Erratum: Precipitation-derived effects on the characteristics of proteinaceous soil organic matter across the continental United States
- 1School of Plant and Environmental Sciences, Virginia Tech, Blacksburg, VA, United States
- 2Key Laboratory of the Ministry of Education for Coastal and Wetland Ecosystems, Xiamen University, Xiamen, China
- 3US Geological Survey, Denver, CO, United States
- 4Rhizosphere and Soil Microbial Ecology Laboratory, Virginia Tech, Blacksburg, VA, United States
Proteinaceous amino acids composed up to 50% of microbial biomass, are a primary building block of soil organic nitrogen, and play a key role in soil organic N and C cycling. However, the large-scale drivers on these organic nitrogen pools is less explored. We hypothesized that the trends related to vegetation, soil mineralogy and climate will change the composition of hydrolyzable amino acids (HAAs), both within and between each horizon. Herein we report on the patterns of HAAs, and their dependence on soil (e.g., Al, Fe, pH) and climate (e.g., precipitation) factors between soil horizons across the continental U.S. It was found that the effect of vegetation type on HAAs was greater in the A horizon than in the C horizon, which was related to the different stages of the vegetation-associated decomposition and pedogenesis processes. A similar Leu-Phe-Ile-Gly co-occurrence structure was found in both soil horizons suggesting some similarity in processes that enrich organics in soil. Precipitation, but not temperature, showed significant associations with HAA composition. The chemical properties of the soil, including pH and mineral metals (Fe, Mn, Al, Ca), also influenced the HAAs’ characteristics. In particular, some specific HAAs (Glx, Asn, and Ala) mainly reflected the HAAs’ response to the environmental gradients in both horizons. The effect of precipitation on HAAs exhibits as a complex relationship mediated through organic matter, pH and minerals. To our knowledge, this is the first study to assess continental-wide descriptors of the largest soil organic N pool, showing that pH, Fe, Ca, precipitation and vegetation explain soil AA composition. The role played by each of these drivers in the accrual and turnover of organic matter over large regional scales deserve further scrutiny. The large surface and subsurface HAA data set from this study should help change the way micro-scale conceptual and mechanistic models describe the chemical interactions and source pools that drive soil organic nitrogen, and possibly soil organic matter composition over regional scales.
Introduction
Proteinaceous amino acids, such as those found in proteins, peptides, and free forms in soil microbial biomass, ultimately define the bioavailable and long-term stabilized pools of C and N (1, 2). The breakdown of soil peptides and proteins to amino acids (AAs) is a primary limiting step for N mineralization (3). AAs, peptides, and proteins are thus an important determinant of available N for plants and pool sizes of OM in soil. Research has been conducted to determine the spatial-temporal patterns of soil proteinaceous organic matter (OM) composition, which play a key role in biogeochemical processes and OM accrual in soil (4, 5). There remains, however, a lack of field-based evidence across climate gradients and vegetation types to determine whether there are environmental factors that influence OM composition, which may have ramifications for their accrual and turnover at a continental scale.
The role and stabilization of proteinaceous compounds have been described in the molecular aggregates and other models (6–9), which show great potential in interpreting and forecasting global C and N cycling (10, 11). Numerous functional groups of proteinaceous compounds are predicted to interact with each other, with other soil molecules and mineral surfaces, causing them to be less mobile and more protected from disassociation and decomposition (12, 13).
Minerals play a critical role in the selective preservation of proteinaceous OM (14, 15). Microbial-derived compounds are one of crucial sources of the mineral-associated OM (16–18). The distribution of proteinaceous compounds was investigated in a Hawaiian rainforest chronosequence soil with ~4.1 million years of development, showing the importance of noncrystalline or short-range ordered minerals in the retention of acidic amino acids like aspartic- and glutamic acids (16). Similarly, positive amino acids are found more commonly in younger soils due to electrostatic interactions and organic-organic interactions (1, 19). These results highlight the connection between pedogenesis and molecular details of OM accumulation over tens of thousands of years and more (20).
In addition to minerals, proteinaceous amino acids produced by microorganisms can bind to root-derived compounds, thus prolonging their presence in the environment (21). For instance, root-derived condensed tannins would transform fungal residues into more persistent forms and slow decomposition (22). Plants may also influence OM composition. Plant litter is a crucial source of OM in the soil (23) and a carbon source for microbial growth (18, 24). Plant litter quality can also affect microbial succession during decomposition (25). Moreover, root rhizodeposits can recruit specific microorganisms and change rhizosphere microbial communities (26). These microbes are the major conduit through which organic matter flows to form soil organic matter (21).
It is expected that different dynamics would occur in soils associated with different parent materials, associated with different climates, and soil factors such as mineralogy, pH and elemental composition (e.g., Al, Fe). Discerning how these factors influence OM content and composition will move us toward a more mechanistic understanding of OM stabilization in soils, and thus a better idea of how they may respond to global environmental changes (3).
The composition of hydrolyzable AA (HAA) pools, which represent a major portion of AAs from biomass and residues in soils, have been reported to be rather uniform across the limited number of soils investigated, with aspartic acid, glutamic acid, glycine, and alanine as dominant amino acids (27–30). Even less information is available on HAA pools in subsurface soils, despite the mass of OM in subsurface soils accounting for up to 50% of the global soil organic C and N (31, 32). The objective of this research was, therefore, to conduct a systematic investigation on the HAA pools in the A- and C-horizon soils across a range of ecosystems, soil types, temperature and precipitation regimes along a North-South (N-S) and East-West (E-W) transect, respectively, of the continental United States. In both the A and C horizon, it was hypothesized that mineralogy, vegetation, precipitation, pH, and elemental concentrations of Al and Fe would be associated with soil AA composition (33).
Materials and methods
Soil samples used for this study were a subset of samples from a total of 4871 sites (1 site/1600 km2) collected by the USGS from 2007 to 2010 for the USGS Geochemical Landscapes Project (34). As shown in Figure 1, there were 46 and 103 sites selected along transects of East-West (annual average precipitation gradient) and North-South (annual average temperature gradient), respectively, of the continental United States. All the sites were away from urban development and could be categorized into vegetation cover that included cultivated row crops, pasture, native grass, deciduous, shrub, and evergreen dominated. Both A- and C-horizon soils from each site were analyzed for total and individual hydrolysable amino acids (HAAs). Elemental, mineralogical data were described; details of soil sampling and preservation methods and results of soil characteristics are described in the USGS project report (34). The A-horizon soil samples included all textures except sandy clay, sandy clay loam, and silt and the C-horizon soil samples included all textures except sandy clay and silt (Figure S1).
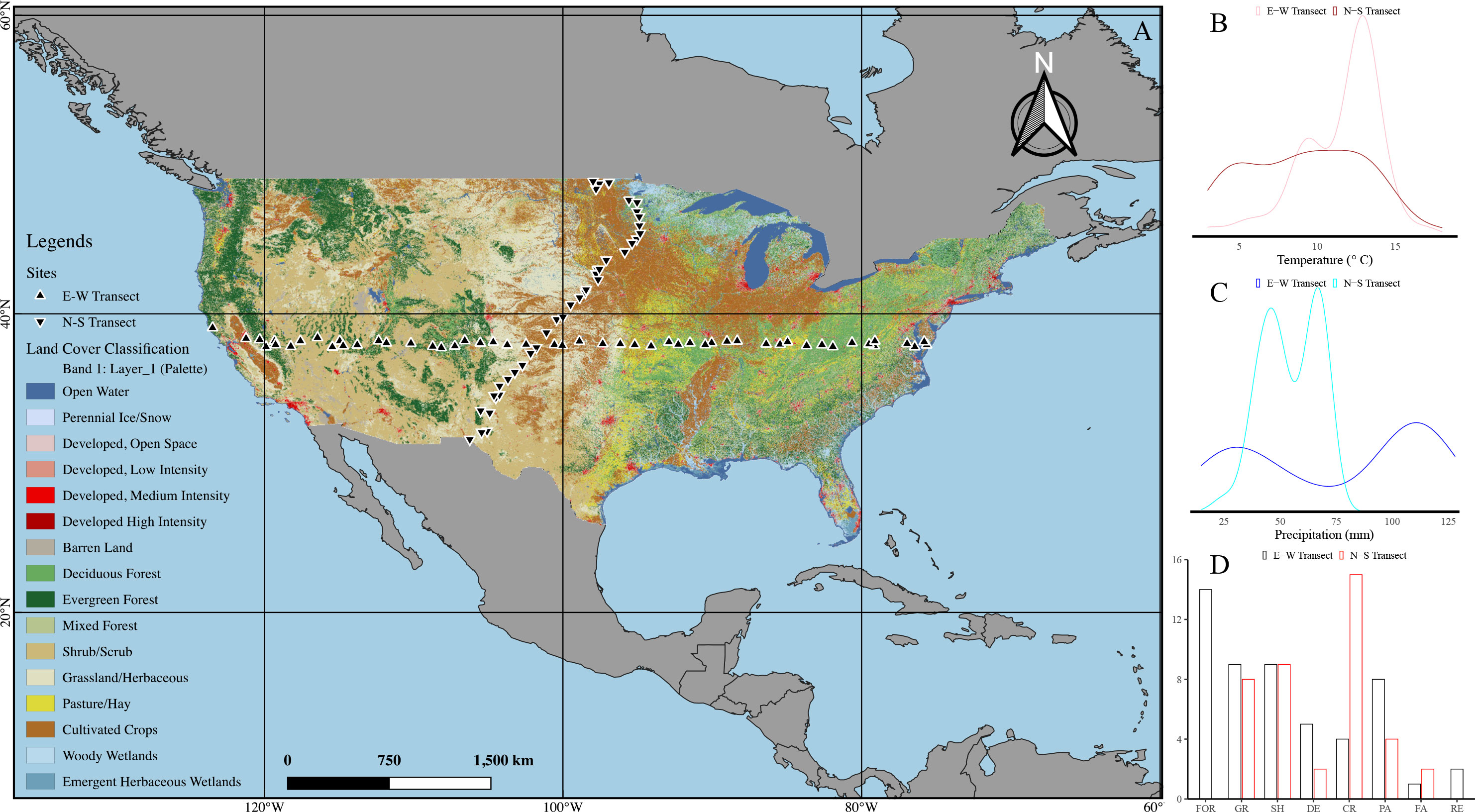
Figure 1 Location of soil sampling sites along North-South and East-West transects of continental United States. The transects are grouped into sub-continental regions (A) as shown in circles along mean temperature (B) and mean annual precipitation (C). The number of stations where data was collected well represent the vegetation types of the continental United States (D). The legend color shown at the right corresponds to the color of the circles on the maps. Black triangles represent the sampling sites. FOR, evergreen or mixed forest; GR, grassland/herbaeous; SH, shrubland; DE, decidous forest; CR, small grains or crop; PA, pasture/hay; FA, fallow; RE, low intensity residential. Map was generated using QGIS (3.2.2). Map data source: National Land Cover Database (2011) from USGS.
