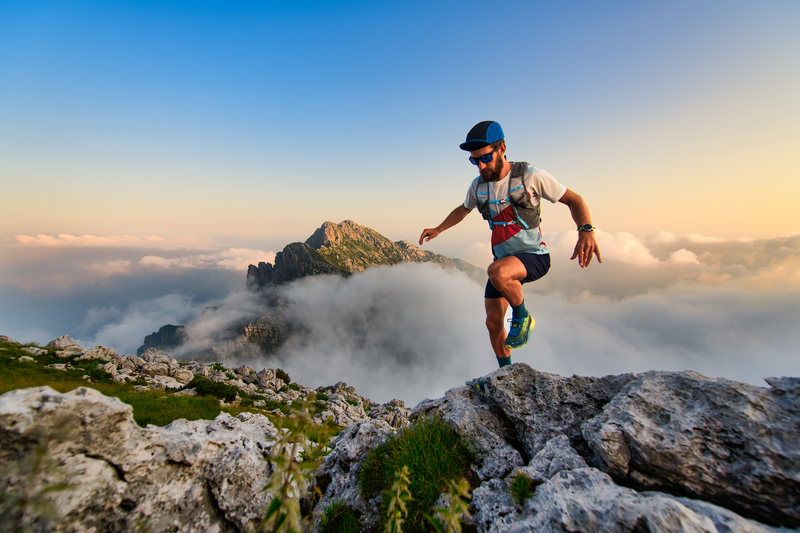
95% of researchers rate our articles as excellent or good
Learn more about the work of our research integrity team to safeguard the quality of each article we publish.
Find out more
REVIEW article
Front. Res. Metr. Anal. , 19 November 2024
Sec. Research Assessment
Volume 9 - 2024 | https://doi.org/10.3389/frma.2024.1493944
Cervical cancer represents a significant public health challenge, particularly affecting women's health globally. This study aims to advance the understanding of cervical cancer risk prediction research through a bibliometric analysis. The study identified 800 records from Scopus and Web of Science databases, which were reduced to 142 unique records after removing duplicates. Out of 100 abstracts assessed, 42 were excluded based on specific criteria, resulting in 58 studies included in the bibliometric review. Multiple scoping methods such as thematic analysis, citation analysis, bibliographic coupling, natural language processing, Latent Dirichlet Allocation and other visualisation techniques were used to analyse related publications between 2013 and 2024. The key findings revealed the importance of interdisciplinary collaboration in cervical cancer risk prediction, integrating expertise from mathematical disciplines, biomedical health, healthcare practitioners, public health, and policy. This approach significantly enhanced the accuracy and efficiency of cervical cancer detection and predictive modelling by adopting advanced machine learning algorithms, such as random forests and support vector machines. The main challenges were the lack of external validation on independent datasets and the need to address model interpretability to ensure healthcare providers understand and trust the predictive models. The study revealed the importance of interdisciplinary collaboration in cervical cancer risk prediction. It made recommendations for future research to focus on increasing the external validation of models, improving model interpretability, and promoting global research collaborations to enhance the comprehensiveness and applicability of cervical cancer risk prediction models.
Cervical cancer represents a significant public health challenge, particularly affecting women's health globally (Ding et al., 2021). This challenge arises from various factors, including limited access to screening and early detection services, inadequate healthcare infrastructure, low levels of disease awareness, and persistent socioeconomic disparities. Moreover, the high prevalence of human papillomavirus (HPV) infection, the primary cause of cervical cancer, coupled with insufficient HPV vaccination coverage, contributes to elevated incidence rates (WHO, 2024). To effectively combat this challenge, a comprehensive approach is imperative, involving strengthening healthcare systems, expanding access to affordable screening and vaccination programs, and implementing extensive education and awareness initiatives.
The Sustainable Development Goals (SDGs) adopted by United Nations member states highlight the importance of reducing premature mortality from non-communicable diseases (StatsSA, 2023). Efforts to address this align with the World Health Organisation's (WHO) call to action in 2018 to eliminate cervical cancer as a public health concern (Gultekin et al., 2020). WHO's strategy aims to increase HPV vaccination uptake and screening coverage to achieve a significant reduction in cervical cancer incidence rates.
Cervical cancer screening plays a pivotal role in reducing morbidity and mortality associated with the disease. Traditional methods like the Pap smear have evolved into more advanced techniques such as liquid-based cytology (LBC), high-risk HPV (hrHPV) testing, and artificial intelligence (AI)-powered systems (Swanson and Pantanowitz, 2024). These advancements aim to overcome limitations of sensitivity and accuracy associated with traditional approaches, particularly in resource-limited settings.
While traditional cervical cancer prediction models rely on established risk factors and statistical methods, machine learning (ML) techniques offer innovative solutions by using complex algorithms and large datasets (Liu et al., 2023; Meng et al., 2022; Hu et al., 2018). ML algorithms, including support vector machines, random forests, and deep learning networks, have shown promise in enhancing sensitivity, specificity, and overall accuracy in predicting cervical cancer risk (Rahimi et al., 2023; Zhang et al., 2020; Esteva et al., 2019). Challenges such as model interpretability and data bias remain significant concerns in ML application to cervical cancer prediction (Singh and Goyal, 2020).
