- College of Agronomy and Agricultural Engineering Liaocheng University, Liaocheng, China
Genomic structural variation (SV) refers to differences in gene sequences between individuals on a genomic scale. It is widely distributed in the genome, primarily in the form of insertions, deletions, duplications, inversions, and translocations. Due to its characterization by long segments and large coverage, SVs significantly impact the genetic characteristics and production performance of livestock, playing a crucial role in studying breed diversity, biological evolution, and disease correlation. Research on SVs contributes to an enhanced understanding of chromosome function and genetic characteristics and is important for understanding hereditary diseases mechanisms. In this article, we review the concept, classification, main formation mechanisms, detection methods, and advancement of research on SVs in the genomes of cattle, buffalo, equine, sheep, and goats, aiming to reveal the genetic basis of differences in phenotypic traits and adaptive genetic mechanisms through genomic research, which will provide a theoretical basis for better understanding and utilizing the genetic resources of herbivorous livestock.
1 Introduction
Structural variation (SV) is a major source of genetic diversity among organisms (1, 2) and is defined as differences in DNA segments greater than 50 base pairs between genomes. The SVs play a crucial role in generating significant phenotypic variability among individuals and facilitating evolutionary adaptations (3–5). These variations arise from various genetic processes, including DNA recombination, replication, and repair mechanisms, which lead to changes in the structural configuration of genomic regions (6, 7). Studies using murine models show that SVs significantly contribute to the genetic heterogeneity observed within species populations (8). Additionally, many of these variations are linked to the development of various human diseases, highlighting their importance in medical genetics (9–11).
The development of genomic variation detection technologies has progressed through several stages, including chromosomal karyotyping, fluorescence in situ hybridization (FISH), comparative genomic hybridization (CGH), and microsatellite markers. More recent innovations, such as single nucleotide polymorphism (SNP) chip arrays, array CGH, and high-throughput sequencing technologies, have significantly advanced life sciences. Despite advancements in genomic technologies, accurately detecting SVs in herbivorous livestock remains a significant challenge (1, 12, 13). This difficulty is compounded by lower-quality genome assemblies and incomplete gene annotations, often leading to the misidentification of SVs (1, 13). Understanding SVs is essential in animal genetics, particularly in herbivorous livestock such as cattle and sheep, where SVs are strongly associated with economically significant traits. Substantial evidence suggests that artificial selection has favored advantageous SVs in these species, exemplified by the duplication of the agglutinin signaling protein gene, which is linked to the white coat phenotype in sheep (13). This review provides a comprehensive overview of recent research advancements in the study of SVs—such as copy number variations (CNVs), inversions, and translocations—within the genomes of various livestock species, including cattle, buffalo, equines, sheep, and goats. It also examines how these genomic variants influence key phenotypic traits, such as growth rate, reproductive performance, milk quality, and disease resistance. Through comparative analysis of genomic data across different livestock species, this paper seeks to elucidate the role of SVs in shaping genetic diversity and phenotypic traits, as well as their potential applications in molecular breeding and genetic improvement. Additionally, the review critically assesses current methodologies for detecting and analyzing SVs, highlighting their strengths and limitations in terms of accuracy and resolution. It concludes by proposing future research directions to deepen our understanding of the genetic basis of complex traits in livestock, and to support the sustainable and effective management of livestock genetic resources.
2 Classification of SVs
The SVs are diverse and include types such as insertion, deletion, duplication, inversion, and translocation of genomic segments exceeding 50 base pairs (14). Deletion is the most common type of SV, referring to the removal of a segment of DNA sequence from the genome, resulting in a decrease in the number of bases in the genome. Depending on the location of the DNA sequence deletion, it can be categorized as intermediate or terminal deletion (15). Insertion refers to adding a DNA segment within the genome, resulting in a change in the base sequence at that location. Insertion can be classified into two types: general DNA segment insertion, in which the inserted segment usually originates from the genome, and transposon insertion (16). Transposons are a class of DNA sequences that can move or change positions autonomously within the genome. They occupy a significant portion of the genome and are widespread across various organisms’ genomes. Transposon insertion affects the number of gene copies, gene order, the distance between genes, and the regulation of gene expression (17). When transposons are inserted in gene regulatory regions (e.g., promoters, enhancers, etc.), they may interfere with normal regulatory mechanisms, leading to changes in gene expression levels. Transposon insertion is a significant mechanism of genetic variation within the genome, increasing genomic instability, mutation rates, and genetic diversity. Its effects on the genome are diverse and complex and can influence individuals’ genetic characteristics and disease development. This mechanism plays an important role in the evolutionary process (18–20).
Duplication involves the replication of a DNA segment within the genome, resulting in the presence of two or more copies of that segment. Duplicated DNA segments can vary in length, ranging from 10 to millions of base pairs. Duplication can be further categorized into two types: tandem duplications (21) and interspersed segmental duplications. Tandem duplications occur when the duplicated segments are directly linked to form a tandem structure. These duplications usually result from errors or recombination events during DNA replication. Tandem duplications can further be classified as short tandem duplications (22) or long tandem duplications. Short tandem duplications generally range from a few to a few 100 base pairs, while long tandem duplications can comprise several 1,000 base pairs or even more. Interspersed segmental duplications are repetitive segments that occur multiple times in the genome, but they are separated from each other by other DNA sequences (23). Interspersed segmental duplications can involve both duplications of genes and non-gene sequences (24). Duplications contribute to genome evolution by driving the emergence of new genes and isoforms, thereby increasing functional diversity and promoting evolutionary changes. Further, they can predispose individuals to the onset of certain genetic diseases.
Deletion, insertion, and duplication of genomic fragments longer than 1 kb are classified as CNVs (25). These CNVs are the primary source of genomic SVs (26). The other two categories of SVs, inversion and translocation, involve significant rearrangements, including the relocation of DNA segments between different regions of the genome. SVs can be further divided into balanced and unbalanced events based on the presence of CNVs (14). Unbalanced rearrangements, which include deletions, insertions, and duplications, occur alongside CNVs. In contrast, balanced rearrangements, such as inversions and translocations, involve changes in the order of genomic bases without alterations in CNVs. Distinguishing between these two categories is crucial because the methods used to detect SVs are closely linked to the proportion of genomic sequences that are created or eliminated. In the unbalanced category of SVs, CNVs typically represent a significant portion of the genome. Figure 1 illustrates deletions, insertions, mobile element insertions, tandem repeats, scattered repeats, inversions, and translocations in relation to the reference genome in the test genome (27).
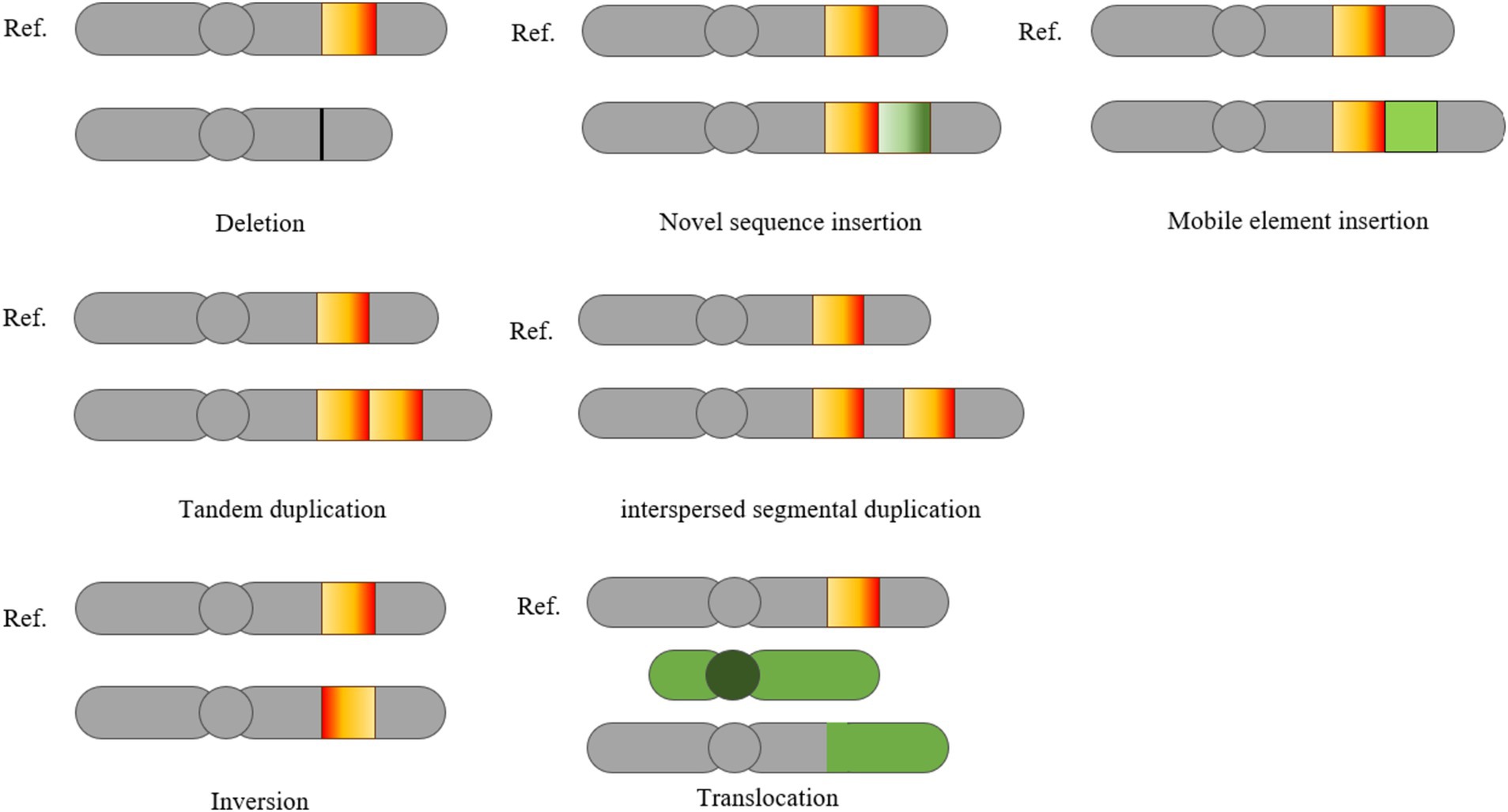
Figure 1. Classification of SVs (27). This schematic illustrates various types of SVs in a test genome compared to the reference genome, including deletions, novel sequence insertions, mobile element insertions, tandem duplication, interspersed segmental duplication, inversions and translocations.
3 Mechanisms of SVs formation in the genome
The mechanisms underlying the formation of SVs can arise from various mutational mechanisms (28), which mainly include mobile-element insertion (MEI), fork stalling and template switching (FoSTeS), non-homologous end joining (NHEJ), and non-allelic homologous recombination (NAHR) (13). These mechanisms, including DNA recombination, replication, and repair, are believed to be responsible for structural alterations in DNA segments, resulting in the creation of SVs within the genome.
3.1 Mobile elements
The MEIs are discrete segments of genomic DNA that can insert new copies elsewhere in the genome via RNA intermediates (29). In humans, the vast majority of MEIs no longer retain the ability to generate new insertions. However, a few MEIs, mainly from the L1, Alu, and SVA families (30), remain active and capable of generating new insertions. Estimates suggest that approximately 1 in every 12 to 14 live births has a de novo MEI (31), making MEIs an endogenous and persistent source of variation in the human genome. They can cause disease by directly disrupting coding sequences or otherwise altering messenger RNAs (mRNAs). For example, the first disease-causing MEI variant identified in humans was the hemizygous variant in F8, which causes hemophilia through loss of function (32). This form of genetic alteration may have significant clinical implications (33).
3.2 Fork stalling and template switching
The FoSTeS is a DNA replication-based mechanism that explains complex genomic rearrangements and CNVs (34). In the process of DNA replication, the DNA double helix is unwound to form two replication forks that move in opposite directions along the DNA strand (35). Fork stalling occurs when one or both of the replication forks encounter an obstacle that impedes their progress or DNA damage (36). Template switching is one mechanism that may occur during fork stalling (37), enabling the continuation of the replication process by switching a stalled fork to a nearby intact DNA template (38, 39). FoSTeS is an important mechanism for DNA replication and repair, ensuring that DNA synthesis can proceed even in the presence of barriers or damage (40). Additionally, changes in the site of replication initiation can lead to duplications or deletions.