Sample extraction and cleanup
The method for determination of soil HAA was modified from a previous procedure (35). One gram of air-dried soil was weighed into a 12 mL glass vial. Ten mL 6 M HCl was added into the vial. An aliquot of 50 µl internal standard solution containing 1.25 µmol L-norvaline was spiked as an internal standard to the mixture. The vials were then sealed and incubated in a bead bath at 115°C for 24h to complete the acid hydrolyzation. After the hydrolysis, ~1.5 mL of cooled supernatant from each vial was transferred to a 2-mL polypropylene centrifuge tube and centrifuged at 10,000×g for 10 min. After the centrifugation, 400 µl supernatant was pipetted into a 50 mL centrifuge tube and diluted to 50 mL with ultra-pure water. The diluted solution was loaded to a polypropylene sample preparation cartridge packed with 3 g cation exchange resin (Dowex®50WX8, hydrogen form, 200-400 mesh, Sigma-Aldrich Corp., St. Louis, MO). Before packing a sample preparation cartridge, the cation exchange resin was first conditioned with 25 mL of 2 M NaOH, then neutralized with 25 mL 2 M HCl, and finally rinsed with 40 ml ultrapure water until the pH of the eluant reached ~7 (36, 37). After loading the 50 mL diluted sample hydrolyte onto the cartridge, the packed cartridge was washed first with 25 mL 0.1 M oxalic acid (pH 1.7 ± 0.1, adjusted with NH4OH), then with 5mL 0.01 M HCl, and finally with 8 mL ultrapure water. Finally, the amino acids retained on the packing material were eluted with 5 mL 3 M NH4OH for five consecutive times with each eluate combined into a collection vial. After filtering the eluant through a 0.22 µm PVDF syringe filter, 500 µL filtrate was transferred into a glass vial and completely dried down using a vacuum evaporator (SpeedVac, ThermoFisher, Pittsburgh, PA) at 60°C.
Sample derivatization
The completely dried sample residue was immediately stored at -20°C for up to 2 weeks. The dried residue was reconstituted with 10 µL 50 mM HCl followed by derivatization. The amino acids were derivatized using a reagent kit (AccQ-Tag-Fluor Reagent Kit, Waters Corporation, Milford, MA). The reagent kit was prepared using procedures provided by the manufacturer. Sixty microliter borate buffer (pH = 8-10) was added into the 10 µL reconstituted soil extract. The mixture was vortexed for 10 s followed by addition of 20 µL prepared AccQ-Tag-Fluor derivatization reagent solution. The mixture was immediately capped with a silicon-lined septum, mixed on a vortex for 15 s, and incubated at room temperature for 1 min. The mixture was then incubated at 55°C in an oven for 10 min to complete amino acid derivatization. Mixtures were analyzed using high performance liquid chromatography and a fluorescence detector (HPLC/FLD). Amino acid standards were derivatized using the same procedure as that for soil extract sample derivatization.
HPLC/FLD analysis
Derivatized samples were analyzed for amino acids on a HPLC/FLD (Agilent 1260 Infinity system, Agilent Technologies, Santa Clara, CA). Chromatographic separation of amino acids was carried out on a C18 column (X-Terra MS, 2.1 mm x 150 mm, 3.5 µm particle size, Waters Corporation, Milford, MA). The column temperature was maintained at 50°C. The mobile phase consisted of A: a solution containing 140 mM sodium acetate, 17 mM triethylamine (TEA), and 0.1% (w/v) disodium EDTA (pH 5.05, adjusted with phosphoric acid) and B: acetonitrile/water (60:40, v/v). The gradient conditions were 0-17 min 100-93% A, 17-21 min 93-90% A, 21-30 min 90-70% A, 30-35 min 70% A, 35-36 min 70-0% A, and 36-40 min 0% A. The mobile phase flow rate was 0.35 mL min-1. The sample injection volume was 5 µL. The detection was accomplished by fluorescence with excitation and emission wavelengths of 250 nm and 395 nm, respectively. Each derivatized amino acid in a sample was identified by comparing its retention time with that of the corresponding derivatized amino acid standard and quantified using the internal standard method (Figure S1).
Statistical analysis
Degradation index (DI) was calculated based on the standardized molar percentage of amino acids (38):
where vari represents molar proportation of specific amino acid i, AVGvari and SDvari represent its average and standard deviation among all samples, respectively, and coefi represents the factor coefficient as “freshness”. HAA’s contribution to organic carbon pools (%HAAC) were calculated as the ratio between carbon identified as amino acid carbon and soil organic carbon (39):
where vari represents molar proportation of specific amino acid i, ni represents carbon number in each amino acid, AC represents the standard atomic weight of carbon (12.011g mol-1). wSOC represents SOC content. Then the path analysis were conduct using R’s package lavaan (40) to predict the direct and indirect effects of climate change on the HAAs’ composition and characteristics in carbon cycle (DI and %HAAC).
Statistical analysis was performed using R (version 4.1.1), and the significance criterion was p< 0.05. Linear mixed models (LMMs) with vegetation-ecosystem types (VET)/site as nested random effect were conducted using lme4 (41) and car to determine the significance of the difference in HAAs’ molar ratio between horizons (41). Multi-dimensional statistical analysis (Permutational Multivariate Analysis of Variance, PERMANOVA; Multiple Response Permutation Procedure, MRPP) as well as sorting (Principal coordinate analysis, PCoA; Nonmetric multidimensional scaling, NMDS; Principal Component Analysis, PCA; Distance-based Redundancy Analysis, dbRDA) were performed using vegan (42) to assess the multivariate statistical effects of texture, precipitation, temperature, vegetation on HAA composition.
Results
Abundance and composition of HAAs
For the soil HAA pool, 17 amino acids were separated and identified: alanine (Ala), arginine (Arg), glycine (Gly), histidine (His), isoleucine (Ile), leucine (Leu), lysine (Lys), methionine (Met), phenylalanine (Phe), proline (Pro), serine (Ser), threonine (Thr), tyrosine (Tyr), valine (Val), cystine (as Cys-Cys), aspartic acid (Asp), and glutamic acid (Glu). During the acid hydrolysis, asparagine (Asn) and glutamine (Gln) were transformed to Asp and Glu, respectively. Hence, the sum of Asp and Asn and the sum of Glu and Gln were reported as Asx and Glx. Overall, HAA pool sizes varied greatly across locations and within horizon at specific locations. Up to 360-fold variation in the levels of total soil HAAs was observed for the A- and C-horizon soils from the 149 sites (Figures 2A, S2). For the A-horizon samples, total HAA concentrations ranged from 1.8 to 127 mmol/kg (d.w.), with 90% of the samples containing total HAAs ranged from 4.8 to 74 mmol/kg (d.w.). For the C-horizon samples, the levels of total HAAs spanned from 0.15 to 54 mmol/kg (d.w.), while 90% of the samples containing HAAs at a range of 0.47 to 17 mmol/kg (d.w.).
