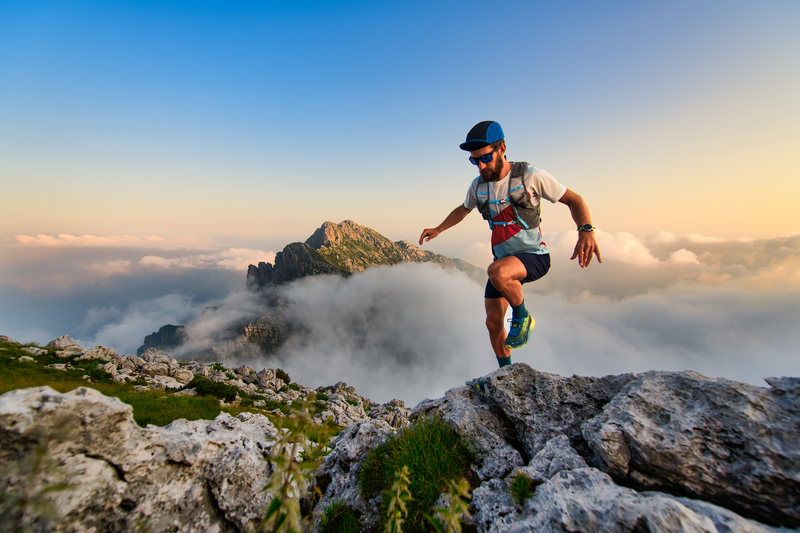
94% of researchers rate our articles as excellent or good
Learn more about the work of our research integrity team to safeguard the quality of each article we publish.
Find out more
ORIGINAL RESEARCH article
Front. Trop. Dis. , 21 September 2022
Sec. Emerging Tropical Diseases
Volume 3 - 2022 | https://doi.org/10.3389/fitd.2022.969307
Early diagnosis is crucial in controlling tuberculosis globally and in developing countries with the emergence of drug-resistant Mycobacterium tuberculosis strains. Long non-coding RNAs (lncRNAs) are promising tuberculosis diagnostic biomarkers. Two lncRNA diagnostic markers, lncRNA THRIL and lincRNA-p21, were studied as tuberculosis diagnostic biomarkers. This cross-sectional study was conducted at the Center of Respiratory Diseases of LAQUINTINIE hospital and the National Veterinary Laboratory of Douala from December 2020 to August 2021. The ability of lncRNAs to distinguish between 19 healthy controls, 15 latent tuberculosis, and 21 active tuberculosis was estimated using quantitative polymerase chain reaction and Receiver Operating Characteristic curve analysis. Our analysis showed that lncRNA THRIL and lincRNA-p21 were significantly upregulated (P <0.05) in active and latent tuberculosis compared with healthy controls. LincRNA-p21 expression was significantly increased (P <0.05) in active tuberculosis compared with latent tuberculosis, whereas lncRNA THRIL was not significantly affected (P ≥0.05). Both lncRNA THRIL and lincRNA-p21 showed excellent performance in classifying latent tuberculosis and healthy controls (AUC = 92.86%). Furthermore, lncRNA THRIL was good at discriminating active tuberculosis from healthy controls (AUC = 89.79%), while lincRNA-p21 showed excellent discriminating performance (AUC = 100%). LncRNA THRIL was identified as a poor discriminator of latent tuberculosis from active tuberculosis (AUC = 64.28%), while lincRNA-p21 showed excellent diagnostic performance in this distinction (AUC = 92.86%). Our cross-sectional study suggests that lncRNA THRIL and lincRNA-p21 are promising tuberculosis diagnostic biomarkers that can differentiate between latent and active infection.
Tuberculosis (TB) is an airborne disease (1), which exists in two forms: active and latent (2, 3). It is caused by bacteria of the Mycobacterium tuberculosis complex (MTBC) (4). Usually, these bacteria affect the lungs but can also affect other parts of the body, such as the spine, the brain, or kidneys, and if untreated, cause a fatal infection (5). Mtb bacteria can be transmitted through air inhalation by a healthy person when he stays in close proximity to an active TB patient who coughs, spits, speaks, sings, laughs, or sneezes (6).
About two billion people (one-quarter of the world population) are infected with M. tuberculosis. Most of these people (85%–95%), the so-called latent tuberculosis infected (LTBI) people, will be able to control the growth and live with the bacteria all their life without developing TB symptoms or spreading it to others (7). However, 5%–15% of the people with LTBI will develop active tuberculosis and are capable of spreading the bacteria to others. Some factors that favor this progression include a compromised immune system by a disease such as HIV (8, 9) or aging (9).
Active TB is predominant in people who smoke or are immunocompromised due to HIV/AIDS. These people cannot contain the Mtb, hence it multiplies in the body, causing the TB disease symptoms and the spread of the disease to others (8).
In 2020, 10 million cases of tuberculosis were recorded (10). Ninety-eight percent of these cases were reported in low- and middle-income countries (LMICs) (10). In addition to the prevalence, the emergence of drug-resistant M. tuberculosis strains is a serious public health concern in these countries. To end this global TB epidemic, the World Health Organization (2014) made early diagnosis a key element of its strategy (11).
