- 1La Follette School of Public Affairs, University of Wisconsin–Madison, Madison, WI, United States
- 2Nelson Institute Center for Sustainability and the Global Environment, University of Wisconsin–Madison, Madison, WI, United States
- 3Department of Atmospheric and Oceanic Sciences, University of Wisconsin–Madison, Madison, WI, United States
- 4Space Science and Engineering Center, University of Wisconsin–Madison, Madison, WI, United States
- 5Booz Allen Hamilton, Inc., at NASA Langley Research Center, Hampton, VA, United States
- 6GHGSat Inc., Houston, TX, United States
- 7Atmospheric Chemistry and Dynamics Laboratory, NASA Goddard Space Flight Center, Greenbelt, MD, United States
- 8International Institute for Applied Systems Analysis, Laxenburg, Austria
- 9Centro Euro-Mediterraneo sui Cambiamenti Climatici, Università Ca'Foscari, Venice, Italy
- 10RFF-CMCC European Institute on Economics and the Environment, Venice, Italy
- 11Battelle Memorial Institute, Columbus, OH, United States
- 12Department of Geography, University of Wisconsin–Madison, Madison, WI, United States
- 13DOE Great Lakes Bioenergy Research Center, University of Wisconsin–Madison, Madison, WI, United States
- 14Science and Technology Corporation, NASA Goddard Space Flight Center, Greenbelt, MD, United States
- 15Goddard Earth Sciences Technology and Research (GESTAR) II, Morgan State University, Baltimore, MD, United States
- 16Electric Power Research Institute, Palo Alto, CA, United States
- 17Department of Civil and Environmental Engineering, Princeton University, Princeton, NJ, United States
- 18Yale School of the Environment, Yale University, New Haven, CT, United States
- 19NASA Langley Research Center, Hampton, VA, United States
Transitioning to a sustainable energy system poses a massive challenge to communities, nations, and the global economy in the next decade and beyond. A growing portfolio of satellite data products is available to support this transition. Satellite data complement other information sources to provide a more complete picture of the global energy system, often with continuous spatial coverage over targeted areas or even the entire Earth. We find that satellite data are already being applied to a wide range of energy issues with varying information needs, from planning and operation of renewable energy projects, to tracking changing patterns in energy access and use, to monitoring environmental impacts and verifying the effectiveness of emissions reduction efforts. While satellite data could play a larger role throughout the policy and planning lifecycle, there are technical, social, and structural barriers to their increased use. We conclude with a discussion of opportunities for satellite data applications to energy and recommendations for research to maximize the value of satellite data for sustainable energy transitions.
Introduction
Actors across the energy system – from local, state, and national governments to electric utilities, technology developers, and a wide variety of energy end users – are grappling with options to limit the rise in global temperature to well below 2°C (and preferably 1.5°C) and achieve net-zero carbon dioxide (CO2) emissions targets (Hultman et al., 2020; Klemun et al., 2020). Meeting these ambitious goals will require far-reaching energy transitions in electricity, transportation, buildings, and industry (IPCC, 2018; Cui et al., 2019). Climate change is also shifting patterns in energy demand and increasing disruptions in energy access due to damage to infrastructure caused by extreme temperatures, floods, droughts, hurricanes, and other disasters. New sources of information are needed to support sustainable energy transitions and evaluate whether energy planning and policy decisions are effective and equitable (Carley and Konisky, 2020). By providing observations of Earth from space, satellite data hold new potential to address these global challenges.
Since the first satellite images were made publicly available in 1972, applications of satellite data have expanded significantly (Davis, 2007; Inman et al., 2013). Satellite data vary in spatial resolution (from tens of kilometers to less than a meter), frequency of observations (from weeks to minutes), and coverage (from continuous observations from geostationary satellites to global coverage from polar-orbiting satellites) (Medina-Lopez et al., 2021). There are trade-offs across these design features, with free, publicly available data from government sources tending toward global coverage and a growing number of private companies offering targeted observations of particular locations. Cloud-computing services further enhance the prospects for widespread use of satellite data by allowing broad user communities to process large amounts of data on the fly (Gorelick et al., 2017). Beyond the satellite technology itself, research has also advanced applications of satellite data to decision-making through comparisons with other data sources, integration with models, and case studies applying satellite data to particular contexts and examining barriers to use (Milford and Knight, 2017; Holloway et al., 2018)1,2.
Decisions related to energy supply, demand, impacts, and resilience all stand to benefit from growing integration of satellite data. Satellite applications for energy supply include mapping renewable resource potential to support infrastructure siting, development, and maintenance. Applications for energy demand include assessing energy use patterns to predict future needs and identify locations with unserved demand, both on an ongoing basis and in the aftermath of power disruptions. Applications for energy impacts include monitoring the effects of energy use on climate, air quality, and water and land systems, as well as efforts to reduce these impacts. Existing information sources used in the past have often been limited in spatial coverage and accessibility for a diversity of stakeholders and decision-making needs. These stakeholders also frequently lack access to timely information needed to support cross-cutting reliability and resilience goals, as well as disaster response. Expanded use of satellite data can now help address these information gaps.
This paper reviews the current state of satellite data for energy applications and potential future directions for research. We focus specifically on satellite tools for remote sensing because of their broad scale and routine measurements, as well as their underutilized potential for energy policy and planning. Each section presents an overview of conceptual and practical applications of satellite data, drawing primarily from the peer-reviewed literature. Applications vary in their level of maturity, from well-established uses with strong links to decision frameworks to emerging areas where there are significant technical, social, and/or structural barriers to applying satellite data to decision-making. While previous work examines satellite data for various energy applications in isolation, there is significant potential to increase the value of satellite data for energy decision needs by bridging insights across energy issues. Understanding the value and potential of satellite data to address energy-related challenges is particularly salient given the speed and scale of energy transitions required to mitigate and adapt to climate change.
The author team represents experts in a wide variety of energy and satellite topics from academia, government organizations, research institutions, and private companies. Following the introduction, we discuss satellite data applications in energy supply, energy demand, energy impacts, and energy resilience. We then describe an example of a satellite data distribution platform for energy users. We conclude with a discussion of the potential and limitations of satellite data across energy applications and recommendations for research to enhance the usefulness of satellite data for energy stakeholders.
Energy Supply
Many studies have quantified the enormous expansion in renewable energy needed to achieve global climate policy goals (IPCC, 2022). Satellite data can support the development, deployment, and forecasting of renewable energy sources such as bioenergy, hydropower, solar photovoltaics, wind turbines, and geothermal energy. Beyond assessing the potential for new systems, satellite data can also help optimize performance and track the rate of technology adoption.
Bioenergy Resources and Production
Satellite data are a leading source of information for policy and planning decisions related to bioenergy feedstock supply and productivity. Space-based data routinely inform assessments of biofuel feedstock availability and land use impacts, as well as potential competition with food production and impacts on other ecosystem goods and services. Productivity can be quantified from satellite observations of vegetation greenness and further constrained or refined using indirect satellite-based information on climate, soil conditions, and other co-determinants of productivity. Satellite-based estimates of land availability and supply have been used by industry, policymakers, and other bioenergy stakeholders in the evaluation and design of production systems and regulations.
Data on land cover have been used to identify abandoned agricultural lands with potential to support bioenergy feedstock production (Zumkehr and Campbell, 2013; Baxter and Calvert, 2017; Goga et al., 2019; Næss et al., 2021) and to screen for land that may be deemed as marginal for food production (Nalepa and Bauer, 2012; Kang et al., 2013; Khanna et al., 2021) due to economic instability (Jiang et al., 2021), environmental sensitivity (Wang et al., 2020), and biophysical limitations in climate, soils, or topography (Gelfand et al., 2013; Gu and Wylie, 2016). For example, satellite-based productivity thresholds on low-yielding lands have been used to identify marginal areas for second generation bioenergy production (Longato et al., 2019). From local to global scales, estimates of the maximum potential production of bioenergy can support energy planning and policy (Cai et al., 2011; Smith et al., 2012; Haberl et al., 2013). Bioenergy producers or investors can also use estimates of local feedstock supply (e.g., corn) to identify locations for siting future biorefineries.
Satellite-constrained estimates of total bioenergy production potential have also been used to project the contribution that bioenergy might make toward global climate policy goals or to meet national pledges to the Paris Agreement (IPCC, 2018; Creutzig et al., 2021). Policies such as the Low Carbon Fuel Standard and the Renewable Fuel Standard in the U.S. have used satellite-based estimates of land use change associated with bioenergy to measure and regulate greenhouse gas emissions intensity associated with different bioenergy systems, as well as to determine the eligibility of various fuels in each regulation (US EPA, 2010; Leland et al., 2018). Other work has used field-level remote sensing data to analyze changes in bioenergy feedstock supply caused by these policies, finding that the U.S. Renewable Fuel Standard, for example, led to an 8.7% increase in U.S. corn cultivation (Lark et al., 2022).
New data sources and advances in data science will open the door for highly detailed and precise ground-based data to complement data from satellites. For example, parcel-level data on land ownership and sales could enable a more refined understanding of how producers respond to policy and market incentives, and productivity measurements collected directly from agricultural equipment could significantly expand data availability. Nonetheless, satellite data will continue to provide irreplaceable information on bioenergy production that covers large geographic extents in a consistent manner over time, particularly with the increased availability of high-quality, high-resolution, and low-cost commercial and small-satellite platforms.
Hydropower and Water Supply
Satellite data are commonly used in water resource assessment for planning hydropower projects, monitoring reservoir size, and evaluating the environmental impacts of rerouting or damming water. Hydrological and hydrometeorological variables, such as precipitation, snow extent, soil moisture, runoff, and evapotranspiration, influence the availability of water resources to support power generation. Hydropower currently accounts for ~60% of global renewable electricity production and is projected to play a major role in flexible power systems as the world transitions to cleaner energy sources (International Hydropower Association, 2021). Planned hydroelectric projects also dominate the renewable energy sector in sub-Saharan Africa, where significant untapped potential exists (Stiles and Murove, 2019), offering opportunities for new uses of satellite data products (Leibrand et al., 2019). Tracking and monitoring water resources is critical to ensuring and managing future water supply, especially given projected changes in water resources due to climate change (Fletcher et al., 2019).
Landsat and Terra satellites have been collecting environmental and climate data for several decades and provide a long historical record to help identify trends and spatial patterns in river flow, snow melt, land cover, and other variables that impact water availability, which is useful in decision-making for hydropower operations (see Figure 1 for an example). Other satellites provide data in near real time, such as the National Aeronautics and Space Administration's (NASA's) Soil Moisture Active Passive (SMAP) mission, which measures global soil moisture in increments as short as 3 hours, with a latency of 24 hours and a revisit time of 2–3 days, thus reducing the need for field evaluation.
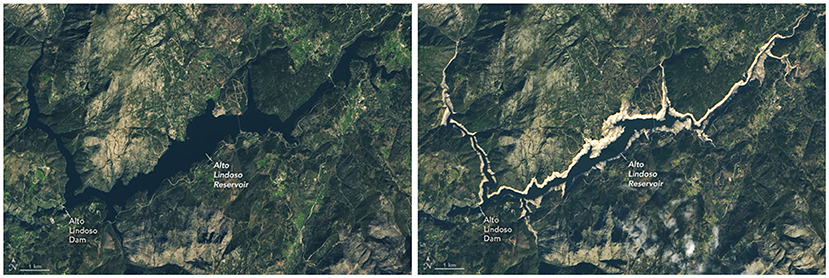
Figure 1. Tracking the impact of drought on a hydropower reservoir at the Alto Lindoso Dam in Portugal from March 6, 2021 (L) to February 5, 2022 (R), using Landsat 8 data [Credit: NASA Earth Observatory image by Lauren Dauphin, using Landsat data from the U.S. Geological Survey (NASA Earth Observatory, 2022)].
Satellite-based data on groundwater, surface water height and extent, and precipitation may be used to assess seasonal and historical changes in water storage. Freeze-thaw data derived from satellite microwave radiometry from NASA's Scanning Multichannel Microwave Radiometer (SMMR), Special Sensor Microwave/Imager (SSM/I), and Advanced Microwave Scanning Radiometers (AMSR-E and AMSR2) have been used to evaluate the dynamics of seasonal snow, ice melt, and soil thaw as a proxy for measuring water mobility over time (Kimball and McDonald, 2020). Taken together, satellite-derived hydrological and hydrometeorological data can identify trends in water availability, potential for flooding and drought, and other environmental aspects for improved decision-making in the hydropower sector.
Machine learning and data assimilation are advancing data analysis to improve observations for hydropower in areas where ground-based data are scarce. For example, machine learning has been combined with near-real-time rainfall data from NASA's Tropical Rainfall Measuring Mission (TRMM) and soil moisture data from the National Oceanic and Atmospheric Administration's (NOAA's) Advanced Scatterometer (ASCAT) to simulate streamflow in India (Kumar et al., 2021). Machine learning with various satellite-derived hydrometeorological variables has also been used to calculate streamflow in the Hanjiang River in China (He et al., 2021). Data assimilation, another approach to data fusion, has also improved land surface model predictions of water storage, particularly when multiple satellite data products are combined (Khaki et al., 2020).
There are new opportunities to use satellite data for hydropower planning and management (International Hydropower Association, 2020). NASA, NOAA, the European Space Agency (ESA), and other Earth observing organizations provide open-source data and offer training on how to apply data to real-world decisions, working to reduce barriers to use and accessibility. The value of these data is especially high in regions with gaps in ground-based data and with high climate variability, where uncertainties in water resources present challenges for hydropower planning and operations.
Solar Photovoltaic Systems
Satellite data have long been used to measure annual solar insolation in conjunction with ground-based pyranometer data (Perez et al., 2013). For example, the U.S. National Solar Radiation Data Base (NSRDB) from the National Renewable Energy Laboratory (NREL) uses data from the NOAA Geostationary Operational Environmental Satellite (GOES), NASA Moderate Resolution Imaging Spectroradiometer (MODIS) instrument, and NASA's Modern-Era Retrospective analysis for Research and Applications Version 2 (MERRA-2) assimilation model to create a dataset that shows historical levels of solar energy resources in any location in the U.S. (Sengupta et al., 2018). The multiple source dataset goes back to 1998 at a temporal resolution of half an hour. Additionally, global solar radiation data are made available back to the early 1980's using fused geosynchronous and polar orbiting satellites, including data products available since 2020 from NASA's Clouds and Earth's Radiance Energy System (CERES) (Zhang et al., 2004; Rutan et al., 2015; Karlsson et al., 2017; Stackhouse et al., 2021).
With increased penetration of variable wind and solar power on the grid, there is a new focus on system performance and short-term wind and solar resource forcasting (Janjai et al., 2011; Pfenninger and Staffell, 2016; Peters et al., 2018). For example, machine learning has been used to predict cloud velocities to understand where drops in photovoltaic (PV) system production might occur (Cheng et al., 2022), and satellite-derived aerosol levels may be used to assess the impact of air pollution on PV arrays (see example in Figure 2) (Li et al., 2017). Local decision-makers can also use satellite-derived maps to inform cost-effective renewable energy project upkeep, such as vegetation management (Yu et al., 2018).
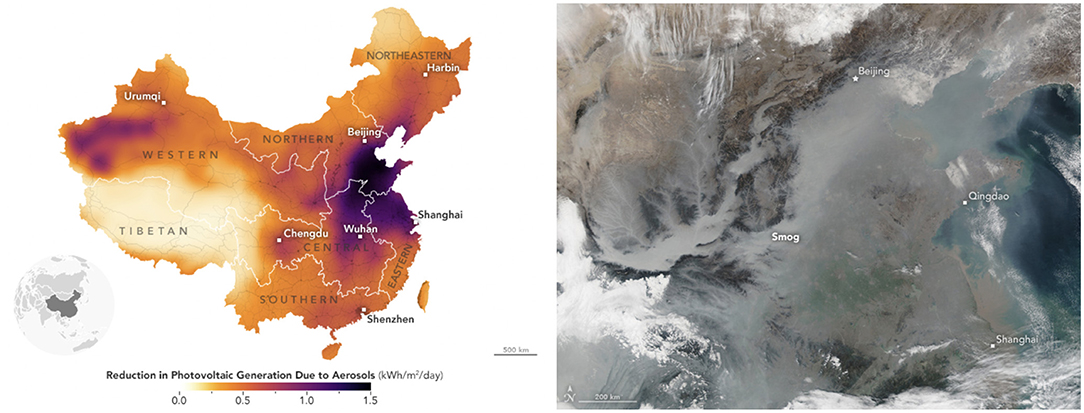
Figure 2. (L) Impact of aerosols on the average amount of radiation reaching the land surface of China between 2003 and 2014 [Credit: Joshua Stevens, NASA Earth Observatory, using data from Li et al. (2017)]. (R) Natural-color image of haze over eastern China from the Visible Infrared Imaging Radiometer Suite (VIIRS) on the Suomi National Polar-orbiting Partnership (NPP) satellite on January 25, 2017. (Credit: Jeff Schmaltz, NASA, LANCE/EOSDIS).
Satellite data can also be used to track renewable energy deployment, assess solar access disparities, and potentially support third party validation of renewable energy adoption under climate agreements (see example in Figure 3)3. Standard solar PV accounting methods generally focus on limited regions and often miss smaller systems. Satellite image processing offers an efficient method for tracking growth in solar energy across large geographic areas (Kruitwagen et al., 2021), but smaller residential and microgrid systems are still difficult to track (Ishii et al., 2016).
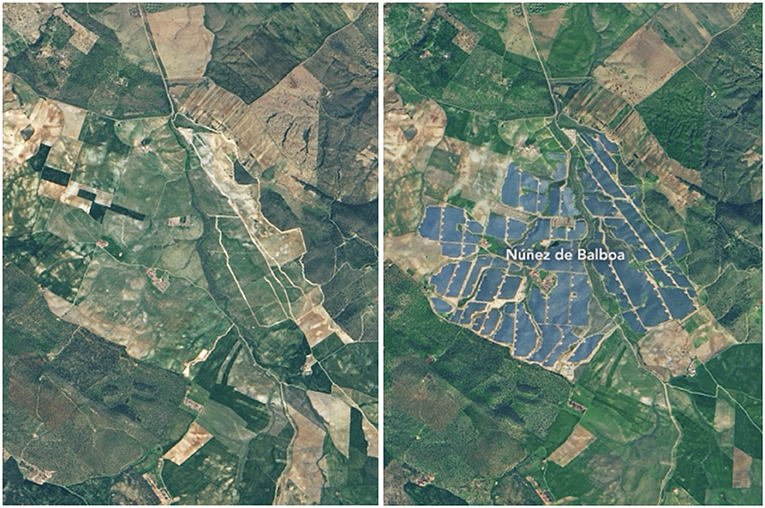
Figure 3. Satellite images of a 500 MW solar power plant on the Iberian Peninsula. (L) shows imaging before installation in 2020, (R) shows imaging after installation (Credit: NASA Earth Observatory image by Lauren Dauphin, using Landsat data from the U.S. Geological Survey).