To gain a comprehensive understanding of the current state of research and identify potential future directions, a bibliometric analysis is a valuable tool. This approach allows for the systematic exploration of the intellectual landscape by analysing publication patterns, citations, keywords, and collaborations within a specific research field (Vargas-Cardona et al., 2023). Bibliometric analysis, a quantitative methodology, offers a powerful tool to explore the evolving knowledge structure within a specific research field (Jimma, 2023). This method provides researchers with a systematic approach to analysing publication patterns, citations, keywords, and collaborations.
Through investigating these aspects, bibliometric analysis yields measurable, accurate, and detailed information on the field (Donthu et al., 2021). This comprehensive understanding empowers researchers to not only gain a thorough grasp of the subject matter but also to foster a multidisciplinary approach, a key factor in advancing scientific progress (Motamedi et al., 2023).
In the context of cervical cancer risk prediction modelling, a bibliometric analysis can reveal how this field has developed over time, identify prominent researchers and institutions contributing to the field, and uncover emerging research trends. This knowledge will be crucial for guiding future research ventures and improving our ability to predict and prevent cervical cancer. In this review, the authors employed a bibliometric analysis along with other scoping methods to investigate the field of predictive modelling for cervical cancer risk assessment.
The aim of this study was to identify foundational literature and thematic content in cervical cancer risk prediction modelling through citation analysis, and to examine research trends, collaboration patterns, and niche areas to uncover gaps and propose new directions for future research.
The study contributes to cervical cancer research by enhancing understanding of cervical cancer risk prediction through comprehensive thematic analysis, revealing core themes, relationships, and broader trends. It advances methodological approaches by integrating Braun and Clarke's framework with Natural Language Processing (NLP). The study identifies emerging niche themes like “machine learning algorithms” and “predictive models”, and highlights a multidisciplinary approach involving machine learning, deep learning, and clinical validation.
These insights can facilitate interdisciplinary collaborations and improve prevention, diagnosis, and treatment outcomes in cervical cancer research. For example, the identification of emerging themes such as “machine learning algorithms” can lead to collaborations between data scientists, statisticians, computer scientists, pathologists, general practitioners and oncologists, resulting in the development of advanced predictive models that enhance early detection and personalised treatment plans for cervical cancer patients.
The remainder of the study is structured as follows: the next section details the methodology that guided the study. It is followed by the results and discussion section, the limitations, and the conclusion.
This review uses bibliometric analysis, a method for studying scientific publications, to examine research on machine learning for cervical cancer risk prediction. It analyses the structure and content of existing research articles to understand how this field is developing. This review aims to provide a clear picture of current research trends and how machine learning is being used to improve cervical cancer risk assessment.
The Population, Intervention, Comparison, and Outcome (PICO) framework was used to structure the search strategy. This is summarised in Table 1. This method ensured a comprehensive search strategy to gather relevant publications related to predictive modelling in cervical cancer risk assessment, covering key aspects such as population characteristics, intervention methods, comparison with traditional approaches, and outcome measures.
The entire data collection process is illustrated in a flowchart (see Figure 1). This flowchart visually depicts the number of records identified, screened, excluded, and ultimately included in the final analysis, promoting transparency and replicability of our research methods.
Both Scopus and Web of Science databases were used to retrieve relevant publications. Scopus was chosen for its comprehensive coverage across various disciplines, while Web of Science provided access to high-quality scholarly literature, ensuring a thorough exploration of the research landscape. The search strategy was refined by including proximity operators.
Proximity operators function as specialised search query keywords designed to refine retrieval by dictating the closeness of terms within a document (Goldman et al., 1998). Unlike Boolean operators that focus on presence or absence, proximity operators emphasise the conceptual proximity of search terms. This ensures retrieved documents not only contain the specified keywords but also discuss them in close association, enhancing the relevance of results.
In Web of Science, the NEAR/x operator dictates the maximum number of intervening words between terms (e.g., “cancer NEAR/3 risk”). Conversely, Scopus utilises W/x expressions, requiring terms to appear in the specified order within a defined word range (e.g., “Cervical Cancer” W/2 “Machine learning”). Researchers can significantly improve the focus and relevance of their literature searches within Web of Science and Scopus by strategically employing proximity operators within each platform's designated syntax.
The retrieved data was saved in various file formats, e.g., .csv and .bib. The software EndNote was employed to facilitate the management of articles from both databases. EndNote allows for efficient organisation and storage of citation information, aiding in the subsequent stages of the research process.
The retrieved publications from both databases underwent initial screening to identify and eliminate duplicates, thereby ensuring the integrity of the dataset. Subsequently, citation information and metadata were standardised and formatted uniformly to facilitate seamless analysis and comparison across datasets. The datasets obtained from Scopus and Web of Science were merged, and duplicates were subsequently removed using R Studio. As a result, the final dataset comprised 100 unique articles for further analysis.
The articles were distributed among five authors, with each responsible for reviewing twenty articles. Each publication underwent a thorough assessment for relevance and quality, guided by predefined inclusion and exclusion criteria outlined in Table 2. The authors convened to collectively discuss each article and reached a consensus on whether to include or exclude it based on the established criteria. Publications pertaining to treatment or involving patients already diagnosed with cervical cancer were excluded from the final dataset. Consequently, the refined dataset consisted of 58 distinct articles selected for further analysis.
The accuracy of citation details and publication metadata was verified to minimise errors and inconsistencies in the dataset.