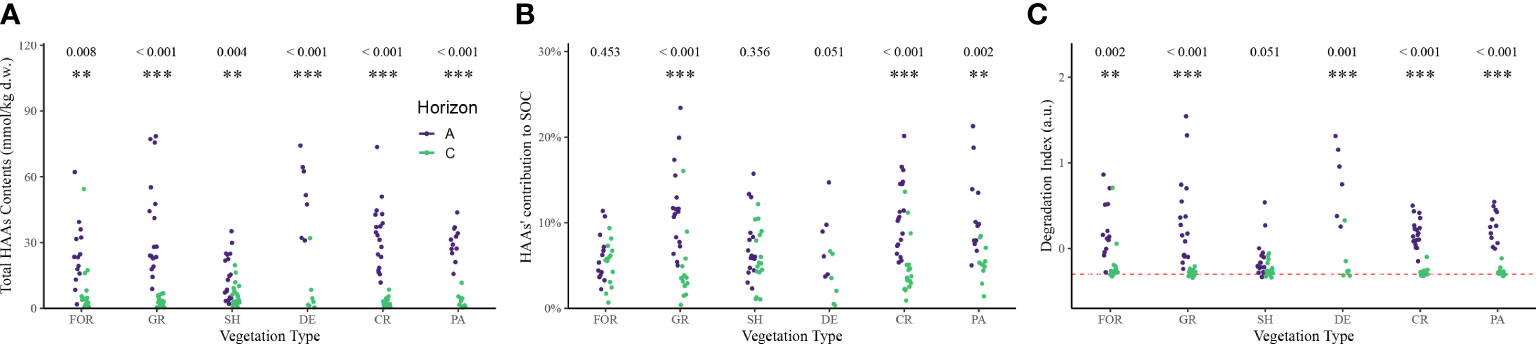
Figure 2 Significant differences in the content of HAAs (A), their contribution to organic carbon (B) and the degradation index (C) between A-horizon and C-horizon across continental United States. FOR, evergreen or mixed forest; GR, grassland/herbaeous; SH, shrubland; DE,decidous forest; CR, small grains or crop; PA, pasture/hay. Samples from fallow or low intensity residential are not shown here due to the small sample size. Different colors represent different soil horizons. The numbers and marks at the top represent the results and significance of the t-test. Red dashed line in the C-subfigure is the reference line indicating the stability of DI in the C-horizon. **0.001< p <0.01; ***p<0.001.
Nearly 99% of the sites had higher levels of total HAAs in the A-horizon than in the C-horizon (Figure 2A). The A- to C-horizon concentration ratios for total HAAs were in the range of 1-10 for ~60% of the sites. For about 30% of the sites, the A- to C-horizon concentration ratios were in the range of 10-50. Close to 10% of the sites had A- to C-horizon concentration ratios that were > 50. We speculate that vegetation characteristics played an important role in determining the HAA ratio between A- and C- horizon. The dominant vegetation on the few sites with A- to C-horizon concentration ratios< 1 were shrubs, while grass and forest were the dominant vegetation on the sites with A-to C- horizon concentration ratios that are > 100. A similar A- to C-horizon concentration ratio distribution pattern was found for soil organic C of the sites investigated, indicating a close relationship between soil organic C and soil amino acids, which could be confirmed by the close correlation between total HAAs and soil organic C (Figure S3, F1,182 = 130.2, p< 0.001). The ratio of non-polar: polar/negative charged AA was also positively associated with SOC (Figure S5, F1,182 = 22.2, p< 0.001). HAAs directly contributed 1.05-23.4% (9.17 ± 4.62%) of the SOC in A horizon and 0.36-16.0% (4.88 ± 3.06%) of the SOC in C horizon (Figure 2B). The %HAAC (C, carbon) was significantly higher in the A horizon (t = 7.259, p< 0.001), which might suggest selective degradation. For different vegetation-ecosystem types (VET), significantly higher %HAAC was observed in grassland, cropland, and pasture relative to forest and shrubland.
The top ten dominant amino acids detected were: Gly > Asx > Ala > Glx > Ser > Thr > Pro > Lys > Val > Leu (Figures S4, S7). However, in spite of these trends in average composition, there was considerable variation between observations. For example, Asx ranged from 8.6 to 27.4, and Gly from 7.2-25.9 mol%. In both A- and C-horizon soils, 53-65% of the HAAs are neutral amino acids, while acidic, basic, and aromatic amino acids consist of 24-36%, 6-10%, and 4-5% of HAAs, respectively. The overall composition of HAAs was different between the A- and C- horizons (Figure 3, S3; MRPP, p< 0.0001). For example, the mol% of Asx and Ser were 21% more and 40% less in the A compared to the C horizon, respectively (Figure S4).
In this study, we calculated a degradation index (DI), an indicator of the degree of amino acid transformation, based on the weighted molar ratio of HAAs to reflect the reactivity of SON (38). As shown in Figure 2C, DI ranged from -0.34 to 1.54, with its value higher in horizon A (t = 10.4, p< 0.001), meaning that the SON is more accessible and regulated by organisms than in the C horizon. However, we did not find significant difference between DI between horizons in shrubland where the DI in horizon A is much lower than other VETs. Interestingly, we found that regardless of the remarkable difference in the DI in the A horizon, the DI in the C horizon tended to approach a relatively stable value (red dashed line in Figure 2C, ~ -0.3). In the C horizon, this could be the result of extensive degradation of relatively old organic inputs. In the A horizon this may suggest selective utilization during early decomposition helps to shape the composition of HAAs across plant types.
The co-distribution of HAAs was also demonstrated by the co-occurrence network (Figure 3). The coupling associations between HAAs in the A horizon were greater and richer than that in the C horizon. The HAAs’ co-occurrence network of the A horizon has more edges as well as higher clustering coefficient, betweenness centralization, and degree centralization. Regardless of the path strength, a similar Leu-Phe-Ile-Gly co-occurrence structure was found in both soil horizons.
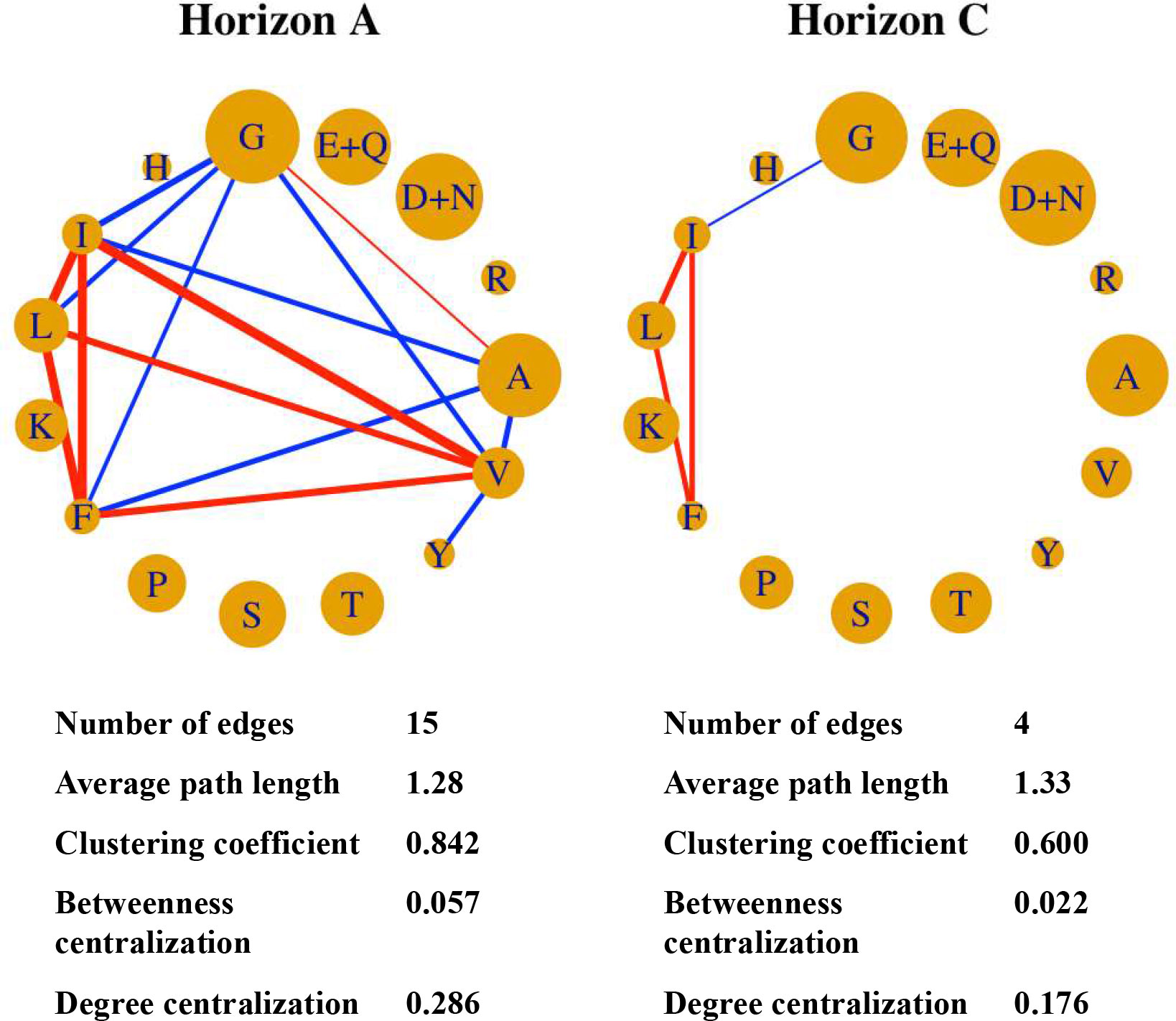
Figure 3 HAAs in soils from A-horizon have a stronger coupling distribution compared to those from C-horizon, supported by the network analysis. The single letters in blue represent the corresponding amino acids. Connected lines represent rubust correlations (r > 0.6, p < 0.05). Red and blue lines represent positive and negative correlations. The thickness of the line represents the absolute value of the correlation coefficient. A, Ala; R, Arg; G, Gly; H, His; I, Ile; L, Leu; K, Lys; M, Met; F, Phe; P, Pro; S, Ser; T, Thr; Y, Tyr; V, Val; D, Asp; N, Asn; E, Glu; Q, Gln.
