- 1HCTI–EA 4249, Université de Bretagne Occidentale, Brest, France
- 2Analyse et Traitement Informatique de la Langue Française (ATILF), Université de Lorraine, Nancy, France
- 3Direction de la Documentation, Ecole des Ponts ParisTech, Champs-sur-Marne, France
- 4LISIS, Centre National de la Recherche Scientifique, Université Paris-Est Marne-la-Vallée, Institut National de la Recherche Agronomique, ESIEE Paris, Champs-sur-Marne, France
An abstract is not only a mirror of the full article; it also aims to draw attention to the most important information of the document it summarizes. Many studies have compared abstracts with full texts for their informativeness. In contrast to previous studies, we propose to investigate this relation based not only on the amount of information given by the abstract but also on its importance. The main objective of this paper is to introduce a new metric called GEM to measure the “generosity” or representativeness of an abstract. Schematically speaking, a generous abstract should have the best possible score of similarity for the sections important to the reader. Based on a questionnaire gathering information from 630 researchers, we were able to weight sections according to their importance. In our approach, seven sections were first automatically detected in the full text. The accuracy of this classification into sections was above 80% compared with a dataset of documents where sentences were assigned to sections by experts. Second, each section was weighted according to the questionnaire results. The GEM score was then calculated as a sum of weights of sections in the full text corresponding to sentences in the abstract normalized over the total sum of weights of sections in the full text. The correlation between GEM score and the mean of the scores assigned by annotators was higher than the correlation between scores from different experts. As a case study, the GEM score was calculated for 36,237 articles in environmental sciences (1930–2013) retrieved from the French ISTEX database. The main result was that GEM score has increased over time. Moreover, this trend depends on subject area and publisher. No correlation was found between GEM score and citation rate or open access status of articles. We conclude that abstracts are more generous in recent publications and cannot be considered as mere teasers. This research should be pursued in greater depth, particularly by examining structured abstracts. GEM score could be a valuable indicator for exploring large numbers of abstracts, by guiding the reader in his/her choice of whether or not to obtain and read full texts.
Introduction
Scientific journals use abstracts to succinctly communicate research results. Acting as separate entities with respect to full papers, abstracts are generally a free material with easy access.
Abstracts of published manuscripts were introduced in the 1950s (Zhang and Liu, 2011). The notion of an abstract is part of everyday language, but its definitions are multiple: the term “abstract” is used loosely to refer to almost any brief account of a longer paper. Most definitions refer to ideal abstracts produced by professional summarizers. Orasan (2001) argues that it is very unlikely that an abstract produced by the author(s) of a paper is intended to be used as a replacement for the whole document. Therefore, we suggest using a simple functional definition of an abstract: “a concise representation of a document's contents to enable the reader to determine its relevance to a specific information” (Johnson, 1995). So, the abstract is no longer a “mirror” of the document; instead it is intended to draw attention to the most important information of the document it is supposed to summarize (Orasan, 2001).
The abstract represents the primary point of entry to a scientific article, a “point de passage obligé” (Callon and Latour, 1991; Crosnier, 1993). In the context of a rapid increase in the number of scientific journals, abstracts are useful to capture a large volume of documents. Abstracts are also an answer to external demands: publishers of some periodicals and the ANSI NISO standard [ANSI/NISO Z39.14-1997 (R2009)] require or recommend specific information that represents the content of texts reporting results of experimental work, or descriptive or discursive studies to be present in abstracts.
Scientific articles typically have a number of different audiences: the referees, who help the journal editor decide whether a paper is suitable for publication; the journal readers themselves, who may be more or less knowledgeable about the topic addressed in the paper1. Most journals ask for between 150 and 200 words for traditional abstracts (i.e., those without subheadings). Structured abstracts, which are divided into a number of named sections, can be longer than traditional ones (Hartley, 2004).
The abstract has been the subject of many research projects, including attempts to evaluate their quality (Narine et al., 1991; Timmer et al., 2003; Sharma and Harrison, 2006; Prasad et al., 2012; Fontelo et al., 2013). In the past two decades, researchers have carried out a number of studies on structured abstracts from different perspectives, and compared abstracts in biomedical journals with those from social sciences journals (see review of James Hartley's research on structured abstracts; Zhang and Liu, 2011).
What we argue here is that the abstract is based on a series of terminological, syntactical and stylistic choices made by the author(s) (Crosnier, 1993). Through a psycholinguistic analysis and readability tests, Guerini et al. (2012) showed that the linguistic style of abstracts contributes to determining the success and viral capability of a scientific article. Scientific texts allow the construction of knowledge claims (Myers, 1985). The act of writing a paper corresponds to an attempt to claim ownership of a new piece of knowledge, which is to be integrated into the repository of scientific knowledge in the author's field by the process of peer review and publication (Teufel et al., 2009).
In this paper, we look at the issue from the perspective of the researcher, who is both an author and a reader. We introduce cognitive processes, i.e., the intention of the author when writing what we call a “generous” or “non-generous” abstract. While the journal may issue instructions for the abstract, in the act of writing, the author2 makes his/her own choices (in terms of terminology, syntax and style) and this is what we aim to catch through our measurement of generosity. Our goal in this paper is to define a set of principles from which the generosity score (of an abstract X to its corresponding full text Y) can be calculated. It differs from previous work in that it weights different sections of the paper by their importance.
In our definition, generosity means more than informativeness (a ratio of Y found in X). Indeed, we could have an abstract that scores excellently compared to the full text it summarizes, but which is not very generous. Schematically speaking, a generous abstract should have the best possible score of similarity with the sections that are important to the reader; sections must therefore be weighted according to their importance in the calculation. Matching sentences from the abstract with those issued from the full text was inspired by the work of (Atanassova et al., 2016), who aimed to compare abstract sentences with sentences issued from a full text.
Our study aims to answer the following research questions:
1) Is the abstract a teaser rather than an exact reflection of the article content? By teaser we mean a promotional device or advert intended to arouse interest or curiosity for what will follow.
2) Are authors who write generous abstracts also generous in providing open access to their work?
3) Has generosity of abstracts evolved over time in the case study field of environmental sciences?
These are the questions addressed in remainder of this paper using text-mining techniques and the voluminous database available from ISTEX, combined with the results of an online questionnaire. In the Related Work section, we clarify the motivation for the work presented and situate the focus of our research. The Materials and Methods section includes the constitution of a dataset of 36,237 articles in the environmental sciences and details the two approaches chosen: on one hand, an online questionnaire on researchers' practices and their relationship with the abstract; on the other hand, the definition of the automatic metric GEM (for GEnerosity Measure) that calculates an abstract's generosity. The Results section presents evaluations of the section classification tool and GEM score. Finally, we conducted an experiment aiming to apply GEM to the defined dataset.
Related Work
Overview of Studies On Scientific Abstract
Our research is relevant to several aspects of the scientific literature, on which we have chosen to focus. First, there is a need to apply text-mining techniques to retrieve information from the ever-increasing number of scientific documents, in order to help researchers identify the most appropriate work to base their future research upon.