3.3 Non-homologous end joining
The NHEJ mechanism serves as a physiological mechanism utilized by cells to repair DNA double-strand breaks induced by ionizing radiation or reactive oxygen species (41). This repair process typically occurs at low-copy repetitive sequences and is closely related to DNA replication (42, 43). The NHEJ-associated proteins are triggered by double-strand breaks in DNA sequences to facilitate the repair and joining of DNA strands (44). Initially, end repair replaces the lost nucleotides at the double-strand break, after which DNA ligase joins the broken DNA fragments together. The joining of segments from different chromosomes can lead to the duplication or deletion of sequences (13).
3.4 Non-allelic homologous recombination
The NAHR produces SVs when a genomic segment exhibits high sequence similarity to a non-allelic locus (13). This recombination can lead to the duplication of similar sites on one chromosome and the corresponding deletion of sites on the other chromosome. The NAHR commonly occurs during meiosis and mitosis because two regions with similar sequences on non-homologous chromosomes are susceptible to recombination (45–47). This process can disrupt genetic information, potentially resulting in abnormal phenotypes. Duplicate elements are often located at the breakpoints of NAHR events that are associated with cancer and various genetic disorders (48–51). Additionally, the process of crossover between sister chromatids may result in the addition or loss of DNA segments, leading to duplications, deletions, and inversions of chromosomal segments (Figure 2).
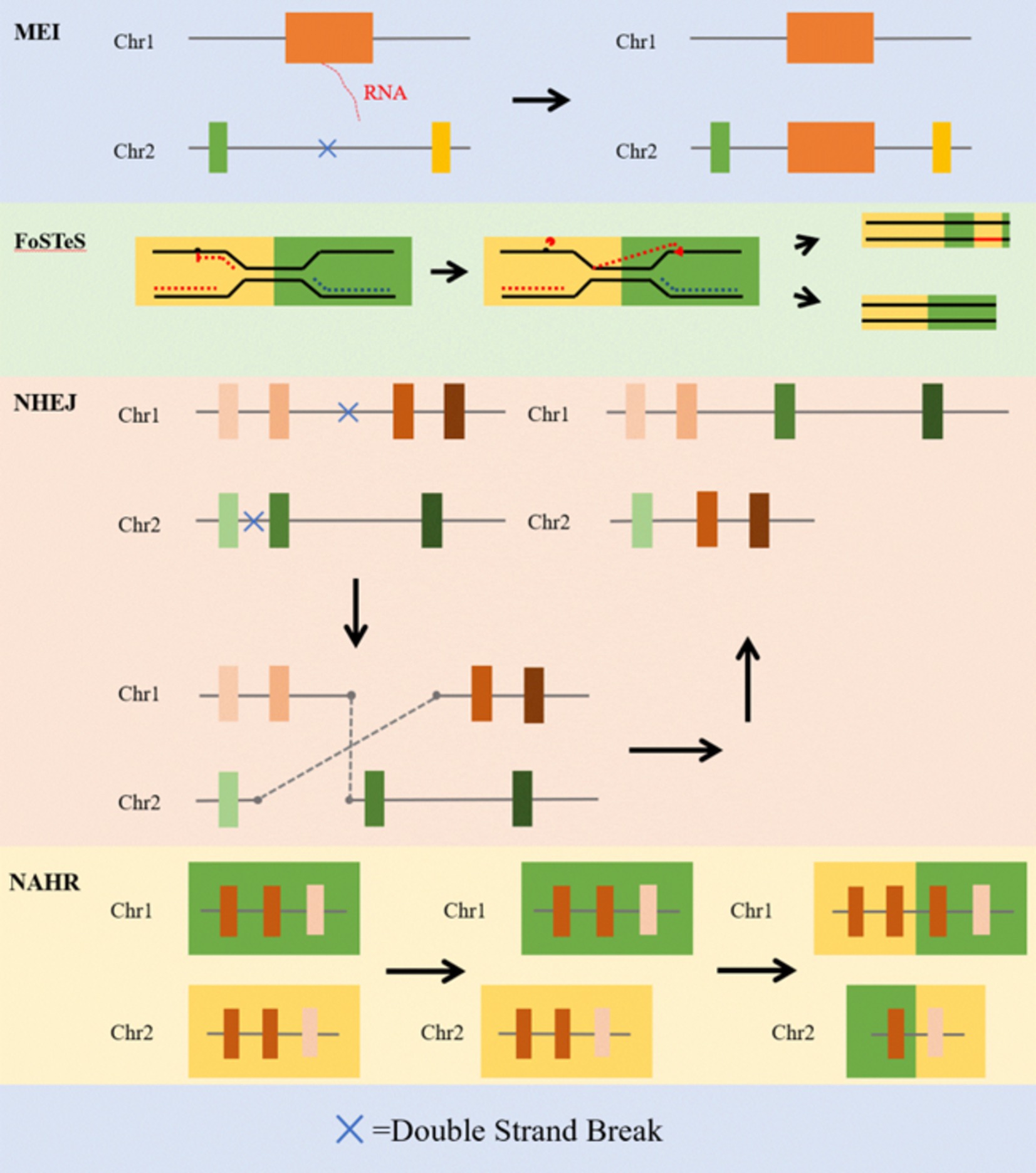
Figure 2. The main formation mechanisms of SV (12). The schematic depicts the process of the main formation mechanisms of SV, including mobile-element insertion (MEI), fork stalling and template switching (FoSTeS), non-homologous end joining (NHEJ) and non-allelic homologous recombination (NAHR).
4 Detection methods for SVs in genome
The unbalanced events are typically detected through the loss or gain of genomic sequence (referred to as “read depth” or RD) (52, 53) or the array probe signal intensity (54, 55) in the affected region when compared to the reference genome. There is a need to identify sequence breakpoints for the detection of balanced events, and methods designed to identify unbalanced SVs from array and sequence data are more sophisticated than those focusing on balanced events (27). Balanced SVs, such as inversions and chromosomal translocations, have the potential to impact the phenotype of an organism but remain particularly challenging to identify as de novo events due to their negligible impact on gene copy numbers (56). Inversions are almost undetectable, with viable detection methods limited to PCR (57) and sequencing (6). Specialized sequencing methods utilizing bipartite sequence data (referred to as “read pairs” or RPs) have subsequently been developed to detect these inversions.
Sanger sequencing technologies offer high accuracy but have low throughput. In contrast, next-generation sequencing technologies excel in cost and throughput, although they have shorter read lengths and higher error rates. Third-generation sequencing technologies provide significant advantages in read lengths but are associated with higher error rates and require more complex data processing (58–61). Several bioinformatics technologies, including RNA-Seq, ChIP-Seq, FAIRE-Seq, ChIA-PET, and Hi-C, utilize next-generation sequencing (NGS), a technology named for its significantly higher throughput compared to first-generation sequencing (58). Presently, Illumina sequencing technology (62) is the most commonly employed, capable of generating 100 of gigabytes or even several terabytes of sequencing data within a matter of hours, thus satisfying the throughput demands of high-throughput sequencing, while ensuring the accuracy of its sequencing. The fundamental principle of Illumina sequencing involves the reversible termination of fluorescently labeled dNTP to facilitate synthesis-while-sequencing (63).
Third-generation sequencing technology, known as single-molecule real-time sequencing technology or de novo sequencing technology, distinguishes itself from previous generations by its primary feature of single-molecule sequencing without the need for PCR amplification, enabling individual sequencing of each DNA molecule. While second-generation short-read sequencing technology currently dominates the sequencing market, the third-generation technology has gained momentum in recent years and has been applied to genome sequencing, methylation research, mutation identification, and other research fields. The primary third-generation sequencing technologies are nanopore electrical signal sequencing and single-molecule fluorescence signal sequencing. Nanopore electrical signal sequencing encompasses single-molecule nanopore DNA sequencing by Oxford Nanopore Technologies (ONT). Single-molecule fluorescence signal sequencing comprises single molecule real-time (SMRT) technology by Pacific Biosciences (PacBio). Among these, the cornerstone of third-generation sequencing is the Nanopore sequencing technology developed by Oxford Nanopore (64, 65). The principle behind Nanopore sequencing involves using a nanopore covalently bound with molecular junctions inside the pore, with nanopore proteins immobilized on a resistive membrane. Kinetic proteins are then used to pull the nucleic acids through the nanopore. As the nucleic acid moves through the nanopore, it causes a change in charge, resulting in a change in the electrical current across the resistive membrane. Due to the small diameter of the nanopore, only a single nucleic acid polymer can pass through, and the charged nature of individual ATCG bases causes varying interference to the current, enabling real-time monitoring and decoding of current signals to determine the base sequence and achieve sequencing (66). Technologies for third-generation sequencing (TGS) can produce read lengths up to 10 of kilobase pairs (kb) or longer, allowing for detailed characterization of complex genomic regions, such as duplications, which are difficult to accurately analyze with short-read sequencing methods. Due to its long-read capability, TGS improves the accuracy of SV breakpoint and type identification, essential for understanding the biological impact of these variations (59). Various methods and tools for SV detection based on third-generation sequencing include PanPop (67), cuteSV (68, 69), cuteSV2 (70), DeBreak (71, 72), DELLY (73–75), and SVision (76).
Optical genome mapping (OGM) is a genome analysis technique that visualizes structural variation by directly imaging ultra-long DNA molecules (77, 78). This OGM technology employs restriction endonucleases and fluorescent markers for labeling DNA, followed by high-resolution imaging to capture the labeling patterns. These patterns reveal structural details across the genome, such as fragment size, position, and relationships. In addition, OGM technology provides advantages in high resolution (79, 80), ultra-long read lengths (79), sensitivity, specificity (80), and PCR-free analysis. However, OGM technology remains relatively new, with limitations in maturity, higher costs, and longer processing times (79). Genome-wide association study (GWAS) involves the detection of genome-wide polymorphisms in multiple individuals to obtain their respective genotypes (81). Subsequently, statistical analysis at the population level is carried out to examine the relationship between the obtained genotypes and the corresponding phenotypes. Genetic variants most likely to influence the trait are filtered based on statistical significance, followed by the identification of genes associated with these trait variants (82). GWAS analysis utilizes two kinds of data: genotypic data, usually in the form of a vcf file, and phenotypic data, typically in the format of a txt file containing sample names and their corresponding trait lists. These genetic markers, derived from these data, can subsequently be utilized for the development of breeding-related test chips or for their value in medical diagnosis (83). While the principles underpinning GWAS for plant and animal breeding and human disease treatment do not significantly differ, the practical applications vary considerably. Consequently, the GWAS process for one species may not be directly transferable to another species (84). By using GWAS analysis, a study identifies structural variants, including insertions, deletions, inversions, and translocations, by comparing sequencing data to a reference genome with software tools such as cuteSV (68, 69), BreakDancer (85), Pindel (86, 87), and SVMerge (88).
5 SVs in livestock genome and their association with phenotypic traits
The importance of genetic variations has been extensively discussed in livestock animals (89, 90). These variations can impact gene expression and regulatory mechanisms, influencing phenotypic traits such as growth rate, milk production, disease resistance, and fertility in various livestock (91–96). Understanding SVs in livestock genomes enhances our ability to predict and manipulate traits, contributing to advancements in agriculture and food security and improved breeding programs and more efficient livestock production. Below, we have discussed the research development on SVs in livestock genomes including cattle, buffalo, equine, sheep and goat.
5.1 SVs in cattle genome and their association with phenotypic traits
Genomic SVs represent an important source of genetic variation in cattle genomes and are commonly linked to phenotypic expressions (97–108). Substantial progress has been made in understanding SVs concerning cattle breed genetic characteristics (109–118) and their associations with essential phenotypes, including feed intake (119), growth traits (120), milk production (121–123), disease resistance or susceptibility (124–127), reproductive health (128–130), coat color patterning (57, 131–133) and environmental adaptability (134, 135) in cattle.