Offshore Wind Projects
Using traditional in-situ measurements such as buoys to measure offshore wind resources is expensive, time consuming, and limited in its geographic coverage. As an alternative, synthetic aperture radar (SAR) data from satellites is being used to estimate wind power from wave heights and direction. Recent efforts have focused on improving the SAR method's accuracy. For example, calibrating satellite data on sea winds can help improve estimates of wind speeds (Soukissian and Papadopoulos, 2015), and advanced data analysis methods like machine learning can help predict wind energy production (Majidi Nezhad et al., 2021a). To estimate wind energy at actual wind hub heights (~100 m), near sea-surface (~10 m) wind readings from the ESA Envisat Advanced Synthetic Aperture Radar (ASAR) are used to extrapolate wind speeds at greater elevations (Badger et al., 2016). In areas impacted by wake effects, SAR data available from missions such as the Envisat ASAR and Sentinel 1 can also measure wind speeds (Ahsbahs et al., 2018).
Although satellites cannot “see” future winds, satellite data can be used to improve forecasts of wind resource availability for wind projects (Inman et al., 2013). In offshore applications, SAR data can be used to constrain short-term weather predictions and provide temporally and spatially expansive estimates of wind speeds and wave heights (Zen et al., 2021). Both measures are important for the design, planning, and operation phases of offshore wind projects, including efficiently screening for promising offshore wind resource areas and reducing uncertainty around installation weather windows. Future areas for research include improving the spatial resolution of wave and wind detection, as the current practice is to assume similar conditions across an entire wind farm based on a limited set of estimates (Medina-Lopez et al., 2021). Additionally, inter-hour offshore wind resource forecasting is becoming more critical as coastal power grids rely on greater penetration of offshore turbines, which recent satellite products, such as ESA's Aeolus mission, will help improve (Medina-Lopez et al., 2021).
Geothermal Energy
Satellite data is supporting the exploration and monitoring of geothermal energy sources, which have the potential to provide non-emitting baseload power (Vargas et al., 2022). Remotely sensed thermal infrared data has been used since the 1980's to detect geothermal activity and identify potential sites for geothermal plants, providing a less costly data source than field investigations (Majidi Nezhad et al., 2021b). Thermal infrared bands that are sensitive to surface temperatures are used to identify anomalies that are potentially the result of subsurface geothermal activity. Instruments that have been used for geothermal prospecting include MODIS, the Advanced Spaceborne Thermal Emission and Reflection Radiometer (ASTER), Landsat's Enhanced Thematic Mapper Plus (ETM+), and SAR (Howari, 2015). For example, one recent study used ASTER data to map geothermal potential along a section of the East African Rift System, where previous mapping coverage was limited, using a combination of surface temperature estimates and indicator minerals (Hewson et al., 2020).
The coarse resolution of thermal sensors provides a means to target field activities but limits their usefulness to broader scale detection of geothermal anomalies. However, satellite data can be useful for studying geothermal potential and ground temperature recovery because of the ability to construct long-term datasets. A key tool that allows for this type of analysis is the Interferometric Synthetic Aperture Radar (InSAR) technique, which can map ground deformation through clouds and at night, providing expansive temporal and spatial coverage (Mellors et al., 2018; Majidi Nezhad et al., 2021b). For example, two years of Sentinel-1 SAR data was used to analyze Iceland's untapped geothermal energy, as well as pressure changes from geothermal fluid extraction for a new power plant (Receveur et al., 2019). Future research can look to relate satellite-derived prospecting with existing geothermal data (or exploratory drilling) to improve data relevance to future geothermal applications (Howari, 2015).
Energy Demand
Tracking energy demand, both temporally and spatially, is critical to a just and sustainable energy transition. Nighttime lights (NTL) data have been actively used to monitor energy use and electrification and identify gaps for further policy development. With 770 million people worldwide without access to electricity, and many others lacking reliable and affordable heat and power (Hernández, 2015; Reames, 2016; IEA, 2021c), NTL data may be the most important data product to inform decisions to support energy access and restoration.
Energy Use and Infrastructure
Nighttime lights are a widely used indicator of energy use and infrastructure (NASA Earthdata, 2021) and have been correlated with economic activity, urbanization, population density, and energy consumption and access (Falchetta and Noussan, 2019). There are two principal datasets that provide NTL. The first digital NTL dataset is available from 1992-2013 through the Defense Meteorological Satellite Program-Operational Linescan System (DMSP-OLS). However, each pixel in these images has only 64 potential values, a consequence of the 6-bit radiometric resolution of the satellite instrument. Due to this limited range, the data become saturated when NTL levels are high, especially in urban areas, limiting NTL applications to planning and policy at the city scale. Limited low-light detection also curtails NTL utility in dimly lit regions such as rural areas. The 2.7 km spatial resolution further limits energy-related applications at local scales.
The second and more recent NTL dataset is developed from the VIIRS Day Night Band (DNB) onboard the Suomi-NPP satellite, launched in 2011. VIIRS NTL is a significant improvement over DMSP-OLS NTL in two ways: the spatial resolution is much improved at 750 m, and the sensor has a larger dynamic range, with improved calibration that allows for accurate measurements of very low and high intensity nighttime lights. Recent advances to harmonize the DMSP and VIIRS NTL data have made them easier to access and integrate for wider applications (Li et al., 2020a).
For scientific studies, the most robust NTL dataset is Black Marble, which uses raw VIIRS data and corrects for atmospheric and radiometric issues (Romn et al., 2018). These data are calibrated across time, validated against ground-based data, and available at daily resolution. NASA scientists are currently working on a high-definition version of Black Marble, which will allow researchers to downscale NTL data at finer spatial resolutions by integrating Landsat and Sentinel Earth observations and street-level GIS data into the Black Marble product, and thereby improve NTL visualization in dense urban areas (NASA Goddard Space Flight Center, 2021). In 2021, the World Bank created the Light Every Night dataset, which is a complete archive of all NTL data collected over the past three decades (Min et al., 2021)4. Higher-resolution NTL images are available via photographs taken from the International Space Station (ISS) and the private company NOKTOsat (de Miguel et al., 2014; Noktosat, 2021). Additional sources of NTL data exist, but many are not publicly accessible (Li et al., 2019).
The combination of finer spatial, radiometric, and temporal resolutions, as well as integration of new data sources and processing techniques, can provide near-real-time estimates of energy use. To evaluate energy access, NTL data may be combined with on-the-ground information from utilities, GIS data, and local knowledge of energy access (Zhao et al., 2019). Fusing satellite data products with data from mobile phones can also support assessments of energy use, energy poverty, and disaster response (Steele et al., 2017), while NTL data combined with census data, national household surveys, or meter data can help users better understand and address inequities in energy infrastructure and access at scale (Mann et al., 2016; Pandey et al., 2022). Satellites can also identify changes in energy demand, such as those associated with COVID-19 or holidays from different cultures (Román and Stokes, 2015; Elvidge et al., 2021; Stokes and Román, 2022).
There are several important limitations to the use of NTL as an indicator of energy and other socioeconomic variables to inform policy. Broadly, NTL are an imperfect proxy for energy use and access. Current satellite data products cannot accurately measure energy use at smaller scales relevant for many policy questions, such as at household, street, or neighborhood levels, especially in high-density areas (Falchetta et al., 2020a). NTL data are less accurate for measuring electrification in areas where energy supply is intermittent, as conventional uses of NTL and other satellite observations are often binary (i.e., the lights are on or off) (Dugoua et al., 2017). Streetlights, car lights, and LED lighting may also make an area appear more or less electrified than it truly is (Zhao et al., 2019). Finally, NTL data may be more appropriate for estimating energy and other variables in some regions than others (Zhu et al., 2019a). For example, in areas with fires or oil and gas flaring, NTL may reflect these sources rather than electrification.
Global Energy Access
Over the past few decades, countries around the world have made large investments to support the goal of universal energy access and improve the reliability of electricity supply (Aklin et al., 2018), yet access to electricity and modern cooking fuels and technologies remains low in some regions (World Bank, 2019). The main gaps are found in sub-Saharan Africa (570 million lacking electricity), Central and Southern Asia (103 million), and Southeast Asia (40 million) (World Bank, 2019). While these regional statistics provide a general understanding of the existing gap, it is critical to develop tools to map the geographic distribution and temporal dynamics of these populations to provide a fine-grained, up-to-date understanding of electricity access across the world.
Tracking of energy poverty and access has generally been carried out through household surveys administered by national governments and international organizations. Satellite-based NTL data can serve as a proxy for electricity access to support electrification planning, complementing traditional survey methods (see example in Figure 4) (Min et al., 2013; Burlig and Preonas, 2016; Dugoua et al., 2017; Fobi et al., 2018; Avtar et al., 2019). These data are often combined with data on population density and other socioeconomic indicators (Stokes and Seto, 2019; Zhao et al., 2019; Falchetta et al., 2020b). NTL data have shown that lack of electrification is most pronounced in countries where a large proportion of the population lives in dispersed, rural settlements with few resources (Doll and Pachauri, 2010). However, these data also suggest that energy access can decline in urban areas that were once more reliably electrified as utilities struggle to keep pace with increasing energy needs associated with rapid urbanization, especially peri-urban areas and informal settlements (Falchetta et al., 2020b).
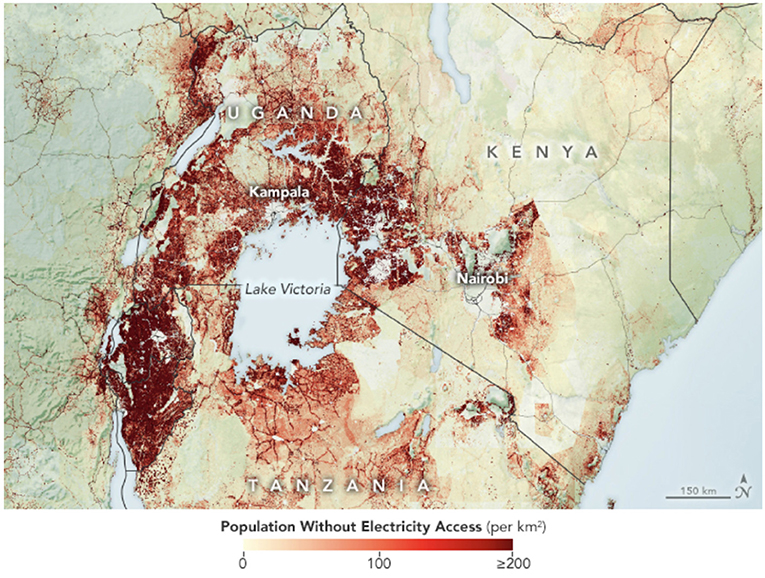
Figure 4. Estimate of the number of people without access to electricity in East Africa using data from the VIIRS instrument on the NOAA-NASA Suomi NPP satellite, land cover type data from NASA's MODIS instrument, and data from Oak Ridge National Laboratory's LandScan. [Credit: NASA Earth Observatory image by Lauren Dauphin, using data from Falchetta et al. (2019, 2020a) (NASA Earth Observatory, 2021a)].
Nighttime lights have also been combined with utility data to inform renewable energy and microgrid infrastructure planning, as well as electrification of essential services such as healthcare facilities (Korkovelos et al., 2019; Moner-Girona et al., 2019, 2021). If utility data are unavailable (e.g., after a natural disaster or in rural or low-resource settings), NTL data can be used as a proxy to estimate energy access (Fragkias et al., 2017). The stability of NTL radiance over time has also allowed it to be used to evaluate supply reliability and to measure the impact of hydroelectricity disruptions due to drought events (Arderne et al., 2020; Falchetta et al., 2020b). These studies seek to go beyond the binary classification of energy access and lack of access, which is crucial as energy poverty is a multi-dimensional challenge (Pelz et al., 2018, 2021). Thus, despite limitations of NTL data, its usefulness for understanding energy access continues to grow.
Sub-Saharan Africa stands to particularly benefit from the use of NTL data for electrification planning. Lack of energy access and unreliable electricity have hampered economic growth, and policymakers across the region face the challenge of expanding energy access to almost half the continent (IEA, 2019). Using NTL, population, and settlement data, one study estimated that between 2014 and 2019, 115 million people in sub-Saharan Africa gained access to electricity. However, in some cases, energy access did not equate to energy use, and some countries that had made strides in expanding access saw limited use in newly electrified households (Falchetta et al., 2019, 2020a). These studies highlight that increases in access must be accompanied by increases in generation and grid infrastructure to improve the quality and reliability of electricity that is delivered to households (Falchetta et al., 2020b).
Improvements to NTL data, primarily via increases in resolution and reductions in uncertainty as instruments and algorithms advance, will enable broader data use by policymakers, utility managers, emergency response personnel, and other stakeholders. Usefulness of NTL data can be further improved with integration of GIS maps and geoprocessing tools (Dugoua et al., 2017). Data at finer resolutions will also increase the usability of NTL and expand applications in which it can be used. With higher spatial and radiometric resolution, and finer time scales of collection, researchers can start to examine a range of issues related to the quality and consistency of energy availability – not just whether energy infrastructure exists, but the frequency (reliability) of lighting and how quickly lighting is restored after a major disaster such as a hurricane (Romn et al., 2019), blackout, or conflict. Similarly, these data can be used to track the urban development process and to identify locations that have inadequate energy infrastructure (Stokes and Seto, 2019).
Urban Areas and Urbanization
Urban areas account for approximately 75% of global final energy use, and this demand is strongly correlated with urban form and structure (Seto et al., 2014). Therefore, characterizing urban areas can inform estimates of energy demand, even at the global scale, and can be useful in planning future energy investments to support sustainability and other goals. Urban expansion can lead to categorical changes in land cover, such as when agricultural areas become urban, as well as magnitude changes, such as urban intensification. The distinction between measurement of categorical vs. magnitude changes is important because the optimal methods and reliability of estimates differ between the two. Measuring categorical change is typically easier than measuring the magnitude of urban change.
The majority of published studies have focused on mapping two-dimensional urban expansion, or outward urban growth (Zhu et al., 2019b; Reba and Seto, 2020). It is only in the past decade that the research community began to examine volumetric growth of urban areas (see Figure 5 for an example). Three-dimensional characterization of the built environment reveals more about urban form, structure, and resource use, such as the demand for reinforced steel and concrete or embodied and operational energy use. Backscatter data from the QuikSCAT SeaWinds scatterometer have been shown to be able to characterize urban volumetric infrastructure growth for large cities (Frolking et al., 2013; Creutzig et al., 2016; Mahtta et al., 2019; Li et al., 2020b). The recent development of a time series with ERS, QuikSCAT, and ASCAT backscatter data covering three decades will enable new studies of urban built structures and their energy implications (Frolking et al., 2022).
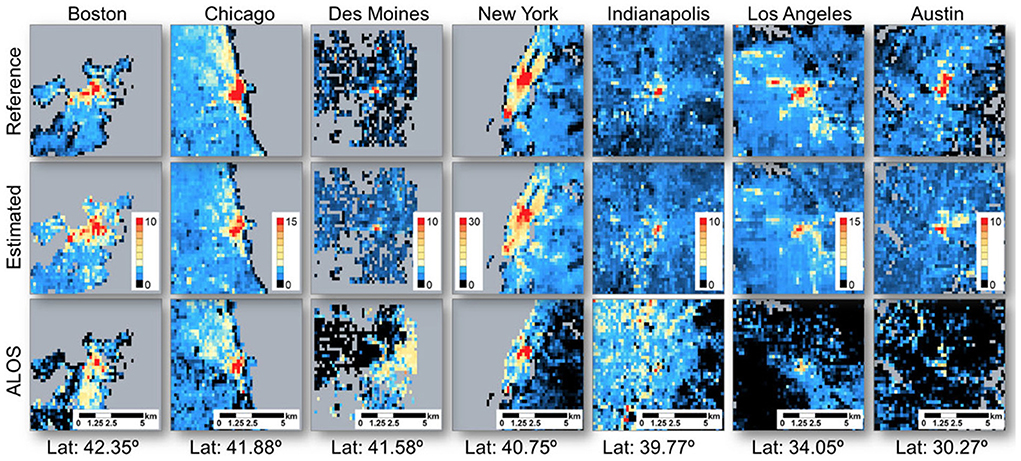
Figure 5. Estimated spatial patterns in building heights in seven U.S. cities using Sentinel-1 data, compared to reference (non-satellite) measurements and Advanced Land Observing Satellite (ALOS) data (unit: m). Measurements are presented at 500 m resolution with a spatial extent of 20 × 20 km. Areas outside the study domain are colored as gray. [Credit: Li et al. (2020b)].
A recent review of algorithms to detect, characterize, and monitor urban land changes found that most methods have been developed and applied for only a few regions (e.g., the U.S. and China), with 75% of studies focused on high-income or upper-middle-income countries (Reba and Seto, 2020). Furthermore, while 11% of the world's urban population lives in cities with populations greater than 5 million, 41% of studies have focused on these very large cities, whereas most future urban growth will occur in towns and cities with populations of less than 1 million (Seto et al., 2014). Applying satellite data to urban growth at smaller scales could support urban planning and policies related to these growing sources of urban energy demand and resource use.
Energy Impacts
Energy impacts on land, water, and air quality have long histories of regulation and management in environmental policy. Even for these well-established contexts, satellite data introduce new opportunities and challenges in connecting with decision frameworks. Extending the relevance of satellite data to greenhouse gas emissions is a growing area of research, recognizing the complexities in connecting space-based detection of gases with on-the-ground decision needs (Esparza and Gauthier, 2021).
Land and Water Impacts
Land use and water represent two of the largest impacts of crop-based bioenergy production as well as mining and other infrastructure for fossil fuels and nuclear energy. Satellites offer the potential to track and monitor energy-related impacts on land and water, which are key to successful resource management and disaster response. Because bioenergy systems rely on large amounts of biomass feedstocks, typically grown on land, they can result in particularly large land and water impacts. These impacts include direct changes in land use as well as indirect impacts via price effects that lead to expansion or contraction of crops used for biofuels or other purposes. Land use associated with bioenergy systems can also have ensuing consequences for biodiversity, water quality and use, and CO2 emissions (Berndes et al., 2013; Popp et al., 2014). Satellite data can inform assessments of these impacts, as well as emissions from bioenergy and fossil fuel infrastructure, including refineries and power plants.
Satellite data have been instrumental in tracking patterns in land use and land cover change associated with existing bioenergy development. For example, the expansion of corn ethanol production in the U.S. has led to increases in corn cultivation, with satellite data being used to monitor resulting changes in crop rotations, land conversion, and participation in land conservation programs (Brown et al., 2014; Motamed et al., 2016; Wright et al., 2017). These changes may also contribute to shifts in water quality, which can be monitored directly by satellites or modeled using satellite data on land use, climate, and other environmental determinants (Haag et al., 2009; Hendricks et al., 2014). Similarly, satellite data have been used to track the expansion of palm oil, intended for biodiesel and other market uses, across the tropics (Koh et al., 2011; Carlson et al., 2012). These data have helped identify solutions to stymie the widespread environmental consequences of palm oil on rainforests, biodiversity, and local communities (Rose et al., 2015; Leidner and Buchanan, 2018; Meijaard et al., 2020).