In many developing countries, such as Cameroon, sputum smear microscopy is used as the laboratory technique for TB diagnosis. However, a person with latent TB infection can have normal chest X-rays and negative sputum smear microscopy results but test positive for a blood or skin prick test (12). However, active TB patients may display abnormal chest X-rays while testing positive for sputum smear or culture (12). Therefore, although sputum smear microscopy is rapid and low-cost (13), it cannot be used to detect people with latent TB. Additionally, its reliability is influenced by the quality of sputum collected and the proper preparation and interpretation of slides. This quick diagnostic method is also limited by its non-specificity and low sensitivity (13). To make up for the lack of very accurate TB diagnostic tools, research on TB-specific biomarkers is gaining momentum, and these host- and pathogen-derived biomolecules have proven to be important targets for the early diagnosis of several diseases (14).
A biomarker or biological marker is a measure of what is happening in a cell, tissue, or organism at a given moment (15, 16). Several biomarkers are used for the detection, screening, diagnosis, treatment, and monitoring of various diseases. For TB, many good biomarkers have been described, including TB antigens (17), and host molecules (18), such as microRNAs, whole blood genes (19) and ong non-coding RNAs (lncRNAs) (20–27).
Long non-coding RNAs are nucleic acid molecules of more than 200 nucleotides in size, which are not translated into functional proteins (28, 29). The genes coding for the lncRNA THRIL are located on chromosome 12q24.31 (30). This lncRNA binds specifically to heterogeneous nuclear ribonucleoprotein L (hnRNPL) and forms a functional complex that regulates the expression of the Tumor Necrosis Factor-alpha (TNF-α) gene by binding to its promoter (31). TNFα is one of the most central pro-inflammatory cytokines (32). It is involved in many host-protective pathways against M. tuberculosis. Indeed, TNFα can promote phagosome–lysosome maturation and Mtb antigen presentation (33). The latter process can stimulate the CD4+ T-cell response (33). Additionally, TNFα can induce apoptosis in mycobacteria-infected macrophages, hence leading to cross-priming of CD8+ T cells (33). This cytokine can also stimulate the accumulation of effector interleukins (IL) such as IL-6 (34).
Interleukin-6 can exhibit pro- or anti-inflammatory activities (35). It plays an important function in the immune response against Mtb infection (34), particularly in the acute phase (36). A study reported that the expression of the pro-inflammatory IL-6 in LPS-stimulated BV2 microglia cells was induced by lincRNA-p21 (37). This lncRNA, which is found on chromosome 6p21.2 (38) activates its proximal gene p21 and represses distant genes involved in the p53 transcriptional network (39). This lncRNA plays some important functions in the cell cycle, apoptosis, cell proliferation (40), inflammatory responses, and oxidative stress (41).
The lncRNA THRIL has been reported as a biomarker in COVID-19 (42), multiple sclerosis (43), systemic lupus erythematosus (44), childhood immune thrombocytopenia (45), and allergic rhinitis (46). Meanwhile, lincRNA-p21 has been described as a potential biomarker in various human cancers (40). To date, no study has explored the potential of lncRNA THRIL and lincRNA-p21 as biomarkers for the diagnosis of TB. The link between these lncRNAs and the proinflammatory cytokines TNFα and IL-6 made us hypothesize that these lncRNAs might be useful biomarkers for tuberculosis onset or progression. Hence, this study investigated the ability of lncRNA THRIL and lincRNA-p21 to distinguish between healthy controls, latent tuberculosis, and active tuberculosis. We report for the first time that lincRNA-p21 is an excellent discriminator of active from latent tuberculosis.
The participant recruitment in this cross-sectional study was performed at the Center of Respiratory Diseases at Laquintinie Hospital. Laboratory analyses were carried out at the National Veterinary Laboratory in Douala. The study was conducted from December 2020 to August 2021.
The Healthy Control (HC) group included 19 non-smoking subjects with no history of TB who underwent a routine physical examination at the Laquintinie Hospital, Douala, during the study period. No abnormalities were found on their chest X-rays. They had not had contact with tuberculosis patients within the last two months and had negative results for human immunodeficiency virus (HIV), sputum smear, and TB LAMP tests. The healthy controls did not have chronic diseases and were not receiving immunosuppressive therapy.
The Active Tuberculosis (ATB) group comprised 21 HIV-negative patients with no history of tuberculosis and presented a positive sputum smear. Their chest X-rays were consistent with the proven pulmonary TB. They did not have chronic disease and were not on immunosuppressive therapy. The active tuberculosis participants had not started TB treatment.
The Latent Tuberculosis (LTB) group was made up of 15 non-smokers who were HIV negative and had no history of tuberculosis. They presented a negative sputum smear and a TB-LAMP-positive test. They did not have any chronic diseases and were not receiving immunosuppressive therapy. The latent tuberculosis participants had been in contact with tuberculosis patients within the last two months.
This study was approved by the Institutional Ethics Committee for Research on Human Health, University of Douala (No. 2434CEI/Udo/09/2020/M). A unique Patient Identification Number (PIN) was used to ensure confidentiality. All participants or the legal guardian/next of kin of the participants gave a written informed consent form. The questionnaire was completed before blood collection and processing.
Venous blood was collected in 5-ml Ethylene Diamine Tetraacetic Acid (EDTA) tubes and centrifuged at 3,000 rpm for 10 min. The supernatant (plasma) was separated, after which 500 µl of the cellular fraction was aliquoted into a 1.5 ml-labeled tube and 500 µl of Qiazol reagent (Qiagen, Germany) was added. The mixture was homogenized and stored at −20°C until total RNA extraction.
Total RNA was purified from the stored cellular lysate (500 µl cellular fraction + 500 µl Qiazol) using the miRNeasy Mini Kit (Qiagen, Germany) according to the instructions of the manufacturer (47). cDNA was then synthesized using a Transcriptor First Strand cDNA Synthesis Kit (Roche, Germany) according to the instructions of the manufacturer (48).