In studies focusing on growth traits, research on the EIF4A2 gene in four cattle breeds—Qinchuan, Yunling, Pinang, and Jiaxian—demonstrated that the EIF4A2-CNV gene significantly influenced hip width and rump length in Qinchuan cattle, heart girth, chest depth, and rump length in Yunling cattle, and hip width in Pinang cattle (136). No significant effect on hip width was observed in Jiaxian cattle, suggesting the potential of EIF4A2 gene SVs as molecular markers in yellow cattle breeding, with implications for enhancing the selection of superior beef breeds (136). Consistently, studies analyzing GWAS data for CNVs and body growth traits in beef cattle have focused on the Nellore breed (120, 137). Using data from over 700,000 SNP probes in 2,230 cattle, CNVs such as EPHB3-CNV98, COL26A-CNV121, GBP6-CNV204, ZNF280B-CNV96, and TSPY-CNV99 were found to be significantly associated with growth traits in the Nellore breed (137). In addition, a CNV100 overlaps with the KCNJ12 gene, was observed to be a key candidate for muscle development (137). Accordingly, a study investigated CNVR in the Brazilian Gir dairy cattle genome, focusing on traits relevant to tropical breeding conditions. By analyzing sequencing and SNP genotyping data from 38 animals, 48 high-confidence CNVR were identified. These regions were associated with genes linked to traits like environmental adaptation, immune response, and reproduction (138).
In relation to milk production, 24,908 high-quality SVs were identified in a cohort of 478 Holstein and Jersey cows through whole-genome sequencing. An interpolation technique estimated 4,489 SVs with an R2 greater than 0.5 in 35,568 Holstein and Jersey cows, utilizing two pipelines: FImpute and Quille2.3-Minimac 3 (139). Their findings further revealed that SVs typically explained less than 10% of the phenotypic variation in key dairy traits, with four SVs significantly associated with these traits (139). Concerning genetic characteristics, Talenti et al. employed optical mapping to construct a high-quality SV database among various cattle breeds from different geographical regions, thereby advancing research on SVs in cattle. Specifically, Bionano optical mapping data at 100X coverage were generated for 18 cattle from nine ancestral lines across three continents and two subspecies. This study identified 13,457 SVs, with 1,200 of which overlapped coding regions (140). In the context of disease resistance and climate adaptation, a comparison of chromosome-scale genome assemblies in two cattle genealogies identified 123,898 non-redundant SVs. Functional studies suggested that a 108 bp exon insertion in the sialophorin (SPN) gene may affect macrophage uptake of Mycobacterium tuberculosis, contributing to the reduced susceptibility of Hainan yellow cattle to bovine tuberculosis (141). In line other studies also reported the association of CNVs with mastitis resistance in Dutch Holstein cattle (142), hoof and reproductive health in Canadian Holstein cattle (143, 144). In addition, research developed a novel SV detection pipeline, identifying millions of deletions, inversions, and duplicated regions in the cattle genome. A deletion variant in the APPL2 gene’s first exon was found to impact gene expression related to immune response, metabolism, and other functions, highlighting its role in selective adaptation across different regions. (145). Furthermore, a study focuses on mapping expression and splicing quantitative trait loci (e/sQTL) to understand phenotypic variability in cattle (146). The researchers created a pangenome using 16 HiFi haplotype-resolved cattle assemblies and genotyped 307 short-read samples, identifying over 21 million small and 43,000 structural variants. They validated 85% of structural variants and mapped e/sQTLs in 117 cattle with testis transcriptome data, identifying 92 structural variants as causal candidates for eQTL and 73 for sQTL. Transposable elements were found to be key contributors to expression and splicing variation. Despite strong linkage disequilibrium between small and structural variants, only 28 additional eQTL and 17 sQTL were discovered (146).
5.2 SVs in buffalo genome and their association with phenotypic traits
The SVs in the buffalo genome and their association with phenotypic traits have been a subject of increasing interest in recent studies. For instance, Ahmad et al. (147) employed a coverage-based approach to generate high-resolution CNV maps of six major buffalo breeds globally using whole-genome resequencing data. By analyzing data at two sequencing coverage levels, 10X and 30X, they detected a total of 14,368 CNVs at 10X coverage and 127,222 CNVs at 30X coverage, with deletions outnumbering duplications in all breeds. At 10X coverage, the Murrah breed exhibited the highest number of CNVs, while the Surti breed had the lowest. Conversely, at 30X coverage, the Pandharpuri breed had the highest CNV count, while the Surti breed retained the lowest (147). Comparison of CNV profiles across these breeds highlighted evolutionary divergences among major buffalo breeds worldwide. This study enhances our understanding of SV in buffaloes and holds promise for applications in selective breeding and genetic improvement efforts (147). In another study, Li et al. characterized genomic differences between the water buffalo genome and the well-studied Bos taurus cattle genome. By comparing whole-genome sequencing datasets of 14 river buffaloes to the cattle reference genome, they identified 13,444 deleted CNV regions and 11,050 merged MEI events located upstream of annotated cattle genes. These findings provide essential data for the functional annotation of genes that may be linked to phenotypic differences between cattle and buffalo, laying the groundwork for future genomic analyses (148). Further advancing the understanding of buffalo genomics, Wang et al. reported a chromosome-level genome assembly with a 72.2 Mb contig N50 and a high-resolution recombination map for male buffalo. Their study revealed that transposable elements (TEs) and SVs have potentially contributed to buffalo evolution by influencing neighboring gene expression. Notably, the pseudoautosomal region (PAR) of the Y chromosome was found to be under strong purifying selection. Additionally, two distinct recombination hotspots were identified on chromosome 8, near genes associated with tooth development, which may enhance buffalo adaptation to low-quality feeds. Additionally, they found that the TE subfamily SINE/tRNAs may play a role in driving recombination into SVs, offering important insights into buffalo genome evolution and adaptation (149). Moreover, Strillacci et al. performed a genome-wide CNV scan on 361 buffaloes from three Iranian river breeds (Azeri, Khuzestani, and Mazandarani), detecting 9,550 CNVs and 302 CNV regions (CNVRs), which encompassed 1.97% of the buffalo genome. Notably, 22 CNVRs were common across all breeds, and 409 genes mapped to CNVRs were linked to traits such as morphology, health, milk production, meat quality, and reproduction, as annotated in the Bovine Genome Database. These results advance our understanding of the natural adaptations and recent environmental pressures faced by buffaloes, particularly in relation to milk production, their primary food source (150). In addition, Li et al. used comparative genomic and transcriptomic analyses to highlight significant structural genomic differences between river buffalo and taurine cattle. These differences may hold important implications for the biology, adaptation, and evolution of the two species, providing a comprehensive understanding of the river buffalo genome. As a result, this research offers a robust framework for future investigations into genetic improvement and disease resistance in buffaloes (151). Deng et al. further expanded the knowledge base by resequencing the genomes of 387 buffaloes from 29 Asian breeds, including river, swamp, and crossbred buffaloes. They identified 36,548 CNVs through the CNV caller, covering 133.29 Mb of the buffalo genome, alongside 2,100 CNVRs, of which 1,993 were shared among the studied breeds. Population differentiation analysis using Vst identified 11 genes significantly differentiated across buffalo breeds, many of which were associated with milk production traits. Furthermore, expression quantitative trait loci (eQTL) analysis revealed differentially expressed CNVR-derived genes (DECGs) linked to milk production. Through a GWAS analysis, three CNVRs were found to be significantly associated with peak milk production. Collectively, this study provides comprehensive genomic insights into buffalo populations, identifying candidate genes for milk production traits that can inform genetic breeding programs aimed at enhancing milk yield and quality in buffaloes (152).
5.3 SVs in sheep and goat genomes and their association with phenotypic traits
SVs in the genomes of sheep and goats have emerged as key contributors to understanding phenotypic traits, especially regarding growth, genetic characteristics, reproduction, and adaptation in sheep and goat (26, 153–165). For example, Jiang et al. examined growth traits by analyzing the CNVs of the Src Homology 2 Domain Containing E (SHE) gene in 750 sheep specimens, including Chaka sheep, Hu sheep, small tail Han sheep, and large tail Han sheep. The study revealed a 2000 bp CNV in the SHE gene. This CNV was associated with traits such as body length, chest width, heart girth, and height at the withers. The study also highlighted breed differences, with deletions in SHE more frequents in Chaka and Hu sheep than in small and large tail Han sheep. The researchers concluded that the CNV of the SHE gene may be a critical factor in sheep molecular breeding, offering insights for improving economic traits through breeding practices (166). Similarly, CNVs have been identified as playing a significant role in goat reproduction. For instance, in a study on highly fertile dairy goats, researchers found that PRP 1 and PRP 6, both associated with the prolactin (PRL) signaling pathway, had repeated copy numbers in highly fertile goats (167). PRP 1 copy numbers were repeated three times, while PRP 6 copy numbers were repeated six times in the high fertility group, contrasting with the normal copy numbers in low fertility goats. These results suggest that the copy number repeats might influence the expression pattern of PRP 1 and PRP 6, though further research is required to clarify the underlying mechanisms (168). In another study, Li et al. performed high-depth resequencing on 16 wild Asian mouflon sheep, 172 local breed specimens, and 60 individuals from various sheep breeds across Asia, Europe, Africa, and Middle East (169). Their analysis identified candidate genes associated with domestication traits like tail fat, horn type, ear size, and other production traits such as wool, milk and meat. This research offered crucial genomic resources for sheep genetics and holds promise for future molecular-assisted breeding efforts (169). Furthermore, a detailed catalog of SVs in sheep was developed using high-quality de novo assemblies, revealing a 168 bp insertion segment in the 5′ untranslated region (5’ UTR) of the Homeobox B13 (HOXB13) gene (170). This specific mutation was linked to the long-tailed trait in sheep through a combination of GWAS and gene expression analyses (170). Additionally, Shi et al. conducted an in-depth analysis of CNVs in Tibetan sheep, comparing local Oula sheep with synthetic Panou sheep, and identified 60,429 CNV events, including 368 differential CNV regions. Of particular interest, the duplication of the ABCB1 gene was suggested as a key factor aiding Panou sheep in adapting to high-altitude environments (171). This research provided an extensive CNV map of Tibetan sheep, serving as a valuable genomic resource for future breeding initiatives (171). Consistently, another study identified a CNVR on chromosome 6, which encompasses the HGFAC and LRPAP1 genes—both of which are associated with fat deposition and environmental adaptability in Iranian fat-tailed breeds (Baluchi and Lori-Bakhtiari sheep) as well as thin-tailed breeds (Zel sheep) (172).
In a large-scale genomic study, Liu et al. (26) identified 6,286 potential CNVs across 1,023 samples from 50 goat breeds, covering approximately 262 Mb or 8.96% of the goat genome. Several noteworthy CNV-overlapping genes, including EDNRA, ADAMTS20, ASIP, and DGAT1, were found to be involved in local adaptations such as coat color, muscle development, metabolic processes, and bone formation. This comprehensive CNV map provides new insights into the functional annotation of the goat genome (26). The findings highlight the significant role of SVs, particularly CNVs, in influencing phenotypic variation, breed-specific traits, and local adaptations in sheep and goats. Moreover, these results serve as a crucial genomic resource for future breeding programs and genetic improvement strategies in these species.