Satellite data can also support interventions to minimize the environmental impacts of energy infrastructure on natural habitats and existing land conditions. For example, a pilot study from the Electric Power Research Institute (EPRI) tested the use of satellite data in identifying the effects of energy infrastructure on monarch butterfly habitats and wetlands (Madsen, 2021)5. Another analysis used satellite data to monitor impacts along Azerbaijan gas and oil pipeline right-of-ways (ROW) spanning 10 million square miles (Bayramov, 2013).
Satellite data have also been used to assess the impacts of energy systems on water quality, particularly those arising from thermal power plants (i.e., bioenergy, fossil fuels, and nuclear). For example, studies have used satellite data to estimate water demand (Luo et al., 2018), monitor thermal discharge from power plants (Wu et al., 2007), detect turbidity (Alkan, 2009), and estimate water quality impacts (Sridhar and Vincent, 2009). Remotely sensed data have also been used as inputs to advanced modeling and prediction of water quality outcomes from bioenergy production. Off the coast of the U.S., the size of the Gulf of Mexico hypoxic zone, the world's second largest oxygen-depleted “dead zone” (Dybas, 2005), can be tracked and modeled using satellite data (Haag et al., 2009), and contributions from changes in bioenergy-related land use can be estimated using satellite-based inputs (Donner and Kucharik, 2008; Hendricks et al., 2014). Similarly, satellite data have been used to track the size and occurrence of harmful algal blooms (Klemas, 2012; Shen et al., 2012) and estimate the contribution of bioenergy to water quality impairments (Hamada et al., 2015; Lin et al., 2015; Chen et al., 2017). Satellite data have also been critical in real-time monitoring of oil spills (see Figure 6).
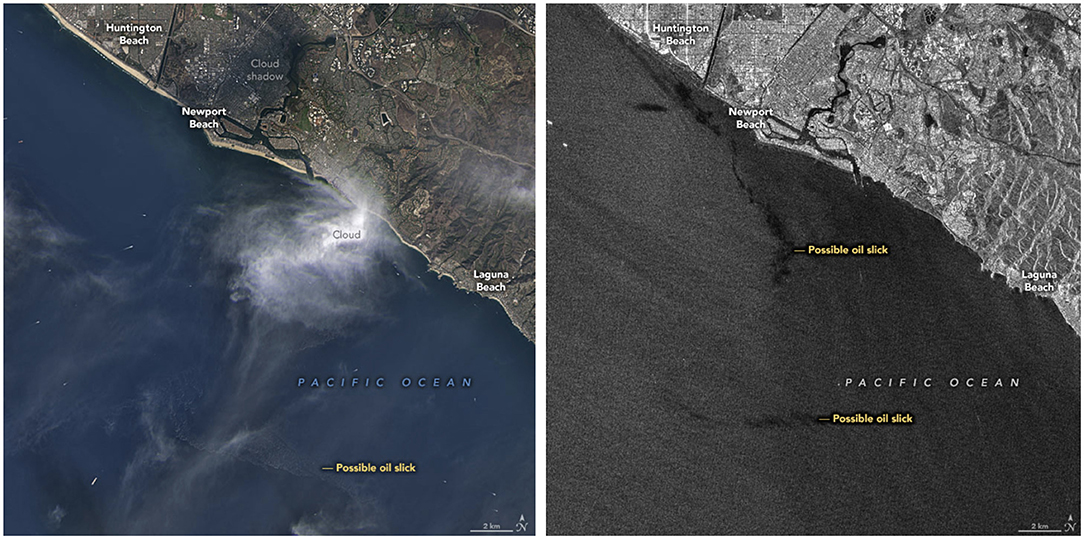
Figure 6. Satellites were able to spot an oil slick from a major oil spill in Southern California in 2021. (L) is an image from October 2, 2021, from OLI on Landsat 8, and (R) is a SAR image from the ESA Sentinel-1B satellite. [Credit: NASA Earth Observatory image by Joshua Stevens, using Landsat data from the U.S. Geological Survey and modified Copernicus Sentinel data processed by the ESA (NASA Earth Observatory, 2021b)].
Satellite-based assessments can inform water resources conservation and planning for energy and other uses (Bastiaanssen et al., 2012). For example, analyses of evapotranspiration can inform estimates of potential water use associated with bioenergy feedstock production (Bhattarai et al., 2017; Wagle et al., 2017). These estimates can also be compared to the evapotranspiration of alternative (e.g., food) crops or native ecosystems, and inform assessments of the overall water use intensity of bioenergy feedstocks relative to other energy systems and land uses (Sanders and Masri, 2016).
The ability of satellites to capture frequent observations of changes in land and water use creates exceptional opportunities to evaluate the causal outcomes of energy policies, many of which began after routine satellite data collection (Blackman, 2013; Donaldson and Storeygard, 2016). Publicly available, space-based data can provide transparency and credibility for certification schemes that go beyond industry-reported results. For example, the Roundtable for Sustainable Palm Oil (RSPO) certification schemes rely on satellite technology to strengthen fire prevention efforts and protect forests (RSPO, 2021). Bonsucro's certification scheme for sugarcane production, which is used as a feedstock for ethanol, also relies on satellite data to map changes in land use (Bonsucro, 2021).
Health and Air Quality Impacts
A wide range of gas and particle species are emitted from fossil fuel combustion in the energy system, especially nitrogen oxides (NOx), carbon monoxide (CO), and sulfur dioxide (SO2), as well as suspended liquid and solid particles, referred to as particulate matter (PM). These traditional air pollutants represent the most direct linkage between energy policy and health outcomes. The World Health Organization (WHO) estimates that 92% of the global population lives in areas where air quality levels exceed WHO limits (World Health Organization, 2016), and 4.2 million people die each year due to outdoor air pollution6.
In many ways, the experience of the air quality and health communities serves as a success story for satellite data integration into existing energy-related decision frameworks (Holloway et al., 2021). As satellite technology advanced to detect gases and particles in the atmosphere, early research highlighted the potential for these datasets to inform model evaluation, support improved emission inventories, and assess surface abundance of health-relevant pollutants. In 2011, NASA launched the first Applied Sciences team around the theme of air quality (Jacob et al., 2014), which was expanded to address health and air quality in 2016 (Holloway et al., 2018) and renewed in 2021. The three generations of these teams represent a systematic research and outreach enterprise, wherein applied research projects have advanced rapidly over the past 10 years, in collaboration with stakeholder partners.
These experiences highlight key areas where satellite data can inform energy-related air quality and health issues (World Health Organization, 2016). Nitrogen dioxide (NO2) has emerged as perhaps the most useful air quality indicator from satellites, which has been used as an indicator of NOx emissions, including trends in NOx emissions associated with emission controls on power plants as well as transportation patterns, fuel shifts, and economic changes. As an example, satellite NO2 from the TROPOMI instrument was used as an indicator of energy use changes during the early stage of the COVID-19 lockdowns in early 2020 (see Figure 7) (NASA Earth Observatory, 2020).
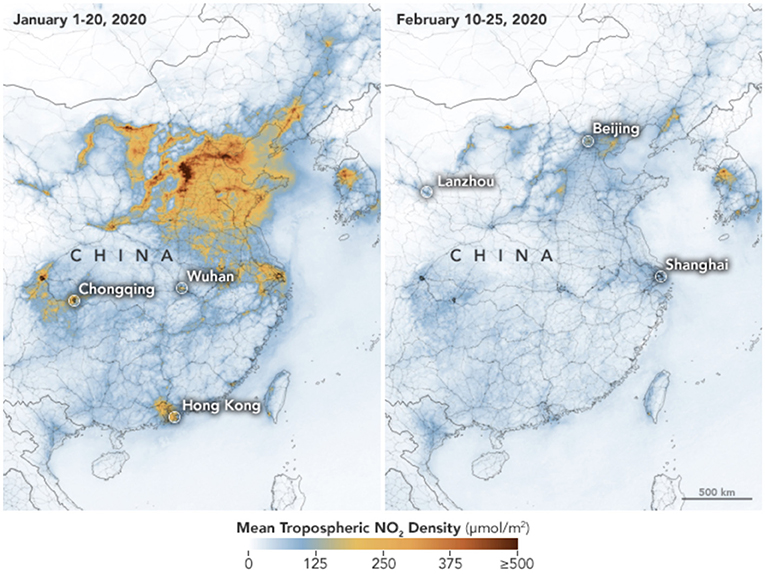
Figure 7. Changes in NO2 concentration due to COVID-19 lockdown in China using data collected from TROPOMI from ESA's Sentinel-5P satellite (Credit: NASA Earth Observatory images by Joshua Stevens, using modified Copernicus Sentinel 5P data processed by the ESA).
Because most NO2 in the troposphere is emitted near the surface, the column abundance detected by satellites is well-correlated with concentrations detected by ground monitors (Goldberg et al., 2021). Furthermore, the short atmospheric lifetime of NO2 limits mixing of the pollutant in the atmosphere, such that satellite images capture the sources of emissions and track closely with spatial patterns in combustion activities at the ground level. Satellite NO2 has been used to evaluate health outcomes from NO2 (Anenberg et al., 2022) and environmental justice dimensions of air pollution exposure (Kerr et al., 2021). NO2 is also a key ingredient in ozone production near the surface, and thus an important factor in ozone control strategies (Duncan et al., 2010; Witman et al., 2014).
Many other chemical species observed from space bear relevance to energy emissions, air quality, and health. For example, satellite-derived SO2 can be an important indicator of power plant emissions (Lu et al., 2013), satellite observations of CO show the impact of global pollution transport (NASA, 2015), and satellite observations of “aerosol optical depth” have been used quantify global exposure to fine PM (van Donkelaar et al., 2010). Beyond tracking fuel combustion, satellite data have been used to assess upstream emissions from energy processes, such as dust impacts of cropland expansion from bioenergy (Lambert et al., 2020) and air emissions associated with the pre-harvest sugarcane field burning phase of ethanol production in Brazil (Tsao et al., 2012).
Oil and Gas Emissions
Oil and gas operations release a wide range of chemical emissions, including volatile organic compounds (VOCs, associated with ozone formation and also posing direct health risks) and the powerful greenhouse gas methane. Both the federal government and some states in the U.S. are beginning to consider satellite data to assess oil and gas VOC emissions for regulatory purposes. A proposed rule in New Mexico incentivizes the use and development of new technologies for leak detection and repair (LDAR) such as remote monitoring via satellites or aircraft, aiming to increase the accuracy and speed of reporting as part of their ozone control effort. Colorado provides operators with the opportunity to submit an Alternative Approved Instrument Monitoring Method (AIMM) for identifying VOC ozone precursors. These changes provide the opportunity to include other monitoring methods as an alternative to current ground-based measurement approaches.
Methane emissions from oil and gas systems have been the subject of significant recent policy action. At the 2021 United Nations Climate Change Conference (COP26), more than 100 countries joined the U.S. and EU in launching the Global Methane Pledge, an initiative to reduce methane emissions by at least 30% from 2020 levels by 2030 (European Commission, 2021). Satellite-based inventory methodologies can play a crucial role in achieving these goals by providing timely data for monitoring and verifying country commitments. The United Nations Environment Program (UNEP) is supporting this effort through the International Methane Emissions Observatory (IMEO), which will use multiple data sources from satellites, ground-based sensors, and national and company inventories (UN Environment Programme, 2021). These data can be combined to identify and reconcile gaps and inconsistencies and enable global stakeholders to track whether emissions reductions are being achieved and take targeted action.
Satellites can monitor oil and gas infrastructure on a frequent basis with an emphasis on high-risk areas, quickly detecting very large emissions sources. The natural gas supply chain is characterized by super-emitter behavior, where a small percentage of sources are responsible for the majority of emissions. A meta-analysis of approximately 15,000 measurements from 18 individual studies in the U.S. showed that the largest 5% of methane leaks typically contribute over 50% of the total emissions by volume (Brandt et al., 2016), and similar phenomena have been observed for individual production sites (Zavala-Araiza et al., 2017) and across sources and sectors (Duren et al., 2019). A recent study also used satellite data to identify large methane releases from “ultra-emitters” worldwide (Lauvaux et al., 2022).
Policies targeting super-emitters could be a cost-effective strategy for reducing overall emissions (Ravikumar et al., 2020; Edwards et al., 2021). Multiple types of measurements can work together to assess methane emissions in a tiered system-of-systems approach, integrating space-based platforms with airborne instruments and ground sensors (Esparza and Mattson, 2021). This tiered approach can enable more complete monitoring, detection, and repair of emissions sources without the need to deploy an impracticably large number of ground-based sensors, consistent with other examples of using satellite data to complement other measurement approaches.
In recent years, greenhouse gas monitoring satellites from the private sector have complemented technology from government space agencies7. For example, the company GHGSat currently has three methane sensing satellites in orbit with spatial resolutions as low as 30 m, allowing for detection of point sources such as individual oil and gas wells. GHGSat has an ongoing collaboration with the Netherlands Institute for Space Research (SRON) whereby elevated methane levels detected by TROPOMI, which makes measurements in 2,600 km swaths at 7 km resolution, are followed up with high-resolution GHGSat imagery that can attribute these methane hot spots to specific facilities (European Space Agency, 2020).
In early 2019, a GHGSat satellite was imaging a natural source of methane emissions known as a mud volcano in the western part of Turkmenistan when it serendipitously discovered an enormous methane leak – assessed to have been 10,000 to 43,000 kg/hour – from a compressor station at the nearby Korpezhe oil and gas field. Other nearby leaks of similar magnitudes were also identified. These were some of the largest methane leaks ever detected by satellite at the time. Archived TROPOMI data confirmed the magnitude of these emissions sources going back at least 14 months (Varon et al., 2019). GHGSat worked with the diplomatic community to identify the industrial operator and contact the relevant authorities, and for a period of time the leaks were stopped. However, in February 2021, another GHGSat satellite detected new leaks from eight natural gas pipelines and unlit flares in the Galkynysh gas field in Turkmenistan (see Figure 8) (Malik Naureen, 2021).
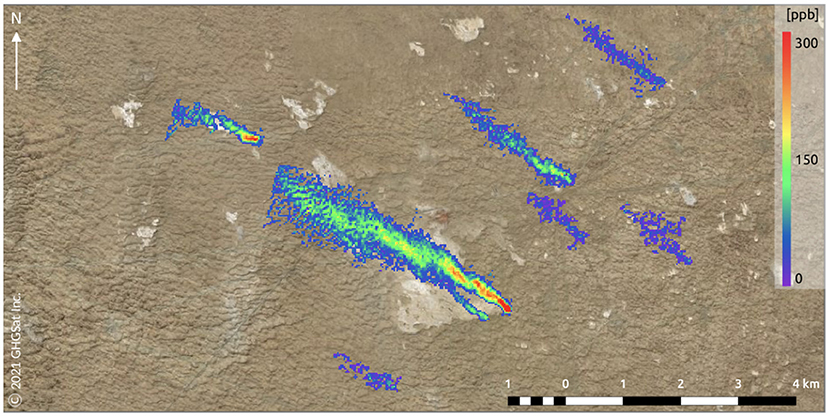
Figure 8. Eight separate methane plumes captured by GHGSat in a single image, representing a total emission rate of about 10,000 kg/hour. Four of the larger plumes on the left are emissions from pipelines (likely problems with valves), with the remaining emissions from unlit flares.
There are barriers to increasing the use of satellite data to inform policy on oil and gas emissions. For example, the EPA has had an alternative means of emission limitation (AMEL) program since 1977 (42 U. S., and Code § 7401, 1977), but the current AMEL application process is complex and requires EPA review prior to public notice and public hearing events. This complexity may limit the use of satellite data in satisfying regulatory requirements, such those targeting methane and VOC emissions from new and existing sources in the oil and gas sector.
Energy-Related CO2 Emissions
Accurate estimates of the distribution and magnitude of CO2 emissions from energy systems are important for improving predictions of climate change, designing policies to reduce emissions, and monitoring and verifying their effects. Historically, anthropogenic CO2 emissions have been inferred through bottom-up approaches using reported or estimated data on fuel consumption, emission factors, and fuel properties for thermal power plants, transportation, and industry. However, there are uncertainties in these data, even in high-income economies (Wheeler and Ummel, 2008; Gurney et al., 2019). For example, there is generally about a 20% difference between U.S. thermal power plant emissions estimated from fuel usage and those reported from a continuous emissions monitoring system (CEMS) program (Ackerman and Sundquist, 2008). Data uncertainties and gaps have prompted policymakers to look to satellite data to enhance tracking of greenhouse gas emissions and to monitor and verify reduction efforts.
The first space-based measurements of the global distribution of near-surface greenhouse gases were performed by an instrument called SCIAMACHY (European Space Agency, 2005), which operated aboard the ESA's Envisat satellite between 2002 and 2012. The first satellites dedicated to greenhouse gas measurements were GOSAT, launched by the Japan Aerospace Exploration Agency in 2009, and the Orbiting Carbon Observatory-2 (OCO-2), launched by NASA in 2014 (see example in Figure 9) (Yokota et al., 2009; Crisp, 2015). These were followed by TROPOMI aboard the ESA Sentinel-5P satellite, which has been in operation since 2017, as well as the GOSAT-2 satellite launched in 2018 and the OCO-3 instrument that was installed on the ISS in 2019. Tracking of greenhouse gas emissions with satellites is set to expand in the upcoming years: the Environmental Defense Fund (MethaneSat), the State of California (Carbon Mapper), and NASA (Geostationary Carbon Cycle Observatory, or Geocarb) are all planning launches of satellites to track emissions between 2022 and 2025 (Dennis, 2021). The growth in new dedicated satellite instrumentation, combined with existing measurements, may allow for easier independent monitoring, verification, and enforcement of the national emissions reduction commitments under Paris Agreement (Ganesan et al., 2019; Kaminski et al., 2022).
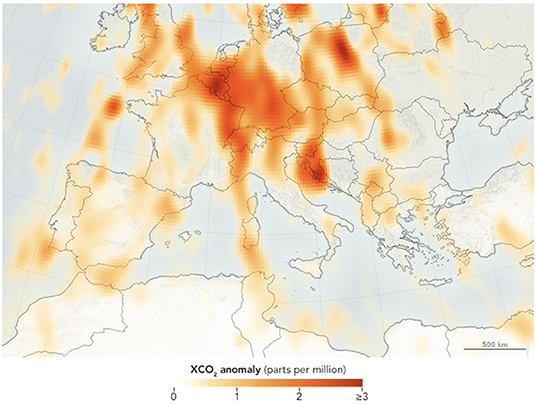
Figure 9. Tracking human contribution to atmospheric CO2 using data from NASA's OCO-2 satellite. Here, anomalies are shown from between 2014-2016. [NASA Earth Observatory maps by Joshua Stevens, using OCO-2 anomaly data courtesy of Hakkarainen et al. (2016) (NASA, 2016)].