Science mapping, as defined by Donthu et al. (2021) examines the relationships between research constituents, focusing on intellectual interactions and structural connections among them. This analysis employed techniques such as citation analysis, thematic analysis, bibliographic coupling, co-word analysis, and co-authorship analysis (Donthu et al., 2021; Öztürk et al., 2024). When combined with network analysis, these techniques were instrumental in presenting the bibliometric structure and the intellectual structure of the research field.
Citation analysis was utilised to identify highly cited publications and uncover their thematic content (Khare and Jain, 2022). This involved examining the number of citations received by each publication to gauge its impact in the field. Relevant bibliometric software, such as VOSviewer and BiblioShiny, were employed to analyse citation data and visualise citation networks, aiding in the identification of influential publications and their intellectual connections.
This research approach merged the strengths of thematic analysis with the power of Natural Language Processing (NLP) techniques. Braun and Clarke's thematic analysis framework provided a systematic approach for identifying, analysing, and reporting patterns within qualitative data (Öztürk et al., 2024). Thematic analysis, a qualitative analysis method, involves coding documents to identify themes and capturing qualitative attributes relevant to the research subject (Braun and Clarke, 2006).
Initially, the dataset underwent Term Frequency-Inverse Document Frequency (TF-IDF) vectorisation and stop word removal to preprocess the text, followed by the application of Latent Dirichlet Allocation for topic modelling to identify five topics within the abstracts. The second approach employed Generate Similar (Gensim) dictionary to convert the text data into a bag-of-words representation, followed by LDA-based topic modelling configured to identify 6 topics. LDA is a statistical technique that identifies latent thematic structures within a collection of documents (Jelodar et al., 2019).
Two approaches were combined for examining the abstract text data utilising LDA to identify topics within the abstracts, with the LDA model extracting the most prominent words associated with each topic, revealing prevalent themes related to cervical cancer risk prediction modelling. Subsequently, the topics from both approaches were merged to form four overarching themes.
The thematic analysis process involved familiarisation with the data, followed by documenting and coding, similar to tokenisation. Irrelevant words were removed to focus on pertinent information. Themes were generated by observing patterns in the codes and assessing their correlation, ensuring alignment with the research objectives. The iterative review of themes led to their refinement and finalisation, ultimately contributing to the clarification of prevalent themes within the abstracts pertaining to cervical cancer risk prediction modelling.
However, to gain a more comprehensive understanding, three complementary methods were used: thematic mapping, thematic evolution analysis, and trend topic analysis. Thematic mapping involves visually representing the relationships between identified themes, creating a map-like depiction that clarifies how themes interact and influence each other (Agbo et al., 2021). This visual representation enhances our comprehension of the thematic landscape and the interplay of themes within the data. Using BiblioShiny, a software program for analysing research publications, this study was able to identify how the main themes in cervical cancer research have shifted focus over time.
This revealed interesting trends, such as one topic becoming less popular while another gained more attention (Khare and Jain, 2022). This analysis also revealed the emergence of entirely new themes within the data. Finally, trend topic analysis complemented thematic analysis by exploring broader trends within the data. This involved analysing the frequency of specific terms or concepts to identify areas of growing interest or decline over time (Liang et al., 2021).
Bibliographic coupling was employed to identify recent and niche publications that share common references. This technique is based on the assumption that scholarly articles that cite a significant number of the same sources are likely to be similar in their content and research focus (Donthu et al., 2021). The study analysed a dataset of 48 articles and created a visual representation to explore these connections.
Due to VOSviewer's criteria for bibliographic coupling, only 48 out of the 58 articles were included in the visualisation of research trends. This visualisation helped to understand the thematic landscape and how prominent themes connect with specific research fields and journals. Researchers can identify thematic clusters within a body of literature by analysing these citation patterns.
BiblioShiny was used to analyse publication trends and geographic distribution of research output relevant to cervical cancer risk prediction. VOSviewer complemented this by creating visualisations like citation networks and thematic maps. These techniques helped interpret results, identifying key themes, research gaps, emerging trends, and prominent research institutions/authors in cervical cancer risk prediction research.
Table 3 illustrates the data, comprising 58 articles sourced from 49 publications, exhibited a robust annual growth rate of 13.43%, reflecting a growth trend. The average document age of 2.97 years and an average citation count of 14.84 per document further highlighted the recent nature and influential reach of these publications.
Collaboration is a defining characteristic of authorship on the prediction of cervical cancer, with an average of 5.28 co-authors per document. The study observed a significant trend of interdisciplinary collaboration in the field of cervical cancer risk predictive modelling. This collaboration was characterised by the integration of expertise from diverse academic domains, including computer science, mathematics, statistics, biomedical engineering, epidemiology, oncology, pathology, obstetrics, gynaecology, and public health. International co-authorships were absent, suggesting potential avenues for expanding research collaborations and fostering global knowledge exchange in cervical cancer research.
Notably, only one document had a single author, further emphasising the collaborative nature of research in this domain. As all documents are classified as articles, the data underscores the emphasis on scholarly rigour and methodological depth within cervical cancer research. These findings highlighting the importance of interdisciplinary collaborations and innovative discoveries in the fight against predictive cervical cancer. For example, one highly cited work documented a successful collaboration between computer scientists, biomedical engineers, pathologists, and oncologists. This collaboration resulted in a computer-aided diagnosis (CAD) system for cervical cancer screening. The system achieved high accuracy in detecting and classifying cervical tissue abnormalities, potentially leading to improved patient outcomes and increased survival rates for women (Alquran et al., 2022).
