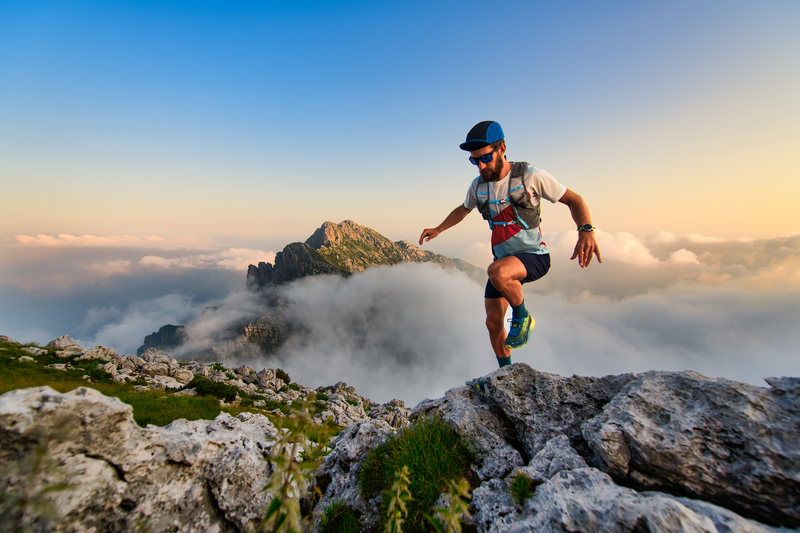
94% of researchers rate our articles as excellent or good
Learn more about the work of our research integrity team to safeguard the quality of each article we publish.
Find out more
CORRECTION article
Front. Sports Act. Living , 20 February 2025
Sec. Sports Science, Technology and Engineering
Volume 7 - 2025 | https://doi.org/10.3389/fspor.2025.1570591
This article is part of the Research Topic Harnessing Artificial Intelligence in Sports Science: Enhancing Performance, Health, and Education View all articles
This article is a correction to:
Addressing Grading Bias in Rock Climbing: Machine and Deep Learning Approaches
A corrigendum on
Addressing grading bias in rock climbing: machine and deep learning approaches
O’Mara B, Mahmud MS. (2025). Addressing grading bias in rock climbing: machine and deep learning approaches. Front. Sports Act. Living 6:1512010. doi: 10.3389/fspor.2024.1512010
In the published article. [Dataset] Singh S. Rock. Climbing Gym Market Size, Share, Report, Forecast 2032 (2024) was not cited in the article. The citation has now been inserted in Introduction, paragraph 2 and should read:
Climbing accessibility is highly dependent on route setters. Route setters produce climbing routes, the central service of a climbing gym. They are responsible for producing routes that are varied yet consistent in difficulty. Gyms vary their route difficulties to capture the largest audience possible (1), catering to a range of climber experience levels from novice to advanced. However, the grading scales used to rate climbing route difficulty are often subjective according to the region, the gym, and the setter of the route (2). General factors considered when determining route difficulty are rock hold types, the number of rock holds on a route, the distance between the rock holds, and the angle of ascent (3). Therefore, it seems that the positioning and sequencing of holds are critical to route difficulty. But holds may be positioned and sequenced in an almost infinite number of ways. Setting a route is like composing a song (4, 5); there are constraints that govern its composition, but the liberty to operate within those constraints is quite large. When operating within these constraints, a route can be developed in a multitude of ways. This wide variance of route generation is a challenge for generalizing route difficulty. without a large sample size, route setters introduce their own biases when determining route difficulty, which then inadvertently affects the climber (i.e., the customer).
The authors apologize for this error and state that this does not change the scientific conclusions of the article in any way. The original article has been updated.
All claims expressed in this article are solely those of the authors and do not necessarily represent those of their affiliated organizations, or those of the publisher, the editors and the reviewers. Any product that may be evaluated in this article, or claim that may be made by its manufacturer, is not guaranteed or endorsed by the publisher.
3. Seifert L, Wolf P, Schweizer A. Climbing grades: systems and subjectivity. In: The Science of Climbing and Mountaineering. Paperback ed. Routledge (2017). p. 227–43. Routledge Research in Sport and Exercise Science.
4. Phillips C, Becker L, Bradley E. Strange beta: an assistance system for indoor rock climbing route setting using chaotic variations and machine learning. Chaos. (2012) 22:013130. doi: 10.1063/1.3693047
Keywords: rock climbing, bouldering, route grade difficulty, deep learning, machine learning
Citation: O’Mara B and Mahmud MS (2025) Corrigendum: Addressing grading bias in rock climbing: machine and deep learning approaches. Front. Sports Act. Living 7:1570591. doi: 10.3389/fspor.2025.1570591
Received: 3 February 2025; Accepted: 10 February 2025;
Published: 20 February 2025.
Edited and Reviewed by: Pedro Forte, Higher Institute of Educational Sciences of the Douro, Portugal
Copyright: © 2025 O’Mara and Mahmud. This is an open-access article distributed under the terms of the Creative Commons Attribution License (CC BY). The use, distribution or reproduction in other forums is permitted, provided the original author(s) and the copyright owner(s) are credited and that the original publication in this journal is cited, in accordance with accepted academic practice. No use, distribution or reproduction is permitted which does not comply with these terms.
*Correspondence: M. S. Mahmud, bWRzaGFhZC5tYWhtdWRAdW5oLmVkdQ==
Disclaimer: All claims expressed in this article are solely those of the authors and do not necessarily represent those of their affiliated organizations, or those of the publisher, the editors and the reviewers. Any product that may be evaluated in this article or claim that may be made by its manufacturer is not guaranteed or endorsed by the publisher.
Research integrity at Frontiers
Learn more about the work of our research integrity team to safeguard the quality of each article we publish.