Many studies have been conducted to compare scientific texts, particularly between the different contents or versions of a publication: title, abstract, keywords, preprint, and published version. Because of the massive quantities of information produced in biological and medical research within a short period of time and the necessity for researchers to stay up to date, experiments have been carried out in life sciences and medicine to check whether it was worth the effort to mine full texts or whether the title, abstract, and keywords freely available could be sufficient to gain a clear picture of what is relevant and useful. Shah et al. (2003) demonstrated that even though abstracts display many keywords in a small space there is much more relevant information (at least in a ratio of 1:4 regarding gene names, anatomical terms, organism names, etc.) in the rest of the article.
PubMed Central is the most comprehensive index to medical literature and has been pioneering in open access since 1997. It opened the door to the free building of text collections for automatic extraction leading to the first web-based platform in molecular biology, called iHOP (Information Hyperlinked over Proteins)3. By using specific genes and proteins as hyperlinks between sentences and abstracts, the information in PubMed can be converted into one navigable resource. Based on named entity recognition, iHOP processed 14 million abstracts to extract 11 million molecular relationships for 2,700 living organisms (Blaschke et al., 1999). In the field of biomedicine, some studies for drug target discovery (Kafkas et al., 2017) integrated full texts and abstracts into a massive database, successfully mining more than 26 million abstracts and about 1.2 million full texts for 1.1 million target-drug discoveries. However, when considering paragraph-sized segments of full text articles, searching performed on abstracts only is shown to be far less efficient.
Using their own technology to compare 23 million PubMed abstracts and 2.5 million full text biology articles, Elsevier (2015) showed that more relevant and interesting facts are retrieved from a full text corpus than one containing abstracts alone. More recently, with a similar corpus and methodology, Westergaard et al. (2017) came to the same conclusion. In fields other than biology, Klein et al. (2016) investigated the textual similarity of scholarly preprints and their final published counterparts (12,202 published versions of articles on physics, mathematics, statistics, and computer sciences) and found no significant difference between preprints and published versions.
Using the TREC-2007 genomics track test collection (162,259 full text articles assembled in 2006), Lin (2009) showed that treating an entire article as an indexing unit is not consistently more effective than an abstract-only search. However, when considering paragraph-sized segments of full text articles, searching performed on abstracts alone was shown to be far less efficient. These findings are consistent with Corney's (Corney et al., 2004) conclusions showing that the density of ‘interesting' facts found in the abstract is much higher than the corresponding density in the full text.
Scientific papers are highly discursive since they aim to show a view with demonstrative arguments (or proofs). Discourse analysis can help to capture the organization of discursive elements related to argumentation: alternative views, arguments from authority, pros and cons arguments, etc. (Perelman and Olbrechts-Tyteca, 1958; Toulmin, 2003). Khedri et al. (2013) used what they call meta-discourse markers (such as “firstly” and “in conclusion”) that refer explicitly to aspects of the organization of a text. Mann and Thompson (1988) developed a grammar theory called “Rhetorical Structure Theory” (RST) about the recurrent structure of scientific paper content. Teufel and Kan (2011) investigated the potential of weakly-supervised learning for argumentative zoning of scientific abstracts. They chose seven categories of argumentative zone: background, objectives, methods, results, conclusion, related work, and future work. Our work builds upon a method relating to such zoning and introduces weighting of sections from the full text that match content of the abstract.
Automatic Metrics For Summary Evaluation
As far as descriptive statistics are concerned, different notions of “similarity” between texts have been incorporated in text-comparison algorithms. The literature provides many string metrics (also known as a string similarity metrics or string distance functions) that are used for approximate string matching or comparison and in fuzzy string searching, e.g., cosine (Manning et al., 2008), Dice (Sørensen, 1948), or Jaccard similarity (Tanimoto, 1958). Similarity between the full text and an abstract may also be estimated by the number of shared n-grams or longest common subsequence, etc. (Cormen et al., 2009).
Other metrics are more specific to the task of document summarization. The simplest metric is a compression rate, i.e., the proportion of summary length in relation to full text length. This metric is opposed to a retention rate, i.e., the proportion of information retained, which is difficult to formalize (Gholamrezazadeh et al., 2009). Thus, a good summary should have a low compression rate and a high retention rate.
The metrics commonly used in information retrieval, such as recall and precision over the number of terms/sentences appearing in the full text and the abstract (Gholamrezazadeh et al., 2009) could also be applied. The F-measure (Lin, 2004) is less useful in summary analysis than in search engines since it is based on recall, and the results returned by search engines are potentially infinite while a summary is limited.
One of the most commonly used metrics of summary evaluation is the Recall-Oriented Understudy for Gisting Evaluation (ROUGE) family (Lin, 2004): ROUGE-N (n-grams recall), ROUGE-N-MULTI (maximal value of pairwise n-gram recalls), ROUGE-L (longest common substring shared by two sentences), ROUGE-S (shared bigrams which may be separated by other words), ROUGE-SU (unigram smoothing). ROUGE-BE, DemokritosGR2, catholicasc1, and CLASSY1 significantly outperformed ROUGE-2, which is the best performing of all ROUGE variants at the Automatically Evaluating Summaries of Peers (AESOP) task within the Text Analysis Conference (TAC) (Owczarzak et al., 2012). Normalized pairwise comparison LCS-MEAD (Radev et al., 2002) is similar to ROUGE-L, but LCS-MEAD takes the maximal value of longest common substring (LCS), while ROUGE-L deals with the union of LCSs (Hovy and Tratz, 2008). One of the serious shortcomings of LCS is the fact that it does not consider the distance between words. An attempt was made to overcome this drawback by using weighted LCS, which takes into account the length of consecutive matches. LCS-based algorithms are a special case of edit distance (Bangalore et al., 2000).
Campr and JeŽek (2015) proposed to use the similarity within semantic representation such as LSA, LDA, Word2Vec, and Doc2Vec. However, ROUGE-1 outperformed all these metrics. In (Ng and Abrecht, 2015), the ROUGE metric was modified by word embedding, but this variant showed lower results than the standard one.
A Pyramid score is based on the number of repetitions of information in the gold-standard model summaries (Nenkova et al., 2007), which can be replaced by a full text. Because Pyramid score requires heavy manual annotation of both gold-standard and candidate summaries it is not applicable to large corpora.
In (Owczarzak et al., 2012), a responsiveness metric is proposed. This metric shows how well a summary satisfies the user's information need expressed by a given query and is completely manual. Louis and Nenkova (2013) suggest using the full text instead of a set of reference summaries for summary evaluation. They estimated summary score by Kullback–Leibler divergence, Jensen Shannon divergence, and cosine similarity measure. Although these metrics have some correlation with ROUGE score, ROUGE-1 gave better results. In the INEX Tweet Contextualization Track 2011–2014, summaries were evaluated by the Kullback–Leibler divergence and simple log difference (Bellot et al., 2016). The authors state that the Kullback–Leibler divergence is very sensitive to smoothing in case of small numbers of relevant passages in contrast to the absolute log-diff between frequencies (Bellot et al., 2016). Cabrera-Diego et al. (2016) introduced a trivergent model that outperformed the divergence score.
