V., et al. (2022). On the opportunities and risks of foundation models. doi:10.48550/arXiv.2108.07258
Brehm, S. S., and Brehm, J. W. (1981). Psychological reactance: a theory of freedom and control. Academic Press. doi:10.1016/C2013-0-10423-0
Byrne, B. M. (1994). Structural equation modeling with EQS and EQS/Windows. Thousand Oaks, CA: Sage Publications.
Del Giudice, M. (2017). Heterogeneity coefficients for Mahalanobis’ D as a multivariate effect size. Multivar. Behav. Res. 52, 216–221. doi:10.1080/00273171.2016.1262237
Dewaele, J.-M., and Furnham, A. (1999). Extraversion: the unloved variable in applied linguistic research. Lang. Learn. 49, 509–544. doi:10.1111/0023-8333.00098
Ekman, P., Friesen, W. V., O’Sullivan, M., and Scherer, K. (1980). Relative importance of face, body, and speech in judgments of personality and affect. J. Personality Soc. Psychol. 38, 270–277. doi:10.1037/0022-3514.38.2.270
Epley, N. (2018). A mind like mine: the exceptionally ordinary underpinnings of anthropomorphism. J. Assoc. Consumer Res. 3, 591–598. doi:10.1086/699516
Fink, J. (2012). Anthropomorphism and human likeness in the design of robots and human-robot interaction. Lect. notes Comput. Sci., 199–208. doi:10.1007/978-3-642-34103-8_20
Furlough, C., Stokes, T., and Gillan, D. J. (2021). Attributing blame to robots: I. The influence of robot autonomy. Hum. Factors 63, 592–602. doi:10.1177/0018720819880641
García-Corretjer, M., Ros, R., Mallol, R., and Miralles, D. (2023). Empathy as an engaging strategy in social robotics: a pilot study. User Model User-Adap Inter 33, 221–259. doi:10.1007/s11257-022-09322-1
Ghazali, A. S., Ham, J., Barakova, E. I., and Markopoulos, P. (2017). “Pardon the rude robot: social cues diminish reactance to high controlling language,” in 2017 26th IEEE International Symposium on Robot and Human Interactive Communication (RO-MAN), Lisbon, Portugal, 28 August 2017 - 01 September 2017, 411–417. doi:10.1109/ROMAN.2017.8172335
Gideoni, R., Honig, S., and Oron-Gilad, T. (2022). Is it personal? The impact of personally relevant robotic failures (PeRFs) on humans’ trust, likeability, and willingness to use the robot. Int J Soc Robotics 16, 1049–1067. doi:10.1007/s12369-022-00912-y
Habler, F., Schwind, V., and Henze, N. (2019). “Effects of smart virtual assistants’ gender and language,” in Proceedings of Mensch und Computer 2019 MuC ’19 (New York, NY, USA: Association for Computing Machinery), 469–473. doi:10.1145/3340764.3344441
Horstmann, A. C., and Krämer, N. C. (2019). Great expectations? Relation of previous experiences with social robots in real life or in the media and expectancies based on qualitative and quantitative assessment. Front. Psychol. 10, 939. doi:10.3389/fpsyg.2019.00939
Hu, C.-C., Yang, Y.-F., and Chen, N.-S. (2023). Human–robot interface design – the “Robot with a Tablet” or “Robot only,” which one is better? Behav. and Inf. Technol. 42, 1590–1603. doi:10.1080/0144929X.2022.2093271
Kim, T., and Hinds, P. (2006). “Who should I blame? Effects of autonomy and transparency on attributions in human-robot interaction,” in ROMAN 2006 - The 15th IEEE International Symposium on Robot and Human Interactive Communication, Hatfield, UK, 06-08 September 2006, 80–85. doi:10.1109/ROMAN.2006.314398
Kratzenberg, M. (2020). Witzige Allgemeinwissens-und Schätzfragen (mit Antworten). Available at: https://www.giga.de/ratgeber/specials/witzige-allgemeinwissens-fragen-lustige-quizfragen-mit-antworten/ (Accessed February 25, 2024).
Kulk, J., and Welsh, J. (2008). “A low power walk for the NAO robot,” in Proceedings of the 2008 Australasian Conference on Robotics and Automation, ACRA 2008, Canberra, Australia, 3-5 December 2008.
Lee, K. M., Peng, W., Jin, S.-A., and Yan, C. (2006). Can robots manifest personality? an empirical test of personality recognition, social responses, and social presence in human–robot interaction. J. Commun. 56, 754–772. doi:10.1111/j.1460-2466.2006.00318.x
Li, B., Blijd-Hoogewys, E., Stockmann, L., Vergari, I., and Rieffe, C. (2023). Toward feeling, understanding, and caring: the development of empathy in young autistic children. Autism 27, 1204–1218. doi:10.1177/13623613221117955
Lüdecke, D., Ben-Shachar, M. S., Patil, I., Wiernik, B. M., Bacher, E., Thériault, R., et al. (2022). Easystats: framework for easy statistical modeling, visualization, and reporting. CRAN. Available at: https://easystats.github.io/easystats/.