The top 20 highly cited research articles presented in Table 4 explored various methods for improving cervical cancer screening, diagnosis, and risk prediction. Machine learning algorithms were a common theme, with studies applying them to analyse clinical data, Pap smear images, and self-collected samples. These approaches have achieved high accuracy in some cases, exceeding the performance of traditional methods.
Recent advancements in cervical cancer research are revolutionising screening, diagnosis, and risk prediction methods. Machine learning algorithms are at the forefront, with studies such as Ijaz et al. (2020) utilising them to analyse clinical data for early cancer detection. Deep learning, a specialised machine learning technique, is also proving valuable. Hussain et al. (2020) demonstrate its effectiveness in Pap smear image analysis and classification using convolutional neural networks.
Beyond machine learning, the table highlights other promising techniques. Yaman and Tuncer (2022) introduced a novel deep feature extraction method for accurate cancer detection, while Ali et al. (2021) showcased the efficacy of machine learning models in analysing various clinical datasets to identify early-stage cervical cancer. Additionally, research on HPV testing, a major risk factor, is ongoing, with studies exploring its potential to improve screening programs (Zhao et al., 2021). Furthermore, Figure 2 shows the most globally cited authors. Overall, these advancements offer significant hope for improved cervical cancer outcomes and enhanced patient care.
The top 20 highly cited publications in cervical cancer research revealed a multi-pronged approach using recent advancements. Machine learning and deep learning techniques were prominent, demonstrating effectiveness in analysing various data sources—clinical data, Pap smear images, and HPV test results. Algorithms such as Random Forest and convolutional neural networks achieved high accuracy in classification and detection tasks.
Furthermore, researchers developed methods for efficient feature selection and model optimisation (e.g., Boruta analysis) to refine these tools and enhance model performance. Early detection remains a critical focus, with novel models such as the Cervical Cancer Prediction Model utilising risk factors and clinical data for early identification. This focus aimed to enhance patient outcomes through timely intervention.
Enhanced screening strategies are another key theme. Integration of advanced technologies such as digital colposcopy and image analysis were explored to improve accuracy and efficiency. In addition, the role of HPV testing in identifying high-risk individuals was investigated for improved screening protocols. Finally, the importance of clinical validation and translation of research findings into practical applications was emphasised. Extensive validation studies ensure the generalisability of proposed methodologies, thus, bridging the gap between research and improved patient care.
Thematic content analysis revealed a multidisciplinary approach in cervical cancer research. Machine learning, deep learning, clinical validation, and translation contributed significantly to innovation in cervical cancer detection, diagnosis, and management.
The reviewed studies on machine learning in cervical cancer research reveal several gaps. Many studies lack external validation on independent datasets, limiting the robustness and generalisability of their findings. Most studies do not address model interpretability, crucial for understanding prediction mechanisms. Socioeconomic factors, such as income and access to healthcare, are largely ignored despite their significant impact on cervical cancer risk and outcomes. Furthermore, information on dataset size and diversity is often missing, hindering the assessment of model performance and applicability across different populations. Addressing these gaps is essential for advancing the practical application of machine learning in cervical cancer care.
The visual representation in Figure 3 depicts a co-citation network with authors as nodes connected by lines indicating their co-citations, with the central node labelled “Zhang” prominently displayed. Surrounding this central node are numerous red lines connecting to other author nodes, suggesting that Zhang is highly cited with these authors. Other author nodes, labelled with various names or abbreviations, are scattered around the central node, with connections represented by red lines of varying opacities.
The denser lines indicate stronger co-citation relationships, resembling a starburst pattern emanating from the central “Zhang” node. This network structure implies that “Zhang” holds significant influence in the field, with the connections revealing collaborative relationships and influential connections among authors. Co-citation networks offer valuable insights into scholarly collaboration, research trends, and influential figures, aiding researchers in understanding the dynamics of scientific collaboration and impact.
The analysis of abstracts identified emerging themes within cervical cancer risk prediction modelling, represented by codes extracted from the text data. Table 5 summarises the frequency counts of these codes, showcasing the prominence of keywords such as “cervical,” “cancer,” “model,” “patient,” and “accuracy.” Additionally, codes such as “pap,” “study,” and “machine” were also prevalent, indicating themes related to diagnostic methods, research studies, and machine learning techniques, respectively. These codes provided valuable insights into the key concepts and areas of focus within the abstracts, facilitating a deeper understanding of the research landscape in cervical cancer risk prediction.
TF-IDF vectorisation and LDA produced topics characterised by prominent keywords, delineating prevalent themes within the abstracts. These topics encompassed a spectrum of concepts, with Topic 0 focusing on cancer and cervical, Topic 1 emphasising patient-related terms, Topic 2 highlighting medical images, Topic 3 focusing on patient-centred models, and Topic 4 showcasing features pertinent to predictive modelling. The weightings in Tables 6, 7 represent the probability of a word belonging to a specific topic, with higher weights indicating a stronger association.
Additionally, Gensim and LDA topic modelling revealed five key themes within cervical cancer risk prediction research. Topics 0 and 1 featured core concepts such as “cancer,” “cervical,” “model,” and “accuracy,” emphasising model development and machine learning. Topic 2 suggested the use of medical images for detection, while Topic 3 focused on patient-centred models with interpretable results. Topic 4 pointed to research on feature engineering for improved model performance, highlighting a strong focus on model development and translating research into practical clinical applications for improved patient outcomes.