In this paper, our main task is to provide a measure of the generosity of an abstract of a scientific article with regard to the full text. The use of the full text rather than a set of reference summaries for summary evaluation provides low results (Louis and Nenkova, 2013) since traditional metrics are designed for the comparison with summaries created by humans. Thus, they are not appropriate for comparison of an abstract produced by humans with the full text. All these existing metrics have relative values allowing candidate summaries to be ranked, which has two major consequences. First, these measures are not applicable for comparison of an isolated abstract with the full text, e.g., ROUGE score would depend on the length of the full text. Second, it is not possible to compare metric scores for abstracts of different documents.
Another problem with the existing metrics is their output values. Theoretically, the majority of metrics are normalized, but in practice, the values tend to be quite small (usually < 0.2).
Last, but not least, the final drawback is that none of these measures take into account document structure. As demonstrated by Fontelo et al. (2013), “structured abstracts appear to be informative.” One of the metrics considering document structure is BM25F (Robertson et al., 2004) which is a field-based extension of Okapi's BM25 widely used in information retrieval. However, it is not suitable for abstract scoring since it also gives a relative score allowing search result ranking.
In contrast to the state-of-the-art measures listed above, the metric proposed in this paper (GEM) has absolute values in the interval [0,1]. It also considers the importance of different sections by introducing weighting of sections in full text that match with sentences in the abstract. These weightings were determined by an online questionnaire of researchers' opinions described in the next section.
Materials and Methods
Dataset
Our analysis was based on a corpus of articles in the field of environmental sciences published from 1930 to 2013. This corpus was obtained from the Excellence Initiative for Scientific and Technical Information (ISTEX) database4. ISTEX provides the French higher education and research community with online access to scientific archives in all disciplines. At the time of writing of the present article, this archive contains collections of scientific literature from all disciplines, covering journal archives, digital books, databases, text corpora, etc. from the following publishers: Elsevier, Wiley, Springer, Oxford University Press, British Medical Journal, IOP Publishing, Nature, Royal Society of Chemistry, De Gruyter, Ecco Press, Emerald, Brill, and Early English Books Online.
The ISTEX platform provides a set of services via an HTTP-based web Application Programming Interface (API)5 within a RESTful (REpresentational State Transfer) paradigm, i.e., the platform allows access and manipulation of textual representations of resources using a uniform and predefined set of stateless operations. A Graphical User Interface (GUI) is also available as a form of demonstration6. The API enables to search for documents and their metadata. Search results and document metadata in JSON or MODS formats are available on open access, while access to retrieved documents is restricted and requires authentication. Documents are available in the following formats:
• PDF (full text);
• TEI (full text and enrichments);
• XML provided by a publisher;
• Different formats (images, videos, sounds, etc.) corresponding to appendices and publication covers.
We retrieved 66,518 articles (tagged as research-article or article in the ISTEX database) categorized by ISTEX as “Environmental Studies” or “Environmental Science” (according to the Web of Science classification). We selected articles for which we could retrieve a full text and an abstract from the PDF file. Out of the 59,419 article/abstract pairs thus obtained, we then chose to filter out documents having less than four section classes in the full text: 23,181 articles were therefore considered unsuitable for further analysis. The definitive dataset was composed of 36,237 articles (see published dataset of results in Bordignon and Ermakova, 2018).
Online Questionnaire
An online questionnaire was designed to analyze the way in which a sample of researchers read and write abstracts. The questionnaire was developed on the basis of a broad definition of the abstract, which is divided into seven sections. The following definitions of abstract section classes were provided in the questionnaire:
Introduction—Context
This section describes what is already known about the subject in a way that is understandable to researchers from all fields.
Objectives
The aim here is to describe what is not yet known but which can be discovered or answered by the research or reasoning developed in the article.
Methods—Design
This section informs the reader of the techniques and strategies used to conduct the research and demonstrate its validity (for instance, material used, methodological framework, population being studied, data collection process, sample size, etc.).
Results—Observations—Findings
The main results are presented here, accompanied by the data (possibly quantified) that made it possible to characterize them. These may also be negative results that do not support the initial hypothesis.
Conclusions
This part contains the main message of the article. It shows how the results are interpreted and how the initial question from the objectives is answered.
Limits
If any limitations have been identified, they are presented here.
Perspectives
The aim here is to position the results of the study in a more general context in order to show to what extent there has been progress in understanding and how further studies could lead to new developments.
The questionnaire was strictly anonymous (identities, first and last names, contact details, or e-mail addresses were not asked), and no consent was needed as we retrieved no individual information. The questionnaire had no commercial intent, didn't target individuals and participants were informed of their participation in a research project. It was signed by us and respondents were informed of our status. The link to respond was open to anyone and sent via our professional mailing lists and Twitter accounts. The data did not need to be anonymized and are published (Bordignon and Noël, 2018).
This online questionnaire was completed by 630 individuals between 08/24/2016 and 09/27/2016, to whom these definitions were presented. The large majority of respondents are researchers: 50% are PhD students or postdocs, 43% are professors or permanent researchers. Interviewees were asked to provide a maximum of two disciplinary fields (from a list of 12) that characterize their research.
We asked the respondents to rank the seven sections in their respective fields according to the following scale: essential, important, marginal, optional or unusual, or unknown. We also asked if a good abstract is more like a summary or a teaser. They had the opportunity to send us one or two abstracts that they consider successful, and examples of journal names whose abstracts they consider satisfactory (see published dataset of answers; Bordignon and Noël, 2018).
The last question in the questionnaire was about generosity, a concept that we intentionally did not define in the questionnaire: “In your opinion, which section must imperatively be present in the abstract so that it can be qualified as ‘generous'?”
Out of the respondents, 32% considered that the Results—Observations—Findings section must be present in the abstract if it is to be considered generous and 27% thought mentioning the Objectives in the abstract to be a sign of generosity. Conclusions (16%) and Methods—Design (12%) were in third and fourth place in terms of interest, respectively. Introduction—Context (5%), Perspectives (5%), and Limits (3%) were the sections considered to be of least interest with regard to generosity (see Figure 1). These results were then used to weight the sections detected in the full texts, whose equivalents were either found or not found in the abstract. Table 1 shows there was no significant difference among the disciplines the respondents identify themselves with, more particular for fields with more than 30 respondents. There was indeed no need to take various disciplines into account when weighting sections differently.
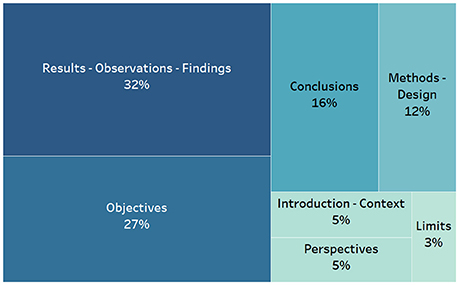
Figure 1. Online questionnaire answers to the question “Which section must imperatively be present in the abstract so that it can be qualified as “generous”?” (630 respondents).
Gem, a Measure of Abstract Generosity
We introduce here a completely automatic metric for the estimation of abstract generosity called GEM (for GEnerosity Measure), which attributes an absolute score [0,1] to an abstract. GEM relies on the importance of the different sections of a scientific paper according to the researchers' opinions obtained from the questionnaire results described above (Figure 2).