5.4 SVs in equine (horses and donkeys) genomes and their association with phenotypic traits
SVs in equine genomes, particularly in horses and donkeys, have been the focus of recent research due to their potential impact on phenotypic traits. In particular, advances in genome sequencing technologies have facilitated a more detailed exploration of SVs, revealing their associations with traits such as fertility, environmental adaptability, and high-altitude survival. The rapid progress in science and technology has spurred significant growth in the horse and donkey industries, contributing significantly to the field of animal husbandry (173). Consequently, research into SVs within these animals’ genomes holds substantial importance. Equine genome has been investigated for structural variations and their consequent correlation with phenotypic traits (174–178). Similarly, the copy number of five genes located on the donkey’s Y chromosome—CUL4BY, ETSTY1, ETSTY4, ETSTY5, and SRY—was quantified, revealing variability in their copy numbers, which offers essential genetic data for future donkey research (179). Additionally, a chromosome-level Equus kiang genome was assembled using Hi-C sequencing, leading to the identification of SVs potentially linked to high-altitude adaptation, specifically through species-specific insertions and deletions in genes such as PIK3CB and AKT, which are implicated in hypoxia-related pathways (180). Further research identified that while moderate expression levels of equine CUL4BY were found across various tissues, ETSTY1, ETSTY4, and ETSTY5 showed exclusive expression in the testis of horses, though the status of the equine SRY gene as a single-copy gene remains debatable (181). For instance, a study using whole genomes from six diverse horse breeds (Mangalarga Marchador, Percheron, Arabian, Native Mongolian Chakouyi, Tennessee Walking Horse and American Miniature) were sequenced and mapped to the EquCab3.0 genome, generating 1.3 billion reads with coverage between 15x to 24x per horse. After rigorous filtration, they reported 1,923,693 Insertions/Deletions (INDELs), 1,540 CNVs, and 3,321 SVs per horse and functionally annotated. Key genes associated with size variation, such as LCORL (in all horses), ZFAT (in Arabian, American Miniature, and Percheron), and ANKRD1 (in Native Mongolian Chakouyi), were detected. Additionally, a copy number variation in the Latherin gene, linked to thermoregulation by sweating, was found (182). A genome-wide map of CNVs in Chinese local horses identified candidate genes overlapping with CNVRs in Jinjiang horses, uncovering genes linked to hemoglobin binding. This discovery is of particular interest, as it suggests a role in the adaptation of Jinjiang horses to high-temperature and high-humidity environments, providing key insights into the genetic mechanisms underlying equine adaptation to diverse environmental conditions (183). Consequently, Castaneda et al. (184) analyzed CNVs in horse Y chromosome genes using digital droplet PCR, examining 209 normal males, 73 XY horses with disorders of sex development and/or infertility and 5 Przewalski’s horses and 2 kulans. TSPY showed high variability, while SRY copy variations linked to RBMY may cause XY disorders of sex development and/or infertility. The CNVs in TSPY and ETSTY2 differed in cryptorchid cases but not in infertility. They suggested further research to refine Y chromosome assembly and its reproductive implications (184) (Table 1).
6 Limitations and challenges of SVs in herbivorous livestock
The research on SVs is very important in the field of genomics, which involves variations in DNA sequences such as deletions, insertions, duplications, inversions, and translocations within large segments of the genome. These variations have significant implications for gene expression regulation, disease occurrence, and species evolution. However, SVs research faces several limitations and challenges (13), including constraints of sequencing technology, algorithmic and software issues, sample and population coverage, difficulties in functional verification, lack of phenotypic data, environmental and genetic interactions, challenges for breeding applications, technology costs and accessibility, as well as data sharing and standardization.
Conventional sequencing technologies, such as short-read sequencing, have limitations in detecting structural variants in large fragments, as they struggle to capture DNA sequence changes over long distances. While third-generation sequencing technologies, such as PacBio and Nanopore, offer longer read lengths that can improve the accuracy of structural variant detection, they are also more costly and complex to analyze. SV detection requires complex bioinformatics algorithms that must accurately recognize and distinguish between different types of structural variants. Existing algorithms still struggle with highly repetitive sequence regions, which can lead to false-positive or false-negative results.
The population structure of livestock is complex, with significant differences in genetic background between species and populations. This complexity requires researchers to consider the representativeness and diversity of samples in their analyses, as well as how to verify the biological significance of SVs in different populations. Although computational methods can predict SVs, these predictions usually need to be validated through experimental methods such as PCR and FISH, increasing the complexity and cost of the study. Research into the associations between SVs and production traits in livestock requires large amounts of phenotypic data; however, the collection and integration of these data can be time-consuming and costly.
Experimentation on domestic animals must adhere to strict ethical and welfare standards, which may limit certain types of research. Additionally, production traits in herbivorous livestock are influenced not only by genetic factors but also by environmental ones. Understanding these reciprocal effects is crucial for unraveling the biological functions of SVs. Translating findings on structural variants into practical breeding strategies presents many challenges, including the assessment of variant pathogenicity, genetic counseling, and the development of personalized treatment protocols. Although the cost of sequencing technology is decreasing, accessing and analyzing high-throughput sequencing data remains a financial burden for many researchers. The sharing and standardization of SV data are essential for facilitating global research collaboration and improving research efficiency, yet there is a lack of uniform data formats and sharing platforms.
To overcome these limitations and challenges, researchers need to develop new sequencing technologies, improve algorithms, increase computational power, and promote data sharing and standardization. For example, the SVision (76) and SVision-pro (185) algorithms developed by Prof. Kai Ye’s team enhance the accuracy and reduce the false-positive rate in SV detection by transforming the sequence problem into a variation instance segmentation problem in the image space. These efforts will help improve our understanding of SVs in herbivorous livestock and ultimately enhance their production and health.
7 Conclusion
Altogether, we concluded that SVs are a significant source of genetic diversity among individuals. The advent of high-throughput sequencing technology has made genome sequencing of herbivorous livestock more accessible and cost-effective. By comparing genome sequences across different species or individuals, we can identify genomic SVs associated with specific traits. These variations may be linked to important characteristics such as growth rate, reproductive ability, disease resistance, and environmental adaptation. Understanding how these variants affect gene function and expression can help clarify the relationship between genomic SVs and the traits of herbivorous livestock, as well as inform more effective conservation and breeding strategies. Additionally, this research can reveal the evolutionary history and relationships of these animals, enhancing our understanding of their origin and evolution. Both domestic and international studies on genomic SVs in livestock have progressed rapidly, offering valuable insights into the genetic traits, evolutionary history, and population structure of herbivorous livestock.
Future investigations into SVs in livestock genomes should prioritize the development of more efficient and cost-effective long-read sequencing technologies. Such advancements will enhance the accuracy of SV detection, enabling comprehensive studies across large and genetically diverse populations. Additionally, there is a critical need for improved bioinformatics algorithms designed to manage the complexity inherent in genomic regions. These algorithms should aim to minimize sequencing errors and accurately differentiate functional SVs from neutral variations, thereby increasing the reliability of genomic analyses. The expansion of population-scale datasets is essential, along with the establishment of robust data-sharing platforms. These initiatives will facilitate cross-species analyses and comparative genomics, thereby deepening our understanding of SVs across various livestock species. Furthermore, the integration of multi-omics approaches, including transcriptomics and epigenomics, is vital for linking SVs to phenotypic traits. This integration will provide valuable insights into the functional roles of SVs within the context of livestock genetics. Collaborative efforts toward data standardization and the establishment of ethical frameworks are crucial for advancing research and its practical applications in livestock breeding and management.
Author contributions
YC: Conceptualization, Data curation, Formal analysis, Investigation, Methodology, Software, Validation, Visualization, Writing – original draft, Writing – review & editing. MK: Conceptualization, Data curation, Formal analysis, Investigation, Methodology, Software, Supervision, Visualization, Writing – original draft, Writing – review & editing. XW: Data curation, Formal analysis, Investigation, Methodology, Validation, Writing – review & editing. HL: Conceptualization, Data curation, Investigation, Methodology, Validation, Writing – review & editing. WR: Conceptualization, Data curation, Formal analysis, Investigation, Methodology, Software, Validation, Writing – review & editing. XK: Conceptualization, Data curation, Methodology, Software, Validation, Writing – review & editing. XL: Conceptualization, Data curation, Investigation, Writing – review & editing. WC: Conceptualization, Data curation, Investigation, Software, Writing – review & editing. YP: Conceptualization, Data curation, Formal analysis, Investigation, Methodology, Project administration, Resources, Software, Supervision, Validation, Visualization, Writing – original draft, Writing – review & editing. CW: Formal analysis, Investigation, Methodology, Project administration, Resources, Supervision, Validation, Visualization, Writing – original draft, Writing – review & editing, Funding acquisition, Data curation.
Funding
The author(s) declare that financial support was received for the research, authorship, and/or publication of this article. This research was funded by the National Key R&D Pro-gram of China (grant number 2022YFD1600103; 2023YFD1302004), the Shandong Province Modern Agricultural Technology System Donkey Industrial Innovation Team (grant no. SDAIT-27), Live-stock and Poultry Breeding Industry Project of the Ministry of Agriculture and Rural Affairs (grant no. 19211162), The National Natural Science Foundation of China (grant no. 31671287), The Open Project of Liaocheng University Animal Husbandry Discipline (grant no. 319312101–14), The Open Project of Shandong Collaborative Innovation Center for Donkey Industry Technology (grant no. 3193308), Research on Donkey Pregnancy Improvement (grant no. K20LC0901), and Liaocheng University scientific research fund (grant no. 318052025).
Conflict of interest
The authors declare that the research was conducted in the absence of any commercial or financial relationships that could be construed as a potential conflict of interest.
Publisher’s note
All claims expressed in this article are solely those of the authors and do not necessarily represent those of their affiliated organizations, or those of the publisher, the editors and the reviewers. Any product that may be evaluated in this article, or claim that may be made by its manufacturer, is not guaranteed or endorsed by the publisher.