Gene-specific primers (IDT, CA, United States) targeting specific portions of lncRNA THRIL, lincRNA-p21, and glyceraldehyde-3-phosphate dehydrogenase (GAPDH) as well as LightCycler® 480 SYBR Green I Master (Roche, Germany) were used to realize quantitative real-time PCR (qPCR). The amplification program and the sequences of the qPCR primer sets used are described in Tables 1, 2. A melting curve analysis was performed at the end of the PCR reaction to confirm specific amplifications, followed by agarose gel electrophoresis for specific amplicon size verification. Relative lncRNA levels were calculated using the 2−ΔΔCq method (49) with GAPDH as an internal control.
Relative expression data of lncRNA THRIL and lincRNA-p21 obtained from the qPCR analyses were normalized by logarithmic transformation. These data were then subjected to a paired-sample T-Test with HC-ATB, HC-LTB, and ATB-LTB as paired variables, using SPSS 20.0 software (Statsoft Inc., Carey, J, USA) and the results were expressed as means with standard errors (SE). The paired differences were considered significant when the p-values were less than 0.05 (at a 95% confidence level). Microsoft Excel software version 2013 was used to draw curves and graphs.
The software EasyROC (ver. 1.3.1) is a web-based tool used to analyze ROC (Receiver Operating Characteristics) curves (50). In addition to creating ROC curves, this application calculates the Area Under the Curve (AUC) (50), which is a measure of the capacity of a parameter to distinguish between two groups (51). In ROC curve analysis, the AUC values lie between 50% and 100%, with 50% being a bad classifier and 100% being a perfect classifier (52). Values within the range of 90 and 100% are generally considered excellent, 80 and 90% good, 70 and 80% fair, 60 and 70% poor, and 50 and 60% bad (or failed) (53). An AUC of below 50% indicates a set of random values unable to distinguish between two groups (54). Here, the ROC curves were used to assess the ability of targeted lncRNAs (lncRNA THRIL and lincRNA-p21) to discriminate among the various groups (HC, ATB, and LTB). These curves were set up with the normalized 2−ddCq generated through the calculations done using the LIVAK method.
Fifty-five (55) participants were enrolled during this study, with 69% (n = 38) being male and 31% (n = 17) female. Twenty-seven percent (n = 15) of the participants were aged between 25 and 34 years. Table 3 shows the details of the sociodemographic and clinical characteristics of the study population.
The expression levels of lncRNA THRIL in the whole blood of latent TB (2.34 ± 0.81) and active patients (2.69 ± 1.05) were significantly higher (P <0.05) compared to healthy controls (Figure 1). However, the whole blood of patients with latent TB displayed similar levels of THRIL (P ≥0.05) compared to patients with active TB (Figure 1). Thus, THRIL is more expressed in latent and active TB than in the healthy state.
Figure 1 Relative expression of lncRNA THRIL in whole blood samples of the different subject groups. Total RNA was extracted. The lncRNA THRIL level was assessed by RT-qPCR. The 2−ΔΔCq method was used to calculate the lncRNA relative expression of the healthy controls (HC), active (ATB), and latent tuberculosis patients (LTB).
The expression levels of lincRNA-p21 in the whole blood of latent TB (2.07 ± 0.71) and active patients (4.33 ± 1.33) were significantly (P <0.05) upregulated compared to healthy controls (Figure 2). Similarly, patients with latent TB expressed higher levels of lincRNA-p21 (P <0.05) than patients with active TB (Figure 2). Thus, lincRNA-p21 is most upregulated in the case of active TB.
Figure 2 Relative expression of lincRNA-p21 in whole blood samples of the study population. After the extraction of total RNA, the expression of lincRNA-p21 was evaluated by RT-qPCR. The lncRNA relative expression of the healthy controls (HC), active (ATB), and latent tuberculosis patients (LTB) was worked out using the 2[−Delta Delta C(q)] method.
When comparing the latent TB patients and healthy controls, both lncRNAs exhibited an AUC value of 92.86% (Figure 3). This implies that THRIL and lincRNA-p21 are excellent categorizers of these two groups.
Figure 3 LncRNA THRIL and lincRNA-p21 levels discriminate latent tuberculosis patients from healthy controls. Total RNA was obtained from the whole blood samples of latent tuberculosis patients (LTB) and healthy controls (HC). The LncRNA THRIL and lincRNA-p21 relative expression levels were measured by RT-qPCR coupled to the 2−ΔΔCq method. The ROC curves were generated and the area under the curve (AUC) values were calculated using the software easyROC.
In terms of the discrimination of patients with active pulmonary TB from healthy controls, THRIL showed an AUC of 89.79%, while lincRNA-p21 showed 100% (Figure 4). This result indicates that THRIL is good, whereas lincRNA-p21 is excellent at distinguishing between active pulmonary TB and healthy controls.
Figure 4 LncRNA THRIL and lincRNA-p21 levels distinguish between active pulmonary tuberculosis patients and healthy controls. Total RNA was purified from the whole blood samples of active tuberculosis patients (ATB) and healthy controls (HC). The LncRNA THRIL and lincRNA-p21 relative expression levels were determined by RT-qPCR associated with the Livak method. The software easyROC was used to set up ROC curves and calculate the area under the curve (AUC) values.
When looking at latent versus active pulmonary tuberculosis, THRIL and lincRNA-p21 displayed respective AUCs of 64.28% and 92.86% (Figure 5). Thus, THRIL is a poor classifier, whereas lincRNA-p21 is excellent in the distinction of active from latent pulmonary TB.