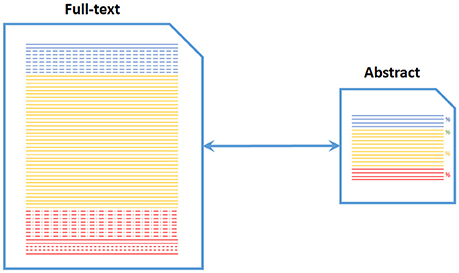
Figure 2. Principle of GEM score as a comparison between the full text and abstract relying on detection of sections.
First of all, we considered that the score calculated by GEM was reliable only if at least four section classes (out of the seven section classes we listed above and submitted to the respondents of the questionnaire) could be automatically identified in the full text using the GROBID tool for section splitting and our algorithm for sentence classification presented below. Otherwise, we considered the estimated score to be unreliable, as GEM is based on the weighting of the detected sections.
Thus, the main steps were the following:
1. Section detection in the full text (using GROBID to split it into sections);
2. Classification of the sections from the full text (position, section embedding, regular expressions, and quantitative features such as number of tables, references, and figures);
3. Sentence splitting in the abstract by Stanford CoreNLP7 (Manning et al., 2014) and estimation of similarity between article sections and corresponding abstract sentences (cosine similarity measure between TF-IDF representations);
4. Calculation of the GEM score. The informativeness rate was weighted according to the importance of the sections.
Figure 3 presents the flow diagram of the algorithm. This model was implemented in Java (Ermakova, 2018).
Article Section Detection
The first step of our algorithm is section detection by GROBID software8. GeneRation Of BIbliographic Data (GROBID) is a machine-learning library for parsing PDF documents into structured TEI format designed for technical and scientific publications. The tool was conceived in 2008 and became available in open source in 2011. Its applications include ResearchGate, HAL Open Access repository, the European Patent Office, INIST, Mendeley and CERN.
GROBID enables:
• Header extraction and parsing from articles in PDF format (e.g., extraction of title, abstract, authors, affiliations, keywords, address, etc.);
• Reference extraction and parsing from articles in PDF format, including references in footnotes, isolated references, and patent references;
• Parsing of dates;
• Full text extraction from PDF articles with document segmentation.
Extraction and parsing algorithms use the Wapiti CRF (Conditional Random Fields) library9. Wapiti is a toolkit for segmenting and labeling sequences with discriminative models based on maximum entropy Markov models and linear-chain CRF. GROBID is available in batch mode, as well as RESTful and JAVA APIs. We integrated GROBID in our tool using JAVA API.
Section Classification
After extracting sections from a PDF article we classified them into the seven classes described below. As a first step, we classified the sections into four classes: INTRO, METHODS, RESULTS, and CONCLUSION, according to the following rules. The rules were applied as it is given in Figure 3. Thus, only one rule can be applied (i.e. only one section is assigned) since if a rule is activated the following rules are not evaluated. We looked for section embedding based on section numbers if they were provided by GROBID or analysis of empty sections with titles only; otherwise, we considered that a section was not embedded in another, i.e., that it was not a subsection. If a section was a subsection, it was assigned the class of its parent; otherwise, we tried to apply regular expressions to a section title in order to classify it (see Table 2). If the title did not match any regular expression, we analyzed its relative position in the text, e.g., the first section was considered to be the INTRO. If none of the previous rules were applicable, we assigned the class RESULTS if the section contained figures or tables, or the class INTRO if it contained more than five references. The default class was METHODS.
Second, we applied regular expressions for searching for sentences related to OBJECTIVES in sections attributed to INTRO and sentences referring to LIMITS and PERSPECTIVES in sections already assigned the class CONCLUSION. Splitting into sentences was performed by Stanford CoreNLP.
Words in regular expressions were considered as representative, but we are aware that they are not exhaustive.
Abstract Sentence Splitting and Classification
Our approach to abstract segmentation is inspired by the work of Atanassova et al. (2016), which aimed to compare abstract sentences with sentences issued from a full text. At this step, splitting into sentences was performed by Stanford CoreNLP. Then, we searched for the most similar sentence in the full text and assigned its class to the abstract sentence under consideration. Thus, only one class can be assigned the class of the section that contains the sentence the most similar to the sentence from the abstract under consideration.
Many researchers consider text content as weighted phrases (Radev and McKeown, 1998; Erkan and Radev, 2004; Seki, 2005). Phrases are often identified by their frequency in a document or collection or by their distribution in a text.
We hypothesized that TF-IDF cosine similarity should be suitable for capturing similarity between sentences. TF-IDF is a short for term frequency–inverse document frequency. It is a numerical statistics that reflects how important a word is to a document in a corpus. A TF-IDF score is achieved with a high term frequency in the document and a low document frequency of the term in the collection. IDF refers to term specificity. As a term appears in more documents, the IDF (and, therefore, TF-IDF) becomes closer to 0. Hence, the weights tend to filter out common terms. We tested the hypothesis that the TF-IDF measure is able to capture keywords by comparison with author-provided keywords and expert analysis. More than 70% of the top words retrieved by the TF-IDF measure coincided with human-provided keyword lists.
Thus, we applied the TF-IDF-based cosine similarity measure between an abstract sentence Sa and a sentence from the full text Si:
where Saj and Sij are TF-IDF scores of the term j in Sa and Si, respectively, and |V| is vocabulary size. Then, we selected the sentence with the maximal cosine similarity and assigned its class to Sa.
It should be noticed here that, in contrast to section classification in the full text, classification in the abstract is performed based on the similarity with sentences from the full text only. Thus, we do not directly consider the regular expressions mentioned above. This decision makes impossible to use key phrases to a trigger section score without any relation to the full text, e.g., the use of the phrase “we report our results” without actually reporting any results does not necessarily provoke the assignment of the result section score. However, the quality of the full text is out of scope of this research.
GEM Score
The GEM score is an interval [0,1]. If we detected less than four section classes in a full text, we assigned the score −1. This was motivated by the fact that GEM is based on section detection and classification and we believe that our score is more reliable in cases where we detect at least four section classes. The GEM score was calculated as a sum of weights of section classes w(sc) retrieved both in an abstract and a full text normalized over the total sum of weights of section classes in a full text:
where FTSC denotes section classes in the full text, ASC refers to section classes in the abstract, wsc corresponds to section weight. Dividing by the sum of all weights of sections from the full text penalizes abstracts that do not reflect sections from the full text, e.g., an abstract representing only result section would have lower score that an abstract of the same length that contains also limits. However, an abstract that presents limits only would be scored lower than an abstract that only details results. GEM does not consider the number nor the length of sentences in the abstract that reflect different full text sections. It measures the presence/absence of the sections in the abstract weighted by their importance according to the scientific community.
Examples of GEM score calculation are given for two articles having different contents and styles above (Example 1 and Example 2).