Satellite observations of column-averaged CO2 concentrations have demonstrated that, in some circumstances, satellites can provide top-down constraints on source emissions, but the capabilities of current satellite instruments are limited (Nassar et al., 2017, 2021; Hill and Nassar, 2019; Zheng et al., 2019; Wu et al., 2020). Data from GOSAT and OCO-2 do show statistically significant CO2 enhancements over metropolitan regions (Kort et al., 2012; Schneising et al., 2013; Janardanan et al., 2016; Buchwitz et al., 2018; Wang et al., 2018; Reuter et al., 2019), and top-down methods have been applied to a few large thermal power plants (Bovensmann et al., 2010; Velazco et al., 2011), which are some of the largest point sources of anthropogenic CO2 (Janssens-Maenhout et al., 2019). A recent analysis presented the first quantification of CO2 emissions from individual power plants using OCO-2 observations (Nassar et al., 2017). However, because of the narrow swath (~10 km at nadir) and 16-day repeat cycle of the sensor, the number of clear-day overpasses is too few for the development of a global CO2 emissions inventory (Kiel et al., 2021; Nassar et al., 2021). The sparse sampling of the OCO-2 sensor will partly be overcome by the planned CO2 imaging satellites that have denser spatial coverage, such as the CO2 Monitoring mission (CO2M) and the GeoCarb instrument (Moore et al., 2018; Sierk et al., 2019).
An alternate method uses auxiliary satellite data, such as co-emitted NOx, as a proxy for CO2 emissions. Recent studies have shown that using NO2 data for plume detection improves quantification of annual CO2 emissions from point and urban sources as compared to CO2 data alone (Kuhlmann et al., 2019, 2021; Reuter et al., 2019). This method takes advantage of the higher spatial resolution and spatiotemporal coverage of satellite NO2, from which NOx emissions are inferred, and have been shown to compare well to independent observations (Beirle et al., 2011; Duncan et al., 2013, 2016; de Foy et al., 2015; Lu et al., 2015; Krotkov et al., 2016; Liu et al., 2016, 2017; Goldberg et al., 2019). This approach is particularly useful for identifying new combustion sources as they come online and changes in existing point sources and urban areas (Duncan et al., 2016; Krotkov et al., 2016). The highest resolution satellite instrument for NO2 is TROPOMI (2017-present) (Levelt et al., 2006, 2018; Veefkind et al., 2012; Munro et al., 2016; Krotkov et al., 2017). NO2 and satellite-based CO and CO2 data can also provide constraints on emissions inventories and be useful in monitoring trends and understanding regional-scale combustion (Silva and Arellano, 2017; Goldberg et al., 2019; Liu et al., 2020; Park et al., 2021).
CO2 emissions from individual power plants and large cities may also be inferred from satellite NO2. For power plants, these calculations have been performed using linear relationships between reported NOx and CO2 emissions by coal type, firing method, and emission control device (Liu et al., 2020). Ratios of NOx to CO2 emissions derived from U.S. power plants, where power plants have CEMS stack-height emissions monitors, offer a reasonable approximation for power plants in other countries, especially where coal type is known (Zoogman et al., 2017; Kim et al., 2019; Timmermans et al., 2019). City-scale emissions may be inferred through related statistical approaches to fit a collection of satellite-observed NO2 plumes and inferred CO2 emissions (Goldberg et al., 2019). While conducted and validated in the U.S., these approaches show potential for estimating CO2 emissions in other countries as well.
Moving forward, a synergistic combination of bottom-up and top-down approaches would likely provide the greatest constraint on global anthropogenic CO2 emissions. CO2M will carry instruments to observe both NO2 and CO2, which will allow for the estimation of NOx/CO2 ratios, although these ratios may have large regional and technological uncertainties (Kuhlmann et al., 2021). It has been shown that satellite NO2 and CO2 data could be used to infer a ratio to allow the estimation of CO2 emissions using TROMPOMI and OCO-2 data for an individual power plant (Hakkarainen et al., 2021). These methods ideally would be complemented by a database with region-specific NOx/CO2 ratios from CEMS measurements or other bottom-up sources.
Energy Resilience
Extreme weather events have long been a major risk factor for energy infrastructure, with climate change worsening these risks. Satellite data can provide a cost effective means for tracking vulnerable energy infrastructure, planning for new climate normals, and providing real-time support for operations and maintenance.
Energy Resilience and Global Change
Power outages, infrastructure damage, and challenges with adequately managing energy demand are well-known consequences of extreme weather and weather-related disruptions, including storms, heat waves, wildfires, and flooding (IEA, 2021a). In the U.S., for example, blackouts from extreme weather events cost an estimated $20 to $55 billion annually (Nik et al., 2021), and hurricanes are a major cause of power outages that have contributed to substantial loss of life and infrastructure (Alemazkoor et al., 2020). Extreme heat stresses the electric grid, resulting in increased demand for air conditioning and a loss in transmission and distribution efficiency (Añel et al., 2017). In February 2021, historic snowfall and ice across Texas led to blackouts that left millions of people without power (Nazir, 2021). Transmission line failure caused by extreme wind or heat can also result in wildfires, as in the 2009 Australian “Black Saturday” fires, where line failures ignited one of the most disastrous bushfires in Australian history, resulting in 173 deaths and $4 billion (Australian) in property damage (Mitchell, 2013).
Within the energy management sector, there is a strong push to design climate-resilient infrastructure that can continue operating or recover quickly after immediate shocks and adapt to long-term changes in climate and environmental conditions (IEA, 2021b). In the U.S., increased emphasis on embedding climate adaptation and resilience into federal programs could support investments in the energy sector. Efforts currently underway include the Biden Administration's Executive Order 14008 on Tackling the Climate Crisis at Home and Abroad, Build Back Better Agenda, Infrastructure Investment and Jobs Act, and Justice40 Initiative, which focus on shifting energy supply to reduce environmental and health risks and support economic development for communities impacted by energy transitions (The White House, 2021a,b). Complementing these efforts, the Department of Energy (DOE) is deploying climate-resilient energy technologies nationwide, including in underserved communities (U.S. Department of Energy, 2021).
Satellite data can be used to better understand the impacts of a changing climate on energy infrastructure, advance the development of forecast models, and reduce the effort needed to assess environmental risks, which in turn can improve site-specific resilience planning (Leibrand et al., 2019). To support the climate adaptation and resilience efforts underway in the Biden Administration, NASA and NOAA are planning to provide data and services to stakeholders to increase understanding of threats and vulnerabilities due to climate change (Margetta, 2021; US Department of Commerce, 2021). Satellite data can be used to inform planning to mitigate various energy infrastructure risks (Hauer and Miller, 2021). For example, several utility companies' wildfire mitigation plans use satellite data to monitor wildfire risks (Horizon West Transmission, 2021; Idaho Power, 2021; Pacific Gas and Electric Company, 2021; San Diego Gas and Electric Company, 2021; Southern California Edison Company, 2021), and satellite data has been used to identify vegetation encroachment and stressed or dead trees (Matikainen et al., 2016; Mahdi Elsiddig Haroun et al., 2020)4,8.
Satellite data are also already being used to support disaster response in the energy sector. For example, in 2004, Eskom, South Africa's largest energy company developed a mobile fire alert system to mitigate line faults and provide near-real-time fire information. This system relies in part on NASA MODIS data and Meteosat Second Generation (MSG) data from the European Organization for the Exploitation of Meteorological Satellites (EUMETSAT) and is still in use today (Davies et al., 2008)9. MODIS data are also an integral part of NASA's Fire Information for Resource Management System (FIRMS), which integrates data from both MODIS and VIIRS instruments to deliver global active fire and hotspot data in near real time, within three hours of satellite observation (NASA, 2021).
Following Hurricane Maria's devastating impacts on Puerto Rico in 2017, NTL data from NASA's Black Marble product were used to understand the extent of power outages and characteristics of areas that withstood the greatest impact. These data were later used to monitor the effects of electricity restoration policies (see example in Figure 10), including how these policies can exacerbate inequality and unintentionally burden vulnerable populations (Romn et al., 2019). The high spatial resolution of Black Marble enables researchers to overcome four primary limitations of power outage data: timeliness, continuous data collection, consistent data across large geographic areas, and availability of data at a very fine spatial resolution (Romn et al., 2019).
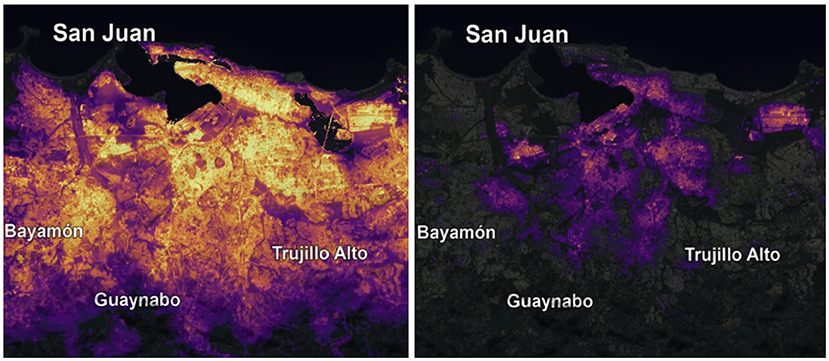
Figure 10. Baseline (pre-storm) view of San Juan, Puerto Rico, nighttime lights (L) and average nighttime lights two months (Sep. 20 - Nov. 20, 2017) after Hurricane Maria (R). (Credit: Kel Elkins, NASA Scientific Visualization Studio).
Data Distribution
Distribution of satellite data for use by decision makers and researchers is a continuing challenge, especially as the number and complexity of data products grows. While researchers and high-end data users may choose to navigate data distribution platforms, many stakeholders prefer GIS-enabled web interfaces developed for their application area. The most developed energy-specific platform for satellite data distribution is the NASA Prediction Of Worldwide Energy Resources (POWER) project. POWER has provided Earth observation data for energy applications since 2002, with the goal of improving the accessibility and use of NASA data to support community research in three focus areas: (1) renewable energy development, (2) building energy efficiency and sustainability, and (3) agroclimatology applications (Zell et al., 2008; Eckman and Stackhouse, 2012). POWER allows users to select community-specific parameters, units, time periods, and output formats to efficiently retrieve data. The output data can then be directly applied in decision support tools, modeling and forecasting packages, and as inputs to scientific research.
The solar energy parameters in POWER are compiled using multiple satellite data sources. Hourly to long-term averaged parameters are provided for each parameter and can be used to support applications such as solar cooking, sizing solar panels, and designing battery backup systems. The daily and hourly time series include the basic solar and meteorological parameters as well as additional calculated parameters such as diffuse and direct normal radiation. For example, a community solar installation in a rural village in West Africa appeared to be working poorly. POWER data revealed that the solar array was in fact performing up to specifications, but cloudiness affected the available solar energy. Local management made the necessary adjustments to power usage and billing, drawing from data only available via satellite (NASA POWER and NASA ARSET, 2021). Similarly, a consultant responsible for solar installations at various locations in North Carolina uses the low latency data products to help assess changes in output (NASA POWER and NASA ARSET, 2021).
POWER data products have also been used to support a variety of energy system operation and maintenance decisions. For example, the Ottawa Renewable Energy Cooperative used the RETScreen Expert Clean Energy Technology software suite, which directly links the POWER web suite, to assess the potential benefits of paying for snow removal for a rooftop PV system by comparing lost generation to the building's actual load (NASA POWER and NASA ARSET, 2021). NASA's Office of Strategic Infrastructure also uses RETScreen for building energy management (Rosenzweig et al., 2014). Additionally, satellite estimates suggest that increases in the solar irradiance in the maize growing regions of the U.S. from the late 1980's through about 2012 were responsible for 27% of the productivity increase observed during this time period, relevant to corn-based biofuels (Tollenaar et al., 2017). Finally, the value of packaging correlated solar, wind, and other meteorological parameters has been demonstrated for a smart energy management system for hybrid solar-wind-biomass systems (Bhattacharjee and Nandi, 2021).
Discussion
Applications of satellite data are growing across a wide range of energy policy and planning problems. Recent developments are increasing the potential for satellite data to support energy decision-making, with new public and private satellites being launched, advances in data processing techniques, and efforts by government and private organizations to increase uptake in new user communities. With more complete spatial and temporal coverage, satellite data can fill gaps in traditional data sources. Often the value of space-based data is greatest through integration with existing data and decision tools. Many types of satellite data have been collected for years, enabling analysts to track changes in energy supply, demand, and impacts over time and evaluate the effectiveness of policy interventions. While collecting satellite observations entails high fixed costs, the marginal costs are generally low, especially for datasets that are freely available to the public. Since data collection is remote, it also does not directly disturb local communities or the environment.
Our review points to many applications and opportunities for further use of space-based measurements. For energy supply, this includes resource potential and risk assessment to inform siting, development, and maintenance of energy infrastructure as well as real-time resource availability to support grid management and ensure reliability of supply. For energy demand, it includes energy use patterns to predict energy needs and identify locations with unserved demand. For energy impacts, it includes the effects of energy use on climate, air quality, and water and land systems, as well as monitoring efforts to reduce these impacts. Satellite data are also playing an increasing role in supporting investments in energy resilience, both in advance and in the aftermath of disruptions to energy access. The expansive coverage of many measurements allows for global indexing of critical metrics, and the increase in temporal resolution of new products means that satellite data can be used to track progress toward policy commitments to reduce energy-related emissions, increase energy access, and support sustainable energy transitions around the world.
The technical limitations in the use of satellite data for energy applications are primarily driven by insufficient spatial and temporal resolution. For example, polar orbiting satellites such as the Landsat 8 Operational Land Imager (OLI) and the recently launched Landsat 9 Operational Land Imager 2 (OLI-2) provide radiance measurements that are high spatial resolution (30 m) and multispectral (visible, near-infrared, and shortwave infrared bands). These measurements are suitable for land use characterization at urban and individual agricultural field scales. However, the 16-day repeat cycle, relatively narrow swath width (165 km), and likelihood of cloudy scenes limits temporal sampling to typically a single observation at one location each month, which does not allow for rapid responses to changing conditions. The European Sentinel-2 MultiSpectral Instrument (MSI) partially addresses these limitations with a 5-day repeat cycle and higher spatial resolution (10 m for the visible channels and 20 m for near-infrared and shortwave infrared). Other polar orbiting instruments, such as VIIRS, have more channels and larger swath widths (3000 km) and can observe the entire planet each day. However, VIIRS has significantly lower spatial resolution than either Landsat or Sentinel-2.
Unlike polar orbiting satellites, geostationary satellites continuously observe the same area and consequently have very high temporal sampling, and a constellation of geostationary satellites can allow for near global, continuous sampling of the Earth throughout the day. The U.S. Advanced Baseline Imager (ABI) on GOES East and West, Japanese Advanced Himawari Imager (AHI), and European Meteosat Third Generation (MTG) are all third-generation geostationary instruments with similar retrieval capabilities as VIIRS but even coarser spatial resolution. A new generation of instruments such as the recently launched Geostationary Environmental Monitoring Spectrometer (GEMS), Tropospheric Emissions: Monitoring of Pollution (TEMPO), and the European UltraViolet/Visible/Near-Infrared (UVN) instrument will provide the first geostationary hourly ultraviolet radiance measurements suitable for a wide variety of energy applications, from tracking photosynthetic activity for biofuel production to monitoring NO2 emissions from fossil fuel combustion. The GeoCarb instrument will provide similar measures of photosynthetic activity as well as geostationary CO2, CO, and CH4 retrievals over North America.
Combining high spatial resolution polar orbiting measurements with high temporal resolution measurements from geostationary satellites will create unprecedented opportunities for energy policy and planning. For example, accurate short-term cloud forecasts are critical for optimizing electric power generation and load balancing. Improved use of geostationary cloud measurements for solar PV forecasting could include data assimilation for short-term surface irradiance forecasts and advanced pattern recognition estimates of cloud motion. Using satellite data for improved monitoring and prediction of droughts (in particular, flash droughts) and resulting changes in photosynthetic activity could have significant impacts on improving biofuel production efficiency. Satellite data with high spatial and temporal resolution also can uncover patterns of energy injustice. For example, new satellite-based research can help us understand who has access to energy infrastructure and the reliability, quality, and impacts of energy services for different groups. With the ability to monitor changes over time, we can also assess equity in energy transitions.
Beyond spatial and temporal resolution, analysts must also carefully consider the appropriateness of satellite data for energy policy and planning applications. It is particularly important to understand the distribution of potential errors in satellite measurements when they are used as a proxy for energy-relevant variables, and how these errors affect causal inference (Jain, 2020). For example, NTL datasets measure nighttime luminosity but are often used to estimate energy use and access, economic activity, and other variables. This approach can lead to biased inferences on the effects of policies if NTL data undercounts energy access in areas with intermittent service or underestimates economic activity in high luminosity areas due to saturation effects. Other types of errors can also be systematic, such as when agroforestry or plantations are classified as tree cover or when small-scale logging is undetected, which may lead to an underestimate of the effects of policies on forest loss. These challenges underscore the importance of data validation and the value of combining other types of data with satellite measurements to create a more complete picture of the energy system.
While researchers are actively working to address the technical limitations of satellite data for energy applications, addressing social and structural barriers will be equally important. While social science studies specifically on the use of satellite data for decision-making are more limited, in the case of air quality – especially for policy organizations implementing the Clean Air Act in the U.S. – a range of social and structural as well as technical barriers impede data use relative to traditional monitoring and assessment methods (Milford and Knight, 2017). Satellite data do not fit with decision and policy frameworks in a clear manner, and users have expressed uncertainty about whether data will be accepted for regulatory purposes. Research also indicates a number of social barriers to satellite data use, including difficulty finding data, data formats that are unfamiliar or difficult to use, and lack of staff time, training, and expertise to acquire and process data. Two-way dialogue between end-users and satellite experts has helped identify specific areas where space-based data can contribute effectively to information needs.
Collaboration between researchers with expertise in satellite data analysis, energy systems and policy, and a broader set of social science disciplines will be essential to realizing the potential for satellite data to support energy decision-making. Research has pointed to the importance of active communication between experts and decision-makers and investing in translational work to bridge the gap between scientific data and decision processes (Cash et al., 2003; Klemun et al., 2020). Researchers themselves can engage in this boundary work – for example, NASA encourages engagement with the full satellite data application process through its Application Readiness Levels (ARLs), which range from initial discovery to full integration of satellite data into a partner's decision-making systems and processes. However, research also points to the vital role of boundary organizations and boundary objects (including data portals, interactive maps, and training workshops) in facilitating this work. Several organizations are actively working to enhance the usability of satellite data for energy applications.
Satellite applications for energy planning and policy are growing rapidly, with novel information needs to support sustainable energy transitions, a suite of new satellites recently launched or planned to be launched soon, and advances in methods for analyzing satellite data products and translating the results into useful information for energy decision-making. Our review suggests that, while there are many energy application areas where satellite data are already playing an important role, there is significant untapped potential to apply satellite data to support decision-making around energy supply, demand, impacts, and resilience. As advances in satellite data analysis open up new opportunities to support decision-making, active dialogue between experts in satellite data and energy planning and policy, as well as decision-makers across energy sectors, will be essential to maximize the usefulness of satellite data for sustainable energy transitions.