Figure 5 The ability of lncRNA THRIL and lincRNA-p21 levels to discriminate between latent and active pulmonary tuberculosis patients. Total RNA derived from the whole blood samples of latent (LTB) and active (ATB) tuberculosis patients was used to detect the lncRNA THRIL and lincRNA-p21 by RT-qPCR. The relative expression levels pf lncRNAs were obtained through the 2[−Delta Delta C(q)] method. The ROC curves were constructed and the corresponding area under the curve (AUC) values were gotten from the software easyROC.
Therefore, these data suggest that THRIL and lincRNA-p21 can be considered potential biomarkers in the diagnosis of TB, and lincRNA-p21 might serve as a biomarker in the diagnosis of latent TB.
The early diagnosis of tuberculosis is one of the key elements of the End TB Strategy. Infection with M. tuberculosis leads to an altered expression profile of many non-coding RNAs (ncRNAs) that could be exploited as biomarkers for the early diagnosis of TB. Although there is limited knowledge on lncRNA alteration during the onset and progression of several diseases, using these biomolecules as diagnostic biomarkers is advantageous in that they are altered early in the disease process compared to proteins, and hence are more suitable for early diagnosis (55). Moreover, they are found stable while circulating in extreme body fluid milieus and conditions (56) and display high cell, tissue, and context-specific expression profiles (57). Hence, this study aimed to investigate lncRNA THRIL and lincRNA-p21 as TB diagnostic biomarkers useful for accurate early diagnosis of this disease.
The expression levels of these two lncRNAs were first measured in the whole blood collected from healthy volunteers and latent and active TB patients. This study revealed that lncRNA THRIL and lincRNA-p21 levels were increased in the whole blood of latent and active TB patients as compared with healthy controls. Since TB is known as an inflammatory disease (58), we speculate that this lncRNA upregulation probably resulted from the inflammatory response to infection by bacteria of the M. tuberculosis complex. Indeed, the lncRNA THRIL was reportedly upregulated in rheumatoid arthritis, which is a well-known inflammatory disease. In this disease model, the lncRNA THRIL upregulation was positively linked with the level of TNF-α, which is a proinflammatory cytokine (59). It was also demonstrated that lincRNA-p21 exerts pro-inflammatory effects in the microglia (37). Whether the upregulation of these lncRNAs mediates, regulates, or is just a consequence of inflammation remains to be investigated.
In this study, the whole blood lincRNA-p21 level showed excellent performance (AUC = 100%) in distinguishing active tuberculosis patients from healthy controls. Meanwhile, the THRIL level was a good discriminator (AUC = 89.79%) of active tuberculosis patients from healthy individuals. Similarly, an lncRNA: LOC152742 was reported to be an excellent classifier of active tuberculosis compared to healthy controls either in sputum or in plasma with AUC of 91.4% and 92.3%, respectively (60). Moreover, several microRNAs have been reported as biomarkers to discriminate active tuberculosis patients from healthy individuals. For instance, miR-292 (61), miR-361 (62), miR-889 (62), miR-576-3p (62), and miR-155 (63) were found to be diagnostic biomarkers of active tuberculosis in the blood with AUC values of 83.1%, 84.8%, 76.5%, 71.1%, and 89.7%, respectively. Tu et al. (64) demonstrated that miR-17-5p, miR-20b-5p, and miR-378a-3p could discriminate between the serum of active tuberculosis from those of healthy controls with an AUC of 82.2%, 74.7%, and 67.7%, respectively. The combination of these three miRNAs gave an AUC of 90.8%. None of these miRNAs alone showed excellent diagnostic performance.
The diagnosis of latent tuberculosis remains a challenge nowadays, one of the reasons being that there is no reference test (65). The usual tests are the tuberculin skin test and the interferon-gamma release assay (IGRA) (66–68). None of these two tests can discriminate between active and latent tuberculosis infection. Ndzi et al. (53) found that miR-29a-3p was a good discriminator (AUC = 84.35%) of latent and active TB. This study reported for the first time that the whole blood lincRNA-p21 level displays excellent (AUC = 92.86%) performance in distinguishing active versus latent TB. However, THRIL showed an AUC of 64.28% and thus, is a poor classifier of latent and active TB. THRIL is linked to TNFα, which is involved in granuloma formation (69). M. tuberculosis is recruited in a granuloma and can survive inside in a dormant state, causing latent tuberculosis (70). On the other hand, TNFα is a key cytokine in pro-inflammatory processes. Hence, latent and active tuberculosis patients expressed similar levels of THRIL. Therefore, this lncRNA could not differentiate them.
The ability of lncRNAs THRIL and lincRNA-p21 to distinguish drug-susceptible from drug-resistant tuberculosis patients or to monitor TB therapy progress is still unknown and could constitute good avenues to explore for the enhancement of the fight against TB.
The present study reports that the whole blood lncRNA THRIL and lincRNA-p21 levels are significantly higher in patients with latent and active TB as compared to healthy individuals. The lncRNA THRIL showed good performance in distinguishing between active TB patients and healthy individuals but displayed poor performance in differentiating between latent and active TB patients. lincRNA-p21 was excellent in discriminating between active TB patients and healthy individuals as well as in differentiating between latent and active TB patients. The lncRNA THRIL and lincRNA-p21 are biomarkers that can potentially be used in the diagnosis of human pulmonary tuberculosis.
The raw data supporting the conclusions of this article will be made available by the authors, without undue reservation.