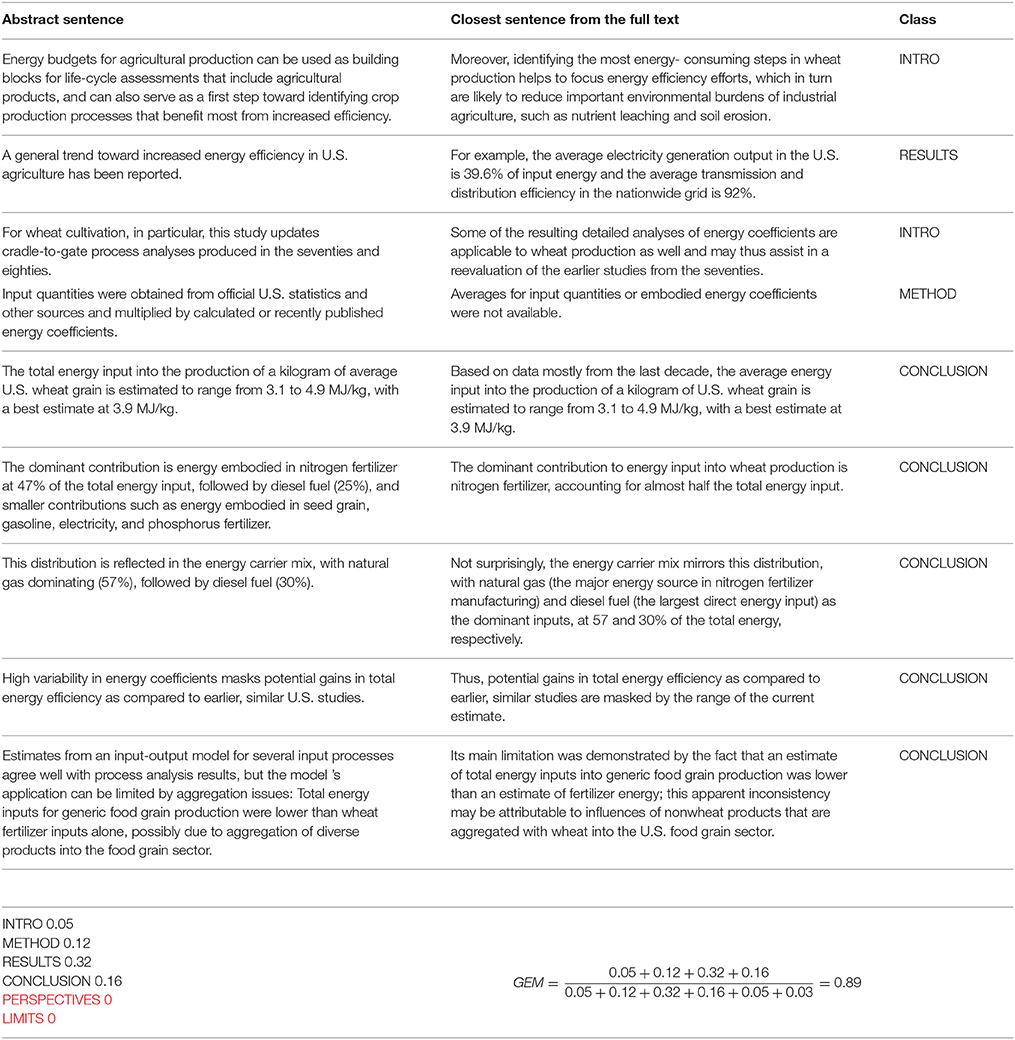
Example 1. GEM score calculation for Piringer and Steinberg (2008).
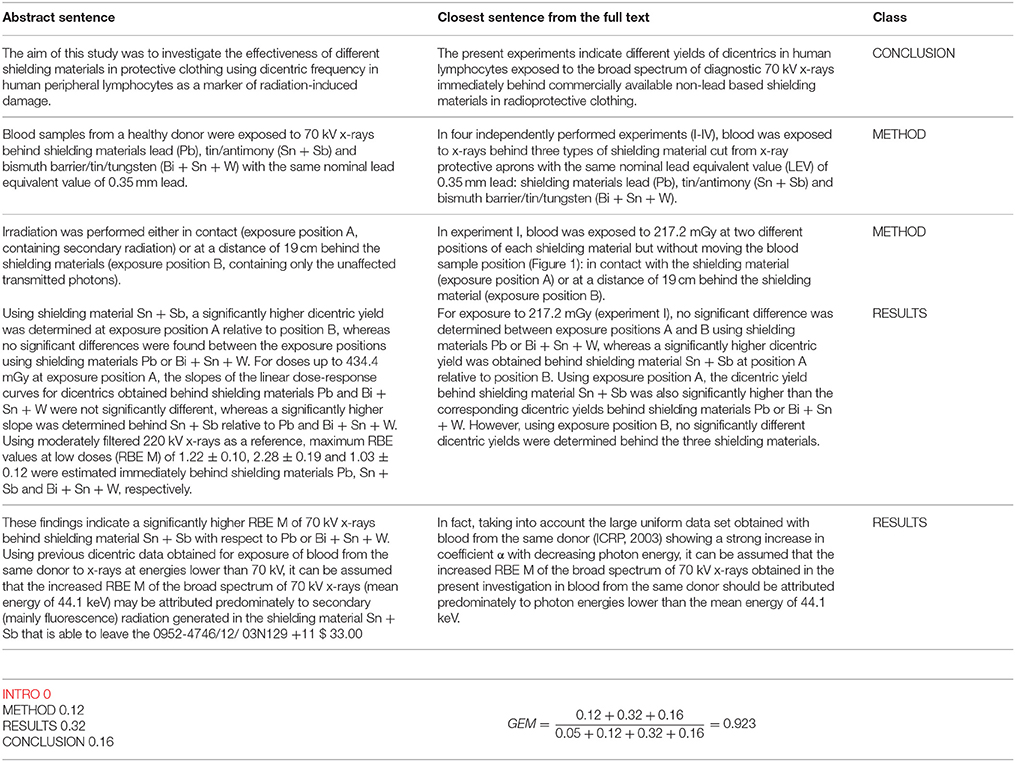
Example 2. GEM score calculation for Schmid et al. (2012).
Results
Section Classification Evaluation
Section classification evaluation was performed over a dataset annotated manually. For manual evaluation, we chose 20 documents at random. For each article, each sentence was tagged by two experts who are both researchers. The first of these experts has expertise in chemistry and the other has experience in economics and environmental sciences. We treated about 4,000 classified sentences. The quality of our classification algorithm was evaluated by a commonly used metric, namely accuracy. Accuracy of our classification was calculated as the number of correctly classified items over the total number of items and was found to be above 80%.
GEM Score Evaluation
We conducted three types of experiment to evaluate the GEM score.
In the first evaluation experiment, we hypothesized that the score assigned to the abstract of a given article should be higher than the score of the abstract coming from another article. Thus, we compared the score assigned to the original abstract with the scores of all other abstracts from the test set. We obtained 25% errors, i.e., in 25% of cases the scores of abstracts corresponding to other articles were higher than the scores of the original ones, while the random score produced 55% of errors on the same dataset. In all cases, the errors of GEM were produced for non-generous original abstracts.