AA signatures of vegetation-ecosystem type associated with soil horizon
Overall, the composition of HAAs exhibited a similar coupling pattern used to differentiate A and C horizon (Figure S8). To show the distribution pattern of HAAs, principal co-ordinates analysis (PCoA) based on Bray-Curtis distance was applied on the 15 most abundant amino acids (Figure S7). The first two PCoA axes explained 33.9% and 17.3% of the variance (Figure 4A), and revealed that HAAs in the C horizon exhibited a wider range of distributions compared to the ones in the A horizon. This could be partly related to selective utilization with greater microbial activity in surface soil. VET explained more variance of HAAs’ composition in A horizon (PERMANOVA, r2 = 0.18, pseudo F5,74 = 3.26, p< 0.001; MRPP, p< 0.001) than that in C horizon (PERMANOVA, r2 = 0.10, pseudo F5,70 = 1.48, p = 0.057; MRPP, p = 0.018). This was consistent with the results of both NMDS (Figure S12) and PCA (Figure S13).
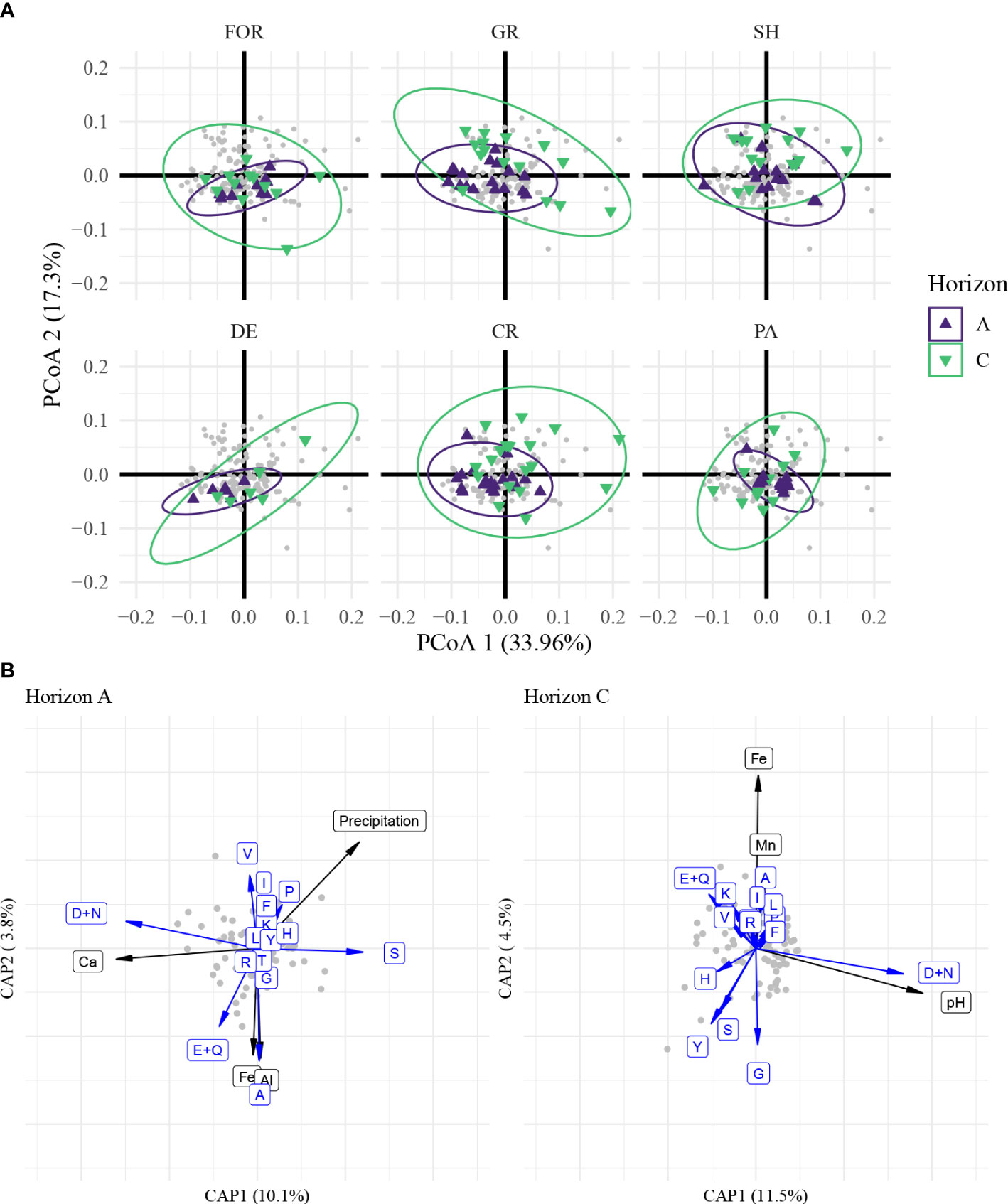
Figure 4 Molecular diversity and environmental factor changes under the influence of selective decomposition of HAAs during pedogenesis, revealed by multidimensional sorting. (A) PCoA-based sorting on the molar ratio of HAAs. Samples from different vegetation types were exhibited separately. Colors represent different soil layers. FOR, evergreen or mixed forest; GR, grassland/herbaceous; SH, shrubland; DE, deciduous forest; CR, small grains or crop; PA, pasture/hay. Samples from fallow or low intensity residential are not shown here due to the small sample size. (B) Driving factors of HAAs in different soil layers exhibited by dbRDA. A-horizonal and C-horizonal samples were fitted separately to compare the changes in environmental factors. The single letters in blue represent the corresponding amino acids. A, Ala; R, Arg; G, Gly; H, His; I, Ile; L, Leu; K, Lys; M, Met; F, Phe; P, Pro; S, Ser; T, Thr; Y, Tyr; V, Val; D, Asp; N, Asn; E, Glu; Q, Gln. Black labels represent specific environmental factors. Data were transformed by log1p (HAAs) or log (environmental factors).
PCoA1 was positively correlated with Gly (r = 0.79) but negatively correlated with Ile (r = -0.83), Leu (r = -0.77), Val (r = -0.77), Phe (r = -0.69), and Pro (r = -0.62). Among these HAAs, Ile, Leu, Phe and Pro differed significantly between horizons, while the effect of VET was not significant (Table S1). These HAAs, furthermore, were all found to be relatively enriched in the A horizon (Figure S5). The interaction term between VET and horizon was significant in describing the distribution of the relative molar ratios of Ile, Leu, and Phe. In the A horizon, Ile, and Phe had greater mol % in evergreen or mixed forest, grassland, and shrubland compared to that of pasture/hay. Leu was higher in evergreen or mixed forest but lower in pasture/hay. The effect of VET, however, did not have a significant effect on differences in the C horizon. PCoA2 was highly described by variation in Asx with an extremely strong positive correlation (r = 0.94). Both horizon and VET significantly influenced the pattern of Asx (Table S1), which was relatively enriched in the C compared to A horizon; and also, in cropland and pasture/hay, while depleted in the evergreen and mixed forests.
The driver of HAAs pool – precipitation, pH, and metal composition
As shown above, VET explained a portion of the spatial pattern of HAAs (Table S2, PERMANOVA, A horizon: r2 = 0.18, pseudo F5,74 = 3.26, p< 0.001, C horizon: r2 = 0.10, pseudo F5,70 = 1.48, p = 0.057). To better understand the effect of VET and that of environmental factors, a dbRDA was conducted to evaluate the correlation of environmental parameters in explaining the overall variation in HAAs’ molar distribution. The first two dbRDA axes (CAP1 & 2) together explained 13.9% in the A horizon and 16.0% in the C horizon (Figure 4B).
The Akaike information criterion (AIC)-based variable selection exhibits different environmental factors affecting the molar distribution of HAAs in both horizons. Precipitation and the content of Ca, Fe, and Al were major environmental factors that had a significant though small effect on the HAA distribution in the A horizon, while there was similarly, a significant association between HAAs’ molar distribution in the C horizon with that of pH and the content of Fe and Mn. The species of HAAs that responded to these environmental factors also differed between horizons. Glx, Asx, and Ala were sensitive to environmental factors in both horizons. Among them, Asx mainly responded to higher pH and Ca content, while Glx and Ala were enriched and may be related to selective protection provided by Fe-related minerals. Val and Ser were more sensitive to environmental responses in the A horizon, while Tyr and Gly might be characteristic of HAAs in the C horizon. By taking environmental factors into account using the dbRDA model, the interpretation of the HAAs’ pattern was greatly enhanced (Table S2). Overall, the relative effect of Fe and Al on HAA molar distribution was smaller than expected, however.