This convergence of findings from both methodologies emphasises the multifaceted nature of cervical cancer risk prediction research. The clusters derived from both approaches included:
1. Cervical cancer diagnosis and risk assessment with machine learning: this cluster merged topics emphasising machine learning models for diagnosis and risk assessment in cervical cancer, incorporating both image-based and non-image-based approaches.
2. HPV testing and risk factors for cervical cancer: this cluster focused on the role of HPV testing, self-sampling methods, and their association with cervical cancer risk, potentially including comparisons with other risk factors.
3. Prognosis and risk stratification in cervical cancer: this cluster highlighted the importance of clinicopathological features, AI, and nomograms in determining a patient's risk grade and prognosis in cervical cancer.
4. Cervical cancer recurrence analysis: this cluster explored factors associated with cervical cancer recurrence, including tumour characteristics, risk models, and survival analysis methods, addressing data imbalance with SMOTE.
The integration of findings from both the Braun and Clarke thematic analysis and the NLP approach underscored the multifaceted nature of cervical cancer risk prediction research. The thematic and NLP analyses provided specific, actionable insights into cervical cancer risk prediction research. Thematic analysis revealed emerging themes such as diagnostic methods, machine learning techniques, and patient-focused models, underscored by the frequent occurrence of keywords like “cervical,” “cancer,” “model,” and “accuracy.”
NLP techniques like TF-IDF vectorisation and LDA highlighted key topics, including the development of predictive models, the use of medical images, and patient-centred approaches, as seen in the prevalence of terms across topics. This integrated methodology found research clusters such as machine learning for diagnosis, HPV testing, prognosis and risk stratification, and recurrence analysis. These findings emphasise the multidisciplinary nature of cervical cancer risk prediction, showing the combination of qualitative and quantitative insights to enhance understanding and guide future research directions.
The provided Figure 4 illustrates the thematic understanding of cervical cancer research and machine learning, aligning with the identified themes using BiblioShiny. A quadrant plot visualises the thematic landscape of cervical cancer research and machine learning. Two axes, developmental degree (vertical) and relevance degree (horizontal), divide the plot into quadrants. Niche themes, like “pap smear images” and “machine learning algorithms,” occupy a specific space, reflecting their focused nature. Conversely, central themes like “cervical cancer prediction” reside in the core area due to their high relevance.
Emerging or declining themes, such as “challenging process due to detecting cervical cancer,” are positioned accordingly. Established themes like “confidence interval (CI)” form the foundational “basic themes” quadrant. Specific terms like “support vector machine,” “human papillomavirus (HPV),” or “squamous intraepithelial lesions” further illustrate the thematic breakdown within each quadrant. The study gained insights into the relative importance and developmental stages of various research areas, ultimately aiding in identifying promising avenues and knowledge gaps within the field of cervical cancer research and machine learning by analysing this thematic distribution.
The study aligns with current trends, as commonly used models in cervical cancer risk prediction include support vector machine (SVM), random forest (RF), and multivariable logistic regression. This is further supported by research comparing machine learning algorithms for disease prediction, which identified SVM and RF as preferred algorithms (Uddin et al., 2019; Zhang et al., 2023; Yang et al., 2019).
The niche themes “detect cervical cancer” and “machine learning algorithms” signify specialised areas of focus within the broader research field. This means that there is a concentrated effort on using advanced computational techniques to improve the accuracy and efficiency of cervical cancer detection.
Researchers are increasingly integrating machine learning algorithms to develop innovative diagnostic tools, which can potentially enhance early detection, optimise treatment plans, and improve patient outcomes. This targeted research highlights the importance of interdisciplinary collaboration in advancing medical technologies and addressing specific challenges within cervical cancer care.
The visualisation in Figure 5 revealed that between 2013 and 2019, research activities primarily focused on refining pap smear imaging techniques, a fundamental aspect of cervical cancer screening. This sustained emphasis on pap smear imaging highlights the pivotal role in identifying precancerous lesions and early-stage malignancies. Concurrently, investigations into cervical cancer risk spanned several years (2013–2021), reflecting ongoing efforts to elucidate risk factors, epidemiology, and preventive measures. Studies explored genetic predispositions, viral associations (such as human papillomavirus), and lifestyle factors contributing to cervical cancer risk, contributing to a comprehensive understanding of the disease's aetiology and prevention strategies.
The years 2020–2021 witnessed a significant shift with the emergence of machine learning algorithms in cervical cancer research. Researchers adopted artificial intelligence to enhance diagnostic accuracy and predictive models, analysing complex datasets comprising imaging results and patient histories for personalised risk assessment. Subsequently (2021–2022), predictive modelling gained prominence, aiming to forecast individualised outcomes such as disease progression, treatment response, and recurrence. Integrating clinical data with machine learning algorithms facilitated more precise risk stratification, marking a notable advancement in cervical cancer risk prediction and management strategies.
The provided Figure 6 offers insight into the evolving landscape of topics related to cervical cancer and machine learning from 2016 to 2021. Each topic is depicted by blue dots, with their sizes indicating the term frequency, denoted by a legend on the right. Notable topics include cervical cancer detection, machine learning models, Pap smear images, cervical cancer screening, machine learning algorithms, support vector machine, cervical cancer risk, cervical cancer patients, and human papillomavirus (HPV). The graph highlights the progressive nature of research and development in cervical cancer detection, machine learning, and associated domains, emphasising the ongoing efforts to advance diagnostic and predictive capabilities for improved healthcare outcomes.