We compared the GEM score with the scores assigned by the experts as in the previous subsection. Forty-two documents were annotated at least by one expert and 20 of these documents were assigned a score by both evaluators. The correlation between GEM scores and the mean of the human assigned scores was 0.59. The correlation between the human annotators was 0.56. We can thus conclude that GEM score reliability is comparable human reliability.
The intuition underlying the third evaluation framework is that a good metric should assign a high score to a generous abstract and a low score to a non-generous one. Rather than calculating the correlation between the scores assigned to abstracts by assessors and metrics, we propose to compare the accuracy, i.e., the percentage of cases where a very generous summary is scored lower than a non-generous one. The motivation is the relative simplicity for a human to distinguish very generous abstracts and abstracts that are not generous at all. We considered only not conflicting assignments as the ground truth. We manually chose 19 generous abstracts and 12 non-generous ones for which we could calculate GEM score. Thus, we had 19 * 12 = 228 pairs for which we know the preferences. In 90% of cases we obtained a higher score for generous abstracts than for non-generous ones. GEM scores are plotted on Figure 4.
Experimental Results
We calculated the GEM score for articles from the definitive dataset (n = 36,237) (see Figure 5).
The most frequent GEM value, 0.6447, occurred 4,683 times. As shown in Table 3, this value was attributed to abstracts where three section types (INTRO, METHODS, and RESULTS) were detected in the abstract out of four found in the full text (OBJECTIVES was missing in the abstract). The second largest value (0.4868) corresponds to detection of INTRO and RESULTS in the abstract while four section types are found in the full text (INTRO, OBJECTIVES, METHODS, and RESULTS). INTRO, METHODS, RESULTS, and CONCLUSION are section types that our algorithm looks for at the first stage. They are often organized as well-defined blocks of text in the articles. These results suggest that the sections INTRO, METHODS, and RESULTS are the most frequently presented in the abstract.
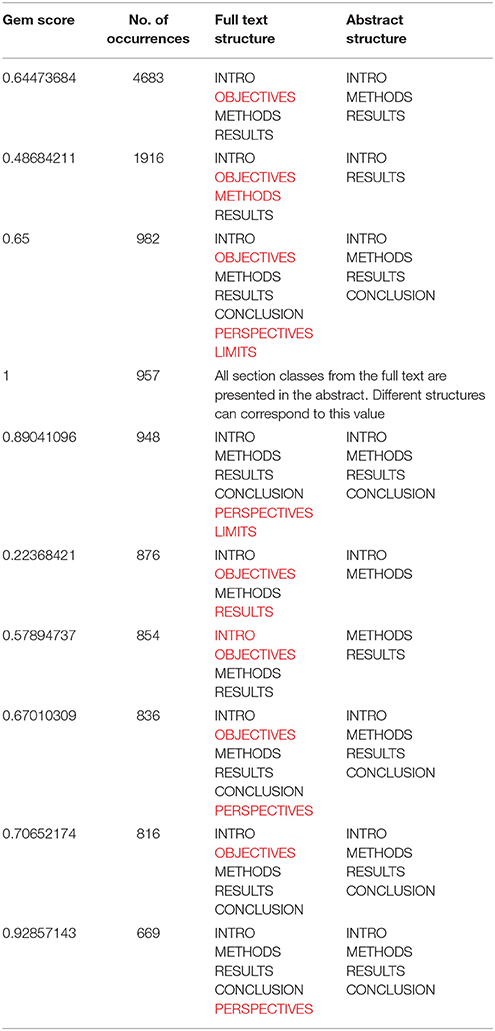
Table 3. Numbers and typology of abstracts according to the structure of the full text (sections missing from the abstract appear in red).
As Figure 6 shows for articles published in the last 40 years, we detected that abstracts tended to become more generous over time. We did not take the period 1930–1975 into account because of the small number of articles.
The fall in the number of articles in 2002 shown in Figure 6 is inherent to the ISTEX database and more particularly to the end of data acquisition from Elsevier. The number of the remaining articles is still significant because it is above 500 articles a year. This fall in numbers had no effect on the growth of the GEM score over time.
In order to illustrate the GEM score potential, we ambitiously propose additional analyses even if they appear to be premature.
Nine publishers were identified in the definitive dataset (Table 4). Half of the dataset articles were published in an Elsevier journal.
We found significant differences between publishers: abstracts from Sage and Springer journals appeared to be less generous than those of other publishers (see Figure 7). These results need to be further investigated in order to identify whether the guidelines for authors or even instructions about structured abstracts could have impacted this trend.
The environmental sciences dataset we tested also includes articles from journals categorized in one or more additional subject areas (according to the Elsevier journal classification).
Table 5 shows the distribution among subject areas and Figure 8 compares the seven most important subject areas excluding environmental sciences that are obviously common to all articles.
This provided an opportunity to compare GEM score between disciplines. No significant differences were found except for the abstracts of articles in the social sciences (n = 3,494) which were the least generous. In the commentaries collected in our online questionnaire, we also came across views consistent with this conclusion:
“In the field of literary studies, we do not have any abstract of this kind […]. I tried to answer your questionnaire anyway, but this type of publication is simply not part of our practice (we're talking about articles, codified but not as rigidly).”
These results need to be investigated further, including making a comparison with a social sciences corpus that could also be retrieved from the ISTEX database.
Finally, we used the oaDOI API10 to look for open access versions of the articles. As far as we know, literature about openness and open access to publications does not deal with abstract content. So we aimed to identify whether authors who wrote generous abstracts were also generous in providing open access to their work. There are two routes for achieving open and unrestricted access: the green and the gold routes. The green route is based on the idea of authors making their work publicly accessible by depositing their manuscripts in a repository, or freely-accessible database. Under the gold route, publications are made open access through publishers' websites. We found no significant difference between mean GEM scores for open access articles (0.57) and non-open access articles (0.58), even with the most recently published articles in the dataset (see Table 6).
There is clearly not a perfect correlation between the GEM score and the mean citation rate (see Table 7), but it should be noted that the lowest citations rates were for the articles with the lowest scores (≤0.4).
Conclusion and Perspectives
In this paper we introduce the notion of generosity of an abstract in relation to the full text that it is supposed to summarize. We developed this concept with a user study (an online questionnaire) in which we questioned researchers.
We propose a new, completely automatic, measure of abstract generosity with absolute values in the interval [0,1], which differs from the state-of-the-art informativeness metrics. Our score (GEM) considers the importance of different sections by introducing the weighting of sections from the full text that match with sentences in the abstract. The accuracy of section splitting and section classification compared with human judgment is above 80%. The error rate of the GEM score compared with scores assigned by experts is not entirely satisfactory but it could be better with improvements to the GEM formulation.
GEM scores show differences among publishers and subject areas based on the analysis of a large corpus in the environmental sciences.
Our results show that GEM scores have increased over time. The evolution of scores over time is consistent with a codification in the writing of articles. The IMRaD structure, which was widely adopted in health sciences journals in the 1980s (Sollaci and Pereira, 2004), was pioneering in the growing use of standards and reporting guidelines developed in the 1990s through 2010s.
Results suggest that abstracts are more generous in recent publications than earlier ones and cannot be considered as mere teasers. These findings are consistent with those of the questionnaire: when asked about the abstract, 74% of respondents considered it as a summary while only 26% considered it a teaser. The questionnaire results provide also section importance weightings, a unique and very useful information.