The studies involving human participants were reviewed and approved by the Institutional Ethics Committee for Research on Human Health, University of Douala. Written informed consent to participate in this study was provided by the participants’ legal guardian/next of kin.
OT, NNN, CFM and CK designed the experimental approach and the writing plan. NNN, CK, JAN, USFS, and FJT recruited participants and performed laboratory analysis. NNN, CK, CFM, RNN, RJEMN, AM and OT did the statistical analysis. CFM, CK, NNN and RJEMN drew all the figures. CFM, CK, NNN, JAN, USFS, FJT, SNN, APCN, MAWP, MHT, ESTM and MFT wrote the draft. FFDD, AW, AM, ARNN, and OT proofread the manuscript. All authors listed have made a substantial, direct, and intellectual contribution to the work and approved it for publication.
This work was supported by the Cameroon head of state's special research fund granted to OT.
Many thanks to all the people who voluntarily accepted to participate in the study, the personnel of the Center of Respiratory Diseases of the Laquintinie Hospital and the National Veterinary Laboratory of Douala for their support throughout the realization of this work.
The authors declare that the research was conducted in the absence of any commercial or financial relationships that could be construed as a potential conflict of interest.
All claims expressed in this article are solely those of the authors and do not necessarily represent those of their affiliated organizations, or those of the publisher, the editors and the reviewers. Any product that may be evaluated in this article, or claim that may be made by its manufacturer, is not guaranteed or endorsed by the publisher.
AUC, Area Under the ROC Curve; Cq, Quantification Cycle; hnRNPL, heterogeneous nuclear ribonucleoprotein L; lincRNA-p21, Long intergenic noncoding RNA-p21; LMICs, Low- and Middle-Income Countries; lncRNAs, Long non-coding RNAs; LTBI, Latent Tuberculosis Infection; Mtb, Mycobacterium tuberculosis; MTBC, Mycobacterium tuberculosis complex; TB, Tuberculosis; THRIL, TNF and HNRNPL Related Immunoregulatory Long Non-Coding RNA.
1. Centers for Disease Control and Prevention. Tuberculosis (TB)- how TB spreads (2016). Cent Dis Control Prev. Available at: https://www.cdc.gov/tb/topic/basics/howtbspreads.htm (Accessed March 22, 2022).
2. Kanabus A. Types of TB - latent TB, TB disease, in: TBFacts (2021). Available at: https://tbfacts.org/types-of-tb/ (Accessed March 27, 2022).
3. Khatri M. Tuberculosis (TB), in: WebMD (2020). Available at: https://www.webmd.com/lung/understanding-tuberculosis-basics (Accessed March 27, 2022).
4. Mtetwa HN, Amoah ID, Kumari S, Bux F, Reddy P. The source and fate of mycobacterium tuberculosis complex in wastewater and possible routes of transmission. BMC Public Health (2022) 22:145. doi: 10.1186/s12889-022-12527-z
5. Centers for Disease Control and Prevention. Tuberculosis (TB)- basic TB facts (2019). Cent Dis Control Prev. Available at: https://www.cdc.gov/tb/topic/basics/default.htm (Accessed July 23, 2022).
6. Centers for Disease Control and Prevention. Tuberculosis facts (2022). Available at: https://www.cdc.gov/tb/publications/factseries/exposure_eng.htm (Accessed July 23, 2022).
7. World Health Organization. Global tuberculosis report 2021 (2021). Geneva: World Health Organization. Available at: https://apps.who.int/iris/handle/10665/346387 (Accessed July 25, 2022).
8. Brett K, Dulong C, Severn M. Tuberculosis in people with compromised immunity: A review of guidelines (2020). Ottawa (ON: Canadian Agency for Drugs and Technologies in Health. Available at: http://www.ncbi.nlm.nih.gov/books/NBK562936/ (Accessed July 23, 2022).
9. Walker NF, Meintjes G, Wilkinson RJ. HIV-1 and the immune response to TB. Future Virol (2013) 8:57–80. doi: 10.2217/fvl.12.123
10. World Health Organization. Tuberculosis (TB) (2021). Available at: https://www.who.int/news-room/fact-sheets/detail/tuberculosis (Accessed February 24, 2022).
11. World Health Organization. THE END TB STRATEGY (2014). Available at: https://www.who.int/tb/strategy/End_TB_Strategy.pdf.
12. Centers for Disease Control and Prevention. Tuberculosis (TB) - latent TB infection and TB disease (2020). Cent Dis Control Prev. Available at: https://youtu.be/wA_fObLY6GE (Accessed July 23, 2022).
13. Sulis G, Centis R, Sotgiu G, D’Ambrosio L, Pontali E, Spanevello A, et al. Recent developments in the diagnosis and management of tuberculosis. NPJ Prim Care Respir Med (2016) 26:16078. doi: 10.1038/npjpcrm.2016.78
14. Verma P, Pandey RK, Prajapati P, Prajapati VK. Circulating MicroRNAs: Potential and emerging biomarkers for diagnosis of human infectious diseases. Front Microbiol (2016) 7:1274. doi: 10.3389/fmicb.2016.01274
15. Haskel R. What is a biomarker? (2019). Available at: https://nursingcenter.com/ncblog/november-2019/biomarker (Accessed March 22, 2022).
16. Mandal A. What is a biomarker? (2019). News-Medicalnet. Available at: https://www.news-medical.net/health/What-is-a-Biomarker.aspx (Accessed March 22, 2022).