To model the direct and indirect effects of precipitation on HAAs, we used path analysis to evaluate the interplay between environmental factors affecting the HAAs’ composition and characteristics in the carbon storage (Figure 5). Four HAAs (i.e., Ala, Ser, Glx, and Asx) were chosen because of their higher correlation with environmental factors (Figures S15-S17). The fitting of the path models was performed separately in horizon A and C to compare the differences in the effects of the controlling factors. Both path models in different horizons indicated an excellent explanation on the molar ratio of these four HAAs (Figure 5A, horizon A: χ2 = 2.014, p = 0.847, RMSEA = 0.000, CFI = 1.000; horizon C: χ2 = 3.562, p = 0.614, RMSEA = 0.000, CFI = 1.000). Consistent with our previous results, the effect of precipitation and other derived environmental factors on HAA is complex and strong in horizon A; however, the influence of precipitation was weakened in horizon C where HAA was more controlled by pH. Our path model also showed a good prediction on the HAA’s characteristics in the carbon cycle (Figure 5B, horizon A: χ2 = 2.783, p = 0.426, RMSEA = 0.000, CFI = 1.000; horizon C: χ2 = 1.242, p = 0.743, RMSEA = 0.000, CFI = 1.000). Positive associations between DI and HAA’s contribution to organic carbon were confirmed by their path coefficients in both horizons (A horizon: 0.56; C horizon: 0.44). Moreover, organic carbon was influenced by precipitation and recognized as a moderating variable in horizon A, while in horizon C, precipitation had slight effect on organic carbon, while the latter became an independent and influential variable for DI on HAA’s contribution to organic carbon. The distribution of annual mean temperature and precipitation and a representative chromatogram are shown in Figures S18, S19.
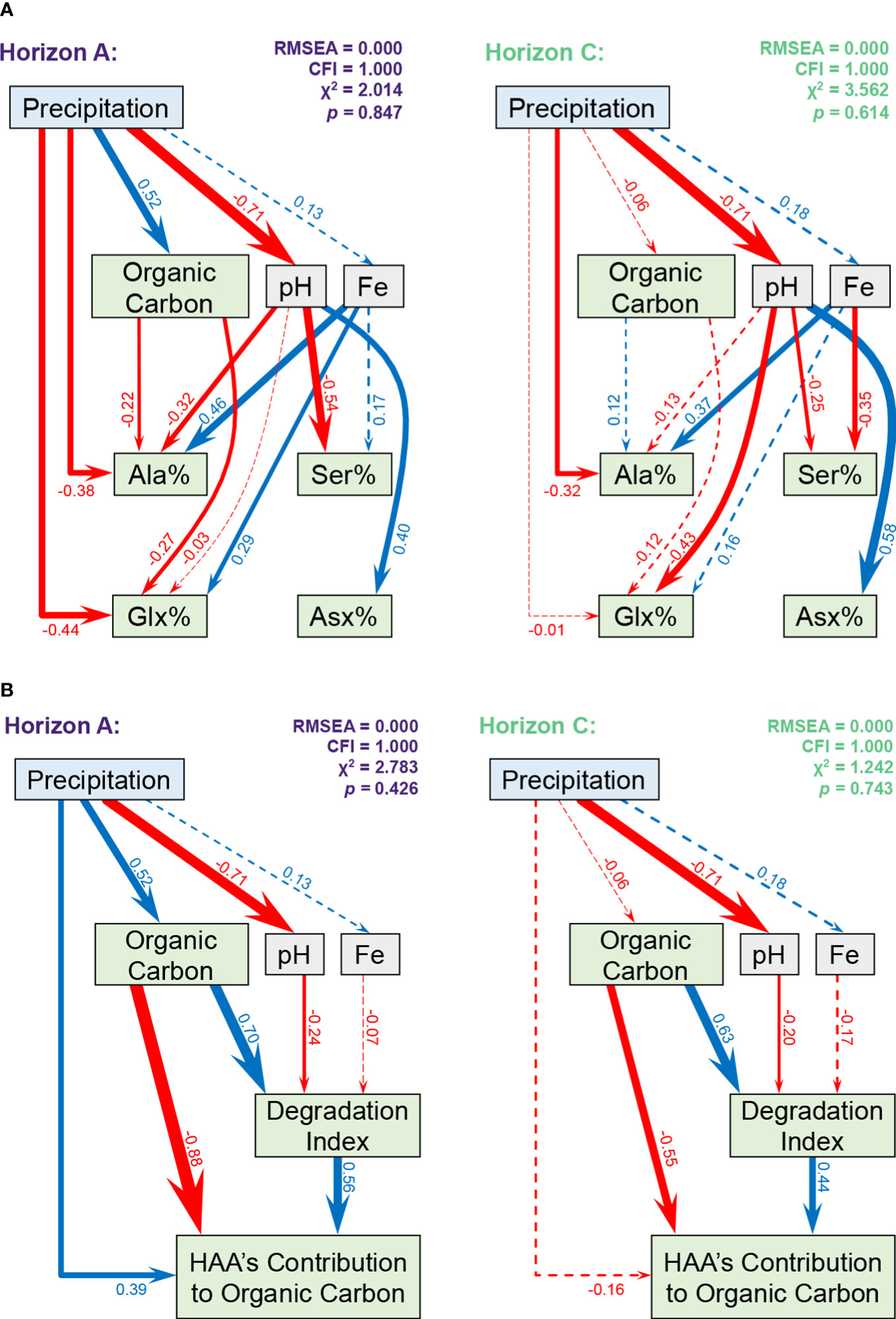
Figure 5 Model describing precipitation, but not temperature, as a proximate and distal mechanism controlling soil HAA composition Path diagrams comparing the direct and indirect effects of precipitation on (A) HAA composition and (B) HAA’s contribution to organic carbon pool in horizon A (n = 84) and horizon C (n = 81). Red and blue paths represent the negative and positive relationships, respectively. Solid and dashed paths indicate significant and insignificant relationships, respectively. The paths’ thickness is scaled based on the standardized path coefficients as shown adjacent to the arrows.
Discussion
To our knowledge, this is the first comprehensive molecular-based survey of a major soil organic N-C pool at a continental scale in two major soil horizons. We looked at three geographical gradients (temperature: N-S profile; precipitation: E-W profile; depth: A-C horizons) using a continental, systematic analysis. The results provide a novel view of how critical factors like vegetation-ecosystem type (VET) and pH impact the spatial pattern of HAAs in soil, which are likely to be important in determining the composition of the soil active organic N pool (43); and with important ramifications on carbon cycle (44). Surprisingly, the hypothesis that temperature would be a strong determinant of mol% HAA was not supported. The hypothesis, in contrast, that precipitation, pH, Fe, Mn, Al and Ca were associated with changes in the mol% HAA (hereafter referred to as composition), and thus potentially affect the chemistry, cycling, and persistence of both N and C, was supported. It is straightforward to see how VET interacts with precipitation and the effects of weathering that alter soil pH and Al content as determinants of HAA, but it is not possible to disentangle these interactions. The chemistry of minerals has long been considered a major force in determining OM composition, and the data herein show that there is room for further investigation into this observation. Some of the strongest effects were due to VET, precipitation, and pH. Because precipitation is a distal factor that influences VET and pH, it is likely a dominant driver of AA composition. These results provide new information that can be used to better describe the molecular mechanisms of proteinaceous OM cycling and accrual, as well as support regional scale models that include C-N cycling.
The continental-wide pattern of amino acids across soil horizon
Our result indicates a strong correlation between total HAAs and SOC, with a ratio of the former to the latter of 1.3 mol/kg, which means that HAAs are important constituents of SOC and SON. The close positive relationships between soil organic C and HAAs, similar to previous observations (29) from ten Iowa surface soils, are likely the result of this pool being a large part of soil OM (29, 45). The presenting work involves continental-wide observations with 10.7 and 61.7% of total soil C and N, (~1 to 80 mol g-1 soil) accounted for by HAAs, and thus are a large OM pool that can provide insight into the processes of soil pedogenesis and N-C cycling. The large variability in HAAs in the A horizon (Figure 2A) was also consistent with previous reports from native prairie vegetation, deciduous forests and sub-alpine grassland soils (45, 46), and supports the idea that the C and N cycles are tightly coupled.
In line with this study, a separate recent study of soils with different development ages observed that His, Lys, and Arg tended to be more dominant in mineral compared to that of the whole soil organic pools. This points to their possible role as persistent or long-lived OM (1, 19), perhaps most important in soils with high CEC. Non-polar HAAs (e.g., Pro, Leu, Ile) in our study in contrast, were most positively correlated with total soil organic C content (Figure S7), suggesting that these residues may accumulate to increase SOM. Non-polar HAAs, however, declined with pedogenesis in two ecosystems suggesting that they may be part of newer pools of accumulating rather than older pools of decomposed OM in soil (19).
HAAs have a broad range of chemical characteristics that can be used to assess molecular interactions and interpreted to understand SOM formation and turnover. However, most of the variability in HAA’s are not described by our environmental factors. We have measured some of the primary environmental drivers and soil properties thought to be chemically, physically, and biologically relevant, but the heterogeneity of soils make it difficult to clearly discern trends that explain most of the data. Many of the AA are also likely linked together into polypeptides by amide bonds, that along with amino acids react with surfaces and each other (1, 19), which can further complicate interpretations of how HAA may vary in soils.
The overall HAAs dominant in A-horizon and their compositional profile reported in the present study (Figures 2A, S3, S5) are coincident with those previously reported (1, 27, 30, 46–48). In general, many biologically dominant AA remain dominant in soil, but the broad descriptions of relative amino acids found in soil herein, describe how the mol% composition of AA can vary considerably across soil gradients, for example, by 2X for AA such as Asx, 6X for AA such as proline, and from non-detectable up to ~2-3 mol% for Met and Phe. These AAs, on the other hand, may be good indicators of environmental changes. For example, Asx was positively related to soil pH in both the A and C horizons (Figures S15 and S16). Soil pH was found to be one of the strongest drivers of HAA composition, and this relationship is important both biologically and chemically.