One of the possible improvements of the proposed measure is to revise the rules we used for section classification to include regular expressions. We also need to supplement the list of words used in the latter. Another means of improvement would be to learn section weights from an annotated corpus.
This research does start the process of measuring the quality of an abstract. It could be taken further, in particular by exploiting structured abstracts that are included in the dataset. It would be interesting to calculate GEM scores for such abstracts, which have a structure imposed by the journals or publishers, and to compare them with those written without guidelines.
Recommendation systems have emerged recently because document databases enable learning from usage. A user can hence define by their own usage a small pool of interesting documents from which recognition will be made for language modeling (Beel et al., 2016). The proposed measure, based on a series of choices made by author(s) and reader(s), is user-oriented. Following our preliminary results, we suggest that GEM score could be a promising recommendation concept and approach. It could be a valuable indicator in exploring a large amount of documents by guiding the reader in his/her choices. It could also be a valuable indicator for exploring a large number of abstracts by guiding the reader in his/her choice of whether to obtain the full text to read it or not. Combined with price information, it could also provide useful information for researchers who have very limited access to journal subscriptions from their institutions and who are thus forced to purchase individual articles on a limited budget.
Ethics Statement
An ethics approval was not required as per the Institution's guidelines and national regulations and the consent of the participants was obtained by virtue of survey completion.
Author Contributions
MN, FB, and NT initiated the study and designed the work with LE. LE collected the data and wrote the code. LE and FB made the calculations. FB and MN designed the online questionnaire. LE, FB, and MN participated equally in the analysis of the results, the drawing of conclusions and the writing of most of the manuscript. NT contributed in the state-of-the-art.
Funding
The work was supported by the ISTEX project (reference: ANR-10-IDEX-0004, Chantiers d'usage program) under the acronym FULLAB. LE was funded through a postdoctoral scholarship at the Université de Lorraine in partnership with the LISIS.
Conflict of Interest Statement
The authors declare that the research was conducted in the absence of any commercial or financial relationships that could be construed as a potential conflict of interest.
Footnotes
1. ^ https://www.nature.com/scitable/topicpage/scientific-papers-13815490
2. ^ In case of a multiple authorship, we make the hypothesis that the authorship is endorsed collectively.
3. ^ http://www.ihop-net.org (Accessed December 2017)
5. ^ https://api.istex.fr/documentation/
7. ^ https://stanfordnlp.github.io/CoreNLP/
References
Atanassova, I., Bertin, M., and Larivière, V. (2016). On the composition of scientific abstracts. J. Document. 72, 636–647. doi: 10.1108/JDOC-09-2015-0111
Bangalore, S., Rambow, O., and Whittaker, S. (2000). “Evaluation metrics for generation,” in Proceedings of the First International Conference On Natural Language generation (Mitzpe Ramon), 1–8.
Beel, J., Gipp, B., Langer, S., and Breitinger, C. (2016). Research-paper recommender systems: a literature survey. Int. J. Digit. Libr. 17, 305–338. doi: 10.1007/s00799-015-0156-0
Bellot, P., Moriceau, V., Mothe, J., SanJuan, E., and Tannier, X. (2016). INEX tweet contextualization task: evaluation, results and lesson learned. Inform. Process. Manage. 52, 801–819. doi: 10.1016/j.ipm.2016.03.002
Blaschke, C., Andrade, M. A., Ouzounis, C. A., and Valencia, A. (1999). Automatic extraction of biological information from scientific text: protein-protein interactions. Proc. Int. Conf. Intell. Syst. Mol. Biol. 7, 60–67.
Bordignon, F., and Ermakova, L. (2018). Data for: ‘Is the abstract a mere teaser? Evaluating generosity of article abstracts in the environmental sciences’ 1. doi: 10.17632/j39gjcjz5p.1
Bordignon, F., and Noël, M. (2018). Données d'enquête pour la construction d'un indice de générosité des abstracts 1. doi: 10.17632/43trgycgmh.1
Adrián, L., Torres-Moreno, J.-M., and Durette, B. (2016). “Evaluating multiple summaries without human models: a first experiment with a trivergent model,” in Natural Language Processing and Information Systems: 21st International Conference on Applications of Natural Language to Information Systems, NLDB 2016, Salford, UK, June 22-24, 2016, Proceedings, eds E. Métais, F. Meziane, M. Saraee, V/ Sugumaran, and S. Vadera (Cham: Springer International Publishing), 91–101. doi: 10.1007/978-3-319-41754-7_8
Campr, M., and JeŽek, K. (2015). “Comparing semantic models for evaluating automatic document summarization,” in Text, Speech, and Dialogue: 18th International Conference, TSD 2015, Pilsen,Czech Republic, September 14-17, 2015, Proceedings, eds P. Král and V. Matoušek (Cham: Springer International Publishing), 252–260. doi: 10.1007/978-3-319-24033-6_29
Cormen, T. H., Leiserson, C. E., Rivest, R. L., and Stein, C. (2009). Introduction to Algorithms, 3rd Edn. Cambridge, MA; London, UK: The MIT Press.
Corney, D. P., Buxton, B. F., Langdon, W. B., and Jones, D. T. (2004). BioRAT: extracting biological information from full-length papers. Bioinformatics 20, 3206–3213. doi: 10.1093/bioinformatics/bth386
Crosnier, E. (1993). L'abstract scientifique anglais - français : contraintes et libertés. ASp. Rev. GERAS 2, 177–198. doi: 10.4000/asp.4287
Erkan, G., and Radev, D. R. (2004). LexRank: graph-based lexical centrality as salience in text summarization. J. Artif. Intell. Res. 22, 457–479.
Ermakova, L. (2018). GEM: measure of the generosity of the abstract comparing to the full text. doi: 10.5281/zenodo.1162951
Elsevier (2015). Extracting Value from Scientific Literature: The Power of Mining Full-Text Articles for Pathway Analysis Harnessing the Power of Content. Available online at: https://www.elsevier.com/__data/assets/pdf_file/0016/83005/R_D-Solutions_Harnessing-Power-of-Content_DIGITAL.pdf
Fontelo, P., Gavino, A., and Sarmiento, R. F. (2013). Comparing data accuracy between structured abstracts and full-text journal articles: implications in their use for informing clinical decisions. Evid. Based Med. 18, 207–211. doi: 10.1136/eb-2013-101272
Gholamrezazadeh, S., Salehi, M. A., and Gholamzadeh, B. (2009). “A comprehensive survey on text summarization systems,” 2nd International Conference on Computer Science and Its Applications (Jeju), 1–6.
Guerini, M., Pepe, A., and Lepri, B. (2012). “Do linguistic style and readability of scientific abstracts affect their virality?,” in ArXiv:1203.4238 [Cs]. Proceedings of the Sixth International AAAI Conference on Weblogs and Social Media (ICWSM 2012) (Dublin). Available online at: http://arxiv.org/abs/1203.4238
Hartley, J. (2004). Current findings from research on structured abstracts. J. Med. Libr. Assoc. 92, 368–371. doi: 10.3163/1536-5050.102.3.002
Hovy, E., and Tratz, S. (2008). “Summarization evaluation using transformed basic elements,” in Proceedings TAC 2008 (Gaithersburg, MD).