References
1. Pokrovac, I, and Pezer, Ž. Recent advances and current challenges in population genomics of structural variation in animals and plants. Front Genet. (2022) 13:1060898. doi: 10.3389/fgene.2022.1060898
2. Yang, L. A practical guide for structural variation detection in the human genome. Curr Protoc Hum Genet. (2020) 107:e103. doi: 10.1002/cphg.103
3. Hollox, EJ, Zuccherato, LW, and Tucci, S. Genome structural variation in human evolution. Trends Genet. (2022) 38:45–58. doi: 10.1016/j.tig.2021.06.015
4. Ho, SS, Urban, AE, and Mills, RE. Structural variation in the sequencing era. Nat Rev Genet. (2020) 21:171–89. doi: 10.1038/s41576-019-0180-9
5. Laufer, V, Glover, TW, and Wilson, TE. Applications of advanced technologies for detecting genomic structural variation. Mutation Res Rev Mutation Res. (2023) 792:108475. doi: 10.1016/j.mrrev.2023.108475
6. Tuzun, E, Sharp, AJ, Bailey, JA, Kaul, R, Morrison, VA, Pertz, LM, et al. Fine-scale structural variation of the human genome. Nat Genet. (2005) 37:727–32. doi: 10.1038/ng1562
7. Tattini, L, D’Aurizio, R, and Magi, A. Detection of genomic structural variants from next-generation sequencing data. Front Bioeng Biotechnol. (2015) 3:92. doi: 10.3389/fbioe.2015.00092
8. Keane, TM, Wong, K, Adams, DJ, Flint, J, Reymond, A, and Yalcin, B. Identification of structural variation in mouse genomes. Front Genet. (2014) 5:192. doi: 10.3389/fgene.2014.00192
9. Popic, V, Rohlicek, C, Cunial, F, Hajirasouliha, I, Meleshko, D, Garimella, K, et al. Cue: a deep-learning framework for structural variant discovery and genotyping. Nat Methods. (2023) 20:559–68. doi: 10.1038/s41592-023-01799-x
10. Willson, J. Resolving the roles of structural variants. Nat Rev Genet. (2020) 21:507–8. doi: 10.1038/s41576-020-0264-6
11. Levy, S, Sutton, G, Ng, PC, Feuk, L, Halpern, AL, Walenz, BP, et al. The diploid genome sequence of an individual human. PLoS Biol. (2007) 5:e254. doi: 10.1371/journal.pbio.0050254
12. Clop, A, Vidal, O, and Amills, M. Copy number variation in the genomes of domestic animals. Anim Genet. (2012) 43:503–17. doi: 10.1111/j.1365-2052.2012.02317.x
13. Bickhart, DM, and Liu, GE. The challenges and importance of structural variation detection in livestock. Front Genet. (2014) 5:37. doi: 10.3389/fgene.2014.00037
14. Feuk, L, Carson, AR, and Scherer, SW. Structural variation in the human genome. Nat Rev Genet. (2006) 7:85–97. doi: 10.1038/nrg1767
15. Zong, W, Wang, J, Zhao, R, Niu, N, Su, Y, Hu, Z, et al. Associations of genome-wide structural variations with phenotypic differences in cross-bred Eurasian pigs. J Animal Sci Biotechnol. (2023) 14:136. doi: 10.1186/s40104-023-00929-x
16. Jiang, T, Liu, B, Li, J, and Wang, Y. rMETL: sensitive mobile element insertion detection with long read realignment. Bioinformatics. (2019) 35:3484–6. doi: 10.1093/bioinformatics/btz106
17. Lanciano, S, and Cristofari, G. Measuring and interpreting transposable element expression. Nat Rev Genet. (2020) 21:721–36. doi: 10.1038/s41576-020-0251-y
18. Ewing, AD, Smits, N, Sanchez-Luque, FJ, Faivre, J, Brennan, PM, Richardson, SR, et al. Nanopore Sequencing Enables Comprehensive Transposable Element Epigenomic Profiling. Mol Cell. (2020) 80:915–928.e5. doi: 10.1016/j.molcel.2020.10.024
19. Chuong, EB, Elde, NC, and Feschotte, C. Regulatory activities of transposable elements: from conflicts to benefits. Nat Rev Genet. (2017) 18:71–86. doi: 10.1038/nrg.2016.139
20. Kazazian, HH Jr, and Moran, JV. Mobile DNA in health and disease. N Engl J Med. (2017) 377:361–70. doi: 10.1056/NEJMra1510092
21. Redon, R, Ishikawa, S, Fitch, KR, Feuk, L, Perry, GH, Andrews, TD, et al. Global variation in copy number in the human genome. Nature. (2006) 444:444–54. doi: 10.1038/nature05329
22. Sharp, AJ, Locke, DP, McGrath, SD, Cheng, Z, Bailey, JA, Vallente, RU, et al. Segmental duplications and copy-number variation in the human genome. Am J Hum Genet. (2005) 77:78–88. doi: 10.1086/431652
23. Bailey, JA, and Eichler, EE. Primate segmental duplications: crucibles of evolution, diversity and disease. Nat Rev Genet. (2006) 7:552–64. doi: 10.1038/nrg1895
24. Cheng, Z, Ventura, M, She, X, Khaitovich, P, Graves, T, Osoegawa, K, et al. A genome-wide comparison of recent chimpanzee and human segmental duplications. Nature. (2005) 437:88–93. doi: 10.1038/nature04000
25. Conrad, DF, Pinto, D, Redon, R, Feuk, L, Gokcumen, O, Zhang, Y, et al. Origins and functional impact of copy number variation in the human genome. Nature. (2010) 464:704–12. doi: 10.1038/nature08516
26. Liu, M, Zhou, Y, Rosen, BD, Van Tassell, CP, Stella, A, Tosser-Klopp, G, et al. Diversity of copy number variation in the worldwide goat population. Heredity (Edinb). (2019) 122:636–46. doi: 10.1038/s41437-018-0150-6
27. Alkan, C, Coe, BP, and Eichler, EE. Genome structural variation discovery and genotyping. Nat Rev Genet. (2011) 12:363–76. doi: 10.1038/nrg2958
28. Carvalho, CM, and Lupski, JR. Mechanisms underlying structural variant formation in genomic disorders. Nat Rev Genet. (2016) 17:224–38. doi: 10.1038/nrg.2015.25
29. Wardell, GE, Hynes, MF, Young, PJ, and Harrison, E. Why are rhizobial symbiosis genes mobile? Philos Trans R Soc Lond Ser B Biol Sci. (1842) 377:20200471. doi: 10.1098/rstb.2020.0471
30. Devine, SE. Emerging opportunities to study Mobile element insertions and their source elements in an expanding universe of sequenced human genomes. Genes (Basel). (2023) 14:1923. doi: 10.3390/genes14101923
31. Gardner, EJ, Prigmore, E, Gallone, G, Danecek, P, Samocha, KE, Handsaker, J, et al. Contribution of retrotransposition to developmental disorders. Nat Commun. (2019) 10:4630. doi: 10.1038/s41467-019-12520-y
32. Kazazian, HH, Wong, C, Youssoufian, H, Scott, AF, Phillips, DG, and Antonarakis, SE. Haemophilia a resulting from de novo insertion of L1 sequences represents a novel mechanism for mutation in man. Nature. (1988) 332:164–6. doi: 10.1038/332164a0
33. Durrant, MG, Li, MM, Siranosian, BA, Montgomery, SB, and Bhatt, AS. A Bioinformatic analysis of integrative Mobile genetic elements highlights their role in bacterial adaptation. Cell Host Microbe. (2020) 27:140–153.e9. doi: 10.1016/j.chom.2019.10.022
34. Cocquempot, O, Brault, V, Babinet, C, and Herault, Y. Fork stalling and template switching as a mechanism for polyalanine tract expansion affecting the DYC mutant of HOXD13, a new murine model of synpolydactyly. Genetics. (2009) 183:23–30. doi: 10.1534/genetics.109.104695
35. Leman, AR, and Noguchi, E. The replication fork: understanding the eukaryotic replication machinery and the challenges to genome duplication. Genes (Basel). (2013) 4:1–32. doi: 10.3390/genes4010001
36. Burssed, B, Zamariolli, M, Bellucco, FT, and Melaragno, MI. Mechanisms of structural chromosomal rearrangement formation. Mol Cytogenet. (2022) 15:23. doi: 10.1186/s13039-022-00600-6
37. Carvalho, CM, Zhang, F, Liu, P, Patel, A, Sahoo, T, Bacino, CA, et al. Complex rearrangements in patients with duplications of MECP2 can occur by fork stalling and template switching. Hum Mol Genet. (2009) 18:2188–203. doi: 10.1093/hmg/ddp151
38. Seo, SH, Bacolla, A, Yoo, D, Koo, YJ, Cho, SI, Kim, MJ, et al. Replication-based rearrangements are a common mechanism for SNCA duplication in Parkinson's disease. Mov Disord. (2020) 35:868–76. doi: 10.1002/mds.27998
39. Haddock, J, and Domyan, ET. A DNA replication mechanism can explain structural variation at the pigeon recessive red locus. Biomol Ther. (2022) 12:1509. doi: 10.3390/biom12101509
40. Lee, JA, Carvalho, CM, and Lupski, JR. A DNA replication mechanism for generating nonrecurrent rearrangements associated with genomic disorders. Cell. (2007) 131:1235–47. doi: 10.1016/j.cell.2007.11.037
41. Zhao, B, Rothenberg, E, Ramsden, DA, and Lieber, MR. The molecular basis and disease relevance of non-homologous DNA end joining. Nat Rev Mol Cell Biol. (2020) 21:765–81. doi: 10.1038/s41580-020-00297-8
42. Feng, W, Smith, CM, Simpson, DA, and Gupta, GP. Targeting non-homologous and alternative end joining repair to enhance Cancer Radiosensitivity. Semin Radiat Oncol. (2022) 32:29–41. doi: 10.1016/j.semradonc.2021.09.007
43. Stankiewicz, P, and Lupski, JR. Molecular-evolutionary mechanisms for genomic disorders. Curr Opin Genet Dev. (2002) 12:312–9. doi: 10.1016/S0959-437X(02)00304-0
44. Audoynaud, C, Vagner, S, and Lambert, S. Non-homologous end-joining at challenged replication forks: an RNA connection? Trends Genet. (2021) 37:973–85. doi: 10.1016/j.tig.2021.06.010
45. Chen, JM, Cooper, DN, Chuzhanova, N, Férec, C, and Patrinos, GP. Gene conversion: mechanisms, evolution and human disease. Nat Rev Genet. (2007) 8:762–75. doi: 10.1038/nrg2193
46. Piazza, A, and Heyer, WD. Moving forward one step back at a time: reversibility during homologous recombination. Curr Genet. (2019) 65:1333–40. doi: 10.1007/s00294-019-00995-7
47. Savocco, J, and Piazza, A. Recombination-mediated genome rearrangements. Curr Opin Genet Dev. (2021) 71:63–71. doi: 10.1016/j.gde.2021.06.008
48. Beck, CR, Garcia-Perez, JL, Badge, RM, and Moran, JV. LINE-1 elements in structural variation and disease. Annu Rev Genomics Hum Genet. (2011) 12:187–215. doi: 10.1146/annurev-genom-082509-141802
49. Kundaje, A, Meuleman, W, Ernst, J, Bilenky, M, Yen, A, Heravi-Moussavi, A, et al. Integrative analysis of 111 reference human epigenomes. Nature. (2015) 518:317–30. doi: 10.1038/nature14248
50. Zhang, F, Gu, W, Hurles, ME, and Lupski, JR. Copy number variation in human health, disease, and evolution. Annu Rev Genomics Hum Genet. (2009) 10:451–81. doi: 10.1146/annurev.genom.9.081307.164217
51. Pascarella, G, Hon, CC, Hashimoto, K, Busch, A, Luginbühl, J, Parr, C, et al. Recombination of repeat elements generates somatic complexity in human genomes. Cell. (2022) 185:3025–3040.e6. doi: 10.1016/j.cell.2022.06.032
52. Alkan, C, Kidd, JM, Marques-Bonet, T, Aksay, G, Antonacci, F, Hormozdiari, F, et al. Personalized copy number and segmental duplication maps using next-generation sequencing. Nat Genet. (2009) 41:1061–7. doi: 10.1038/ng.437
53. Sudmant, PH, Kitzman, JO, Antonacci, F, Alkan, C, Malig, M, Tsalenko, A, et al. Shendure J; 1000 genomes project; Eichler EE. Diversity of human copy number variation and multicopy genes. Science. (2010) 330:641–6. doi: 10.1126/science.1197005
54. Lockwood, WW, Chari, R, Chi, B, and Lam, WL. Recent advances in array comparative genomic hybridization technologies and their applications in human genetics. Eur J Hum Genet. (2006) 14:139–48. doi: 10.1038/sj.ejhg.5201531
55. Wang, K, Li, M, Hadley, D, Liu, R, Glessner, J, Grant, SF, et al. PennCNV: an integrated hidden Markov model designed for high-resolution copy number variation detection in whole-genome SNP genotyping data. Genome Res. (2007) 17:1665–74. doi: 10.1101/gr.6861907
56. Durkin, K, Coppieters, W, Drögemüller, C, Ahariz, N, Cambisano, N, Druet, T, et al. Serial translocation by means of circular intermediates underlies colour sidedness in cattle. Nature. (2012) 482:81–4. doi: 10.1038/nature10757
57. Liu, J, Liu, Q, Liang, Y, Wang, L, Nozary, G, Xiao, B, et al. PCR assay for the inversion causing severe hemophilia a and its application. Chin Med J. (1999) 112:419–23.