The significance of the word cloud (see Figure 7) analysis lies in its ability to highlight key themes and trends within cervical cancer screening research. It reveals the central focus on cervical cancer screening, indicating the ongoing efforts to improve detection and diagnosis methods. The prominence of terms like “Pap Smear Images,” “Human Papillomavirus (HPV),” “Low-Grade Squamous Intraepithelial,” “High-Grade Squamous Intraepithelial,” and “Machine Learning Algorithms” suggests a shift towards incorporating advanced technology for more accurate and efficient screening processes.
This implies a potential transformation in clinical practise, with the adoption of innovative approaches to enhance early detection rates and improve patient outcomes. Terms like “Cervical Cancer Detection,” “Random Forest (RF),” and “Support Vector Machine” show the importance of early diagnosis and the specific techniques employed to achieve this goal. Overall, the word cloud analysis shows the evolving field of cervical cancer screening research, highlighting the integration of technology and the ongoing pursuit of improved screening methods for better patient care.
A key finding was the recurring theme of “diagnostics” (see Figure 8). This theme appeared as a central element in the visualisation, highlighting its widespread relevance across the analysed articles. The prominence of diagnostics suggested its importance as a major area of research focus.
The visualisation also revealed a diverse range of journals and research fields connected to diagnostics. Each surrounding node represented a specific journal or field of study, such as the Asian Journal of Information Technology, Healthcare Technology Letters, International Journal of Molecular Sciences, Abdominal Radiology, Multimedia Tools and Applications, Computational Mathematics International Journal, and Indian Journal of Science & Technology. This variety of nodes showcased the broad scope of research areas associated with diagnostics.
Red lines connect the central “diagnostics” theme to other nodes, indicating bibliographic coupling. This means there were close relationships between diagnostics research and various research domains. These connections highlighted the interdisciplinary nature of diagnostics research, suggesting its interconnectedness with multiple fields. The visualisation provided evidence for the role diagnostics plays in integrating different areas of research.
This approach went beyond traditional citation analysis by uncovering interconnected relationships between research topics and journals, offering valuable insights into the multidisciplinary nature of diagnostics research. Unlike traditional citation analysis, which primarily focuses on citation counts and direct references, Figure 8 provided a more comprehensive view of the complex interplay between different areas of scholarly inquiry.
The findings demonstrated the collaborative nature of interdisciplinary research efforts in driving advancements in cervical cancer diagnosis by highlighting the interconnectedness between diagnostics research and various research domains. This deeper understanding emphasises the importance of interdisciplinary collaboration in addressing complex healthcare challenges and highlights the pivotal role of diagnostics research in advancing diagnostic capabilities for cervical cancer.
The map in Figure 9 illustrates the distribution of scientific output across different countries regarding predictive modelling for cervical cancer risk. Countries are shaded in varying shades of blue to denote the volume of publications, with darker shades indicating higher production. Notably, India, United States, China, and Australia emerge as significant contributors to this field. Conversely, Africa appears mostly unshaded, indicating minimal research output, underscoring the disparity in scientific contributions between developed nations and African countries.
This observation emphasises the necessity for heightened investment and focus on scientific research concerning cervical cancer risk prediction in African nations. Closing this research gap not only aids in addressing the burden of cervical cancer within Africa but also holds promise for enhancing screening, prevention, and treatment strategies on a global scale. Redirecting resources and support towards scientific activities in Africa can pave the way for achieving greater equity in healthcare access and mitigating the global burden of cervical cancer.
The provided Figure 10 illustrates a succinct overview of the number of articles produced each year in the field of predictive modelling for cervical cancer risk. Spanning from ~2013 to 2023, the x-axis denotes the years, while the y-axis indicates the number of articles published. Notably, until around 2018, there was a steady production of fewer than five articles per year. However, post-2018, there is a notable surge in article production, reaching a peak in 2022 with over 15 articles. Subsequently, there is a sharp decline in 2022. This spike in 2021 indicates a surge in research activity, while the decline in 2022 may suggest a shift in research focus or external factors influencing publication trends.
This analysis of publication trends highlights the growing importance of predictive modelling in cervical cancer risk assessment, signifying its potential to improve preventative healthcare strategies. While the decline in 2023 publications requires further exploration, it highlights the dynamic nature of this research field. Continued monitoring of publication trends alongside a deeper understanding of the underlying reasons for these shifts can provide valuable insights for researchers and stakeholders invested in advancing this critical field.
Limitations of this study include the exclusive reliance on the Scopus and Web of Science databases, potentially omitting relevant studies from other sources. Our search encompassed all literature on predictive modelling for cervical cancer risk. However, scholarly attention to this topic became prominent only from 2013 onwards. No literature predating 2013 addressed predictive modelling for cervical cancer risk, leading to the retrieval of relevant articles exclusively from 2013 onwards.
This study aimed to identify literature and thematic content in cervical cancer risk prediction modelling through citation analysis and to explore research trends, collaboration patterns, and niche areas. The study significantly enhanced the understanding of cervical cancer risk prediction by integrating Braun and Clarke's framework with NLP and LDA. It provided insights into core themes, relationships, and broader trends, offering a solid foundation for future research and improvements in cervical cancer prevention, diagnosis, and treatment.