Author contributions
MRE led the conceptualization, organization, and editing of the review paper with TH and RBP. All authors drafted sections of the paper, edited the final manuscript, and approved the submitted version.
Funding
TH acknowledges support from NASA Health and Air Quality Applied Sciences Team Grant Number 80NSSC21K0427. HG and TL acknowledge support from the Great Lakes Bioenergy Research Center, U.S. Department of Energy, Office of Science, Office of Biological and Environmental Research under Award Number DE-SC0018409. KS acknowledges support from NASA Grant Number 80NSSC19K0638. Battelle expresses its gratitude for the support from Richard Eckman, Paul Stackhouse, and Brock Blevins of NASA, Craig Zamuda of U.S. DOE and DOE Partnership for Energy Sector Resilience members, Technical Advisory Group members, Chris Johnson of Battelle, Bradley Macpherson of Booz Allen Hamilton, the GEO community, and NASA Grant Number 80NSSC18K0325. This research was partially financially supported by the University of Wisconsin-Madison Office of Sustainability.
Conflict of Interest
Authors EC and ÁE were employed by GHGSat Inc. Author MB was employed by Booz Allen Hamilton, Inc. Author AL was employed by Science and Technology Corporation.
The remaining authors declare that the research was conducted in the absence of any commercial or financial relationship that could be construed as a potential conflict of interest.
Publisher's Note
All claims expressed in this article are solely those of the authors and do not necessarily represent those of their affiliated organizations, or those of the publisher, the editors and the reviewers. Any product that may be evaluated in this article, or claim that may be made by its manufacturer, is not guaranteed or endorsed by the publisher.
Abbreviations
ABI, Advanced Baseline Imager; AHI, Advanced Himawari Imager; AIMM, Alternative Approved Instrument Monitoring Method; AMEL, Alternative Means of Emission Limitation; AMSR-E and AMSR2, Advanced Microwave Scanning Radiometers; ARLs, Application Readiness Levels; ASAR, Advanced Synthetic Aperture Radar; ASTER, Advanced Spaceborne Thermal Emission and Reflection Radiometer; CEMS, Continuous Emissions Monitoring System; CERES, Clouds and Earth's Radiant Energy System; CO, Carbon monoxide; CO2, Carbon dioxide; CO2M, Copernicus Carbon Dioxide Monitoring mission; COP26, 2021 United Nations Climate Change Conference; DMSP-OLS, Defense Meteorological Satellite Program-Operational Linescan System; DNB, VIIRS Day Night Band; DOE, U.S. Department of Energy; EOSDIS, Earth Observing System Data and Information System; EPA, U.S. Environmental Protection Agency; EPRI, Electric Power Research Institute; ESA, European Space Agency; ETM+, The Enhanced Thematic Mapper Plus; EUMETSAT, European Organization for the Exploitation of Meteorological Satellites; FIRMS, NASA's Fire Information for Resource Management System; GEMS, Geostationary Environmental Monitoring Spectrometer; GeoCarb, Geostationary Carbon Cycle Observatory; GOES, Geostationary Operational Environmental Satellite; GOME-2, Global Ozone Monitoring Experiment-2; GOSAT, Greenhouse Gas Observing Satellite; IMEO, International Methane Emissions Observatory; InSAR, Interferometric Synthetic Aperture Radar; ISS, International Space Station; LANCE, Land, Atmosphere Near-real-time Capability for EOS; LDAR, Leak Detection and Repair; MERRA-2, Modern-Era Retrospective analysis for Research and Applications, Version 2; MODIS, Moderate Resolution Imaging Spectroradiometer; MSG, Meteosat Second Generation; MSI, European Sentinel-2 MultiSpectral Instrument; MTG, European Meteosat Third Generation; NASA, National Aeronautics and Space Administration; NMVOC, Methane and Non-methane Volatile Organic Compounds; NO, Nitric oxides; NO2, Nitrogen dioxide; NOAA, National Oceanic and Atmospheric Administration; NOAA ASCAT, National Oceanic and Atmospheric Administration Advanced Scatterometer; NOX, Nitrogen oxides; NREL, National Renewable Energy Laboratory; NSRDB, U.S. National Solar Radiation Data Base; NTL, Nighttime Light; OCO-2, Orbiting Carbon Observatory-2; OLI, Operational Land Imager; OMI, Ozone Monitoring Instrument; POWER, NASA Prediction Of Worldwide Energy Resources project; PV, Photovoltaic; ROW, right-of-way; RSPO, Roundtable for Sustainable Palm Oil; SAR, Synthetic Aperture Radar; SCIAMACHY, SCanning Imaging Absorption spectroMeter for Atmospheric CHartographY; SMAP, Soil Moisture Active Passive; SMMR, Scanning Multichannel Microwave Radiometer; SRON, Netherlands Institute for Space Research; SSMI, Special Sensor Microwave Imager; Suomi NPP satellite, Suomi National Polar-orbiting Partnership; TEMPO, Tropospheric Emissions: Monitoring of Pollution; TRMM, Tropical Rainfall Measuring Mission; TROPOMI, Tropospheric Monitoring Instrument; UNEP, United Nations Environment Program; UVN, UV/Visible/Near-infrared; VCD, Vertical Column Density; VIIRS, Visible Infrared Imaging Radiometer Suite; VOC, Volatile Organic Compounds; WHO, World Health Organization.
Footnotes
1. ^EPRI. Application of Image Processing Algorithms to Improve Predictive Reliability Assessments: Identifying Physical Threats Using GIS and Satellite Imagery. https://www.epri.com/research/products/000000003002018884.
2. ^EPRI. Program on Technology Innovation: Using Hyperspectral Imagery and Artificial Intelligence (AI) to Detect Stressed and Dead Trees. https://www.epri.com/research/products/000000003002022770.
3. ^United Nations. Net Zero Coalition. https://www.un.org/en/climatechange/net-zero-coalition.
4. ^World Bank - Light Every Night. Registry of Open Data on AWS https://registry.opendata.aws/wb-light-every-night/.
5. ^EPRI Program on Technology Innovation: New Frontiers in Milkweed Detection — Evaluating the Potential of Satellite Data and Machine Learning. https://www.epri.com/research/products/000000003002016599.
6. ^World Health Organization. Air pollution. https://www.who.int/westernpacific/health-topics/air-pollution.
7. ^Group on Earth Observations, Climate TRACE and World Geospatial Industry Council. Greenhouse Gas Monitoring from Space: A Mapping of Capabilities Across Public, Private, and Hybrid Satellite Missions. GEO Observations Blog http://www.earthobservations.org/geo_blog_obs.php?id=533.
8. ^EPRI Identification of At-Risk Trees Using Satellite Imagery. https://www.epri.com/research/products/000000003002019050.
9. ^Advanced Fire Information System (AFIS). AFIS. http://www.afis.co.za/.
References
42 U. S Code § 7401. (1977). https://www.govinfo.gov/content/pkg/STATUTE-91/pdf/STATUTE-91-Pg685.pdf.
Ackerman, K. V., and Sundquist, E. T. (2008). Comparison of two U.S. power-plant carbon dioxide emissions data sets. Environ. Sci. Technol. 42, 5688–5693. doi: 10.1021/es800221q
Ahsbahs, T., Badger, M., Volker, P., Hansen, K. S., and Hasager, C. B. (2018). Applications of satellite winds for the offshore wind farm site Anholt. Wind Energy Sci. 3, 573–588. doi: 10.5194/wes-3-573-2018
Aklin, M., Harish, S. P., and Urpelainen, J. (2018). A global analysis of progress in household electrification. Energy Policy 122, 421–428. doi: 10.1016/j.enpol.2018.07.018
Alemazkoor, N., Rachunok, B., Chavas, D. R., Staid, A., Louhghalam, A., Nateghi, R., et al. (2020). Hurricane-induced power outage risk under climate change is primarily driven by the uncertainty in projections of future hurricane frequency. Sci. Rep. 10, 15270. doi: 10.1038/s41598-020-72207-z
Alkan, M. (2009). Detection of Coal Ash Turbidity in Marine Environment Using Remote Sensing. Fresenius Environ. Bull. 18, 2072–2078.
Añel, J. A., Fernández-González, M., Labandeira, X., López-Otero, X., and De la Torre, L. (2017). Impact of cold waves and heat waves on the energy production sector. Atmosphere 8, 209. doi: 10.3390/atmos8110209
Anenberg, S. C., Mohegh, A., Goldberg, D. L., Kerr, G. H., Brauer, M., Burkart, K., et al. (2022). Long-term trends in urban NO2 concentrations and associated paediatric asthma incidence: estimates from global datasets. Lancet Planet. Health 6, e49–e58. doi: 10.1016/S2542-5196(21)00255-2
Arderne, C., Zorn, C., Nicolas, C., and Koks, E. E. (2020). Predictive mapping of the global power system using open data. Sci. Data 7, 19. doi: 10.1038/s41597-019-0347-4
Avtar, R., Tripathi, S., and Aggarwal, A. K. (2019). Assessment of energy–population–urbanization nexus with changing energy industry scenario in India. Land 8, 124. doi: 10.3390/land8080124
Badger, M., Peña, A., Hahmann, A. N., Mouche, A. A., and Hasager, C. B. (2016). Extrapolating satellite winds to turbine operating heights. J. Appl. Meteorol. Climatol. 55, 975–991. doi: 10.1175/JAMC-D-15-0197.1
Bastiaanssen, W. G. M., Simons, G., and Sheridan, P. F. (2012). The Potential of Satellite-based Information for Monitoring Water and Production Related Aspects of the Sustainability of Biofuels.
Baxter, R. E., and Calvert, K. E. (2017). Estimating available abandoned cropland in the United States: possibilities for energy crop production. Ann. Am. Assoc. Geogr. 107, 1162–1178. doi: 10.1080/24694452.2017.1298985
Bayramov, E. (2013). “Optimization of environmental monitoring principles along oil and gas pipelines using GIS and remote sensing,” in 2013 7th International Conference on Application of Information and Communication Technologies p. 1–3. doi: 10.1109/ICAICT.2013.6722681
Beirle, S., Boersma, K. F., Platt, U., Lawrence, M. G., and Wagner, T. (2011). Megacity emissions and lifetimes of nitrogen oxides probed from space. Science 333, 1737–1739. doi: 10.1126/science.1207824
Berndes, G., Ahlgren, S., Börjesson, P., and Cowie, A. L. (2013). Bioenergy and land use change—state of the art. WIREs Energy Environ. 2, 282–303. doi: 10.1002/wene.41
Bhattacharjee, S., and Nandi, C. (2021). Design of a voting based smart energy management system of the renewable energy based hybrid energy system for a small community. Energy 214, 118977. doi: 10.1016/j.energy.2020.118977
Bhattarai, N., Wagle, P., Gowda, P. H., and Kakani, V. G. (2017). Utility of remote sensing-based surface energy balance models to track water stress in rain-fed switchgrass under dry and wet conditions. ISPRS J. Photogramm. Remote Sens. 133, 128–141. doi: 10.1016/j.isprsjprs.2017.10.010
Blackman, A. (2013). Evaluating forest conservation policies in developing countries using remote sensing data: an introduction and practical guide. For. Policy Econ. 34, 1–16. doi: 10.1016/j.forpol.2013.04.006
Bonsucro (2021). Bonsucro Draft Production Standard Version 5, 7. Available online at: https://www.bonsucro.com/wp-content/uploads/2021/06/Bonsucro-Production-Standard-draft-v.5.7-1.pdf (accessed July 11, 2022).
Bovensmann, H., Buchwitz, M., Burrows, J. P., Reuter, M., Krings, T., Gerilowski, K., et al. (2010). Remote sensing technique for global monitoring of power plant CO2 emissions from space and related applications. Atmospheric Meas. Tech. 3, 781–811. doi: 10.5194/amt-3-781-2010
Brandt, A. R., Heath, G. A., and Cooley, D. (2016). Methane leaks from natural gas systems follow extreme distributions. Environ. Sci. Technol. 50, 12512–12520. doi: 10.1021/acs.est.6b04303
Brown, J. C., Hanley, E., Bergtold, J., Caldas, M., Barve, V., Peterson, D., et al. (2014). Ethanol plant location and intensification vs. extensification of corn cropping in Kansas. Appl. Geogr. 53, 141–148. doi: 10.1016/j.apgeog.2014.05.021
Buchwitz, M., Reuter, M., Schneising, O., Nol, S., Gier, B., Bovensmann, H., et al. (2018). Computation and analysis of atmospheric carbon dioxide annual mean growth rates from satellite observations during 2003–2016. Atmospheric Chem. Phys. 18, 17355–17370. doi: 10.5194/acp-18-17355-2018
Burlig, F., and Preonas, L. (2016). Out of the Darkness and Into the Light? Development Effects of Rural Electrification. EPIC https://epic.uchicago.edu/research/out-of-the-darkness-and-into-the-light-development-effects-of-rural-electrification/ (accessed July 11, 2022).
Cai, X., Zhang, X., and Wang, D. (2011). Land availability for biofuel production. Environ. Sci. Technol. 45, 334–339. doi: 10.1021/es103338e
Carley, S., and Konisky, D. M. (2020). The justice and equity implications of the clean energy transition. Nat. Energy 5, 569–577. doi: 10.1038/s41560-020-0641-6
Carlson, K. M., Curran, L. M., Ratnasari, D., Pittman, A. M., Soares-Filho, B. S., Asner, G. P., et al. (2012). Committed carbon emissions, deforestation, and community land conversion from oil palm plantation expansion in West Kalimantan, Indonesia. Proc. Natl. Acad. Sci. 109, 7559–7564. doi: 10.1073/pnas.1200452109
Cash, D., Clark, W., Alcock, F., Dickson, N., Selin, N., Guston, D., et al. (2003). Knowledge systems for sustainable development. Proc. Natl. Acad. Sci. U. S. A. 100, 8086–8091. doi: 10.1073/pnas.1231332100
Chen, Y., Ale, S., Rajan, N., and Munster, C. (2017). Assessing the hydrologic and water quality impacts of biofuel-induced changes in land use and management. GCB Bioenergy 9, 1461–1475. doi: 10.1111/gcbb.12434
Cheng, L., Zang, H., Wei, Z., Ding, T., Xu, R., Sun, G., et al. (2022). Short-term Solar Power Prediction Learning Directly from Satellite Images With Regions of Interest. IEEE Trans. Sustain. Energy 13, 629–639. doi: 10.1109/TSTE.2021.3123476
Creutzig, F., Agoston, P., Minx, J. C., Canadell, J. G., Andrew, R. M., Qur, C. L., et al. (2016). Urban infrastructure choices structure climate solutions. Nat. Clim. Change 6, 1054–1056. doi: 10.1038/nclimate3169
Creutzig, F., Erb, K. H., Haberl, H., Hof, C., Hunsberger, C., et al. (2021). Considering sustainability thresholds for BECCS in IPCC and biodiversity assessments. GCB Bioenergy 13, 510–515. doi: 10.1111/gcbb.12798
Crisp, D. (2015). “Measuring atmospheric carbon dioxide from space with the Orbiting Carbon Observatory-2 (OCO-2),” in Earth Observing Systems XX vol. 9607 p. 960702 (SPIE). doi: 10.1117/12.2187291
Cui, R. Y., Hultman, N., Edwards, M. R., He, L., Sen, A., Surana, K., et al. (2019). Quantifying operational lifetimes for coal power plants under the Paris goals. Nat. Commun. 10, 4759. doi: 10.1038/s41467-019-12618-3
Davies, D., Vosloo, H., Vannan, S., and Frost, P. (2008). “Near real-time fire alert system in South Africa: From desktop to mobile service,” in Proceedings of the 7th ACM Conference on Designing Interactive Systems (New York, NY), 315–322. doi: 10.1145/1394445.1394479
Davis, G. (2007). History of the NOAA satellite program. J. Appl. Remote Sens. 1, 012504. doi: 10.1117/1.2642347
de Foy, B., Lu, Z., Streets, D. G., Lamsal, L. N., and Duncan, B. N. (2015). Estimates of power plant NOx emissions and lifetimes from OMI NO2 satellite retrievals. Atmos. Environ. 116, 1–11. doi: 10.1016/j.atmosenv.2015.05.056
de Miguel, A. S., Castao, J. G., Zamorano, J., Pascual, S., Ngeles, M., Cayuela, L., et al. (2014). Atlas of astronaut photos of Earth at night. Astron. Geophys. 55, 436 doi: 10.1093/astrogeo/atu165
Dennis, B. (2021). How Satellites Could Help Hold Countries to Emissions Promises Made at COP26 Summit. Washington Post. Available online at: https://www.washingtonpost.com/climate-environment/2021/11/09/cop26-satellites-emissions
Doll, C. N. H., and Pachauri, S. (2010). Estimating rural populations without access to electricity in developing countries through night-time light satellite imagery. Energy Policy 38, 5661–5670. doi: 10.1016/j.enpol.2010.05.014
Donaldson, D., and Storeygard, A. (2016). The View from Above: Applications of Satellite Data in Economics. J. Econ. Perspect. 30, 171–198. doi: 10.1257/jep.30.4.171
Donner, S. D., and Kucharik, C. J. (2008). Corn-based ethanol production compromises goal of reducing nitrogen export by the Mississippi River. Proc. Natl. Acad. Sci. 105, 4513–4518. doi: 10.1073/pnas.0708300105
Dugoua, E., Kennedy, R., and Urpelainen, J. (2017). Satellite Data for the Social Sciences: Measuring Rural Electrification with Nighttime Lights. Available online at: https://papers.ssrn.com/abstract=3083505 (accessed July 11, 2022).
Duncan, B. N., Lamsal, L. N., Thompson, A. M., Yoshida, Y., Lu, Z., Streets, D. G., et al. (2016). A space-based, high-resolution view of notable changes in urban NOx pollution around the world (2005–2014). J. Geophys. Res. Atmospheres 121, 976–996. doi: 10.1002/2015JD024121
Duncan, B. N., Yoshida, Y., de Foy, B., Lamsal, L. N., Streets, D. G., Lu, Z., et al. (2013). The observed response of Ozone Monitoring Instrument (OMI) NO2 columns to NOx emission controls on power plants in the United States: 2005–2011. Atmos. Environ. 81, 102–111. doi: 10.1016/j.atmosenv.2013.08.068
Duncan, B. N., Yoshida, Y., Olson, J. R., Sillman, S., Martin, R. V., Lamsal, L., et al. (2010). Application of OMI observations to a space-based indicator of NOx and VOC controls on surface ozone formation. Atmos. Environ. 44, 2213–2223. doi: 10.1016/j.atmosenv.2010.03.010
Duren, R. M., Thorpe, A. K., Foster, K. T., Rafiq, T., Hopkins, F. M., Yadav, V., et al. (2019). California's methane super-emitters. Nature 575, 180–184. doi: 10.1038/s41586-019-1720-3
Dybas, C. L. (2005). Ocean dead zones spreading in world oceans. BioScience 55. doi: 10.1641/0006-3568(2005)055[0552:DZSIWO]2.0.CO;2
Eckman, R. S., and Stackhouse, P. W. (2012). CEOS contributions to informing energy management and policy decision making using space-based Earth observations. Appl. Energy 90, 206–210. doi: 10.1016/j.apenergy.2011.03.001
Edwards, M. R., Giang, A., Macey, G. P., Magavi, Z., Nicholas, D., Ackley, R., et al. (2021). Repair failures call for new policies to tackle leaky natural gas distribution systems. Environ. Sci. Technol. 55, 6561–6570. doi: 10.1021/acs.est.0c07531
Elvidge, C. D., Zhizhin, M., Ghosh, T., Hsu, F. C., and Taneja, J. (2021). Annual time series of global VIIRS nighttime lights derived from monthly averages: 2012 to 2019. Remote Sens. 13, 922. doi: 10.3390/rs13050922
Esparza, Á., and Mattson, R. (2021). Monitoring Leaks at Oil and Gas Facilities Using Satellites in Support of ESG Initiatives. ROGTEC. Available online at: https://www.rogtecmagazine.com/monitoring-leaks-at-oil-and-gas-facilities-using-satellites-in-support-of-esg-initiatives/ (accessed July 11, 2022).
