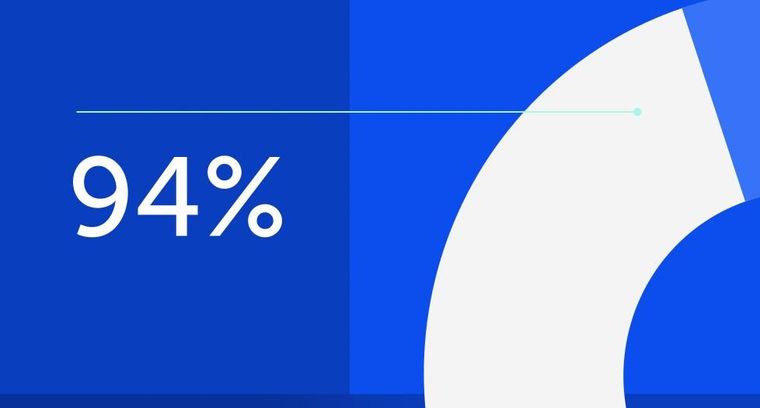
94% of researchers rate our articles as excellent or good
Learn more about the work of our research integrity team to safeguard the quality of each article we publish.
Find out more
EDITORIAL article
Front. Robot. AI, 21 September 2022
Sec. Field Robotics
Volume 9 - 2022 | https://doi.org/10.3389/frobt.2022.1002552
This article is part of the Research TopicAdvancements in Trajectory Optimization and Model Predictive Control for Legged SystemsView all 8 articles
Editorial on the Research Topic
Advancements in trajectory optimization and model predictive control for legged systems
In recent years we witnessed the proliferation of legged systems as advanced robotic platforms, which could be potentially employed in the near future on a huge spectrum of useful tasks. This is not only related to the success of quadrupeds, e.g. for inspection tasks (Bouman et al. (2020); Gehring et al. (2021)), but also to the increased capabilities of humanoid bipedal systems enabling operation in real-world settings (Agility Robotics, 2021; BostonDynamics (2021a)). To achieve such a level of autonomy, the use of advanced control and planning tools has been fundamental, in particular those allowing for the full exploitation of the dynamics of the system within a prediction horizon. In this respect, Trajectory Optimization (TO) and Model Predictive Control (MPC), among other methods, have proved to be highly effective on legged robotics platforms for a multitude of different tasks, including locomotion and manipulation Koenemann et al. (2015); Neunert et al. (2018); Di Carlo et al. (2018); Kuindersma et al. (2016); Belli et al. (2021). While TO is commonly used to plan complex open-loop trajectories over a long prediction horizon, MPC enables fast re-planning and feedback stabilization, over a shorter horizon. Thanks to the combination of these techniques, legged systems have been able to successfully demonstrate complex high-level skills like loco-manipulation in complex environments, agile locomotion, parkour, and much more in the years to come. However, it still remains an open question how to effectively employ TO and MPC on real legged systems. This requires smart formulations of contact-constrained robot dynamics, convenient models of the environment, as well as computationally efficient and real-time optimization algorithms.
The aim of this Research Topic has been to inform the robotics research community on the newest findings and future directions in TO and MPC applied to legged systems. In particular, we collected different contributions concerning recent applications of TO and MPC on legged systems related to climbing, dynamic locomotion and running, whole-body manipulation, robust formulation of contacts under modeling uncertainties, and software frameworks.
The first paper in our Research Topic, by L. Drnach et al. proposes a novel framework to account for contact uncertainty in TO. The authors developed chance complementarity constraints to convert the stochastic constraints into deterministic constraints showing that the chance constraints can mediate a trade-off between feasibility and robustness.
Locomotion in simulated bipedal systems is addressed in two works by K. Wan et al. and F. M. Smaldone et al. respectively. In particular, the first work proposes a fast MPC based on a Quadratic Programming (QP) formulation for automatic footstep placement; the computed controls are consecutively tracked by whole-body inverse dynamics. The locomotion scheme is validated in simulation using a novel platform, called SLIDER, walking blindly in various uneven terrain. The second work presents an MPC for running and walking based on the Variable-Height Inverted Pendulum (VH-IP) model (Koolen et al. (2016)) and adaptive footstep placement. The nonlinearity of the VH-IP is handled by splitting the gait generation into two consecutive stages, both requiring a QP to be solved. The proposed method is validated via dynamic simulations on the full-scale humanoid robot HRP-4 (Kaneko et al. (2011)), and it is experimentally validated on the small-sized robot OP3 (ROBOTIS (2022)).