Integrating diverse expertise from fields such as mathematical disciplines, biomedical health, healthcare practitioners, public health and policy is essential for a comprehensive approach to cervical cancer risk prediction. This interdisciplinary collaboration leads to more robust and holistic solutions. The adoption of advanced machine learning algorithms, transitioning from simpler models like logistic regression to more complex algorithms such as random forest and support vector machines, significantly enhances the accuracy and efficiency of cervical cancer detection and predictive modelling.
These advancements are crucial for early detection and improved patient outcomes, which are vital for effective public health interventions. However, many studies lack external validation on independent datasets, limiting the robustness and generalisability of their findings. Furthermore, addressing model interpretability is crucial for understanding prediction mechanisms and ensuring that healthcare providers can trust and effectively use these models in clinical settings.
FN: Writing – original draft, Writing – review & editing. BM: Writing – original draft, Writing – review & editing. PM: Writing – original draft, Writing – review & editing. PS: Writing – original draft, Writing – review & editing. MM: Writing – original draft, Writing – review & editing. TH: Supervision, Writing – review & editing.
The author(s) declare that no financial support was received for the research, authorship, and/or publication of this article.
The authors declare that the research was conducted in the absence of any commercial or financial relationships that could be construed as a potential conflict of interest.
All claims expressed in this article are solely those of the authors and do not necessarily represent those of their affiliated organizations, or those of the publisher, the editors and the reviewers. Any product that may be evaluated in this article, or claim that may be made by its manufacturer, is not guaranteed or endorsed by the publisher.
Agbo, F. J., Oyelere, S. S., Suhonen, J., and Tukiainen, M. (2021). Scientific production and thematic breakthroughs in smart learning environments: a bibliometric analysis. Smart Learn. Environ. 8:1. doi: 10.1186/s40561-020-00145-4
Ali, M. M., Ahmed, K., Bui, F. M., Paul, B. K., Ibrahim, S. M., Quinn, J. M. W., et al. (2021). Machine learning-based statistical analysis for early stage detection of cervical cancer. Comput. Biol. Med. 13:1049859. doi: 10.1016/j.compbiomed.2021.104985
Alquran, H., Azani Mustafa, W., Abu Qasmieh, I., Mohd Yacob, Y., Alsalatie, M., Al-Issa, Y., et al. (2022). Cervical cancer classification using combined machine learning and deep learning approach. Comp. Mater. Continua 72, 5117–5134. doi: 10.32604/cmc.2022.025692
Braun, V., and Clarke, V. (2006). Using thematic analysis in psychology. Qual. Res. Psychol. 3, 77–101. doi: 10.1191/1478088706qp063oa
Ding, D., Lang, T., Zou, D., Tan, J., Chen, J., Zhou, L., et al. (2021). Machine learning-based prediction of survival prognosis in cervical cancer. BMC Bioinform. 22:331. doi: 10.1186/s12859-021-04261-x
Donthu, N., Kumar, S., Mukherjee, D., Pandey, N., and Lim, W. M. (2021). How to conduct a bibliometric analysis: an overview and guidelines. J. Bus. Res. 133, 285–296. doi: 10.1016/j.jbusres.2021.04.070
Esteva, A., Robicquet, A., Ramsundar, B., Kuleshov, V., DePristo, M., Chou, K., et al. (2019). A guide to deep learning in healthcare. Nat. Med. 25, 24–29. doi: 10.1038/s41591-018-0316-z
Goldman, R., Shivakumar, N., Venkatasubramanian, S., and Garcia-Molina, H. (1998). “Proximity search in databases,” in 24rd International Conference on Very Large Data Bases (VLDB 1998), August 24–27, 1998 (New York, NY).
Gultekin, M., Ramirez, P. T., Broutet, N., and Hutubessy, R. (2020). World Health Organization call for action to eliminate cervical cancer globally. Int. J. Gynecol. Cancer 30, 426–427. doi: 10.1136/ijgc-2020-001285
Hu, B., Dixon, P., Jacobs, J., Dennerlein, J., and Schiffman, J. (2018). Machine learning algorithms based on signals from a single wearable inertial sensor can detect surface-and age-related differences in walking. J. Biomech. 71, 37–42. doi: 10.1016/j.jbiomech.2018.01.005
Hussain, E., Mahanta, L. B., Das, C. R., and Talukdar, R. K. (2020). A comprehensive study on the multi-class cervical cancer diagnostic prediction on pap smear images using a fusion-based decision from ensemble deep convolutional neural network. Tissue Cell 65:101347. doi: 10.1016/j.tice.2020.101347
Ijaz, M. F., Attique, M., and Son, Y. (2020). Data-driven cervical cancer prediction model with outlier detection and over-sampling methods. Sensors 20:2809. doi: 10.3390/s20102809
Jelodar, H., Wang, Y., Yuan, C., Feng, X., Jiang, X., Li, Y., et al. (2019). Latent Dirichlet allocation (LDA) and topic modeling: models, applications, a survey. Multimed. Tools Appl. 78, 15169–15211. doi: 10.1007/s11042-018-6894-4
Jimma, B. L. (2023). Artificial intelligence in healthcare: a bibliometric analysis. Telemat. Inf. Rep. 9:100041. doi: 10.1016/j.teler.2023.100041