58. Slatko, BE, Gardner, AF, and Ausubel, FM. Overview of next-generation sequencing technologies. Curr Protoc Mol Biol. (2018) 122:e59. doi: 10.1002/cpmb.59
59. Athanasopoulou, K, Boti, MA, Adamopoulos, PG, Skourou, PC, and Scorilas, A. Third-generation sequencing: the spearhead towards the radical transformation of modern genomics. Life (Basel). (2021) 12:30. doi: 10.3390/life12010030
60. Al-Shuhaib, MBS, and Hashim, HO. Mastering DNA chromatogram analysis in sanger sequencing for reliable clinical analysis. J Genet Eng Biotechnol. (2023) 21:115. doi: 10.1186/s43141-023-00587-6
61. Satam, H, Joshi, K, Mangrolia, U, Waghoo, S, Zaidi, G, Rawool, S, et al. Next-generation sequencing technology: current trends and advancements. Biology (Basel). (2023) 12:997. doi: 10.3390/biology12070997
62. Jeon, SA, Park, JL, Park, SJ, Kim, JH, Goh, SH, Han, JY, et al. Comparison between MGI and Illumina sequencing platforms for whole genome sequencing. Genes Genomics. (2021) 43:713–24. doi: 10.1007/s13258-021-01096-x
63. Modi, A, Vai, S, Caramelli, D, and Lari, M. The Illumina sequencing protocol and the NovaSeq 6000 system. Methods Mol Biol. (2021) 2242:15–42. doi: 10.1007/978-1-0716-1099-2_2
64. Deamer, D, Akeson, M, and Branton, D. Three decades of nanopore sequencing. Nat Biotechnol. (2016) 34:518–24. doi: 10.1038/nbt.3423
65. Jain, M, Olsen, HE, Paten, B, and Akeson, M. The Oxford Nanopore MinION: delivery of nanopore sequencing to the genomics community. Genome Biol. (2016) 17:239. doi: 10.1186/s13059-016-1103-0
66. Wang, Y, Zhao, Y, Bollas, A, Wang, Y, and Au, KF. Nanopore sequencing technology, bioinformatics and applications. Nat Biotechnol. (2021) 39:1348–65. doi: 10.1038/s41587-021-01108-x
67. Zheng, Z, Zhu, M, Zhang, J, Liu, X, Hou, L, Liu, W, et al. A sequence-aware merger of genomic structural variations at population scale. Nat Commun. (2024) 15:960. doi: 10.1038/s41467-024-45244-9
68. Jiang, T, Liu, Y, Jiang, Y, Li, J, Gao, Y, Cui, Z, et al. Long-read-based human genomic structural variation detection with cuteSV. Genome Biol. (2020) 21:189. doi: 10.1186/s13059-020-02107-y
69. Jiang, T, Liu, S, Cao, S, and Wang, Y. Structural variant detection from Long-read sequencing data with cuteSV. Methods Mol Biol. (2022) 2493:137–51. doi: 10.1007/978-1-0716-2293-3_9
70. Liu, Z, Xie, Z, and Li, M. Comprehensive and deep evaluation of structural variation detection pipelines with third-generation sequencing data. Genome Biol. (2024) 25:188. doi: 10.1186/s13059-024-03324-5
71. Chen, Y, Wang, AY, Barkley, CA, Zhang, Y, Zhao, X, Gao, M, et al. Deciphering the exact breakpoints of structural variations using long sequencing reads with DeBreak. Nat Commun. (2023) 14:283. doi: 10.1038/s41467-023-35996-1
72. Liu, YH, Luo, C, Golding, SG, Ioffe, JB, and Zhou, XM. Tradeoffs in alignment and assembly-based methods for structural variant detection with long-read sequencing data. Nat Commun. (2024) 15:2447. doi: 10.1038/s41467-024-46614-z
73. Rausch, T, Zichner, T, Schlattl, A, Stütz, AM, Benes, V, and Korbel, JO. DELLY: structural variant discovery by integrated paired-end and split-read analysis. Bioinformatics. (2012) 28:i333–9. doi: 10.1093/bioinformatics/bts378
74. Ma, C, Shi, X, Li, X, Zhang, YP, and Peng, MS. Comprehensive evaluation and guidance of structural variation detection tools in chicken whole genome sequence data. BMC Genomics. (2024) 25:970. doi: 10.1186/s12864-024-10875-1
75. Ahsan, MU, Liu, Q, Perdomo, JE, Fang, L, and Wang, K. A survey of algorithms for the detection of genomic structural variants from long-read sequencing data. Nat Methods. (2023) 20:1143–58. doi: 10.1038/s41592-023-01932-w
76. Lin, J, Wang, S, Audano, PA, Meng, D, Flores, JI, Kosters, W, et al. SVision: a deep learning approach to resolve complex structural variants. Nat Methods. (2022) 19:1230–3. doi: 10.1038/s41592-022-01609-w
77. Yuan, Y, Chung, CY, and Chan, TF. Advances in optical mapping for genomic research. Comput Struct Biotechnol J. (2020) 18:2051–62. doi: 10.1016/j.csbj.2020.07.018
78. Hao, N, Zhou, J, Li, MM, Luo, WW, Zhang, HZ, Qi, QW, et al. Efficacy and initial clinical evaluation of optical genome mapping in the diagnosis of structural variations. Zhonghua Yu Fang Yi Xue Za Zhi. (2022) 56:632–9. doi: 10.3760/cma.j.cn112150-20220212-00131
79. Dremsek, P, Schwarz, T, Weil, B, Malashka, A, Laccone, F, and Neesen, J. Optical genome mapping in routine human genetic diagnostics-its advantages and limitations. Genes (Basel). (2021) 12:1958. doi: 10.3390/genes12121958
80. Garcia-Heras, J. Optical genome mapping: a revolutionary tool for "next generation Cytogenomics analysis" with a broad range of diagnostic applications in human diseases. J Assoc Genet Technol. (2021) 47:191–200. doi: 10.3390/genes12030398
81. Dehghan, A. Genome-wide association studies. Methods Mol Biol. (2018) 1793:37–49. doi: 10.1007/978-1-4939-7868-7_4
82. Yoosefzadeh-Najafabadi, M, Eskandari, M, Belzile, F, and Torkamaneh, D. Genome-wide association study statistical models: a review. Methods Mol Biol. (2022) 2481:43–62. doi: 10.1007/978-1-0716-2237-7_4
83. Long, E, Patel, H, Byun, J, Amos, CI, and Choi, J. Functional studies of lung cancer GWAS beyond association. Hum Mol Genet. (2022) 31:R22–36. doi: 10.1093/hmg/ddac140
84. Abdellaoui, A, Yengo, L, Verweij, KJH, and Visscher, PM. 15 years of GWAS discovery: realizing the promise. Am J Hum Genet. (2023) 110:179–94. doi: 10.1016/j.ajhg.2022.12.011
85. Chen, K, Wallis, JW, McLellan, MD, Larson, DE, Kalicki, JM, Pohl, CS, et al. BreakDancer: an algorithm for high-resolution mapping of genomic structural variation. Nat Methods. (2009) 6:677–81. doi: 10.1038/nmeth.1363
86. Ye, K, Schulz, MH, Long, Q, Apweiler, R, and Ning, Z. Pindel: a pattern growth approach to detect break points of large deletions and medium sized insertions from paired-end short reads. Bioinformatics. (2009) 25:2865–71. doi: 10.1093/bioinformatics/btp394
87. Ye, K, Guo, L, Yang, X, Lamijer, EW, Raine, K, and Ning, Z. Split-read Indel and structural variant calling using PINDEL. Methods Mol Biol. (2018) 1833:95–105. doi: 10.1007/978-1-4939-8666-8_7
88. Wong, K, Keane, TM, Stalker, J, and Adams, DJ. Enhanced structural variant and breakpoint detection using SVMerge by integration of multiple detection methods and local assembly. Genome Biol. (2010) 11:R128. doi: 10.1186/gb-2010-11-12-r128
89. Khan, MZ, Chen, W, Huang, B, Liu, X, Wang, X, Liu, Y, et al. Advancements in genetic marker exploration for livestock vertebral traits with a focus on China. Animals. (2024) 14:594. doi: 10.3390/ani14040594
90. Liu, X, Chen, W, Huang, B, Wang, X, Peng, Y, Zhang, X, et al. Advancements in copy number variation screening in herbivorous livestock genomes and their association with phenotypic traits. Front Vet Sci. (2023) 10:10. doi: 10.3389/fvets.2023.1334434
91. Ben-Jemaa, S, Boussaha, M, Mandonnet, N, Bardou, P, and Naves, M. Uncovering structural variants in creole cattle from Guadeloupe and their impact on environmental adaptation through whole genome sequencing. PLoS One. (2024) 19:e0309411. doi: 10.1371/journal.pone.0309411
92. Li, X, Liu, Q, Fu, C, Li, M, Li, C, Li, X, et al. Characterizing structural variants based on graph-genotyping provides insights into pig domestication and local adaption. J Genet Genomics. (2024) 51:394–406. doi: 10.1016/j.jgg.2023.11.005
93. Liang, X, Duan, Q, Li, B, Wang, Y, Bu, Y, Zhang, Y, et al. Genomic structural variation contributes to evolved changes in gene expression in high-altitude Tibetan sheep. Proc Natl Acad Sci. (2024) 121:e2322291121. doi: 10.1073/pnas.2322291121
94. Bhati, M, Mapel, XM, Lloret-Villas, A, and Pausch, H. Structural variants and short tandem repeats impact gene expression and splicing in bovine testis tissue. Genetics. (2023) 225:iyad161. doi: 10.1093/genetics/iyad161
95. Nguyen, TV, Vander Jagt, CJ, Wang, J, Daetwyler, HD, Xiang, R, Goddard, ME, et al. In it for the long run: perspectives on exploiting long-read sequencing in livestock for population scale studies of structural variants. Genet Sel Evol. (2023) 55:9. doi: 10.1186/s12711-023-00783-5
96. Di Gerlando, R, Mastrangelo, S, Moscarelli, A, Tolone, M, Sutera, AM, Portolano, B, et al. Genomic structural diversity in local goats: analysis of copy-number variations. Animals. (2020) 10:1040. doi: 10.3390/ani10061040
97. Delledonne, A, Punturiero, C, Ferrari, C, Bernini, F, Milanesi, R, Bagnato, A, et al. Copy number variant scan in more than four thousand Holstein cows bred in Lombardy, Italy. PLoS One. (2024) 19:e0303044. doi: 10.1371/journal.pone.0303044
98. Lee, S, Clémentine, C, and Kim, H. Exploring the genetic factors behind the discrepancy in resistance to bovine tuberculosis between African zebu cattle and European taurine cattle. Sci Rep. (2024) 14:2370. doi: 10.1038/s41598-024-52606-2
99. Demir, E, Moravčíková, N, Kaya, S, Kasarda, R, Doğru, H, Bilginer, Ü, et al. Genome-wide genetic variation and population structure of native and cosmopolitan cattle breeds reared in Türkiye. Anim Biotechnol. (2023) 34:1–10. doi: 10.1080/10495398.2023.2235600
100. Lee, YL, Bosse, M, Takeda, H, Moreira, GC, Karim, L, Druet, T, et al. High-resolution structural variants catalogue in a large-scale whole genome sequenced bovine family cohort data. BMC Genomics. (2023) 24:225. doi: 10.1186/s12864-023-09259-8
101. Singh, VK, Singh, S, Nandhini, PB, Bhatia, AK, Dixit, SP, and Ganguly, I. Comparative genomic diversity analysis of copy number variations (CNV) in indicine and taurine cattle thriving in Europe and Indian subcontinent. Anim Biotechnol. (2023) 34:3483–94. doi: 10.1080/10495398.2022.2162910
102. Vostry, L, Vostra-Vydrova, H, Moravcikova, N, Kasarda, R, Cubric-Curik, V, Brzakova, M, et al. Genomic diversity and population structure of the Czech Holstein cattle. Livest Sci. (2023) 273:105261. doi: 10.1016/j.livsci.2023.105261
103. Martinez, R, Bejarano, D, Ramírez, J, Ocampo, R, Polanco, N, Perez, JE, et al. Genomic variability and population structure of six Colombian cattle breeds. Trop Anim Health Prod. (2023) 55:185. doi: 10.1007/s11250-023-03574-8
104. Peripolli, E, Stafuzza, NB, Machado, MA, Do Carmo Panetto, JC, Do Egito, AA, Baldi, F, et al. Assessment of copy number variants in three Brazilian locally adapted cattle breeds using whole-genome re-sequencing data. Anim Genet. (2023) 54:254–70. doi: 10.1111/age.13298
105. Salomon-Torres, R, Matukumalli, LK, Van Tassell, CP, Villa-Angulo, C, Gonzalez-Vizcarra, VM, and Villa-Angulo, R. High density LD-based structural variations analysis in cattle genome. PLoS One. (2014) 9:e103046. doi: 10.1371/journal.pone.0103046