17. Correia-Neves M, Fröberg G, Korshun L, Viegas S, Vaz P, Ramanlal N, et al. Biomarkers for tuberculosis: the case for lipoarabinomannan. ERJ Open Res (2019) 5:00115–2018. doi: 10.1183/23120541.00115-2018
18. Conde R, Laires R, Gonçalves LG, Rizvi A, Barroso C, Villar M, et al. Discovery of serum biomarkers for diagnosis of tuberculosis by NMR metabolomics including cross-validation with a second cohort. BioMed J (2021) 21:1–11. doi: 10.1016/j.bj.2021.07.006
19. Wykowski JH, Phillips C, Ngo T, Drain PK. A systematic review of potential screening biomarkers for active TB disease. J Clin Tuberc Mycobact Dis (2021) 25:100284. doi: 10.1016/j.jctube.2021.100284
20. Bhattacharyya N, Pandey V, Bhattacharyya M, Dey A. Regulatory role of long non coding RNAs (lncRNAs) in neurological disorders: From novel biomarkers to promising therapeutic strategies. Asian J Pharm Sci (2021) 16:533–50. doi: 10.1016/j.ajps.2021.02.006
21. Bolha L, Ravnik-Glavač M, Glavač D. Long noncoding RNAs as biomarkers in cancer. Dis Markers (2017) 2017:1–14. doi: 10.1155/2017/7243968
22. Chen J, Wang R, Zhang K, Chen L. Long non-coding RNAs in non-small cell lung cancer as biomarkers and therapeutic targets. J Cell Mol Med (2014) 18:2425–36. doi: 10.1111/jcmm.12431
23. Fu J, Yu Q, Xiao J, Li S. Long noncoding RNA as a biomarker for the prognosis of ischemic stroke: A protocol for meta-analysis and bioinformatics analysis. Med (Baltimore) (2021) 100:e25596. doi: 10.1097/MD.0000000000025596
24. Gutschner T, Richtig G, Haemmerle M, Pichler M. From biomarkers to therapeutic targets–the promises and perils of long non-coding RNAs in cancer. Cancer Metastasis Rev (2018) 37:83–105. doi: 10.1007/s10555-017-9718-5
25. Li Y, Cao X, Li H. Identification and validation of novel long non-coding RNA biomarkers for early diagnosis of oral squamous cell carcinoma. Front Bioeng Biotechnol (2020) 8:256. doi: 10.3389/fbioe.2020.00256
26. Shi T, Gao G, Cao Y. Long noncoding RNAs as novel biomarkers have a promising future in cancer diagnostics. Dis Markers (2016) 2016:1–10. doi: 10.1155/2016/9085195
27. Wang Y, Li Z, Zheng S, Zhou Y, Zhao L, Ye H, et al. Expression profile of long non-coding RNAs in pancreatic cancer and their clinical significance as biomarkers. Oncotarget (2015) 6:35684–98. doi: 10.18632/oncotarget.5533
28. Ma L, Bajic VB, Zhang Z. On the classification of long non-coding RNAs. RNA Biol (2013) 10:924–33. doi: 10.4161/rna.24604
29. Statello L, Guo C-J, Chen L-L, Huarte M. Gene regulation by long non-coding RNAs and its biological functions. Nat Rev Mol Cell Biol (2021) 22:96–118. doi: 10.1038/s41580-020-00315-9
30. Converse PJ. TNF- AND HNRNPL-RELATED IMMUNOREGULATORY LONG NONCODING RNA; THRIL (2014). Available at: https://www.omim.org/entry/615622?search=lncRNA%20THRIL&highlight=lncrna%20thril.
31. Li Z, Chao T-C, Chang K-Y, Lin N, Patil VS, Shimizu C, et al. The long noncoding RNA THRIL regulates TNFα expression through its interaction with hnRNPL. Proc Natl Acad Sci (2014) 111:1002–7. doi: 10.1073/pnas.1313768111
32. Zelová H, Hošek J. TNF-α signalling and inflammation: interactions between old acquaintances. Inflammation Res (2013) 62:641–51. doi: 10.1007/s00011-013-0633-0
33. Olsen A, Chen Y, Ji Q, Zhu G, De Silva AD, Vilchèze C, et al. Targeting mycobacterium tuberculosis tumor necrosis factor alpha-downregulating genes for the development of antituberculous vaccines. mBio (2016) 7:e01023–15. doi: 10.1128/mBio.01023-15
34. Boni FG, Hamdi I, Koundi LM, Shrestha K, Xie J. Cytokine storm in tuberculosis and IL-6 involvement. Infect Genet Evol (2022) 97:105166. doi: 10.1016/j.meegid.2021.105166
35. Hoge J, Yan I, Jänner N, Schumacher V, Chalaris A, Steinmetz OM, et al. IL-6 controls the innate immune response against Listeria monocytogenes via classical IL-6 signaling. J Immunol (2013) 190:703–11. doi: 10.4049/jimmunol.1201044
36. Linge I, Tsareva A, Kondratieva E, Dyatlov A, Hidalgo J, Zvartsev R, et al. Pleiotropic effect of IL-6 produced by b-lymphocytes during early phases of adaptive immune responses against TB infection. Front Immunol (2022) 13:750068. doi: 10.3389/fimmu.2022.750068
37. Ye Y, He X, Lu F, Mao H, Zhu Z, Yao L, et al. A lincRNA-p21/miR-181 family feedback loop regulates microglial activation during systemic LPS- and MPTP- induced neuroinflammation. Cell Death Dis (2018) 9:803. doi: 10.1038/s41419-018-0821-5
38. Hartz PA. TUMOR PROTEIN p53 PATHWAY COREPRESSOR 1, NONCODING; TP53COR1 (2015). Available at: https://www.omim.org/entry/616343?search=lincRNA-p21&highlight=%22lincrna%20p21%22%20%22lincrna%7Cp21%22%20%28lincrnap21%7C%20%29%20lincrnap21.