Esparza, Á. E., and Gauthier, J. F. (2021). Monitoring Leaks at Oil and Gas Facilities Using the Same Sensor on Aircraft and Satellite Platforms. OnePetro. doi: 10.15530/urtec-2021-5666
European Commission (2021). Launch by US, EU and Partners of the Global Methane Pledge. European Commission Available online at: https://ec.europa.eu/commission/presscorner/detail/en/statement_21_5766 (accessed July 11, 2022).
European Space Agency (2005). Envisat enables first global check of regional methane emissions. Available online at: https://www.esa.int/Applications/Observing_the_Earth/Envisat/Envisat_enables_first_global_check_of_regional_methane_emissions (accessed July 11, 2022).
European Space Agency (2020). Detecting methane emissions during COVID-19. Available online at: https://www.esa.int/Applications/Observing_the_Earth/Copernicus/Sentinel-5P/Detecting_methane_emissions_during_COVID-19 (accessed July 11, 2022).
Falchetta, G., Kasamba, C., and Parkinson, S. C. (2020b). Monitoring hydropower reliability in Malawi with satellite data and machine learning. Environ. Res. Lett. 15, 014011. doi: 10.1088/1748-9326/ab6562
Falchetta, G., and Noussan, M. (2019). Interannual variation in night-time light radiance predicts changes in national electricity consumption conditional on income-level and region. Energies 12, 456. doi: 10.3390/en12030456
Falchetta, G., Pachauri, S., Byers, E., Danylo, O., and Parkinson, S. C. (2020a). Satellite Observations Reveal Inequalities in the Progress and Effectiveness of Recent Electrification in Sub-Saharan Africa. One Earth 2, 364–379. doi: 10.1016/j.oneear.2020.03.007
Falchetta, G., Pachauri, S., Parkinson, S., and Byers, E. (2019). A high-resolution gridded dataset to assess electrification in sub-Saharan Africa. Sci. Data 6, 110. doi: 10.1038/s41597-019-0122-6
Fletcher, S., Lickley, M., and Strzepek, K. (2019). Learning about climate change uncertainty enables flexible water infrastructure planning. Nat. Commun. 10, 1782. doi: 10.1038/s41467-019-09677-x
Fobi, S., Deshpande, V., Ondiek, S., Modi, V., and Taneja, J. A. (2018). Longitudinal study of electricity consumption growth in Kenya. Energy Policy 123, 569–578. doi: 10.1016/j.enpol.2018.08.065
Fragkias, M., Lobo, J., and Seto, K. C. (2017). A comparison of nighttime lights data for urban energy research: Insights from scaling analysis in the US system of cities. Environ. Plan. B Urban Anal. City Sci. 44, 1077–1096. doi: 10.1177/0265813516658477
Frolking, S., Milliman, T., Mahtta, R., Paget, A., Long, D. G., Seto, K. C., et al. (2022). A global urban microwave backscatter time series data set for 1993–2020 using ERS, QuikSCAT, and ASCAT data. Sci. Data 9, 88. doi: 10.1038/s41597-022-01193-w
Frolking, S., Milliman, T., Seto, K. C., and Friedl, M. A. (2013). A global fingerprint of macro-scale changes in urban structure from 1999 to 2009. Environ. Res. Lett. 8, 024004. doi: 10.1088/1748-9326/8/2/024004
Ganesan, A. L., Schwietzke, S., Poulter, B., Arnold, T., Lan, X., Rigby, M., et al. (2019). Advancing scientific understanding of the global methane budget in support of the Paris Agreement. Glob. Biogeochem. Cycles 33, 1475–1512. doi: 10.1029/2018GB006065
Gelfand, I., Sahajpal, R., Zhang, X., Izaurralde, R. C., Gross, K. L., Robertson, G. P., et al. (2013). Sustainable bioenergy production from marginal lands in the US Midwest. Nature 493, 514–517. doi: 10.1038/nature11811
Goga, T., Feranec, J., Bucha, T., Rusnk, M., Sakov, I., Barka, I., et al. (2019). A review of the application of remote sensing data for abandoned agricultural land identification with focus on Central and Eastern Europe. Remote Sens. 11, 2759. doi: 10.3390/rs11232759
Goldberg, D. L., Anenberg, S. C., Kerr, G. H., Mohegh, A., Lu, Z., and Streets, D. G. (2021). TROPOMI NO2 in the United States: A Detailed Look at the Annual Averages, Weekly Cycles, Effects of Temperature, and Correlation With Surface NO2 Concentrations. Earths Future 9, e2020EF001665. doi: 10.1029/2020EF001665
Goldberg, D. L., Lu, Z., Oda, T., Lamsal, L. N., Liu, F., Griffin, D., et al. (2019). Exploiting OMI NO2 satellite observations to infer fossil-fuel CO2 emissions from U.S. megacities. Sci. Total Environ. 695, 133805. doi: 10.1016/j.scitotenv.2019.133805
Gorelick, N., Hancher, M., Dixon, M., Ilyushchenko, S., Thau, D., Moore, R., et al. (2017). Google Earth Engine: Planetary-scale geospatial analysis for everyone. Remote Sens. Environ. 202, 18–27. doi: 10.1016/j.rse.2017.06.031
Gu, Y., and Wylie, B. (2016). Mapping marginal croplands suitable for cellulosic feedstock crops in the Great Plains, United States. GCB Bioenergy 9, 836–844. doi: 10.1111./gcbb.12388
Gurney, K. R., Liang, J., O'Keeffe, D., Patarasuk, R., Hutchins, M., Huang, J., et al. (2019). Comparison of Global Downscaled Versus Bottom-Up Fossil Fuel CO2 Emissions at the Urban Scale in Four U.S. Urban Areas. J. Geophys. Res. Atmospheres 124, 2823–2840. doi: 10.1029/2018JD028859
Haag, A. S., Walker, N. D., Pilley, C. T., Comeaux, J. C., Calvasina, J. J., Firth, R., et al. (2009). “Satellite surveillance of the Gulf and beyond: An overview of the LSU Earth Scan Laboratory's ocean observing capabilities,” in OCEANS 2009. p. 1–8. doi: 10.23919/OCEANS.2009.5422173
Haberl, H., Erb, K-. H., Krausmann, F., Running, S., Searchinger, T. D., et al. (2013). Bioenergy: how much can we expect for 2050? Environ. Res. Lett. 8, 031004. doi: 10.1088/1748-9326/8/3/031004
Hakkarainen, J., Ialongo, I., and Tamminen, J. (2016). Direct space-based observations of anthropogenic CO2 emission areas from OCO-2. Geophys. Res. Lett. 43, 11400–6 doi: 10.1002/2016GL070885
Hakkarainen, J., Szelg, M. E., Ialongo, I., Retscher, C., Oda, T., Crisp, D., et al. (2021). Analyzing nitrogen oxides to carbon dioxide emission ratios from space: A case study of Matimba Power Station in South Africa. Atmospheric Environ. X 10, 100110. doi: 10.1016/j.aeaoa.2021.100110
Hamada, Y., Ssegane, H., and Negri, M. C. (2015). Mapping intra-field yield variation using high resolution satellite imagery to integrate bioenergy and environmental stewardship in an agricultural watershed. Remote Sens. 7, 9753–9768. doi: 10.3390/rs70809753
Hauer, R., and Miller, R. (2021). Utility Vegetation Management in North America: Results from a 2019 Utility Forestry Census of Tree Activities and Operations.
He, S., Gu, L., Tian, J., Deng, L., Yin, J., Liao, Z., et al. (2021). Machine learning improvement of streamflow simulation by utilizing remote sensing data and potential application in guiding reservoir operation. Sustainability 13, 3645. doi: 10.3390/su13073645
Hendricks, N. P., Sinnathamby, S., Douglas-Mankin, K., Smith, A., Sumner, D. A., Earnhart, D. H., et al. (2014). The environmental effects of crop price increases: Nitrogen losses in the U.S. Corn Belt. J. Environ. Econ. Manag. 68, 507–26. doi: 10.1016/j.jeem.2014.09.002
Hernández, D. (2015). Sacrifice Along the Energy Continuum: A Call for Energy Justice. Environ. Justice 8, 151–156. doi: 10.1089/env.2015.0015
Hewson, R., Mshiu, E., Hecker, C., van der Werff, H., van Ruitenbeek, F., Alkema, D., et al. (2020). The application of day and night time ASTER satellite imagery for geothermal and mineral mapping in East Africa. Int. J. Appl. Earth Obs. Geoinformation 85, 101991. doi: 10.1016/j.jag.2019.101991
Hill, T., and Nassar, R. (2019). Pixel size and revisit rate requirements for monitoring power plant CO2 emissions from space. Remote Sens. 11, 1608. doi: 10.3390/rs11131608
Holloway, T., Jacob, D. J., and Miller, D. (2018). Short history of NASA applied science teams for air quality and health. J. Appl. Remote Sens. 12, 1. doi: 10.1117/1.JRS.12.042611
Holloway, T., Miller, D., Anenberg, S., Diao, M., Duncan, B., Fiore, A. M., et al. (2021). Satellite monitoring for air quality and health. Annu. Rev. Biomed. Data Sci. 4, 417–447. doi: 10.1146/annurev-biodatasci-110920-093120
Horizon West Transmission, L. (2021). Wildfire Mitigation Plan 2021. Available online at: https://energysafety.ca.gov/wp-content/uploads/docs/wmp-2021/hwt_2021_wmp.pdf
Howari, F. (2015). Prospecting for geothermal energy through satellite based thermal data: Review and the way forward. J Environ Sci Manage. 1, 265–74. doi: 10.7508/GJESM.2015.04.001
Hultman, N. E., Clarke, L., Frisch, C., Kennedy, K., McJeon, H., Cyrs, T., et al. (2020). Fusing subnational with national climate action is central to decarbonization: the case of the United States. Nat. Commun. 11, 5255. doi: 10.1038/s41467-020-18903-w
Idaho Power (2021). Wildfire Mitigation Plan 2022. Available online at: https://docs.idahopower.com/pdfs/Safety/2022Wildfire%20MitigationPlan.pdf (accessed July 11, 2022).
IEA (2019). Africa Energy Outlook – Analysis. IEA. Available online at: https://www.iea.org/reports/africa-energy-outlook-2019 (accessed July 11, 2022).
IEA (2021a). Global Energy Review – Analysis. IEA. Available online at: https://www.iea.org/reports/global-energy-review-2021 (accessed July 11, 2022).
IEA (2021b). Climate Resilience Policy Indicator. Available online at: https://www.iea.org/reports/climate-resilience-policy-indicator/climate-resilience-policy-indicator (accessed July 11, 2022).
IEA (2021c). Access to Electricity. Available online at: https://www.iea.org/reports/sdg7-data-and-projections/access-to-electricity (accessed July 11, 2022).
Inman, R. H., Pedro, H. T. C., and Coimbra, C. F. M. (2013). Solar forecasting methods for renewable energy integration. Prog. Energy Combust. Sci. 39, 535–576. doi: 10.1016/j.pecs.2013.06.002
International Hydropower Association (2020). Hydropower Status Report. Available online at: https://www.hydropower.org/publications/2020-hydropower-status-report (accessed July 11, 2022).
International Hydropower Association (2021). Hydropower Status Report. Available online at: https://www.hydropower.org/publications/2021-hydropower-status-report (accessed July 11, 2022).
IPCC (2018). Global Warming of 1.5°C. An IPCC Special Report on the impacts of global warming of 1.5°C above pre-industrial levels and related global greenhouse gas emission pathways. Special Report Global Warming of 1.5 °C Available online at: https://www.ipcc.ch/sr15/
IPCC (2022). “Climate Change 2022: Mitigation of Climate Change,” in Contribution of Working Group III to the Sixth Assessment Report of the Intergovernmental Panel on Climate Change, eds P. R. Shukla, J. Skea, R. Slade, A. vAl Khourdajie, R. van Diemen, D. McCollum, M. Pathak, S. Some, P. Vyas, R. Fradera, M. Belkacemi, A. Hasija, G. Lisboa, S. Luz, J. Malley (Cambridge, UK; New York, NY: Cambridge University Press), doi: 10.1017/9781009157926
Ishii, T., Simo-Serra, E., Iizuka, S., Mochizuki, Y., Sugimoto, A., Ishikawa, H., et al. (2016). “Detection by classification of buildings in multispectral satellite imagery,” in 2016 23rd International Conference on Pattern Recognition (ICPR) p. 3344–3349 (IEEE). doi: 10.1109/ICPR.2016.7900150
Jacob, D. J., Holloway, T., and Haynes, J. D. (2014). The NASA air quality applied sciences team (AQAST). Environ. Manag. 2. Available online at: https://pubs.awma.org/flip/EM-Feb-2014/coverstory.pdf
Jain, M. (2020). The Benefits and Pitfalls of Using Satellite Data for Causal Inference. Rev. Environ. Econ. Policy 14, 157–169. doi: 10.1093/reep/rez023
Janardanan, R., Maksyutov, S., Oda, T., Saito, M., Kaiser, J. W., Ganshin, A., et al. (2016). Comparing GOSAT observations of localized CO2 enhancements by large emitters with inventory-based estimates. Geophys. Res. Lett. 43, 3486–3493. doi: 10.1002/2016GL067843
Janjai, S., Pankaew, P., Laksanaboonsong, J., and Kitichantaropas, P. (2011). Estimation of solar radiation over Cambodia from long-term satellite data. Renew. Energy 36, 1214–1220. doi: 10.1016/j.renene.2010.09.023
Janssens-Maenhout, G., Crippa, M., Guizzardi, D., Muntean, M., Schaaf, E., Dentener, F., et al. (2019). EDGAR v4.3.2 Global Atlas of the three major greenhouse gas emissions for the period 1970–2012. Earth Syst. Sci. Data 11, 959–1002. doi: 10.5194/essd-11-959-2019
Jiang, C., Guan, K., Khanna, M., Chen, L., and Peng, J. (2021). Assessing marginal land availability based on land use change information in the contiguous United States. Environ. Sci. Technol. 55, 10794–10804. doi: 10.1021/acs.est.1c02236
Kaminski, T., Scholze, M., Rayner, P., Vobeck, M., Buchwitz, M., Reuter, M., et al. (2022). Assimilation of atmospheric CO2 observations from space can support national CO2 emission inventories. Environ. Res. Lett. 17, 014015. doi: 10.1088/1748-9326/ac3cea
Kang, S., Post, W., Wang, D., Nichols, J., Bandaru, V., West, T., et al. (2013). Hierarchical marginal land assessment for land use planning. Land Use Policy 30, 106–113. doi: 10.1016/j.landusepol.2012.03.002
Karlsson, K-. G., Anttila, K., Trentmann, J., Stengel, M., Fokke Meirink, J., et al. (2017). CLARA-A2: the second edition of the CM SAF cloud and radiation data record from 34 years of global AVHRR data. Atmospheric Chem. Phys. 17, 5809–5828. doi: 10.5194/acp-17-5809-2017
Kerr, G. H., Goldberg, D. L., and Anenberg, S. C. (2021). COVID-19 pandemic reveals persistent disparities in nitrogen dioxide pollution. Proc. Natl. Acad. Sci. 118. doi: 10.1073/pnas.2022409118
Khaki, M., Hendricks Franssen, H.-. J, and Han, S. C. (2020). Multi-mission satellite remote sensing data for improving land hydrological models via data assimilation. Sci. Rep. 10, 18791. doi: 10.1038/s41598-020-75710-5
Khanna, M., Chen, L., Basso, B., Cai, X., Field, J. L., Guan, K., et al. (2021). Redefining marginal land for bioenergy crop production. GCB Bioenergy 13, 1590–1609. doi: 10.1111/gcbb.12877
Kiel, M., Eldering, A., Roten, D. D., Lin, J. C., Feng, S., Lei, R., et al. (2021). Urban-focused satellite CO2 observations from the Orbiting Carbon Observatory-3: A first look at the Los Angeles megacity. Remote Sens. Environ. 258, 112314. doi: 10.1016/j.rse.2021.112314
Kim, J., Jeong, U., Ahn, M. H., Kim, J., Park, R., et al. (2019). New Era of Air Quality Monitoring from Space: Geostationary Environment Monitoring Spectrometer (GEMS). Bull. Am. Meteorol. Soc. 101, 00.