106. Fadista, J, Thomsen, B, Holm, L-E, and Bendixen, C. Copy number variation in the bovine genome. BMC Genomics. (2010) 11:284. doi: 10.1186/1471-2164-11-284
107. Liu, GE, Hou, Y, Zhu, B, Cardone, MF, Jiang, L, Cellamare, A, et al. Analysis of copy number variations among diverse cattle breeds. Genome Res. (2010) 20:693–703. doi: 10.1101/gr.105403.110
108. Liu, GE, Ventura, M, Cellamare, A, Chen, L, Cheng, Z, Zhu, B, et al. Analysis of recent segmental duplications in the bovine genome. BMC Genomics. (2009) 10:571. doi: 10.1186/1471-2164-10-571
109. Grant, JR, Herman, EK, Barlow, LD, Miglior, F, Schenkel, FS, Baes, CF, et al. A large structural variant collection in Holstein cattle and associated database for variant discovery, characterization, and application. BMC Genomics. (2024) 25:903. doi: 10.1186/s12864-024-10812-2
110. Low, WY, Tearle, R, Liu, R, Koren, S, Rhie, A, Bickhart, DM, et al. Haplotype-resolved genomes provide insights into structural variation and gene content in Angus and Brahman cattle. Nat Commun. (2020) 11:2071. doi: 10.1038/s41467-020-15848-y
111. Boussaha, M, Esquerré, D, Barbieri, J, Djari, A, Pinton, A, Letaief, R, et al. Genome-wide study of structural variants in bovine Holstein, Montbéliarde and Normande dairy breeds. PLoS One. (2015) 10:e0135931. doi: 10.1371/journal.pone.0135931
112. Canavez, FC, Luche, DD, Stothard, P, Leite, KRM, Sousa-Canavez, JM, Plastow, G, et al. Genome sequence and assembly of Bos indicus. J Hered. (2012) 103:342–8. doi: 10.1093/jhered/esr153
113. Bickhart, DM, Hou, Y, Schroeder, SG, Alkan, C, Cardone, MF, Matukumalli, LK, et al. Copy number variation of individual cattle genomes using next-generation sequencing. Genome Res. (2012) 22:778–90. doi: 10.1101/gr.133967.111
114. Choi, J-W, Lee, K-T, Liao, X, Stothard, P, An, H-S, Ahn, S, et al. Genome-wide copy number variation in Hanwoo, black Angus, and Holstein cattle. Mamm Genome. (2013) 24:151–63. doi: 10.1007/s00335-013-9449-z
115. Koch, CT, Bruggmann, R, Tetens, J, and Drögemüller, C. A non-coding genomic duplication at the HMX1 locus is associated with crop ears in highland cattle. PLoS One. (2013) 8:e77841. doi: 10.1371/journal.pone.0077841
116. Stothard, P, Choi, J-W, Basu, U, Sumner-Thomson, JM, Meng, Y, Liao, X, et al. Whole genome resequencing of black Angus and Holstein cattle for SNP and CNV discovery. BMC Genomics. (2011) 12:559. doi: 10.1186/1471-2164-12-559
117. Zhan, B, Fadista, J, Thomsen, B, Hedegaard, J, Panitz, F, and Bendixen, C. Global assessment of genomic variation in cattle by genome resequencing and high-throughput genotyping. BMC Genomics. (2011) 12:557. doi: 10.1186/1471-2164-12-557
118. Kumar, H, Panigrahi, M, Saravanan, KA, Rajawat, D, Parida, S, Bhushan, B, et al. Genome-wide detection of copy number variations in Tharparkar cattle. Anim Biotechnol. (2023) 34:448–55. doi: 10.1080/10495398.2021.1942027
119. Hou, Y, Bickhart, DM, Chung, H, Hutchison, JL, Norman, HD, Connor, EE, et al. Analysis of copy number variations in Holstein cows identify potential mechanisms contributing to differences in residual feed intake. Funct Integr Genomics. (2012) 12:717–23. doi: 10.1007/s10142-012-0295-y
120. Benfica, LF, Brito, LF, Do Bem, RD, Mulim, HA, Glessner, J, Braga, LG, et al. Genome-wide association study between copy number variation and feeding behavior, feed efficiency, and growth traits in Nellore cattle. BMC Genomics. (2024) 25:54. doi: 10.1186/s12864-024-09976-8
121. Kadri, NK, Sahana, G, Charlier, C, Iso-Touru, T, Guldbrandtsen, B, Karim, L, et al. A 660-kb deletion with antagonistic effects on fertility and milk production segregates at high frequency in Nordic red cattle: additional evidence for the common occurrence of balancing selection in livestock. PLoS Genet. (2014) 10:e1004049. doi: 10.1371/journal.pgen.1004049
122. Xu, L, Cole, JB, Bickhart, DM, Hou, Y, Song, J, VanRaden, PM, et al. Genome wide CNV analysis reveals additional variants associated with milk production traits in Holsteins. BMC Genomics. (2014) 15:683. doi: 10.1186/1471-2164-15-683
123. Lee, H-J, Kim, J, Lee, T, Son, JK, Yoon, H-B, Baek, KS, et al. Deciphering the genetic blueprint behind Holstein milk proteins and production. Genome Biol Evol. (2014) 6:1366–74. doi: 10.1093/gbe/evu102
124. Xu, L, Hou, Y, Bickhart, DM, Song, J, Van Tassell, CP, Sonstegard, TS, et al. A genome-wide survey reveals a deletion polymorphism associated with resistance to gastrointestinal nematodes in Angus cattle. Funct Integr Genomics. (2014) 14:333–9. doi: 10.1007/s10142-014-0371-6
125. Hou, Y, Liu, GE, Bickhart, DM, Matukumalli, LK, Li, C, Song, J, et al. Genomic regions showing copy number variations associate with resistance or susceptibility to gastrointestinal nematodes in Angus cattle. Funct Integr Genomics. (2012) 12:81–92. doi: 10.1007/s10142-011-0252-1
126. Liu, GE, Brown, T, Hebert, DA, Cardone, MF, Hou, Y, Choudhary, RK, et al. Initial analysis of copy number variations in cattle selected for resistance or susceptibility to intestinal nematodes. Mamm Genome. (2011) 22:111–21. doi: 10.1007/s00335-010-9308-0
127. Meyers, SN, McDaneld, TG, Swist, SL, Marron, BM, Steffen, DJ, O’Toole, D, et al. A deletion mutation in bovine SLC4A2 is associated with osteopetrosis in red Angus cattle. BMC Genomics. (2010) 11:337. doi: 10.1186/1471-2164-11-337
128. McDaneld, TG, Kuehn, LA, Thomas, MG, Pollak, EJ, and Keele, JW. Deletion on chromosome 5 associated with decreased reproductive efficiency in female cattle. J Anim Sci. (2014) 92:1378–84. doi: 10.2527/jas.2013-6821
129. Venhoranta, H, Pausch, H, Wysocki, M, Szczerbal, I, Hänninen, R, Taponen, J, et al. Ectopic KIT copy number variation underlies impaired migration of primordial germ cells associated with gonadal hypoplasia in cattle (Bos taurus). PLoS One. (2013) 8:e75659. doi: 10.1371/journal.pone.0075659
130. Flisikowski, K, Venhoranta, H, Nowacka-Woszuk, J, McKay, SD, Flyckt, A, Taponen, J, et al. A novel mutation in the maternally imprinted PEG3 domain results in a loss of MIMT1 expression and causes abortions and stillbirths in cattle (Bos taurus). PLoS One. (2010) 5:e15116. doi: 10.1371/journal.pone.0015116
131. Allais-Bonnet, A, Grohs, C, Medugorac, I, Krebs, S, Djari, A, Graf, A, et al. Novel insights into the bovine polled phenotype and horn ontogenesis in Bovidae. PLoS One. (2013) 8:e63512. doi: 10.1371/journal.pone.0063512
132. Capitan, A, Allais-Bonnet, A, Pinton, A, Marquant-Le Guienne, B, Le Bourhis, D, Grohs, C, et al. A 3.7 Mb deletion encompassing ZEB2 causes a novel polled and multisystemic syndrome in the progeny of a somatic mosaic bull. PLoS One. (2012) 7:e49084. doi: 10.1371/journal.pone.0049084
133. Trigo, BB, Utsunomiya, AT, Fortunato, AA, Milanesi, M, Torrecilha, RB, Lamb, H, et al. Variants at the ASIP locus contribute to coat color darkening in Nellore cattle. Genet Sel Evol. (2021) 53:40. doi: 10.1186/s12711-021-00633-2
134. Kava, R, Peripolli, E, Berton, MP, Lemos, M, Lobo, RB, Stafuzza, NB, et al. Genome-wide structural variations in Brazilian Senepol cattle, a tropically adapted taurine breed. Livest Sci. (2021) 253:104708. doi: 10.1016/j.livsci.2021.104708
135. Fernandes Junior, GA, de Oliveira, HN, Carvalheiro, R, Cardoso, DF, Fonseca, LF, Ventura, RV, et al. Whole-genome sequencing provides new insights into genetic mechanisms of tropical adaptation in Nellore (Bos primigenius indicus). Sci Rep. (2020) 10:9412. doi: 10.1038/s41598-020-66272-7
136. Zhang, Z, Peng, M, Wen, Y, Chai, Y, Liang, J, Yang, P, et al. Copy number variation of EIF4A2 loci related to phenotypic traits in Chinese cattle. Vet Med Sci. (2022) 8:2147–56. doi: 10.1002/vms3.875
137. Zhou, Y, Utsunomiya, YT, Xu, L, Hay, EH, Bickhart, DM, Alexandre, PA, et al. Genome-wide CNV analysis reveals variants associated with growth traits in Bos indicus. BMC Genomics. (2016) 17:1–9. doi: 10.1186/s12864-016-2461-4
138. Braga, LG, Chud, TC, Watanabe, RN, Savegnago, RP, and Sena, TM. Identification of copy number variations in the genome of dairy Gir cattle. PLoS One. (2023) 18:e0284085. doi: 10.1371/journal.pone.0284085
139. Chen, L, Pryce, JE, Hayes, BJ, and Daetwyler, HD. Investigating the effect of imputed structural variants from whole-genome sequence on genome-wide association and genomic prediction in dairy cattle. Animals (Basel). (2021) 11:541. doi: 10.3390/ani11020541
140. Talenti, A, Powell, J, Wragg, D, Chepkwony, M, Fisch, A, Ferreira, BR, et al. Optical mapping compendium of structural variants across global cattle breeds. Sci Data. (2022) 9:618. doi: 10.1038/s41597-022-01684-w
141. Xia, X, Zhang, F, Li, S, Luo, X, Peng, L, Dong, Z, et al. Structural variation and introgression from wild populations in east Asian cattle genomes confer adaptation to local environment. Genome Biol. (2023) 24:211. doi: 10.1186/s13059-023-03052-2
142. Lee, YL, Takeda, H, Costa Monteiro Moreira, G, Karim, L, Mullaart, E, Coppieters, W, et al. A 12 kb multi-allelic copy number variation encompassing a GC gene enhancer is associated with mastitis resistance in dairy cattle. PLoS Genet. (2021) 17:e1009331. doi: 10.1371/journal.pgen.1009331
143. de Oliveira, HR, Chud, TC, Oliveira, GA Jr, Hermisdorff, IC, Narayana, SG, Rochus, CM, et al. Genome-wide association analyses reveal copy number variant regions associated with reproduction and disease traits in Canadian Holstein cattle. J Dairy Sci. (2024) 107:7052–63. doi: 10.3168/jds.2023-24295
144. Butty, AM, Chud, TC, Cardoso, DF, Lopes, LS, Miglior, F, Schenkel, FS, et al. Genome-wide association study between copy number variants and hoof health traits in Holstein dairy cattle. J Dairy Sci. (2021) 104:8050–61. doi: 10.3168/jds.2020-19879
145. Zhou, Y, Yang, L, Han, X, Han, J, Hu, Y, Li, F, et al. Assembly of a pangenome for global cattle reveals missing sequences and novel structural variations, providing new insights into their diversity and evolutionary history. Genome Res. (2022) 32:1585–601. doi: 10.1101/gr.276550.122
146. Leonard, AS, Mapel, XM, and Pausch, H. Pangenome-genotyped structural variation improves molecular phenotype mapping in cattle. Genome Res. (2024) 34:300–9. doi: 10.1101/gr.278267.123
147. Ahmad, SF, Chandrababu Shailaja, C, Vaishnav, S, Kumar, A, Gaur, GK, Janga, SC, et al. Read-depth based approach on whole genome resequencing data reveals important insights into the copy number variation (CNV) map of major global buffalo breeds. BMC Genomics. (2023) 24:616. doi: 10.1186/s12864-023-09720-8