Luis, E. O., Martínez, M., Akrivou, K., Scalzo, G., Aoiz, M., and Orón Semper, J. V. (2023). The role of empathy in shared intentionality: contributions from Inter-Processual Self theory. Front. Psychol. 14, 1079950. doi:10.3389/fpsyg.2023.1079950
Mara, M., Appel, M., and Gnambs, T. (2022). Human-like robots and the uncanny valley: a meta-analysis of user responses based on the godspeed scales. Z. für Psychol. 230, 33–46. doi:10.1027/2151-2604/a000486
Martínez-Córcoles, M., Teichmann, M., and Murdvee, M. (2017). Assessing technophobia and technophilia: development and validation of a questionnaire. Technol. Soc. 51, 183–188. doi:10.1016/j.techsoc.2017.09.007
Mileounis, A., Cuijpers, R. H., and Barakova, E. I. (2015). “Creating robots with personality: the effect of personality on social intelligence,” in Artificial Computation in Biology and medicine lecture notes in computer science. Editors J. M. Ferrández Vicente, J. R. Álvarez-Sánchez, F. de la Paz López, F. J. Toledo-Moreo, and H. Adeli (Cham: Springer International Publishing), 119–132. doi:10.1007/978-3-319-18914-7_13
Millsap, R. E. (2007). Invariance in Measurement and Prediction Revisited. Psychometrika 72, 461–473. doi:10.1007/s11336-007-9039-7
Mirnig, N., Stollnberger, G., Miksch, M., Stadler, S., Giuliani, M., and Tscheligi, M. (2017). To Err is robot: how humans assess and act toward an erroneous social robot. Front. Robotics AI 4. doi:10.3389/frobt.2017.00021
Moshagen, M., and Bader, M. (2024). semPower: general power analysis for structural equation models. Behav. Res. 56, 2901–2922. doi:10.3758/s13428-023-02254-7
Nass, C., Moon, Y., Fogg, B., Reeves, B., and Dryer, C. (1995). Can computer personalities be human personalities? Int. J. Hum.-Comput. Stud. 43, 228–229. doi:10.1145/223355.223538
Nielsen, Y. A., Pfattheicher, S., and Keijsers, M. (2022). Prosocial behavior toward machines. Curr. Opin. Psychol. 43, 260–265. doi:10.1016/j.copsyc.2021.08.004
Pelau, C., Dabija, D. C., and Ene, I. (2021). What makes an AI device human-like? The role of interaction quality, empathy and perceived psychological anthropomorphic characteristics in the acceptance of artificial intelligence in the service industry. Comput. Human Behav. 122, 106855. doi:10.1016/j.chb.2021.106855
Putnick, D. L., and Bornstein, M. H. (2016). Measurement invariance conventions and reporting: The state of the art and future directions for psychological research. Dev. Rev. 41, 71–90. doi:10.1016/j.dr.2016.06.004
Ragni, M., Rudenko, A., Kuhnert, B., and Arras, K. O. (2016). “Errare humanum est: erroneous robots in human-robot interaction,” in 2016 25th IEEE International Symposium on Robot and Human Interactive Communication (RO-MAN), New York, NY, USA, 26-31 August 2016, 501–506. doi:10.1109/ROMAN.2016.7745164
R Core Team (2024). R: a language and environment for statistical computing. Vienna, Austria: R Foundation for Statistical Computing. Available at: https://www.R-project.org/.
Reeves, B., and Nass, C. I. (1996). The media equation: how people treat computers, television, and new media like real people and places. New York, NY, US: Cambridge University Press.
Remmstedt, B., and John, O. P. (2007). Measuring personality in one minute or less: a 10-item short version of the Big Five Inventory in English and German. J. Res. Personality 41, 203–212. doi:10.1016/j.jrp.2006.02.001
Revelle, W. (2024). psych: procedures for psychological, psychometric, and personality research. Evanston, Illinois: Northwestern University. Available at: https://CRAN.R-project.org/package=psych.