In the two works from F. Aller et al. and P. Q. Lee et al. TO is applied to the REEM-C robot (PAL Robotics (2020)) to generate trajectories for a whole-body loco-manipulation task and dexterous manipulation, respectively. In the first work, sit-to-stand trajectories are optimized using optimal control, generating dynamic and human-like motions. Based on the REEM-C sensor data, a unified bench-marking procedure is presented based on two different experimental protocols. In the second paper, the buzzwire task is used to benchmark manipulation capabilities.
The work by F. Ruscelli et al. introduces a novel software framework named Horizon for TO tailored to robotics applications. Horizon is based on CasADi (Andersson et al. (2019)) and permits easily setting up and solving complex TO problems using different approaches and solvers. The authors present contact rich dynamic motions on different types of platforms, including KANGAROO (Roig et al. (2022)) and Spot® (BostonDynamics (2021b)) together with a real demonstration on the CENTAURO robot (Klamt et al. (2019)).
Finally, the paper from J. Lee et al. proposes a robust planning and control framework for climbing robots that provides robustness resistance to slippage in unknown environments. The framework combines TO for the center of mass position with re-planning, according to the uncertainties related to the environment; a whole-body controller is able to adjust the ground reaction force distribution in real-time. The proposed method is tested in simulation using a novel magnetic-legged climbing robot, Magneto.
We conclude by acknowledging the important contribution made by the reviewers of the articles submitted to this collection. They played a significant role in the high quality of the articles presented here. We hope that researchers and practitioners will enjoy this Research Topic and will be fascinated and inspired, as we are, by reading these articles.
EM organized and coordinated the Research Topic, and edited publications on the Research Topic, MP, and CZ participated in the Research Topic organization and edited publications.
Author EH is employed by PAL Robotics. Author MP is employed by Agility Robotics.
The remaining author declares that the research was conducted in the absence of any commercial or financial relationships that could be construed as a potential conflict of interest.
All claims expressed in this article are solely those of the authors and do not necessarily represent those of their affiliated organizations, or those of the publisher, the editors and the reviewers. Any product that may be evaluated in this article, or claim that may be made by its manufacturer, is not guaranteed or endorsed by the publisher.
Agility Robotics (2021). Digit-Advanced mobility for the human world. Available at: https://www.agilityrobotics.com/.
Andersson, J. A., Gillis, J., Horn, G., Rawlings, J. B., and Diehl, M. (2019). Casadi: A software framework for nonlinear optimization and optimal control. Math. Program. Comput. 11, 1–36. doi:10.1007/s12532-018-0139-4
Belli, I., Polverini, M. P., Laurenzi, A., Hoffman, E. M., Rocco, P., and Tsagarakis, N. G. (2021). “Optimization-based quadrupedal hybrid wheeled-legged locomotion,” in IEEE-RAS International Conference on Humanoid Robots (Humanoids), Munich, Germany, 19-21 July 2021, 41–46. doi:10.1109/HUMANOIDS47582.2021.9555780
Boston Dynamics (2021a). Atlas: The most dynamic humanoid robot. Available at: https://www.bostondynamics.com/atlas.
Boston Dynamics (2021b). Spot. Available at: https://www.bostondynamics.com/products/spot.