39. Tamgue O, Chia JE, Brombacher F. Triptolide modulates the expression of inflammation-associated lncRNA-PACER and lincRNA-p21 in mycobacterium tuberculosis–infected monocyte-derived macrophages. Front Pharmacol (2021) 12:618462. doi: 10.3389/fphar.2021.618462
40. Amirinejad R, Rezaei M, Shirvani-Farsani Z. An update on long intergenic noncoding RNA p21: A regulatory molecule with various significant functions in cancer. Cell Biosci (2020) 10:82. doi: 10.1186/s13578-020-00445-9
41. Wang Y, Zhong H, Xie X, Chen CY, Huang D, Shen L, et al. Long noncoding RNA derived from CD244 signaling epigenetically controls CD8 + T-cell immune responses in tuberculosis infection. Proc Natl Acad Sci (2015) 112:E3883–92. doi: 10.1073/pnas.1501662112
42. Abbasi-Kolli M, Sadri Nahand J, Kiani SJ, Khanaliha K, Khatami A, Taghizadieh M, et al. The expression patterns of MALAT-1, NEAT-1, THRIL, and miR-155-5p in the acute to the post-acute phase of COVID-19 disease. Braz J Infect Dis (2022) 26:102354. doi: 10.1016/j.bjid.2022.102354
43. Shaker OG, Hassan A, Mohammed AM, Mohammed SR. lincR-Ccr2-5′AS and THRIL as potential biomarkers of multiple sclerosis. Egypt J Med Hum Genet (2021) 22:32. doi: 10.1186/s43042-021-00151-2
44. Fouad NA, Shaker OG, Mohamed EA, Elsayed HS, Hussein HA, Ahmed NA, et al. Diagnostic potential of metastasis-associated-lung-adenocarcinoma-transcript-1 (MALAT-1) and TNFα and hnRNPL related immunoregulatory long non-coding RNA (THRIL) in systemic lupus erythematosus patients: Relation to disease activity. Egypt Rheumatol (2019) 41:197–201. doi: 10.1016/j.ejr.2018.11.002
45. Ayoub SE, Hefzy EM, Abd El-Hmid RG, Ahmed NA, Khalefa AA, Ali DY, et al. Analysis of the expression profile of long non-coding RNAS MALAT1 and THRIL in children with immune thrombocytopenia. IUBMB Life (2020) 72:1941–50. doi: 10.1002/iub.2310
46. Song J, Liu D, Yin W. Lnc-THRIL and miR-125b relate to disease risk, severity, and imbalance of Th1 cells/Th2 cells in allergic rhinitis. Allergol Immunopathol (Madr) (2022) 50:15–23. doi: 10.15586/aei.v50i3.528
48. Roche. Transcriptor first strand cDNA synthesis kit (2017). Available at: https://www.n-genetics.com/products/1295/1023/13832.pdf.
49. Livak KJ, Schmittgen TD. Analysis of relative gene expression data using real-time quantitative PCR and the 2–ΔΔCT method. Methods (2001) 25:402–8. doi: 10.1006/meth.2001.1262
50. Goksuluk D, Korkmaz S, Zararsiz G, Karaagaoglu E. easyROC: An interactive web-tool for ROC curve analysis using r language environment. R J (2016) 8:213. doi: 10.32614/RJ-2016-042
51. Bhandari A. AUC-ROC curve in machine learning clearly explained, in: Anal vidhya (2020). Available at: https://www.analyticsvidhya.com/blog/2020/06/auc-roc-curve-machine-learning/ (Accessed July 7, 2022).
52. Kamal M. Re: What is the value of the area under the roc curve (AUC) to conclude that a classifier is excellent? (2018). Available at: https://www.researchgate.net/post/What-is-the-value-of-the-area-under-the-roc-curve-AUC-to-conclude-that-a-classifier-is-excellent/5aab2493f7b67e588f65e696/citation/download.
53. Ndzi EN, Nkenfou CN, Mekue LM, Zentilin L, Tamgue O, Pefura EWY, et al. MicroRNA hsa-miR-29a-3p is a plasma biomarker for the differential diagnosis and monitoring of tuberculosis. Tuberculosis (2019) 114:69–76. doi: 10.1016/j.tube.2018.12.001
54. Folador E. Re: What is the value of the area under the roc curve (AUC) to conclude that a classifier is excellent? (2016). Available at: https://www.researchgate.net/post/What-is-the-value-of-the-area-under-the-roc-curve-AUC-to-conclude-that-a-classifier-is-excellent/57e7c1a8eeae39df96718d43/citation/download.