The ratio of non-polar: negatively charged/neutral polar AA was positively associated with SOC (Figure S5, F1,182 = 22.2, p< 0.001), perhaps indicating the potential for non-polar SOC interactions separate from those of mineral-associated OM (Moon et al., 2019) (19). This still leaves considerable room for describing mechanisms and rules that describe variation in SOM formation at a continental scale. Compared to carbon, nitrogen is relatively well conserved to cycling within the soil with lower mass fluxes to the atmosphere (49). The well-known coupling of C-N cycles (50), and the knowledge that AA’s dominate total SON pools, the relevance that amino acid composition plays in both organic C-N cycling and storage in soil deserve further description (45).
The roles taken by surface vegetation on HAAs’ composition
The percentage of soil organic N as AAs has been observed to decrease with soil depth (18, 51), and this pattern was broadly true in the current study (Figure 2B). Surface SOM ranges from fresh plant litter to extensively decomposed SOM (52). In contrast, energy-rich plant materials tend to be depleted in the subsoil, and if they are present, they tend to be highly processed (53). The high level of processing is borne out by differences in DI, where lower values indicate more processed, and likely older OM in the subsurface.
Above-ground plant litter, dead roots, and the turnover of these OMs processed by the decomposer community, contributed to most of the HAAs in the soil (3). Acidic AA such as Asp are common constituents of fresh plant tissue, root tissue and root exudates (54–56); and positively charged amino acids such as Lys and His in free forms and enriched in peptides may bind to negative mineral and organic surfaces to support their relative enrichment (Figure S5) in the C horizons.
Ser was relatively more abundant on the surface compared to the subsurface soil (Figure S5, LMMs, F1,90.1 = 5.90, p = 0.017), which might be related to the presence of Ser-rich microbes, such as methanotrophs and archaea in the surface. Ser is a versatile AA highly utilized in metabolic pathways and important to the formation of nucleic acids, which are likely to be relatively more common because of higher microbial activity (especially fungi) and turnover in the A compared to the C horizon. Ser-rich microbes, such as methanotrophs and archaea on the surface may also contribute to Ser in soil. It has been observed that Ser was relatively greater within the mineral-associated rather than the organic-associated soil pools. (1, 19), which would tend to be lower in the A relative to the C horizon. Hence, Ser content may be an indicator of less processed OM. This otherwise relatively polar and soluble amino acid would likely be susceptible to decomposition.
The HAAs’ composition was described by VET, and thus indicated the potential importance of its influence on HAAs’ biogeochemical processes. The lower variability on the A horizon compared to the C horizon HAAs (Figures 2, 4) may result from the stronger effect of vegetation in the surface providing a stable input of HAAs’ and microbial activity to stabilize HAA’s into the A- horizons. This, furthermore, may be the result of more rhizosphere activity, increasing the direct above- and below-ground inputs. The process of weathering itself may also help to homogenize the surface compared to the subsurface. However, abiotic parameters became a dominant driver during horizon development with less influence from plants, which may have led to increased variability in the C- horizon. Once soil HAAs derived from plant and microbial residues (57) become persistent SOM, they will be expected to undergo more consistent processing and cycling on the surface compared to the subsurface.
While its direct influence is weakened in the deeper soil, VET would likely show an effect that interacts with a changing environmental factor like pH, and mineral contents that could be exacerbated over longer periods of horizon development. Its effect on the C horizon may be underestimated given that not all sampling locations had land use (> 20 years) that would have been consistent before sampling. This variation due to legacy effects of past land use could cause the greater variability in AA composition in the C-horizon than the A-horizon soil (Figure 4A). The lower correlation between VET and HAA composition in the C relative to the A-horizon may also simply be related to the fact that the contribution of microbial-derived organic matter increases with depth.
Direct and indirect drivers of the accrual of amino acids
HAAs did show considerable variation across the soil landscape and horizon that was useful for describing their patterns associated with pH, Al, and Ca. Because of the numerous interacting factors the patterns associated with several different possible drivers of AA composition (e.g., VET, Ca, and pH), it could be difficult to identify their effect from those of metals on soil proteinaceous amino acid composition. These support the importance that VET, pH, Al, Fe, and Ca play as factors, which are likely further controlled by the distal factor, precipitation, as contributors to organic soil N accrual.
Soil pH has a strong influence on the chemistry of both the soil substrate and the HAAs, which interact to affect the soil properties, including Al, Fe and mineralogy with HAA. Our previous study found depletion of mineral-associated Glx and Asx and increasing basic amino acids (His, Lys) with increasing soil development up to 6500y (1), after which continued weathering and loss of cation exchange capacity may favor the accumulation of negative AA. Both Lys and His are basic amino acids that possess positive charges at typical environmental pH ranges. Their strong sorption on negatively charged soil minerals, which is driven by electrostatic attraction (58), may retard their biodegradation in soils (59, 60) and, therefore, result in their enrichment in the HAA pools.
It is notable that concentrations of mineral metals like Fe, Mn, Al, Ca in soil were shown to correlate with shifts in AA composition, but perhaps less than expected. Fe content having been shown to be an important binding and stabilizing mechanism for soil organics (53), is further displayed by differences in A and C horizons, where the mineral content is also one critical driver dominating the composition of HAAs’. Al hydroxides may sorb proteins and amino acids, and could have preferential affinity for some types of peptides and amino acids than others. These results support the role that metal cations have on SOM stabilization (53).
Most of the direct and indirect associations between precipitation and the HAAs’ composition showed to be more pronounced in the surface layer (Figure 5), again supporting the role of precipitation in regulating HAA in the surface horizon. Although precipitation may also contain some HAAs (61) the pool is small, and we found that the precipitation showed negative or non-significant direct effects on representative HAAs’ molar ratio in both horizons. But there was a complex effect of precipitation on the HAA’s composition. Precipitation lowered the soil pH, thus increasing Ala and Ser in the A horizon and Glx and Ser in the C horizon. Moreover, iron was also an influential factor of HAAs independent of precipitation, especially on the molar ratio of Ala and Ser. For instance (Figure 5B), as the %HAAC decreased, there was an increased association of this OM via DI, suggesting that HAA may be good indicators of degradation. Soils with less carbon but relatively similar amounts of carbon input, such as that associated with increasing temperature from north to south, would be expected to have greater relative degradation and OM processing.
It is important to note that precipitation but not temperature, among climate factors, was found to play a major role in determining the HAAs’ characteristics in this study, as some soil warming experiments have shown (62). This does not mean that the role of temperatures can be ignored. Perhaps temperature interacts in a complex way with precipitation and soil minerals. The temperature may also influence the long-term succession of surface vegetation and thus the composition of HAAs. Also, we note that even after accounting for VET and environmental factors, there is still about 70% of the variance in HAAs that is not explained, which suggests that there are deeper mechanisms involved in discerning the pattern of HAA. This outcome indicates a need for more mechanistic and comparative studies across ecosystems and even continents.
Microbial activity plays a crucial role in the decomposition of plant inputs (VET) and the formation of recalcitrant substances (63, 64). Soil peptides/proteins, through turnover by microorganisms (18) and preservation in soil (14, 15), are a major form of both bioavailable and sequestered OM. Once formed, these microbial-derived OMs will become more stable by binding further to minerals (65) or tannins (21, 22). Some other biotic and abiotic factors, including reactive nitrogen deposition (66), past legacies of activity, and extreme events (10), need to be included for consideration in subsequent studies to quantify their relative importance determining the role proteinaceous amino acids play in soil OM formation.
In conclusion, to the best of our knowledge, this is the first comprehensive study reporting the levels and compositional profiles of hydrolyzable proteinaceous amino acids in a wide range of surface and subsurface soils at a continental scale. The results suggested that pH, mineral metals, precipitation and VET were the best predictors describing soil AA composition in both the A and C horizons. The soil pH may be important to consider as a major determinant of OM persistence and turnover because of its capacity to alter both the organic chemistry and soil organo-mineral phases. Studies within ecosystem types may also shed light on ecology specific contexts that determine HAA and SOM distributions. For example, non-polar AA were associated with greater levels of carbon, and with forested ecosystems. These relationships may be a result of the type of OM input or the way forests and precipitation drive ecosystem development. The patterns of AA in VET were relatively strong even in the subsurface, suggesting that it and associated precipitation or distal environmental changes related to root weathering of the subsurface (e.g., pH) can have direct impacts on SOM. It also seems plausible that the strong role that pH plays in determining bacterial communities may partly determine HAA patterns. With increasing awareness of the essential contribution of subsurface C and N to terrestrial OM biogeochemical cycling (53, 67), the large surface and subsurface organic N data set that was generated in this study is timely and valuable for incorporation into future dynamic C and N models.
Data availability statement
The original contributions presented in the study are included in the article/Supplementary Material. Further inquiries can be directed to the corresponding author.