Bouman, A., Ginting, M. F., Alatur, N., Palieri, M., Fan, D. D., Touma, T., et al. (2020). “Autonomous spot: Long-range autonomous exploration of extreme environments with legged locomotion,” in IEEE/RSJ International Conference on Intelligent Robots and Systems (IROS), Las Vegas, USA, 25-29 October 2020, 2518–2525. doi:10.1109/IROS45743.2020
Di Carlo, J., Wensing, P. M., Katz, B., Bledt, G., and Kim, S. (2018). “Dynamic locomotion in the MIT cheetah 3 through convex model-predictive control,” in IEEE/RSJ international conference on intelligent robots and systems (IROS), Madrid, Spain, 01-05 October 2018, 1–9. doi:10.1109/IROS.2018.8594448
Gehring, C., Fankhauser, P., Isler, L., Diethelm, R., Bachmann, S., Potz, M., et al. (2021). “ANYmal in the field: Solving industrial inspection of an offshore HVDC platform with a quadrupedal robot,” in Field and service robotics (Singapore: Springer), 247–260. doi:10.1007/978-981-15-9460-1_18
Kaneko, K., Kanehiro, F., Morisawa, M., Akachi, K., Miyamori, G., Hayashi, A., et al. (2011). “Humanoid robot hrp-4-humanoid robotics platform with lightweight and slim body,” in IEEE/RSJ International Conference on Intelligent Robots and Systems, San Francisco, CA, USA, 25-30 September 2011, 4400–4407. doi:10.1109/IROS.2011.6094465
Klamt, T., Rodriguez, D., Baccelliere, L., Chen, X., Chiaradia, D., Cichon, T., et al. (2019). Flexible disaster response of tomorrow: Final presentation and evaluation of the centauro system. IEEE Robot. Autom. Mag. 26, 59–72. doi:10.1109/mra.2019.2941248
Koenemann, J., Del Prete, A., Tassa, Y., Todorov, E., Stasse, O., Bennewitz, M., et al. (2015). “Whole-body model-predictive control applied to the HRP-2 humanoid,” in IEEE/RSJ International Conference on Intelligent Robots and Systems (IROS), Hamburg, Germany, 28 September 2015 - 02 October 2015, 3346–3351. doi:10.1109/IROS.2015.7353843
Koolen, T., Posa, M., and Tedrake, R. (2016). “Balance control using center of mass height variation: Limitations imposed by unilateral contact,” in IEEE/RAS International Conference on Humanoid Robots (Humanoids), Cancun, Mexico, 15-17 November 2016, 8–15. doi:10.1109/HUMANOIDS.2016.7803247
Kuindersma, S., Deits, R., Fallon, M. F., Valenzuela, A., Dai, H., Permenter, F., et al. (2016). Optimization-based locomotion planning, estimation, and control design for the atlas humanoid robot. Auton. Robots 40, 429–455. doi:10.1007/s10514-015-9479-3
Neunert, M., Stäuble, M., Giftthaler, M., Bellicoso, C. D., Carius, J., Gehring, C., et al. (2018). Whole-body nonlinear model predictive control through contacts for quadrupeds. IEEE Robot. Autom. Lett. 3, 1458–1465. doi:10.1109/lra.2018.2800124
PAL Robotics (2020). REEM-C: A biped humanoid robot platform for boosting your research. Available at: https://pal-robotics.com/robots/reem-c/.
ROBOTIS (2022). OP3. Available at: https://emanual.robotis.com/docs/en/platform/op3/introduction/.
Roig, A., Kishor Kothakota, S., Miguel, P., Fernbach, N., Mingo Hoffman, E., and Marchionni, L. (2022). “On the hardware design and control architecture of the humanoid robot kangaroo,” in 6th Workshop on Legged Robots during the International Conference on Robotics and Automation (ICRA), Philadelphia (PA), USA, May 2022.
Keywords: trajectory optimization, model predictive control, legged robots, humanoid robots, whole-body control
Citation: Mingo Hoffman E, Zhou C and Parigi Polverini M (2022) Editorial: Advancements in trajectory optimization and model predictive control for legged systems. Front. Robot. AI 9:1002552. doi: 10.3389/frobt.2022.1002552
Received: 25 July 2022; Accepted: 01 August 2022;
Published: 21 September 2022.
Edited and reviewed by:
Kostas Alexis, Norwegian University of Science and Technology, NorwayCopyright © 2022 Mingo Hoffman, Zhou and Parigi Polverini. This is an open-access article distributed under the terms of the Creative Commons Attribution License (CC BY). The use, distribution or reproduction in other forums is permitted, provided the original author(s) and the copyright owner(s) are credited and that the original publication in this journal is cited, in accordance with accepted academic practice. No use, distribution or reproduction is permitted which does not comply with these terms.
*Correspondence: Enrico Mingo Hoffman, ZW5yaWNvLm1pbmdvQHBhbC1yb2JvdGljcy5jb20=
Disclaimer: All claims expressed in this article are solely those of the authors and do not necessarily represent those of their affiliated organizations, or those of the publisher, the editors and the reviewers. Any product that may be evaluated in this article or claim that may be made by its manufacturer is not guaranteed or endorsed by the publisher.
Research integrity at Frontiers
Learn more about the work of our research integrity team to safeguard the quality of each article we publish.