Khare, A., and Jain, R. (2022). Mapping the conceptual and intellectual structure of the consumer vulnerability field: a bibliometric analysis. J. Bus. Res. 150, 567–584. doi: 10.1016/j.jbusres.2022.06.039
Liang, C., Qiao, S., Olatosi, B., Lyu, T., and Li., X. (2021). Emergence and evolution of big data science in HIV research: bibliometric analysis of federally sponsored studies 2000–2019. Int. J. Med. Inform. 154:104558. doi: 10.1016/j.ijmedinf.2021.104558
Liu, S., Zhou, Y., Wang, C., Shen, J., and Zheng, Y. (2023). Prediction of lymph node status in patients with early-stage cervical cancer based on radiomic features of magnetic resonance imaging (MRI) images. BMC Med. Imaging 23:101. doi: 10.1186/s12880-023-01059-6
Meng, B., Li, G., Zeng, Z., Zheng, B., Xia, Y., Li, C., et al. (2022). Establishment of early diagnosis models for cervical precancerous lesions using large-scale cervical cancer screening datasets. Virol. J. 19:177. doi: 10.1186/s12985-022-01908-w
Motamedi, N., Sheikhshoaei, F., Ghazimirsaeid, J., Mansourzadeh, M. J., and Dehdarirad, H. (2023). Bibliometric analysis and topic modeling of information systems in maternal health publications. Int. J. Inf. Sci. Manag. 21, 85–101. doi: 10.22034/ijism.2023.1977814.0
Öztürk, O., Kocaman, R., and Kanbach, D. K. (2024). How to design bibliometric research: an overview and a framework proposal. Rev. Manag. Sci. 18, 3333–3361. doi: 10.1007/s11846-024-00738-0
Rahimi, M., Akbari, A., Asadi, F., and Emami, H. (2023). Cervical cancer survival prediction by machine learning algorithms: a systematic review. BMC Cancer 23:341. doi: 10.1186/s12885-023-10808-3
Singh, S. K., and Goyal, A. (2020). Performance analysis of machine learning algorithms for cervical cancer detection. Int. J. Healthc. Inf. Syst. Inf. 15, 1–21. doi: 10.4018/IJHISI.2020040101
Swanson, A. A., and Pantanowitz, L. (2024). The evolution of cervical cancer screening. J. Am. Soc. Cytopathol. 13, 10–15. doi: 10.1016/j.jasc.2023.09.007
Uddin, S., Khan, A., Hossain, M. E., and Moni, M. A. (2019). Comparing different supervised machine learning algorithms for disease prediction. BMC Med. Inform. Decis. Mak. 19:281. doi: 10.1186/s12911-019-1004-8
Vargas-Cardona, H. D., Rodriguez-Lopez, M., Arrivillaga, M., Vergara-Sanchez, C., García-Cifuentes, J. P., Bermúdez, P. C., et al. (2023). Artificial intelligence for cervical cancer screening: scoping review, 2009-2022. Int. J. Gynecol. Obstetr. 165, 566–578. doi: 10.1002/ijgo.15179
Yaman, O., and Tuncer, T. (2022). Exemplar pyramid deep feature extraction based cervical cancer image classification model using pap-smear images. Biomed. Signal Process. Control. 73:103428. doi: 10.1016/j.bspc.2021.103428
Yang, W., Gou, X., Xu, T., Yi, X., and Jiang, M., (eds.). (2019). “Cervical cancer risk prediction model and analysis of risk factors based on machine learning,” in Proceedings of the 2019 11th International Conference on Bioinformatics and Biomedical Technology (Stockholm), 50–54. doi: 10.1145/3340074.3340078
Zhang, C., Xu, J., Tang, R., Yang, J., Wang, W., Yu, X., et al. (2023). Novel research and future prospects of artificial intelligence in cancer diagnosis and treatment. J. Hematol. Oncol. 16:114. doi: 10.1186/s13045-023-01514-5
Zhang, H., Guo, Y., Prosperi, M., and Bian, J. (2020). An ontology-based documentation of data discovery and integration process in cancer outcomes research. BMC Med. Inform. Decis. Mak. 20:292. doi: 10.1186/s12911-020-01270-3
Keywords: cervical cancer, risk prediction, machine learning, artificial intelligence, thematic analysis, natural language processing, latent Dirichlet allocation, predictive modelling
Citation: Ngema F, Mdhluli B, Mmileng P, Shungube P, Makgaba M and Hossana T (2024) A bibliometric review of predictive modelling for cervical cancer risk. Front. Res. Metr. Anal. 9:1493944. doi: 10.3389/frma.2024.1493944
Received: 10 September 2024; Accepted: 25 October 2024;
Published: 19 November 2024.
Edited by:
Dimitrios Katsaros, University of Thessaly, GreeceReviewed by:
Antonis Sidiropoulos, International Hellenic University, GreeceCopyright © 2024 Ngema, Mdhluli, Mmileng, Shungube, Makgaba and Hossana. This is an open-access article distributed under the terms of the Creative Commons Attribution License (CC BY). The use, distribution or reproduction in other forums is permitted, provided the original author(s) and the copyright owner(s) are credited and that the original publication in this journal is cited, in accordance with accepted academic practice. No use, distribution or reproduction is permitted which does not comply with these terms.
*Correspondence: Francis Ngema, ZnJhbmNpc25nZW1hQGdtYWlsLmNvbQ==
Disclaimer: All claims expressed in this article are solely those of the authors and do not necessarily represent those of their affiliated organizations, or those of the publisher, the editors and the reviewers. Any product that may be evaluated in this article or claim that may be made by its manufacturer is not guaranteed or endorsed by the publisher.
Research integrity at Frontiers
Learn more about the work of our research integrity team to safeguard the quality of each article we publish.