148. Li, W, Bickhart, DM, Ramunno, L, Iamartino, D, Williams, JL, and Liu, GE. Genomic structural differences between cattle and river Buffalo identified through comparative genomic and transcriptomic analysis. Data Brief. (2018) 19:236–9. doi: 10.1016/j.dib.2018.05.015
149. Wang, X, Li, Z, Feng, T, Luo, X, Xue, L, Mao, C, et al. Chromosome-level genome and recombination map of the male buffalo. GigaScience. (2023) 12:giad063. doi: 10.1093/gigascience/giad063
150. Strillacci, MG, Moradi-Shahrbabak, H, Davoudi, P, Ghoreishifar, SM, Mokhber, M, Masroure, AJ, et al. A genome-wide scan of copy number variants in three Iranian indigenous river buffaloes. BMC Genomics. (2021) 22:305. doi: 10.1186/s12864-021-07604-3
151. Li, W, Bickhart, DM, Ramunno, L, Iamartino, D, Williams, JL, and Liu, GE. Comparative sequence alignment reveals river Buffalo genomic structural differences compared with cattle. Genomics. (2019) 111:418–25. doi: 10.1016/j.ygeno.2018.02.018
152. Deng, TX, Ma, XY, Duan, A, Lu, XR, and Abdel-Shafy, H. Genome-wide copy number variant analysis reveals candidate genes associated with milk production traits in water buffalo (Bubalus bubalis). J Dairy Sci. (2024) 107:7022–37. doi: 10.3168/jds.2023-24614
153. Jing, Z, Qin, P, Chen, B, Hou, D, Wei, C, Li, T, et al. Research progress of copy number variation in livestock and poultry. China Animal Husbandry Vet Med. (2021) 48:2512–22. doi: 10.16431/j.cnki.1671-7236.2021.07.027
154. Qiao, G, Xu, P, Guo, T, He, X, Yue, Y, and Yang, B. Genome-wide detection of structural variation in some sheep breeds using whole-genome Long-read sequencing data. J Anim Breed Genet. (2024) 141:403–14. doi: 10.1111/jbg.12846
155. Igoshin, AV, Deniskova, TE, Yurchenko, AA, Yudin, NS, Dotsev, AV, Selionova, MI, et al. Copy number variants in genomes of local sheep breeds from Russia. Anim Genet. (2022) 53:119–32. doi: 10.1111/age.13163
156. Di Gerlando, R, Mastrangelo, S, Tolone, M, Rizzuto, I, Sutera, AM, Moscarelli, A, et al. Identification of copy number variations and genetic diversity in Italian insular sheep breeds. Animals. (2022) 12:217. doi: 10.3390/ani12020217
157. Moradi, MH, Mahmodi, R, Farahani, AH, and Karimi, MO. Genome-wide evaluation of copy gain and loss variations in three afghan sheep breeds. Sci Rep. (2022) 12:14286. doi: 10.1038/s41598-022-18571-4
158. Cumer, T, Boyer, F, and Pompanon, F. Genome-wide detection of structural variations reveals new regions associated with domestication in small ruminants. Genome Biol Evol. (2021) 13:evab165. doi: 10.1093/gbe/evab165
159. Goyache, F, Fernández, I, Tapsoba, AS, Traoré, A, Menéndez-Arias, NA, and Álvarez, I. Functional characterization of copy number variations regions in Djallonké sheep. J Anim Breed Genet. (2021) 138:600–12. doi: 10.1111/jbg.12542
160. Nandolo, W, Mészáros, G, Wurzinger, M, Banda, LJ, Gondwe, TN, Mulindwa, HA, et al. Detection of copy number variants in African goats using whole genome sequence data. BMC Genomics. (2021) 22:398. doi: 10.1186/s12864-021-07703-1
161. Guan, D, Martínez, A, Castelló, A, Landi, V, Luigi-Sierra, MG, Fernández-Álvarez, J, et al. A genome-wide analysis of copy number variation in Murciano-Granadina goats. Genet Sel Evol. (2020) 52:1. doi: 10.1186/s12711-020-00564-4
162. Liu, M, Woodward-Greene, J, Kang, X, Pan, MG, Rosen, B, Van Tassell, CP, et al. Genome-wide CNV analysis revealed variants associated with growth traits in African indigenous goats. Genomics. (2020) 112:1477–80. doi: 10.1016/j.ygeno.2019.08.018
163. Di Gerlando, R, Sutera, AM, Mastrangelo, S, Tolone, M, Portolano, B, Sottile, G, et al. Genome-wide association study between CNVs and milk production traits in Valle del Belice sheep. PLoS One. (2019) 14:e0215204. doi: 10.1371/journal.pone.0215204
164. Henkel, J, Saif, R, Jagannathan, V, Schmocker, C, Zeindler, F, Bangerter, E, et al. Selection signatures in goats reveal copy number variants underlying breed-defining coat color phenotypes. PLoS Genet. (2019) 15:e1008536. doi: 10.1371/journal.pgen.1008536
165. Fontanesi, L, Beretti, F, Martelli, PL, Colombo, M, Dall'Olio, S, Occidente, M, et al. A first comparative map of copy number variations in the sheep genome. Genomics. (2011) 97:158–65. doi: 10.1016/j.ygeno.2010.11.005
166. Jiang, R, Cheng, J, Cao, XK, Ma, YL, Chaogetu, B, Huang, YZ, et al. Copy number variation of the SHE gene in sheep and its association with economic traits. Animals (Basel). (2019) 9:531. doi: 10.3390/ani9080531
167. Linzer, DI, and Nathans, D. A new member of the prolactin-growth hormone gene family expressed in mouse placenta. EMBO J. (1985) 4:1419–23. doi: 10.1002/j.1460-2075.1985.tb03796.x
168. Zhang, RQ, Wang, JJ, Zhang, T, Zhai, HL, and Shen, W. Copy-number variation in goat genome sequence: a comparative analysis of the different litter size trait groups. Gene. (2019) 696:40–6. doi: 10.1016/j.gene.2019.02.027
169. Li, X, Yang, J, Shen, M, Xie, XL, Liu, GJ, Xu, YX, et al. Whole-genome resequencing of wild and domestic sheep identifies genes associated with morphological and agronomic traits. Nat Commun. (2020) 11:2815. doi: 10.1038/s41467-020-16485-1
170. Li, R, Gong, M, Zhang, X, Wang, F, Liu, Z, Zhang, L, et al. A sheep pangenome reveals the spectrum of structural variations and their effects on tail phenotypes. Genome Res. (2023) 33:463–77. doi: 10.1101/gr.277372.122
171. Shi, H, Li, T, Su, M, Wang, H, Li, Q, Lang, X, et al. Identification of copy number variation in Tibetan sheep using whole genome resequencing reveals evidence of genomic selection. BMC Genomics. (2023) 24:555. doi: 10.1186/s12864-023-09672-z
172. Taghizadeh, S, Gholizadeh, M, Rahimi-Mianji, G, Moradi, MH, Costilla, R, Moore, S, et al. Genome-wide identification of copy number variation and association with fat deposition in thin and fat-tailed sheep breeds. Sci Rep. (2022) 12:8834. doi: 10.1038/s41598-022-12778-1
173. Huang, B, Khan, MZ, Chai, W, Ullah, Q, and Wang, C. Exploring genetic markers: mitochondrial DNA and genomic screening for biodiversity and production traits in donkeys. Animals. (2023) 13:272. doi: 10.3390/ani13172725
174. Arefnejad, B, Zeinalabedini, M, Talebi, R, Mardi, M, Ghaffari, MR, Vahidi, MF, et al. Unveiling the population genetic structure of Iranian horses breeds by whole-genome resequencing analysis. Mamm Genome. (2024) 35:201–27. doi: 10.1007/s00335-024-10035-6
175. Wang, C, Li, H, Guo, Y, Huang, J, Sun, Y, Min, J, et al. Donkey genomes provide new insights into domestication and selection for coat color. Nat Commun. (2020) 11:6014. doi: 10.1038/s41467-020-19813-7
176. Renaud, G, Petersen, B, Seguin-Orlando, A, Bertelsen, MF, Waller, A, Newton, R, et al. Improved de novo genomic assembly for the domestic donkey. Sci Adv. (2018) 4:eaaq0392. doi: 10.1126/sciadv.aaq0392
177. Ghosh, S, Qu, Z, Das, PJ, Fang, E, Juras, R, Cothran, EG, et al. Copy number variation in the horse genome. PLoS Genet. (2014) 10:e1004712. doi: 10.1371/journal.pgen.1004712
178. Doan, R, Cohen, N, Harrington, J, Veazy, K, Juras, R, Cothran, G, et al. Identification of copy number variants in horses. Genome Res. (2012) 22:899–907. doi: 10.1101/gr.128991.111
179. Al Abri, MA, Holl, HM, Kalla, SE, Sutter, NB, and Brooks, SA. Whole genome detection of sequence and structural polymorphism in six diverse horses. PLoS One. (2020) 15:e0230899. doi: 10.1371/journal.pone.0230899
180. Han, H, Zhao, X, Xia, X, Chen, H, Lei, C, and Dang, R. Copy number variations of five y chromosome genes in donkeys. Arch Anim Breed. (2017) 60:391–7. doi: 10.5194/aab-60-391-2017
181. Zhou, C, Zheng, X, Peng, K, Feng, K, Yue, B, and Wu, Y. Chromosome-level genome assembly of the kiang (Equus kiang) illuminates genomic basis for its high-altitude adaptation. Integr Zool. (2023). 18:225–36. doi: 10.1111/1749-4877.12795
182. Paria, N, Raudsepp, T, Pearks Wilkerson, AJ, O'Brien, PC, Ferguson-Smith, MA, Love, CC, et al. A gene catalogue of the euchromatic male-specific region of the horse Y chromosome: comparison with human and other mammals. PLoS One. (2011) 6:e21374. doi: 10.1371/journal.pone.0021374
183. Wang, M, Liu, Y, Bi, X, Ma, H, Zeng, G, Guo, J, et al. Genome-wide detection of copy number variants in Chinese indigenous horse breeds and verification of CNV-overlapped genes related to heat adaptation of the Jinjiang horse. Genes (Basel). (2022) 13:603. doi: 10.3390/genes13040603
184. Castaneda, C, Radović, L, Felkel, S, Juras, R, Davis, BW, Cothran, EG, et al. Copy number variation of horse Y chromosome genes in normal equine populations and in horses with abnormal sex development and subfertility: relationship of copy number variations with Y haplogroups. G3. (2022) 12:278. doi: 10.1093/g3journal/jkac278
185. Wang, S, Lin, J, Jia, P, Xu, T, Li, X, Liu, Y, et al. De novo and somatic structural variant discovery with SVision-pro. Nat Biotechnol. (2024) 22:1–5. doi: 10.1038/s41587-024-02190-7
186. Zhou, Y, Connor, EE, Wiggans, GR, Lu, Y, Tempelman, RJ, Schroeder, SG, et al. Genome-wide copy number variant analysis reveals variants associated with 10 diverse production traits in Holstein cattle. BMC Genomics. (2018) 19:1–9. doi: 10.1186/s12864-018-4699-5
187. Shi, T, Xu, Y, Yang, M, Huang, Y, Lan, X, Lei, C, et al. Copy number variations at LEPR gene locus associated with gene expression and phenotypic traits in Chinese cattle. Anim Sci J. (2016) 87:336–43. doi: 10.1111/asj.12531
Keywords: structural variations, livestock genome, phenotypic traits, genetic marker, molecular breeding
Citation: Chen Y, Khan MZ, Wang X, Liang H, Ren W, Kou X, Liu X, Chen W, Peng Y and Wang C (2024) Structural variations in livestock genomes and their associations with phenotypic traits: a review. Front. Vet. Sci. 11:1416220. doi: 10.3389/fvets.2024.1416220
Edited by:
Wellison J. S. Diniz, Auburn University, United StatesReviewed by:
Pita Sudrajad, National Research and Innovation Agency (BRIN), IndonesiaSharmila Ghosh, University of California, Davis, United States
Copyright © 2024 Chen, Khan, Wang, Liang, Ren, Kou, Liu, Chen, Peng and Wang. This is an open-access article distributed under the terms of the Creative Commons Attribution License (CC BY). The use, distribution or reproduction in other forums is permitted, provided the original author(s) and the copyright owner(s) are credited and that the original publication in this journal is cited, in accordance with accepted academic practice. No use, distribution or reproduction is permitted which does not comply with these terms.
*Correspondence: Muhammad Zahoor Khan, emFob29ya2hhdHRhazkxQHlhaG9vLmNvbQ==; Changfa Wang, d2FuZ2NoYW5nZmFAbGN1LmVkdS5jbg==; Yongdong Peng, cGVuZ3lvbmdkb25nQGxjdS5lZHUuY24=