55. Chen Y, Zitello E, Guo R, Deng Y. The function of LncRNAs and their role in the prediction, diagnosis, and prognosis of lung cancer. Clin Transl Med (2021) 11:1–21. doi: 10.1002/ctm2.367
56. Lu C, Wei D, Zhang Y, Wang P, Zhang W. Long non-coding RNAs as potential diagnostic and prognostic biomarkers in breast cancer: Progress and prospects. Front Oncol (2021) 11:710538. doi: 10.3389/fonc.2021.710538
57. Sánchez Y, Huarte M. Long non-coding RNAs: Challenges for diagnosis and therapies. Nucleic Acid Ther (2013) 23:15–20. doi: 10.1089/nat.2012.0414
58. Kaufmann SH, Dorhoi A. Inflammation in tuberculosis: interactions, imbalances and interventions. Curr Opin Immunol (2013) 25:441–9. doi: 10.1016/j.coi.2013.05.005
59. Liang Y, Li H, Gong X, Ding C. Long non-coding RNA THRIL mediates cell growth and inflammatory response of fibroblast-like synoviocytes by activating PI3K/AKT signals in rheumatoid arthritis. Inflammation (2020) 43:1044–53. doi: 10.1007/s10753-020-01189-x
60. Wang L, Xie B, Zhang P, Ge Y, Wang Y, Zhang D. LOC152742 as a biomarker in the diagnosis of pulmonary tuberculosis infection. J Cell Biochem (2019) 120:8949–55. doi: 10.1002/jcb.27452
61. Fu Y, Yi Z, Wu X, Li J, Xu F. Circulating MicroRNAs in patients with active pulmonary tuberculosis. J Clin Microbiol (2011) 49:4246–51. doi: 10.1128/JCM.05459-11
62. Qi Y, Cui L, Ge Y, Shi Z, Zhao K, Guo X, et al. Altered serum microRNAs as biomarkers for the early diagnosis of pulmonary tuberculosis infection. BMC Infect Dis (2012) 12:384. doi: 10.1186/1471-2334-12-384
63. Wu J, Lu C, Diao N, Zhang S, Wang S, Wang F, et al. Analysis of microRNA expression profiling identifies miR-155 and miR-155* as potential diagnostic markers for active tuberculosis: a preliminary study. Hum Immunol (2012) 73:31–7. doi: 10.1016/j.humimm.2011.10.003
64. Tu H, Yang S, Jiang T, Wei L, Shi L, Liu C, et al. Elevated pulmonary tuberculosis biomarker miR-423-5p plays critical role in the occurrence of active TB by inhibiting autophagosome-lysosome fusion. Emerg Microbes Infect (2019) 8:448–60. doi: 10.1080/22221751.2019.1590129
65. World Health Organization. Executive summary (2018). World Health Organization. Available at: https://www.ncbi.nlm.nih.gov/books/NBK531240/ (Accessed April 4, 2022).
66. Centers for Disease Control and Prevention. Latent tuberculosis infection: A guide for primary healthcare providers (2020). Available at: https://www.cdc.gov/tb/publications/ltbi/pdf/LTBIbooklet508.pdf.
67. Mwaba P, Chakaya JM, Petersen E, Wejse C, Zumla A, Kapata N. Advancing new diagnostic tests for latent tuberculosis infection due to multidrug-resistant strains of mycobacterium tuberculosis — end of the road? Int J Infect Dis (2020) 92:S69–71. doi: 10.1016/j.ijid.2020.02.011
68. Zellweger JP, Sotgiu G, Corradi M, Durando P. The diagnosis of latent tuberculosis infection (LTBI): currently available tests, future developments, and perspectives to eliminate tuberculosis (TB): The diagnosis of latent tuberculosis infection (LTBI). Med Lav Work Environ Health (2020) 111:170–83. doi: 10.23749/mdl.v111i3.9983
69. Yasui K. Immunity against mycobacterium tuberculosis and the risk of biologic anti-TNF-α reagents. Pediatr Rheumatol (2014) 12:45. doi: 10.1186/1546-0096-12-45
Keywords: THRIL, lincRNA-p21, biomarkers, diagnosis, tuberculosis
Citation: Ngongang NN, Mezajou CF, Kameni C, Ngum JA, Simo USF, Tatang FJ, Ngate Nguengo S, Chakam Nouthio AP, Wandji Pajiep MA, Toumeni MH, Takou Madjoumo ES, Tchinda MF, Ngangue RJEM, Dongmo FFD, Wade A, Akami M, Ngane Ngono AR and Tamgue O (2022) TNF and HNRNPL Related Immunoregulatory Long non-coding RNA (THRIL) and long intergenic noncoding RNA-p21 (lincRNA-p21) as potential useful biomarkers for the diagnosis of tuberculosis. Front. Trop. Dis 3:969307. doi: 10.3389/fitd.2022.969307
Received: 14 June 2022; Accepted: 15 August 2022;
Published: 21 September 2022.
Edited by:
Oluwatoyin Ajibola Asojo, Hampton University, United StatesReviewed by:
Jianhai Yin, National Institute of Parasitic Diseases, ChinaCopyright © 2022 Ngongang, Mezajou, Kameni, Ngum, Simo, Tatang, Ngate Nguengo, Chakam Nouthio, Wandji Pajiep, Toumeni, Takou Madjoumo, Tchinda, Ngangue, Dongmo, Wade, Akami, Ngane Ngono and Tamgue. This is an open-access article distributed under the terms of the Creative Commons Attribution License (CC BY). The use, distribution or reproduction in other forums is permitted, provided the original author(s) and the copyright owner(s) are credited and that the original publication in this journal is cited, in accordance with accepted academic practice. No use, distribution or reproduction is permitted which does not comply with these terms.
*Correspondence: Ousman Tamgue, b3VzdGhlbzIwMTRAZ21haWwuY29t
†These authors have contributed equally to this work
Disclaimer: All claims expressed in this article are solely those of the authors and do not necessarily represent those of their affiliated organizations, or those of the publisher, the editors and the reviewers. Any product that may be evaluated in this article or claim that may be made by its manufacturer is not guaranteed or endorsed by the publisher.
Research integrity at Frontiers
Learn more about the work of our research integrity team to safeguard the quality of each article we publish.