Riek, L. D., Paul, P. C., and Robinson, P. (2009). When my robot smiles at me: enabling human-robot rapport via real-time head gesture mimicry. J. Multimodal User Interfaces 3, 99–108. doi:10.1007/s12193-009-0028-2
Roesler, E., Manzey, D., and Onnasch, L. (2021). A meta-analysis on the effectiveness of anthropomorphism in human-robot interaction. Sci. Robot. 6, eabj5425. doi:10.1126/scirobotics.abj5425
Rosseel, Y. (2012). lavaan: an R package for structural equation modeling. J. Stat. Softw. 48, 1–36. doi:10.18637/jss.v048.i02
Roubroeks, M., Ham, J., and Midden, C. (2011). When artificial social agents try to persuade people: the role of social agency on the occurrence of psychological reactance. Int J Soc Robotics 3, 155–165. doi:10.1007/s12369-010-0088-1
Salem, M., Lakatos, G., Amirabdollahian, F., and Dautenhahn, K. (2015). “Would you trust a (faulty) robot? Effects of error, task type and personality on human-robot cooperation and trust,” in Proceedings of the tenth annual ACM/IEEE international Conference on human-robot interaction HRI ’15 (New York, NY, USA: Association for Computing Machinery), 141–148. doi:10.1145/2696454.2696497
Shen, L. (2010). On a scale of state empathy during message processing. West. J. Commun. 74, 504–524. doi:10.1080/10570314.2010.512278
Sidera, F., Perpiñà, G., Serrano, J., and Rostan, C. (2018). Why is theory of mind important for referential communication? Curr. Psychol. 37, 82–97. doi:10.1007/s12144-016-9492-5
Tapus, A., Ţăpuş, C., and Matarić, M. J. (2008). User—robot personality matching and assistive robot behavior adaptation for post-stroke rehabilitation therapy. Intel. Serv. Robot. 1, 169–183. doi:10.1007/s11370-008-0017-4
Trovato, G., Lopez, A., Paredes, R., and Cuellar, F. (2017). “Security and guidance: two roles for a humanoid robot in an interaction experiment,” in 2017 26th IEEE International Symposium on Robot and Human Interactive Communication (RO-MAN), Lisbon, Portugal, 28 August 2017 - 01 September 2017, 230–235. doi:10.1109/ROMAN.2017.8172307
van Buren, B., Uddenberg, S., and Scholl, B. J. (2016). The automaticity of perceiving animacy: goal-directed motion in simple shapes influences visuomotor behavior even when task-irrelevant. Psychon. Bull. Rev. 23, 797–802. doi:10.3758/s13423-015-0966-5
Walters, M. L., Dautenhahn, K., te Boekhorst, R., Koay, K. L., Kaouri, C., Woods, S., et al. (2005). “The influence of subjects’ personality traits on personal spatial zones in a human-robot interaction experiment,” in ROMAN 2005. IEEE International Workshop on Robot and Human Interactive Communication, Nashville, TN, USA, 13-15 August 2005, 347–352. doi:10.1109/ROMAN.2005.1513803
Walters, M. L., Syrdal, D. S., Dautenhahn, K., te Boekhorst, R., and Koay, K. L. (2008). Avoiding the uncanny valley: robot appearance, personality and consistency of behavior in an attention-seeking home scenario for a robot companion. Auton. Robot. 24, 159–178. doi:10.1007/s10514-007-9058-3
Waytz, A., Heafner, J., and Epley, N. (2014). The mind in the machine: anthropomorphism increases trust in an autonomous vehicle. J. Exp. Soc. Psychol. 52, 113–117. doi:10.1016/j.jesp.2014.01.005
Wickham, H., Averick, M., Bryan, J., Chang, W., McGowan, L. D., François, R., et al. (2019). Welcome to the tidyverse. J. Open Source Softw. 4, 1686. doi:10.21105/joss.01686
Keywords: empathy, anthropomorphism, pedagogical agent, cooperative learning, embodied digital technologies, personality, error rate, human robot interaction (HRI)
Citation: Rehren O, Jansen S, Seemann M and Ohler P (2025) Task-related errors as a catalyst for empathy towards embodied pedagogical agents. Front. Virtual Real. 5:1412039. doi: 10.3389/frvir.2024.1412039
Received: 04 April 2024; Accepted: 12 December 2024;
Published: 07 January 2025.
Edited by:
Bastian Kordyaka, University of Bremen, GermanyReviewed by:
Xingchen Zhou, Liaoning University, ChinaFabian Clemens Weigend, Arizona State University, United States
Neil Daruwala, University of Portsmouth, United Kingdom
Copyright © 2025 Rehren, Jansen, Seemann and Ohler. This is an open-access article distributed under the terms of the Creative Commons Attribution License (CC BY). The use, distribution or reproduction in other forums is permitted, provided the original author(s) and the copyright owner(s) are credited and that the original publication in this journal is cited, in accordance with accepted academic practice. No use, distribution or reproduction is permitted which does not comply with these terms.
*Correspondence: Oliver Rehren, by5yZWhyZW5AZ21haWwuY29t; Sebastian Jansen, c2ViYXN0aWFuLmphbnNlbkBwaGlsLnR1LWNoZW1uaXR6LmRl