Author contributions
HH: Formal analysis, Visualization, Writing - Original Draft. LM: Investigation, Writing - Original Draft. DS: Methodology, Supervision. HL: Resources, Supervision. CY: Resources, Supervision. KX: Conceptualization, Investigation, Methodology, Resources, Supervision, Writing - Review & Editing. MW: Conceptualization, Funding acquisition, Investigation, Methodology, Data Curation, Supervision, Writing - Review & Editing. All authors contributed to the article and approved the submitted version.
Funding
This work was supported by USDA-NIFA (award # 2012-67019-30227), the College of Agricultural Life Sciences at Virginia Tech, and the Virginia Tech Library Subvention Fund.
Conflict of interest
The authors declare that the research was conducted in the absence of any commercial or financial relationships that could be construed as a potential conflict of interest.
Publisher’s note
All claims expressed in this article are solely those of the authors and do not necessarily represent those of their affiliated organizations, or those of the publisher, the editors and the reviewers. Any product that may be evaluated in this article, or claim that may be made by its manufacturer, is not guaranteed or endorsed by the publisher.
Supplementary material
The Supplementary Material for this article can be found online at: https://www.frontiersin.org/articles/10.3389/fsoil.2022.984927/full#supplementary-material
References
1. Moon J, Ma L, Xia K, Williams MA. Plant – microbial and mineral contributions to amino acid and protein organic matter accumulation during 4000 years of pedogenesis. Soil Biol Biochem (2016) 100:42–50. doi: 10.1016/j.soilbio.2016.05.011
2. Canarini A, Schmidt H, Fuchslueger L, Martin V, Herbold CW, Zezula D, et al. Ecological memory of recurrent drought modifies soil processes via changes in soil microbial community. Nat Commun (2021) 12:5308. doi: 10.1038/s41467-021-25675-4
3. Jones DL, Kielland K. Soil amino acid turnover dominates the nitrogen flux in permafrost-dominated taiga forest soils. Soil Biol Biochem (2002) 34:209–19. doi: 10.1016/S0038-0717(01)00175-4
4. Adamczyk B, Sietiö O-M, Straková P, Prommer J, Wild B, Hagner M, et al. Plant roots increase both decomposition and stable organic matter formation in boreal forest soil. Nat Commun (2019) 10:3982. doi: 10.1038/s41467-019-11993-1
5. Craig ME, Geyer KM, Beidler KV, Brzostek ER, Frey SD, Stuart Grandy A, et al. Fast-decaying plant litter enhances soil carbon in temperate forests but not through microbial physiological traits. Nat Commun (2022) 13:1229. doi: 10.1038/s41467-022-28715-9
6. Wershaw RL. A new model for humic materials and their interactions with hydrophobic organic chemicals in soil-water or sediment-water systems. J Contam Hydrol (1986) 1:29–45. doi: 10.1016/0169-7722(86)90005-7
7. Sollins P, Homann P, Caldwell BA. Stabilization and destabilization of soil organic matter: Mechanisms and controls. Geoderma (1996) 74:65–105. doi: 10.1016/S0016-7061(96)00036-5
8. Knicker H, Hatcher PG. Survival of protein in an organic-rich sediment: Possible protection by encapsulation in organic matter. Naturwissenschaften (1997) 84:231–4. doi: 10.1007/s001140050384
9. Knicker H. Soil organic n - an under-rated player for c sequestration in soils? Soil Biol Biochem (2011) 43:1118–29. doi: 10.1016/j.soilbio.2011.02.020
10. Maxwell TL, Canarini A, Bogdanovic I, Böckle T, Martin V, Noll L, et al. Contrasting drivers of belowground nitrogen cycling in a montane grassland exposed to a multifactorial global change experiment with elevated CO 2 , warming, and drought. Glob Change Biol (2022) 28:2425–41. doi: 10.1111/gcb.16035
11. Zhang K, Li M, Yan Z, Li M, Kang E, Yan L, et al. Changes in precipitation regime lead to acceleration of the n cycle and dramatic N2O emission. Sci Total Environ (2022) 808:152140. doi: 10.1016/j.scitotenv.2021.152140
12. Wang Q, Hong H, Liao R, Yuan B, Li H, Lu H, et al. Glomalin-related soil protein: The particle aggregation mechanism and its insight into coastal environment improvement. Ecotoxicol Environ Saf (2021) 227:112940. doi: 10.1016/j.ecoenv.2021.112940
13. Schlüter S, Leuther F, Albrecht L, Hoeschen C, Kilian R, Surey R, et al. Microscale carbon distribution around pores and particulate organic matter varies with soil moisture regime. Nat Commun (2022) 13:2098. doi: 10.1038/s41467-022-29605-w
14. Mikutta R, Kleber M, Torn MS, Jahn R. Stabilization of soil organic matter: Association with minerals or chemical recalcitrance? Biogeochemistry (2006) 77:25–56. doi: 10.1007/s10533-005-0712-6
15. Peng X, Yan X, Zhou H, Zhang YZ, Sun H. Assessing the contributions of sesquioxides and soil organic matter to aggregation in an ultisol under long-term fertilization. Soil Tillage Res (2015) 146:89–98. doi: 10.1016/j.still.2014.04.003
16. Mikutta R, Kaiser K, Dörr N, Vollmer A, Chadwick OA, Chorover J, et al. Mineralogical impact on organic nitrogen across a long-term soil chronosequence (0.3-4100 kyr). Geochim Cosmochim Acta (2010) 74:2142–64. doi: 10.1016/j.gca.2010.01.006
17. Dümig A, Häusler W, Steffens M, Kögel-Knabner I. Clay fractions from a soil chronosequence after glacier retreat reveal the initial evolution of organo-mineral associations. Geochim Cosmochim Acta (2012) 85:1–18. doi: 10.1016/j.gca.2012.01.046
18. Hobara S, Osono T, Hirose D, Noro K, Hirota M, Benner R. The roles of microorganisms in litter decomposition and soil formation. Biogeochemistry (2014) 118:471–86. doi: 10.1007/s10533-013-9912-7
19. Moon J, Xia K, Williams MA. Consistent proteinaceous organic matter partitioning into mineral and organic soil fractions during pedogenesis in diverse ecosystems. Biogeochemistry (2019) 142:117–35. doi: 10.1007/s10533-018-0523-1
20. Torn MS, Trumbore SE, Chadwick OA, Vitousek PM, Hendricks DM. Mineral control of soil organic carbon storage and turnover. Nature (1997) 389:170–3. doi: 10.1038/38260
21. Adamczyk B. How do boreal forest soils store carbon? BioEssays (2021) 43:2100010. doi: 10.1002/bies.202100010
22. Adamczyk B, Sietiö O, Biasi C, Heinonsalo J. Interaction between tannins and fungal necromass stabilizes fungal residues in boreal forest soils. N Phytol (2019) 223:16–21. doi: 10.1111/nph.15729
23. Nordhaus I, Salewski T, Jennerjahn TC. Interspecific variations in mangrove leaf litter decomposition are related to labile nitrogenous compounds. Estuar Coast Shelf Sci (2017) 192:137–48. doi: 10.1016/j.ecss.2017.04.029
24. Müller K, Marhan S, Kandeler E, Poll C. Carbon flow from litter through soil microorganisms: From incorporation rates to mean residence times in bacteria and fungi. Soil Biol Biochem (2017) 115:187–96. doi: 10.1016/j.soilbio.2017.08.017
25. Lindedam J, Magid J, Poulsen P, Luxhøi J. Tissue architecture and soil fertility controls on decomposer communities and decomposition of roots. Soil Biol Biochem (2009) 41:1040–9. doi: 10.1016/j.soilbio.2009.01.023
26. Drigo B, van Veen JA, Kowalchuk GA. Specific rhizosphere bacterial and fungal groups respond differently to elevated atmospheric CO2. ISME J (2009) 3:1204–17. doi: 10.1038/ismej.2009.65
27. Gotoh S, Araragi M, Koga H, Ono Si, Gotoh S. Hydrolyzable organic forms of nitrogen in some rice soil profiles as affected by organic matter application. Soil Sci Plant Nutr (1986) 32:535–50. doi: 10.1080/00380768.1986.10557536
28. Campbell CA, Schnitzer M, Lafond GP, Zentner RP, Knipfel JE. Thirty-year crop rotations and management practices effect on soil and amino nitrogen. Soil Sci Soc Am J (1991) 55:739–45. doi: 10.2136/sssaj1991.03615995005500030017x
29. Senwo ZN, Tabatabai MA. Amino acid composition of soil organic matter. Biol Fertil Soils (1998) 26:235–42. doi: 10.1007/s003740050373
30. Friedel JK, Scheller E. Composition of hydrolysable amino acids in soil organic matter and soil microbial biomass. Soil Biol Biochem (2002) 34:315–25. doi: 10.1016/S0038-0717(01)00185-7
31. Sollins P, Swanston C, Kramer M. Stabilization and destabilization of soil organic matter - a new focus. Biogeochemistry (2007) 85:1–7. doi: 10.1007/s10533-007-9099-x
32. Knops JMH, Bradley KL. Soil carbon and nitrogen accumulation and vertical distribution across a 74-year chronosequence. Soil Sci Soc Am J (2009) 73:2096–104. doi: 10.2136/sssaj2009.0058
33. Jobbágy EG, Jackson RB. The vertical distribution of soil organic carbon and its relation to climate and vegetation. Ecol Appl (2000) 10:423–36. doi: 10.1890/1051-0761(2000)010[0423:TVDOSO]2.0.CO;2
34. Smith DB, Cannon WF, Woodruff LG, Solano F, Kilburn JE, Fey DL. Geochemical and mineralogical data for soils of the conterminous united states. U S Geol Surv Data Ser (2013) 801:1–26.