Kimball, J., and McDonald, K. (2020). An Earth System Data Record for Land Surface Freeze-Thaw State: Quantifying Terrestrial Water Mobility Constraints to Global Ecosystem Processes. NASA. Available online at: https://www.earthdata.nasa.gov/esds/competitive-programs/measures/esdr-for-land-surface-freeze-thaw-state
Klemas, V. (2012). Remote sensing of algal blooms: an overview with case studies. J. Coast. Res. 28, 34–43. doi: 10.2112/JCOASTRES-D-11-00051.1
Klemun, M. M., Edwards, M. R., and Trancik, J. E. (2020). Research priorities for supporting subnational climate policies. WIREs Clim. Change 11, e646. doi: 10.1002/wcc.646
Koh, L. P., Miettinen, J., Liew, S. C., and Ghazoul, J. (2011). Remotely sensed evidence of tropical peatland conversion to oil palm. Proc. Natl. Acad. Sci. 108, 5127–5132. doi: 10.1073/pnas.1018776108
Korkovelos, A., Khavari, B., Sahlberg, A., Howells, M., and Arderne, C. (2019). The role of open access data in geospatial electrification planning and the achievement of SDG7. An OnSSET-based case study for Malawi. Energies 12, 1395. doi: 10.3390/en12071395
Kort, E. A., Frankenberg, C., Miller, C. E., and Oda, T. (2012). Space-based observations of megacity carbon dioxide. Geophys. Res. Lett. 39. doi: 10.1029/2012GL052738
Krotkov, N. A., Lamsal, L. N., Celarier, E. A., Swartz, W. H., Marchenko, S. V., Bucsela, E. J., et al. (2017). The version 3 OMI NO2 standard product. Atmospheric Meas. Tech. 10, 3133–3149. doi: 10.5194/amt-10-3133-2017
Krotkov, N. A., McLinden, C. A., Li, C., Lamsal, L. N., Celarier, E. A., Marchenko, S. V., et al. (2016). Aura OMI observations of regional SO2 and NO2 pollution changes from 2005 to 2015. Atmospheric Chem. Phys. 16, 4605–4629. doi: 10.5194/acp-16-4605-2016
Kruitwagen, L., Story, K. T., Friedrich, J., Byers, L., Skillman, S., Hepburn, C. A., et al. (2021). Global inventory of photovoltaic solar energy generating units. Nature 598, 604–610. doi: 10.1038/s41586-021-03957-7
Kuhlmann, G., Broquet, G., Marshall, J., Clment, V., Lscher, A., Meijer, Y., et al. (2019). Detectability of CO2 emission plumes of cities and power plants with the Copernicus Anthropogenic CO2 Monitoring (CO2M) mission. Atmospheric Meas. Tech. 12, 6695–6719. doi: 10.5194/amt-12-6695-2019
Kuhlmann, G., Henne, S., Meijer, Y., and Brunner, D. (2021). Quantifying CO2 Emissions of Power Plants With CO2 and NO2 Imaging Satellites. Front. Remote Sens. 2, 14. doi: 10.3389/frsen.2021.689838
Kumar, A., Ramsankaran, R., Brocca, L., and Muñoz-Arriola, F. A. (2021). Simple machine learning approach to model real-time streamflow using satellite inputs: Demonstration in a data scarce catchment. J. Hydrol. 595, 126046. doi: 10.1016/j.jhydrol.2021.126046
Lambert, A., Hallar, A. G., Garcia, M., Strong, C., Andrews, E., Hand, J. L., et al. (2020). Dust Impacts of Rapid Agricultural Expansion on the Great Plains. Geophys. Res. Lett. 47, e2020GL. doi: 10.1029/2020GL090347
Lark, T. J., Hendricks, N. P., Smith, A., Pates, N., Spawn-Lee, S. A., Bougie, M., et al. (2022). Environmental outcomes of the US Renewable Fuel Standard. Proc. Natl. Acad. Sci. 119, e2101084119. doi: 10.1073/pnas.2101084119
Lauvaux, T., Giron, C., Mazzolini, M., D'Aspremont, A., Duren, R., Cusworth, D., et al. (2022). Global assessment of oil and gas methane ultra-emitters. Science. 375:557–61. doi: 10.1126/science.abj4351
Leibrand, A., Sadoff, N., Maslak, T., and Thomas, A. (2019). Using Earth observations to help developing countries improve access to reliable, sustainable, and modern energy. Front. Environ. Sci. 7, 123. doi: 10.3389/fenvs.2019.00123
Leidner, A., and Buchanan, G. (2018). Satellite Remote Sensing for Conservation Action: Case Studies from Aquatic and Terrestrial Ecosystems. Cambridge: Cambridge University Press. doi: 10.1017/9781108631129
Leland, A., Hoekman, S. K., and Liu, X. (2018). Review of modifications to indirect land use change modeling and resulting carbon intensity values within the California Low Carbon Fuel Standard regulations. J. Clean. Prod. 180, 698–707. doi: 10.1016/j.jclepro.2018.01.077
Levelt, P., van den Oord, G., Dobber, M., Malkki, A., Visser, H., Vries, J., et al. (2006). The ozone monitoring instrument. IEEE Trans. Geosci. Remote Sens. 44, 1093–1101. doi: 10.1109/TGRS.2006.872333
Levelt, P. F., Joiner, J., Tamminen, J., Veefkind, J. P., Bhartia, P. K., Stein Zweers, D. C., et al. (2018). The ozone monitoring instrument: overview of 14 years in space. Atmospheric Chem. Phys. 18, 5699–5745. doi: 10.5194/acp-18-5699-2018
Li, X., Li, X., Li, D., He, X., and Jendryke, M. (2019). A preliminary investigation of Luojia-1 night-time light imagery. Remote Sens. Lett. 10, 526–535. doi: 10.1080/2150704X.2019.1577573
Li, X., Wagner, F., Peng, W., Yang, J., and Mauzerall, D. L. (2017). Reduction of solar photovoltaic resources due to air pollution in China. Proc. Natl. Acad. Sci. 114, 11867–11872. doi: 10.1073/pnas.1711462114
Li, X., Zhou, Y., Gong, P., Seto, K., and Clinton, N. (2020b). Developing a method to estimate building height from Sentinel-1 data. Remote Sens. Environ. 240, 111705. doi: 10.1016/j.rse.2020.111705
Li, X., Zhou, Y., Zhao, M., and Zhao, X. A. (2020a). Harmonized global nighttime light dataset 1992–2018. Sci. Data 7, 168. doi: 10.1038/s41597-020-0510-y
Lin, Z., Anar, M. J., and Zheng, H. (2015). Hydrologic and water-quality impacts of agricultural land use changes incurred from bioenergy policies. J. Hydrol. 525, 429–440. doi: 10.1016/j.jhydrol.2015.04.001
Liu, F., Beirle, S., Zhang, Q., Drner, S., He, K., Wagner, T., et al. (2016). NOx lifetimes and emissions of cities and power plants in polluted background estimated by satellite observations. Atmospheric Chem. Phys. 16, 5283–5298. doi: 10.5194/acp-16-5283-2016
Liu, F., Beirle, S., Zhang, Q., van der, A. R. J, Zheng, B., et al. (2017). NOx mission trends over Chinese cities estimated from OMI observations during 2005 to 2015. Atmospheric Chem. Phys. 17, 9261–9275. doi: 10.5194/acp-17-9261-2017
Liu, F., Duncan, B. N., Krotkov, N. A., Lamsal, L. N., Beirle, S., Griffin, D., et al. (2020). Methodology to constrain carbon dioxide emissions from coal-fired power plants using satellite observations of co-emitted nitrogen dioxide. Atmospheric Chem. Phys. 20, 99–116. doi: 10.5194/acp-20-99-2020
Longato, D., Gaglio, M., Boschetti, M., and Gissi, E. (2019). Bioenergy and ecosystem services trade-offs and synergies in marginal agricultural lands: A remote-sensing-based assessment method. J. Clean. Prod. 237, 117672. doi: 10.1016/j.jclepro.2019.117672
Lu, Z., Streets, D. G., de Foy, B., Lamsal, L. N., Duncan, B. N., and Xing, J. (2015). Emissions of nitrogen oxides from US urban areas: estimation from Ozone Monitoring Instrument retrievals for 2005–2014. Atmospheric Chem. Phys. 15, 10367–10383. doi: 10.5194/acp-15-10367-2015
Lu, Z., Streets, D. G., Foy, d. e., and Krotkov, B. (2013). Ozone Monitoring Instrument Observations of Interannual Increases in SO2 Emissions from Indian Coal-Fired Power Plants during 2005–2012. Environ. Sci. Technol. 47, 13993–14000. doi: 10.1021/es4039648
Luo, T., Krishnaswami, A., and Li, X. (2018). A Methodology to Estimate Water Demand for Thermal Power Plants in Data-Scarce Regions Using Satellite Images. WRI. Available online at: https://www.wri.org/research/methodology-estimate-water-demand-thermal-power-plants-data-scarce-regions-using-satellite
Madsen, B. (2021). Identifying Wetlands with Deep Learning. EPRI Available online at: https://storymaps.arcgis.com/stories/4f98297b48a94efbbbe0199681539980
Mahdi Elsiddig Haroun, F., Mohamad Deros, S., and Din, N. A. (2020). Review of vegetation encroachment detection in power transmission lines using optical sensing satellite imagery. arXiv preprint arXiv:2010.01757. doi: 10.30534/ijatcse/2020/8691.42020
Mahtta, R., Mahendra, A., and Seto, K. C. (2019). Building up or spreading out? Typologies of urban growth across 478 cities of 1 million+. Environ. Res. Lett. 14, 124077. doi: 10.1088/1748-9326/ab59bf
Majidi Nezhad, M., Heydari, A., Pirshayan, E., Groppi, D., and Astiaso Garcia, D. A. (2021a). Novel forecasting model for wind speed assessment using sentinel family satellites images and machine learning method. Renew. Energy 179, 2198–2211. doi: 10.1016/j.renene.2021.08.013
Majidi Nezhad, M., Nastasi, B., Groppi, D., Lamagna, M., Piras, G., and Astiaso Garcia, D. (2021b). Green energy sources assessment using sentinel-1 satellite remote sensing. Front. Energy Res. 9, 649305. doi: 10.3389/fenrg.2021.649305
Malik Naureen, S. (2021). New Climate Satellite Spotted Giant Methane Leak as It Happened. Bloomberg News. Available online at: https://www.bloomberg.com/news/articles/2021-02-12/new-climate-satellite-spotted-giant-methane-leak-as-it-happened
Mann, M. L., Melaas, E. K., and Malik, A. (2016). Using VIIRS day/night band to measure electricity supply reliability: preliminary results from Maharashtra, India. Remote Sens. 8, 711. doi: 10.3390/rs8090711
Margetta, R. (2021). NASA releases climate action plan. NASA. Available online at: http://www.nasa.gov/press-release/nasa-releases-climate-action-plan
Matikainen, L., Lehtomki, M., Ahokas, E., Hyypp, J., Karjalainen, M., Jaakkola, A., et al. (2016). Remote sensing methods for power line corridor surveys. ISPRS J. Photogramm. Remote Sens. 119, 10–31. doi: 10.1016/j.isprsjprs.2016.04.011
Medina-Lopez, E., McMillan, D., Lazic, J., Hart, E., Zen, S., Angeloudis, A., et al. (2021). Satellite data for the offshore renewable energy sector: Synergies and innovation opportunities. Remote Sens. Environ. 264, 112588. doi: 10.1016/j.rse.2021.112588
Meijaard, E., Brooks, T. M., Carlson, K. M., Slade, E. M., Garcia-Ulloa, J., Gaveau, D. L., et al. (2020). The environmental impacts of palm oil in context. Nat. Plants 6, 1418–1426. doi: 10.1038/s41477-020-00813-w
Mellors, R. J., Xu, X., Matzel, E., and Fu, P. (2018). “New potential of InSAR for geothermal Systems,” in Proceedings of the 43rd Workshop on Geothermal Reservoir Engineering (Stanford, CA).
Milford, J. B., and Knight, D. (2017). Increasing the use of Earth science data and models in air quality management. J. Air Waste Manag. Assoc. 67, 431–444. doi: 10.1080/10962247.2016.1248303
Min, B., Baugh, K., Monroe, T., Goldblatt, R., Steward, B., Kosmidou-Bradley, W., et al. (2021). Light Every Night – New nighttime light data set and tools for development. World Bank Blogs. Available online at: https://blogs.worldbank.org/opendata/light-every-night-new-nighttime-light-data-set-and-tools-development (accessed July 11, 2022).
Min, B., Gaba, K. M., Sarr, O. F., and Agalassou, A. (2013). Detection of rural electrification in Africa using DMSP-OLS night lights imagery. Int. J. Remote Sens. 34, 8118–8141. doi: 10.1080/01431161.2013.833358
Mitchell, J. W. (2013). Power line failures and catastrophic wildfires under extreme weather conditions. Eng. Fail. Anal. 35, 726–735. doi: 10.1016/j.engfailanal.2013.07.006
Moner-Girona, M., Bdis, K., Morrissey, J., Kougias, I., Hankins, M., Huld, T., et al. (2019). Decentralized rural electrification in Kenya: speeding up universal energy access. Energy Sustain. Dev. 52, 128–146. doi: 10.1016/j.esd.2019.07.009
Moner-Girona, M., Kakoulaki, G., Falchetta, G., Weiss, D. J., and Taylor, N. (2021). Achieving universal electrification of rural healthcare facilities in sub-Saharan Africa with decentralized renewable energy technologies. Joule 5, 2687–2714. doi: 10.1016/j.joule.2021.09.010
Moore, I. I. I., Crowell, B., Rayner, S. M., Kumer, P. J., O'Dell, J., O'Brien, C. W., et al. (2018). The potential of the geostationary carbon cycle observatory (GeoCarb) to provide multi-scale constraints on the carbon cycle in the Americas. Front. Environ. Sci. 6, 109. doi: 10.3389/fenvs.2018.00109
Motamed, M., McPhail, L., and Williams, R. (2016). Corn area response to local ethanol markets in the United States: a grid cell level analysis. Am. J. Agric. Econ. 98, 726–743. doi: 10.1093/ajae/aav095
Munro, R., Lang, R., Klaes, D., Poli, G., Retscher, C., Lindstrot, R., et al. (2016). The GOME-2 instrument on the Metop series of satellites: instrument design, calibration, and level 1 data processing – an overview. Atmospheric Meas. Tech. 9, 1279–1301. doi: 10.5194/amt-9-1279-2016
Næss, J. S., Cavalett, O., and Cherubini, F. (2021). The land–energy–water nexus of global bioenergy potentials from abandoned cropland. Nat. Sustain. 4, 525–536. doi: 10.1038/s41893-020-00680-5
Nalepa, R. A., and Bauer, D. M. (2012). Marginal lands: the role of remote sensing in constructing landscapes for agrofuel development. J. Peasant Stud. 39, 403–422. doi: 10.1080/03066150.2012.665890
NASA (2015). Jet Propulsion Laboratory AIRS Animation Shows Carbon Monoxide Plume Transport from U.S. Fires. AIRS Available online at: https://airs.jpl.nasa.gov/news/136/airs-animation-shows-carbon-monoxide-plume-transport-from-us-fires (accessed July 11, 2022).
NASA (2016). Earth Observatory Satellite Detects Human Contribution to Atmospheric CO2. Available online at: https://earthobservatory.nasa.gov/images/89117/satellite-detects-human-contribution-to-atmospheric-co2
NASA (2021). Fire Information for Resource Management System (FIRMS) | Earthdata. Available online at: https://earthdata.nasa.gov/earth-observation-data/near-real-time/firms/ (accessed July 11, 2022).
NASA Earth Observatory (2020). Airborne Nitrogen Dioxide Plummets Over China. Available online at: https://earthobservatory.nasa.gov/images/146362/airborne-nitrogen-dioxide-plummets-over-china (accessed July 11, 2022).
NASA Earth Observatory (2021a). Plugging-in Sub-Saharan Africa. Available online at: https://earthobservatory.nasa.gov/images/148069/plugging-in-sub-saharan-africa
NASA Earth Observatory (2021b). Satellites View California Oil Spill. Available online at: https://earthobservatory.nasa.gov/images/148929/satellites-view-california-oil-spill
NASA Earth Observatory (2022). Iberian Peninsula Drought. Available online at: https://earthobservatory.nasa.gov/images/149469/iberian-peninsula-drought (accessed July 11, 2022).
NASA Earthdata (2021). Nighttime Lights. Available online at: https://earthdata.nasa.gov/learn/backgrounders/nighttime-lights/
NASA Goddard Space Flight Center (2021). NASA Black Marble Product. Available online at: https://viirsland.gsfc.nasa.gov/Products/NASA/BlackMarble.html
NASA POWER NASA ARSET (2021). How to Utilize NASA Resources for Energy Efficiency and Renewable Energy Applications. Available online at: https://appliedsciences.nasa.gov/sites/default/files/2021-06/Energy_Management_Part4.pdf
Nassar, R., Hill, T. G., McLinden, C. A., Wunch, D., Jones, D. B. A., Crisp, D., et al. (2017). Quantifying CO2 emissions from individual power plants from space. Geophys. Res. Lett. 44, 10,045–10,053 doi: 10.1002/2017GL074702
Nassar, R., Mastrogiacomo, J. P., Bateman-Hemphill, W., McCracken, C., MacDonald, C. G., et al. (2021). Advances in quantifying power plant CO2 emissions with OCO-2. Remote Sens. Environ. 264, 112579. doi: 10.1016/j.rse.2021.112579
Nazir, H. (2021). Lessons Learned from the February 2021 Texas Power Outage. Canadian Energy Research Institute Available online at: https://ceri.ca/market-updates/lessons-learned-from-the-february-2021-texas-power-outage
Nik, V. M., Perera, A. T. D., and Chen, D. (2021). Towards climate resilient urban energy systems: a review. Natl. Sci. Rev. 8, nwaa134. doi: 10.1093/nsr/nwaa134
Noktosat (2021). Noktosat Satellite Images - The earth at night in colour. Available online at: https://www.noktosat.com
Pacific Gas Electric Company (2021). 2021 Wildfire Mitigation Plan Report. Available online at: https://energysafety.ca.gov/wp-content/uploads/docs/wmp-2021/pge-2021-wmp-update.pdf
Pandey, B., Brelsford, C., and Seto, K. C. (2022). Infrastructure inequality is a characteristic of urbanization. Proc. Natl. Acad. Sci. 119, e2119890119. doi: 10.1073/pnas.2119890119
Park, H., Jeong, S., Park, H., Labzovskii, L. D., and Bowman, K. W. (2021). An assessment of emission characteristics of Northern Hemisphere cities using spaceborne observations of CO2, CO, and NO2. Remote Sens. Environ. 254, 112246. doi: 10.1016/j.rse.2020.112246
Pelz, S., Pachauri, S., and Groh, S. (2018). A critical review of modern approaches for multidimensional energy poverty measurement. WIREs Energy Environ. 7, e304. doi: 10.1002/wene.304
Pelz, S., Pachauri, S., and Rao, N. (2021). Application of an alternative framework for measuring progress towards SDG 7.1. Environ. Res. Lett. 16, 084048. doi: 10.1088/1748-9326/ac16a1
Perez, R., Cebecauer, T., and Šúri, M. (2013). “Chapter 2 - Semi-Empirical Satellite Models,” in Solar Energy Forecasting and Resource Assessment (ed. Kleissl, J.) p. 21–48 (Academic Press). doi: 10.1016/B978-0-12-397177-7.00002-4
Peters, I. M., Liu, H., Reindl, T., and Buonassisi, T. (2018). Global prediction of photovoltaic field performance differences using open-source satellite data. Joule. 2, 307–322. doi: 10.1016/j.joule.2017.11.012
Pfenninger, S., and Staffell, I. (2016). Long-term patterns of European PV output using 30 years of validated hourly reanalysis and satellite data. Energy 114, 1251–1265. doi: 10.1016/j.energy.2016.08.060
Popp, J., Lakner, Z., Harangi-Rákos, M., and Fári, M. (2014). The effect of bioenergy expansion: Food, energy, and environment. Renew. Sustain. Energy Rev. 32, 559–578. doi: 10.1016/j.rser.2014.01.056
Ravikumar, A. P., Roda-Stuart, D., Liu, R., Bradley, A., Bergerson, J., Nie, Y., et al. (2020). Repeated leak detection and repair surveys reduce methane emissions over scale of years. Environ. Res. Lett. 15, 034029. doi: 10.1088/1748-9326/ab6ae1
Reames, T. G. (2016). Targeting energy justice: Exploring spatial, racial/ethnic and socioeconomic disparities in urban residential heating energy efficiency. Energy Policy 97, 549–558. doi: 10.1016/j.enpol.2016.07.048
Reba, M., and Seto, K. (2020). A systematic review and assessment of algorithms to detect, characterize, and monitor urban land change. Remote Sens. Environ. 242, 111739. doi: 10.1016/j.rse.2020.111739
Receveur, M., Sigmundsson, F., Drouin, V., and Parks, M. (2019). Ground deformation due to steam cap processes at Reykjanes, SW-Iceland: effects of geothermal exploitation inferred from interferometric analysis of Sentinel-1 images 2015–2017. Geophys. J. Int. 216, 2183–2212. doi: 10.1093/gji/ggy540
Reuter, M., Buchwitz, M., Schneising, O., Krautwurst, S., O'Dell, C. W., Richter, A., et al. (2019). Towards monitoring localized CO2 emissions from space: co-located regional CO2 and NO2 enhancements observed by the OCO-2 and S5P satellites. Atmospheric Chem. Phys. 19, 9371–9383. doi: 10.5194/acp-19-9371-2019
Román, M. O., and Stokes, E. C. (2015). Holidays in lights: Tracking cultural patterns in demand for energy services. Earths Future. 3, 182–205. doi: 10.1002/2014EF000285
Romn, M. O., Stokes, E. C., Shrestha, R., Wang, Z., Schultz, L., Carlo, E. A. S., et al. (2019). Satellite-based assessment of electricity restoration efforts in Puerto Rico after Hurricane Maria. PLoS ONE 14, e0218883. doi: 10.1371/journal.pone.0218883
Romn, M. O., Wang, Z., Sun, Q., Kalb, V., Miller, S. D., Molthan, A., et al. (2018). NASA's Black Marble nighttime lights product suite. Remote Sens. Environ. 210, 113–143. doi: 10.1016/j.rse.2018.03.017
Rose, R. A., Byler, D., Eastman, J. R., Fleishman, E., Geller, G., Goetz, S., et al. (2015). Ten ways remote sensing can contribute to conservation. Conserv. Biol. 29, 350–359. doi: 10.1111/cobi.12397
Rosenzweig, C., Horton, R. M., Bader, D. A., Brown, M. E., DeYoung, R., Dominguez, O., et al. (2014). Enhancing climate resilience at NASA centers: a collaboration between science and stewardship. Bull. Am. Meteorol. Soc. 95, 1351–1363. doi: 10.1175/BAMS-D-12-00169.1
RSPO (2021). - Roundtable on Sustainable Palm Oil. Hotspot Hub — RSPO strengthens fire prevention with satellite technology. Available online at: https://rspo.org/news-and-events/news/hotspot-hub–rspo-strengthens-fire-prevention-with-satellite-technology (accessed July 11, 2022).