35. Amelung W, Zhang X. Determination of amino acid enantiomers in soils. Soil Biol Biochem (2001) 33:553–62. doi: 10.1016/S0038-0717(00)00195-4
36. BOAS NF. Method for the determination of hexosamines in tissues. J Biol Chem (1953) 204:553–63. doi: 10.1016/s0021-9258(18)66055-7
37. Küry D, Keller U. Trimethylsilyl-o-methyloxime derivatives for the measurement of [6,6-2H2]-d-glucose-enriched plasma samples by gas chromatography-mass spectrometry. J Chromatogr B Biomed Sci Appl (1991) 572:302–6. doi: 10.1016/0378-4347(91)80494-W
38. Dauwe B, Middelburg JJ, Herman PMJ, Heip CHR. Linking diagenetic alteration of amino acids and bulk organic matter reactivity. Limnol Oceanogr (1999) 44:1809–14. doi: 10.4319/lo.1999.44.7.1809
39. Lomstein BA, Jørgensen BB, Schubert CJ, Niggemann J. Amino acid biogeo- and stereochemistry in coastal Chilean sediments. Geochim Cosmochim Acta (2006) 70:2970–89. doi: 10.1016/j.gca.2006.03.015
40. Rosseel Y. Lavaan: An r package for structural equation modeling. J Stat Software (2012) 48:1–36.
41. Bates D, Mächler M, Bolker B, Walker S. Fitting linear mixed-effects models using lme4. J Stat Software (2015) 67:1–48. doi: 10.18637/jss.v067.i01
42. Oksanen J, Blanchet FG, Friendly M, Kindt R, Legendre P, McGlinn D, et al. Vegan: Community ecology package. (2019).
43. Jones DL, Shannon D, Murphy DV, Farrar J. Role of dissolved organic nitrogen (DON) in soil n cycling in grassland soils. Soil Biol Biochem (2004) 36:749–56. doi: 10.1016/j.soilbio.2004.01.003
44. Sjöberg G, Nilsson SI, Persson T, Karlsson P. Degradation of hemicellulose, cellulose and lignin in decomposing spruce needle litter in relation to n. Soil Biol Biochem (2004) 36:1761–8. doi: 10.1016/j.soilbio.2004.03.010
45. Werdin-Pfisterer NR, Kielland K, Boone RD. Soil amino acid composition across a boreal forest successional sequence. Soil Biol Biochem (2009) 41:1210–20. doi: 10.1016/j.soilbio.2009.03.001
46. Warren CR, Taranto MT. Temporal variation in pools of amino acids, inorganic and microbial n in a temperate grassland soil. Soil Biol Biochem (2010) 42:353–9. doi: 10.1016/j.soilbio.2009.11.017
47. Fiedler F, Schleifer K, Kandler O. Amino acid sequence of the threonine-containing mureins of coryneform bacteria. J Bacteriol (1973) 113:8–17. doi: 10.1128/jb.113.1.8-17.1973
48. El-Naggar A, Rasmussen J, De Neergaard A, El-Araby A, Høgh-Jensen H. Effect of plant species and temperature on amino acid release from plant material. Agron Sustain Dev (2010) 30:679–88. doi: 10.1051/agro/2009049
49. Gruber N, Galloway JN. An earth-system perspective of the global nitrogen cycle. Nature (2008) 451:293–6. doi: 10.1038/nature06592
50. Kuypers MMM, Marchant HK, Kartal B. The microbial nitrogen-cycling network. Nat Rev Microbiol (2018) 16:263–76. doi: 10.1038/nrmicro.2018.9
51. Schmidt MWI, Knicker H, Kögel-Knabner I. Organic matter accumulating in aeh and bh horizons of a podzol - chemical characterization in primary organo-mineral associations. Organic Geochem (2000), 31(7-8):727–34. doi: 10.1016/S0146-6380(00)00045-0
52. Wang X, Dai W, Filley TR, Wang C, Bai E. Aboveground litter addition for five years changes the chemical composition of soil organic matter in a temperate deciduous forest. Soil Biol Biochem (2021) 161:108381. doi: 10.1016/j.soilbio.2021.108381
53. Rumpel C, Kögel-Knabner I. Deep soil organic matter-a key but poorly understood component of terrestrial c cycle. Plant Soil (2011) 338:143–58. doi: 10.1007/s11104-010-0391-5
54. Kraffczyk I, Trolldenier G, Beringer H. Soluble root exudates of maize: Influence of potassium supply and rhizosphere microorganisms. Soil Biol Biochem (1984) 16:315–22. doi: 10.1016/0038-0717(84)90025-7
55. Forde BG, Lea PJ. Glutamate in plants: Metabolism, regulation, and signalling. in. J Exp Bot (2007) 58(9):2339–58. doi: 10.1093/jxb/erm121
56. Top SM, Filley TR. Effects of elevated CO2 on the extractable amino acids of leaf litter and fine roots. N Phytol (2014) 202:1257–66. doi: 10.1111/nph.12762
57. Miltner A, Kindler R, Knicker H, Richnow HH, Kästner M. Fate of microbial biomass-derived amino acids in soil and their contribution to soil organic matter. Org Geochem (2009) 40:978–85. doi: 10.1016/j.orggeochem.2009.06.008
58. Yeasmin S, Singh B, Kookana RS, Farrell M, Sparks DL, Johnston CT. Influence of mineral characteristics on the retention of low molecular weight organic compounds: A batch sorption-desorption and ATR-FTIR study. J Colloid Interface Sci (2014) 432:246–57. doi: 10.1016/j.jcis.2014.06.036
59. Jones DL, Hodge A. Biodegradation kinetics and sorption reactions of three differently charged amino acids in soil and their effects on plant organic nitrogen availability. Soil Biol Biochem (1999) 31:1331–42. doi: 10.1016/S0038-0717(99)00056-5
60. Vieublé Gonod L, Jones DL, Chenu C. Sorption regulates the fate of the amino acids lysine and leucine in soil aggregates. Eur J Soil Sci (2006) 57:320–9. doi: 10.1111/j.1365-2389.2005.00744.x
61. Yan G, Kim G, Kim J, Jeong YS, Kim Y. Dissolved total hydrolyzable enantiomeric amino acids in precipitation: Implications on bacterial contributions to atmospheric organic matter. Geochim Cosmochim Acta (2015) 153:1–14. doi: 10.1016/j.gca.2015.01.005
62. Heinzle J, Wanek W, Tian Y, Kengdo SK, Borken W, Schindlbacher A, et al. No effect of long-term soil warming on diffusive soil inorganic and organic nitrogen fluxes in a temperate forest soil. Soil Biol Biochem (2021) 158:108261. doi: 10.1016/j.soilbio.2021.108261
63. Wang X, Zhang W, Zhou F, Liu Y, He H, Zhang X. Distinct regulation of microbial processes in the immobilization of labile carbon in different soils. Soil Biol Biochem (2020) 142:107723. doi: 10.1016/j.soilbio.2020.107723
64. Osburn ED, McBride SG, Barrett JE. Historical forest disturbance reduces soil microbial efficiency across multiple carbon sources. Soil Biol Biochem (2022) 165:108542. doi: 10.1016/j.soilbio.2021.108542
65. Klink S, Keller AB, Wild AJ, Baumert VL, Gube M, Lehndorff E, et al. Stable isotopes reveal that fungal residues contribute more to mineral-associated organic matter pools than plant residues. Soil Biol Biochem (2022) 168:108634. doi: 10.1016/j.soilbio.2022.108634
66. Hu J, Huang C, Zhou S, Liu X, Dijkstra FA. Nitrogen addition increases microbial necromass in croplands and bacterial necromass in forests: A global meta-analysis. Soil Biol Biochem (2022) 165:108500. doi: 10.1016/j.soilbio.2021.108500
Keywords: proteinaceous amino acids, soil horizon, pH, vegetation type, soil organic matter, Metal-associated organic matter, mineralogy
Citation: Hong H, Ma L, Smith DB, Lu H, Yan C, Xia K and Williams MA (2022) Precipitation-derived effects on the characteristics of proteinaceous soil organic matter across the continental United States. Front. Soil Sci. 2:984927. doi: 10.3389/fsoil.2022.984927
Received: 02 July 2022; Accepted: 05 September 2022;
Published: 06 October 2022.
Edited by:
Shu Kee Lam, The University of Melbourne, AustraliaReviewed by:
Matthias Kaestner, Helmholtz Centre for Environmental Research (HZ), GermanyBartosz Adamczyk, Natural Resources Institute Finland (Luke), Finland
Copyright © 2022 Hong, Ma, Smith, Lu, Yan, Xia and Williams. This is an open-access article distributed under the terms of the Creative Commons Attribution License (CC BY). The use, distribution or reproduction in other forums is permitted, provided the original author(s) and the copyright owner(s) are credited and that the original publication in this journal is cited, in accordance with accepted academic practice. No use, distribution or reproduction is permitted which does not comply with these terms.
*Correspondence: Mark A. Williams, markwill@vt.edu
†These authors have contributed equally to this work and share first authorship
‡ORCID: Mark A. Williams, orcid.org/0000-0002-4766-9399