Rutan, D. A., Kato, S., Doelling, D. R., Rose, F. G., Nguyen, L. T., Caldwell, T. E., et al. (2015). Synoptic product: methodology and validation of surface radiant flux. J. Atmospheric Ocean. Technol. 32, 1121–1143. doi: 10.1175/JTECH-D-14-00165.1
San Diego Gas Electric Company (2021). 2020-2022 Wildfire Mitigation Plan Update. Available online at: https://energysafety.ca.gov/wp-content/uploads/docs/misc/wmp/2021/utility/sdge/sdge-2021-wmp-update.pdf (accessed July 11, 2022).
Sanders, K. T., and Masri, S. F. (2016). The energy-water agriculture nexus: the past, present and future of holistic resource management via remote sensing technologies. J. Clean. Prod. 117, 73–88. doi: 10.1016/j.jclepro.2016.01.034
Schneising, O., Heymann, J., Buchwitz, M., Reuter, M., Bovensmann, H., Burrows, J. P., et al. (2013). Anthropogenic carbon dioxide source areas observed from space: assessment of regional enhancements and trends. Atmospheric Chem. Phys. 13, 2445–2454. doi: 10.5194/acp-13-2445-2013
Sengupta, M., Xie, Y., Lopez, A., Habte, A., Maclaurin, G., Shelby, J., et al. (2018). The National Solar Radiation Data Base (NSRDB). Renew. Sustain. Energy Rev. 89, 51–60. doi: 10.1016/j.rser.2018.03.003
Seto, K., Dhakal, S., Bigio, A., Blanco, H., Delgado, G., Dewar, D., et al. (2014). Chapter 12 - Human Settlements, Infrastructure and Spatial Planning. Cambridge: Cambridge University Press.
Shen, L., Xu, H., and Guo, X. (2012). Satellite remote sensing of harmful algal blooms (HABs) and a potential synthesized framework. Sensors 12, 7778–7803. doi: 10.3390/s120607778
Sierk, B., Bezy, J-. L., Löscher, A., and Meijer, Y. (2019). “The European CO2 Monitoring Mission: observing anthropogenic greenhouse gas emissions from space,” in International Conference on Space Optics—ICSO 2018 (Chania: SPIE), 11180, 237–250. doi: 10.1117/12.2535941
Silva, S., and Arellano, A. (2017). Characterizing Regional-Scale Combustion Using Satellite Retrievals of CO, NO2 and CO2. Remote Sens. 9, 744. doi: 10.3390/rs9070744
Smith, W. K., Cleveland, C. C., Reed, S. C., Miller, N. L., and Running, S. W. (2012). Bioenergy potential of the united states constrained by satellite observations of existing productivity. Environ. Sci. Technol. 46, 3536–3544. doi: 10.1021/es203935d
Soukissian, T. H., and Papadopoulos, A. (2015). Effects of different wind data sources in offshore wind power assessment. Renew. Energy 77, 101–114. doi: 10.1016/j.renene.2014.12.009
Southern California Edison Company (2021). 2021 Wildfire Mitigation Plan Update. Available online at: https://energysafety.ca.gov/wp-content/uploads/docs/wmp-2021/sce-2021-wmp-update.pdf (accessed July 11, 2022).
Sridhar, J. B. M., and Vincent, R. K. (2009). Mapping and Estimation of Phosphorus and Copper Concentrations in Fly Ash Spill Area using LANDSAT TM Images. Sci. Total Environ. 407, 1031. doi: 10.1016/j.scitotenv.2009.01.021
Stackhouse, P. W., Cox, S. J., Mikovitz, J. C., and Zhang, T. (2021). GEWEX (Global Energy and Water Exchanges Project): Surface Radiation Budget (SRB) Release 4 Integrated Product (IP4) - Algorithm Theoretical Basis Document and Evaluation.
Steele, J. E., Sundsy, P. R., Pezzulo, C., Alegana, V. A., Bird, T. J., Blumenstock, J., et al. (2017). Mapping poverty using mobile phone and satellite data. J. R. Soc. Interface 14, 20160690. doi: 10.1098/rsif.2016.0690
Stiles, G., and Murove, C. (2019). 2018. SADC Renewable Energy and Energy Efficiency Status Report. REN21. (2019). Available online at: https://www.ren21.net/2018-sadc-renewable-energy-and-energy-efficiency-status-report/ (accessed July 11, 2022).
Stokes, E., and Seto, K. (2019). Characterizing urban infrastructural transitions for the Sustainable Development Goals using multi-temporal land, population, and nighttime light data. Remote Sens. Environ. 234, 111430. doi: 10.1016/j.rse.2019.111430
Stokes, E. C., and Román, M. O. (2022). Tracking COVID-19 urban activity changes in the Middle East from nighttime lights. Sci. Rep. 12, 8096. doi: 10.1038/s41598-022-12211-7
The White House (2021a). Executive Order on Tackling the Climate Crisis at Home and Abroad. Available online at: https://www.whitehouse.gov/briefing-room/presidential-actions/2021/01/27/executive-order-on-tackling-the-climate-crisis-at-home-and-abroad/
The White House (2021b). FACT SHEET: President Biden Takes Executive Actions to Tackle the Climate Crisis at Home and Abroad, Create Jobs, and Restore Scientific Integrity Across Federal Government. Available online at: https://www.whitehouse.gov/briefing-room/statements-releases/2021/01/27/fact-sheet-president-biden-takes-executive-actions-to-tackle-the-climate-crisis-at-home-and-abroad-create-jobs-and-restore-scientific-integrity-across-federal-government/
Timmermans, R., Segers, A., Curier, L., Abida, R., Atti, J. L., El Amraoui, L., et al. (2019). Impact of synthetic space-borne NO2 observations from the Sentinel-4 and Sentinel-5P missions on tropospheric NO2 analyses. Atmospheric Chem. Phys. 19, 12811–12833. doi: 10.5194/acp-19-12811-2019
Tollenaar, M., Fridgen, J., Tyagi, P., Stackhouse, P. W., and Kumudini, S. (2017). The contribution of solar brightening to the US maize yield trend. Nat. Clim. Change 7, 275–278. doi: 10.1038/nclimate3234
Tsao, C-. C., Campbell, J. E., Mena-Carrasco, M., Spak, S. N., Carmichael, G. R., et al. (2012). Increased estimates of air-pollution emissions from Brazilian sugar-cane ethanol. Nat. Clim. Change 2, 53–57. doi: 10.1038/nclimate1325
U.S. Department of Energy (2021). 2021 Climate Adaptation and Resilience Plan. Available online at: https://www.energy.gov/sites/default/files/2021-09/DOEClimateAdaptationandResiliencePlanSept21.pdf (accessed July 11, 2022).
UN Environment Programme (2021). New global methane pledge aims to tackle climate change. UNEP Available online at: http://www.unep.org/news-and-stories/story/new-global-methane-pledge-aims-tackle-climate-change(accessed July 11, 2022).
US Department of Commerce (2021). Department of Commerce Releases Climate Action Plan for Adaptation and Resilience. Available online at: https://www.commerce.gov/news/press-releases/2021/10/department-commerce-releases-climate-action-plan-adaptation-and (accessed July 11, 2022).
US EPA (2010). Office of Transportation and Air Quality, Assessment and Standards Division. Renewable Fuel Standard Program (RFS2) Regulatory Impact Analysis. Available online at: https://www.eesi.org/files/420r10006.pdf (accessed July 11, 2022).
van Donkelaar, A., Martin, R. V., Brauer, M., Kahn, R., Levy, R., Verduzco, C., et al. (2010). Global Estimates of Ambient Fine Particulate Matter Concentrations from Satellite-Based Aerosol Optical Depth: Development and Application. Environ. Health Perspect. 118, 847–855. doi: 10.1289/ehp.0901623
Vargas, C. A., Caracciolo, L., and Ball, P. J. (2022). Geothermal energy as a means to decarbonize the energy mix of megacities. Commun. Earth Environ. 3, 1–11. doi: 10.1038/s43247-022-00386-w
Varon, D. J., McKeever, J., Jervis, D., Maasakkers, J. D., Pandey, S., Houweling, S., et al. (2019). Satellite Discovery of Anomalously Large Methane Point Sources From Oil/Gas Production. Geophys. Res. Lett. 46, 13507–13516. doi: 10.1029/2019GL083798
Veefkind, J. P., Aben, I., McMullan, K., Frster, H., de Vries, J., Otter, G., et al. (2012). TROPOMI on the ESA Sentinel-5 Precursor: A GMES mission for global observations of the atmospheric composition for climate, air quality and ozone layer applications. Remote Sens. Environ. 120, 70–83. doi: 10.1016/j.rse.2011.09.027
Velazco, V. A., Buchwitz, M., Bovensmann, H., Reuter, M., Schneising, O., Heymann, J., et al. (2011). Towards space based verification of CO2 emissions from strong localized sources: fossil fuel power plant emissions as seen by a CarbonSat constellation. Atmospheric Meas. Tech. 4, 2809–2822. doi: 10.5194/amt-4-2809-2011
Wagle, P., Bhattarai, N., Gowda, P. H., and Kakani, V. G. (2017). Performance of five surface energy balance models for estimating daily evapotranspiration in high biomass sorghum. ISPRS J. Photogramm. Remote Sens. 128, 192–203. doi: 10.1016/j.isprsjprs.2017.03.022
Wang, E., Cruse, R. M., Sharma-Acharya, B., Herzmann, D. E., Gelder, B. K., James, D. E., et al. (2020). Strategic switchgrass (Panicum virgatum) production within row cropping systems: Regional-scale assessment of soil erosion loss and water runoff impacts. GCB Bioenergy 12, 955–967. doi: 10.1111/gcbb.12749
Wang, S., Zhang, Y., Hakkarainen, J., Ju, W., Liu, Y., Jiang, F., et al. (2018). Distinguishing Anthropogenic CO2 emissions from different energy intensive industrial sources using OCO-2 observations: a case study in northern China. J. Geophys. Res. Atmospheres 123, 9462–9473. doi: 10.1029/2018JD029005
Wheeler, D., and Ummel, K. (2008). Calculating Carma: Global Estimation of CO2 Emissions from the Power Sector. Available online at: https://papers.ssrn.com/abstract=1138690 doi: 10.2139/ssrn.1138690 (accessed July 11, 2022).
Witman, S., Halloway, T., and Reddy, P. J. (2014). Integrating Satellite Data into Air Quality Management. Experience from Colorado. NASA Available online at: https://pubs.awma.org/gsearch/em/2014/2/witman.pdf (accessed July 11, 2022).
World Bank (2019). Tracking SDG7: The Energy Progress Report 2019. Available online at: https://www.worldbank.org/en/topic/energy/publication/tracking-sdg7-the-energy-progress-report-2019 (accessed July 11, 2022).
World Health Organization (2016). WHO releases country estimates on air pollution exposure and health impact. Available online at: https://www.who.int/news/item/27-09-2016-who-releases-country-estimates-on-air-pollution-exposure-and-health-impact (accessed July 11, 2022).
Wright, C. K., Larson, B., Lark, T. J., and Gibbs, H. K. (2017). Recent grassland losses are concentrated around U.S. ethanol refineries. Environ. Res. Lett. 12, 044001. doi: 10.1088/1748-9326/aa6446
Wu, C., Wang, Q., Yang, Z., and Wang, W. (2007). Monitoring heated water pollution of the DaYaWan nuclear power plant using TM images. Int. J. Remote Sens. 28, 885–890. doi: 10.1080/01431160600580616
Wu, D., Lin, J. C., Oda, T., and Kort, E. A. (2020). Space-based quantification of per capita CO2 emissions from cities. Environ. Res. Lett. 15, 035004. doi: 10.1088/1748-9326/ab68eb
Yokota, T., Yoshida, Y., Eguchi, N., Ota, Y., Tanaka, T., Watanabe, H., et al. (2009). Global Concentrations of CO2 and CH4 Retrieved from GOSAT: first preliminary results. Sola. 5, 160–163. doi: 10.2151/sola.2009-041
Yu, J., Wang, Z., Majumdar, A., and Rajagopal, R. (2018). DeepSolar: a machine learning framework to efficiently construct a solar deployment database in the United States. Joule 2, 2605–2617. doi: 10.1016/j.joule.2018.11.021
Zavala-Araiza, D., Alvarez, R. A., Lyon, D. R., Allen, D. T., Marchese, A. J., Zimmerle, D. J., et al. (2017). Super-emitters in natural gas infrastructure are caused by abnormal process conditions. Nat. Commun. 8, 14012. doi: 10.1038/ncomms14012
Zell, E., Engel-Cox, J., Eckman, R., and Stackhouse, P. (2008). Application of satellite sensor data and models for energy management. IEEE J. Sel. Top. Appl. Earth Obs. Remote Sens. 1, 5–17. doi: 10.1109/JSTARS.2008.2001142
Zen, S., Hart, E., and Mendia-Lopez, E. (2021). The use of satellite products to assess spatial uncertainty and reduce life-time costs of offshore wind farms. Clean. Environ. Syst. 2, 100008. doi: 10.1016/j.cesys.2020.100008
Zhang, Y., Rossow, W., Lacis, A., Oinas, V., and Mishchenko, M. (2004). Calculation of radiative fluxes from the surface to top of atmosphere based on ISCCP and other global data sets: Refinements of the radiative transfer model and the input data. J Geophys. Res. Atmospheres. 109, 4457. doi: 10.1029/2003JD004457
Zhao, M., Zhou, Y., Li, X., Cao, W., He, C., Yu, B., et al. (2019). Applications of Satellite Remote Sensing of Nighttime Light Observations: Advances, Challenges, and Perspectives. Remote Sens. 11, 1971. doi: 10.3390/rs11171971
Zheng, T., Nassar, R., and Baxter, M. (2019). Estimating power plant CO2 emission using OCO-2 XCO2 and high resolution WRF-Chem simulations. Environ. Res. Lett. 14, 085001. doi: 10.1088/1748-9326/ab25ae
Zhu, Y., Xu, D., Ali, S. H., Ma, R., and Cheng, J. (2019a). Can nighttime light data be used to estimate electric power consumption? New evidence from causal-effect inference. Energies 12, 3154. doi: 10.3390/en12163154
Zhu, Z., Zhou, Y., Seto, K. C., Stokes, E. C., Deng, C., Pickett, S. T. A., et al. (2019b). Understanding an urbanizing planet: strategic directions for remote sensing. Remote Sens. Environ. 228, 164–182. doi: 10.1016/j.rse.2019.04.020
Zoogman, P., Liu, X., Suleiman, R. M., Pennington, W. F., Flittner, D. E., Al-Saadi, J. A., et al. (2017). Tropospheric emissions: monitoring of pollution (TEMPO). J. Quant. Spectrosc. Radiat. Transf. 186, 17–39. doi: 10.1016/j.jqsrt.2016.05.008
Keywords: energy, satellite, sustainability, decision-making, data
Citation: Edwards MR, Holloway T, Pierce RB, Blank L, Broddle M, Choi E, Duncan BN, Esparza Á, Falchetta G, Fritz M, Gibbs HK, Hundt H, Lark T, Leibrand A, Liu F, Madsen B, Maslak T, Pandey B, Seto KC and Stackhouse PW Jr (2022) Satellite Data Applications for Sustainable Energy Transitions. Front. Sustain. 3:910924. doi: 10.3389/frsus.2022.910924
Received: 01 April 2022; Accepted: 21 June 2022;
Published: 03 October 2022.
Edited by:
Shutaro Takeda, Kyoto University, JapanReviewed by:
Deepak Rajagopal, University of California, Los Angeles, United StatesDaikichi Seki, Kyoto University, Japan
Copyright © 2022 Edwards, Holloway, Pierce, Blank, Broddle, Choi, Duncan, Esparza, Falchetta, Fritz, Gibbs, Hundt, Lark, Leibrand, Liu, Madsen, Maslak, Pandey, Seto and Stackhouse. This is an open-access article distributed under the terms of the Creative Commons Attribution License (CC BY). The use, distribution or reproduction in other forums is permitted, provided the original author(s) and the copyright owner(s) are credited and that the original publication in this journal is cited, in accordance with accepted academic practice. No use, distribution or reproduction is permitted which does not comply with these terms.
*Correspondence: Morgan R. Edwards, bW9yZ2FuLmVkd2FyZHNAd2lzYy5lZHU=