Johnson, F. (1995). Automatic abstracting research. Libr. Rev. 44, 28–36. doi: 10.1108/00242539510102574
Kafkas, S., Dunham, I., and McEntyre, J. (2017). Literature evidence in open targets - a target validation platform. J. Biomed. Seman. 8:20. doi: 10.1186/s13326-017-0131-3
Khedri, M., Heng, C. S., and Ebrahimi, S. F. (2013). An exploration of interactive metadiscourse markers in academic research article abstracts in two disciplines. Discour. Stud. 15, 319–331. doi: 10.1177/1461445613480588
Klein, M., Broadwell, P., Farb, S. E., and Grappone, T. (2016). “Comparing published scientific journal articles to their pre-print versions,” in Proceedings of the 16th ACM/IEEE-CS on Joint Conference on Digital Libraries (New Jersey, NJ) 153–162. doi: 10.1145/2910896.2910909
Lin, C.-Y. (2004). “ROUGE: a package for automatic evaluation of summaries,” in Text Summarization Branches Out: Proceedings of the ACL-04 Workshop (Barcelona).
Lin, J. (2009). Is searching full text more effective than searching abstracts? BMC Bioinformatics 10:46. doi: 10.1186/1471-2105-10-46
Louis, A., and Nenkova, A. (2013). Automatically assessing machine summary content without a gold standard. Comput. Linguist. 39, 267–300. doi: 10.1162/COLI_a_00123
Mann, W. C., and Thompson, S. A. (1988). Rhetorical structure theory: toward a functional theory of text organization. Text Interdiscipl. J. Study Disc. 8, 243–281. doi: 10.1515/text.1.1988.8.3.243
Manning, C. D., Raghavan, P., and Schütze, H. (2008). Introduction to Information Retrieval. New York, NY: Cambridge University Press.
Manning, C. D., Surdeanu, M., Bauer, J., Finkel, J., Bethard, S. J., and McClosky, D. (2014). “The Stanford CoreNLP natural language processing toolkit,” in Proceedings of 52nd Annual Meeting of the Association for Computational Linguistics: System Demonstrations (Baltimore, MD), 55–60. Available online at: http://www.aclweb.org/anthology/P/P14/P14-5010.
Myers, G. (1985). Texts as knowledge claims: the social construction of two biology articles. Soc. Stud. Sci. 15, 593–630. doi: 10.1177/030631285015004002
Narine, L., Yee, D. S., Einarson, T. R., and Ilersich, A. L. (1991). Quality of abstracts of original research articles in CMAJ in 1989. Canad. Med. Assoc. J. 144:449.
Nenkova, A., Passonneau, R., and McKeown, K. (2007). The pyramid method: incorporating human content selection variation in summarization evaluation. ACM Trans. Speech Lang. Process. 4:4. doi: 10.1145/1233912.1233913
Ng, J.-P., and Abrecht, V. (2015). “Better summarization evaluation with word embeddings for ROUGE,” in Proceedings of the 2015 Conference on Empirical Methods in Natural Language Processing, 1925–1930 (Lisbon: Association for Computational Linguistics). Available online at: http://aclweb.org/anthology/D15-D1222
Orasan, C. (2001). “Patterns in scientific abstracts,” in Proceedings of Corpus Linguistics 2001 Conference, eds P. Rayson, A. Wilson, T. McEnery, A. Hardie, and S. Khoja (Lancaster), 433–443.
Owczarzak, K., Conroy, J. M., Dang, H. T., and Nenkova, A. (2012). “An assessment of the accuracy of automatic evaluation in summarization,” in Proceedings of Workshop on Evaluation Metrics and System Comparison for Automatic Summarization (Stroudsburg, PA: Association for Computational Linguistics), 1–9. Available online at: http://dl.acm.org/citation.cfm?id=2391258.2391259
Perelman, C., and Olbrechts-Tyteca, L. (1958). Traité de L'argumentation. Logos (Bucureşti. 1996), T. 1. Presses Universitaires de France. Available online at: https://books.google.fr/books?id=CEA6RAAACAAJ
Prasad, S., Lee, D. J., Yuan, C., Barao, V. A., Shyamsunder, N., and Sukotjo, C. (2012). Discrepancies between Abstracts Presented at International Association for Dental Research Annual Sessions from 2004 to 2005 and Full-Text Publication. Int. J. Dent. 2012, 1–7. doi: 10.1155/2012/859561
Piringer, G., and Steinberg, L. J. (2008). Reevaluation of energy use in wheat production in the United States. J. Indus. Ecol. 10, 149–167. doi: 10.1162/108819806775545420
Radev, D. R., and McKeown, K. R. (1998). Generating natural language summaries from multiple on-line sources. Comput. Linguist. Spec. Iss. Nat. Lang. Generat. 24, 470–500.
Radev, D., Teufel, S., Saggion, H., Lam, W., Blitzer, J., Elebi, A., et al. (2002). Evaluation of Text Summarization in a Cross-Lingual Information Retrieval Framework. Baltimore, MD: Center for Language and Speech Processing, Johns Hopkins University.
Robertson, S., Zaragoza, H., and Taylor, M. (2004). “Simple BM25 extension to multiple weighted fields,” in Proceedings of the Thirteenth ACM International Conference on Information and Knowledge Management, CIKM'04 (New York, NY: ACM), 42–49. doi: 10.1145/1031171.1031181
Schmid, E., Panzer, W., Schlattl, H., and Eder, H. (2012). Emission of fluorescent x-radiation from non-lead based shielding materials of protective clothing: a radiobiological problem? J. Radiol. Protect. 32, N129–N139. doi: 10.1088/0952-4746/32/3/N129
Seki, Y. (2005). Automatic summarization focusing on document genre and text structure. ACM SIGIR Forum 39, 65–67. doi: 10.1145/1067268.1067294
Shah, P. K., Perez-Iratxeta, C., Bork, P., and Andrade, M. A. (2003). Information extraction from full text scientific articles: where are the keywords? BMC Bioinformatics 4:20. doi: 10.1186/1471-2105-4-20
Sharma, S., and Harrison, J. E. (2006). Structured abstracts: do they improve the quality of information in abstracts? Am. J. Orthodont. Dentofac. Orthoped. 130, 523–530. doi: 10.1016/j.ajodo.2005.10.023
Sollaci, L. B., and Pereira, M. G. (2004). The introduction, methods, results, and discussion (IMRAD) structure: a fifty-year survey. J. Med. Libr. Assoc. 92, 364–367.
Sørensen, T. J. (1948). A Method of Establishing Groups of Equal Amplitude in Plant Sociology Based on Similarity of Species Content and Its Application to Analyses of the Vegetation on Danish Commons. Biologiske Skrifter. I kommission hos E. Munksgaard. Available online at: https://books.google.fr/books?id=rpS8GAAACAAJ
Tanimoto, T. T. (1958). An Elementary Mathematical Theory of Classification and Prediction. International Business Machines Corporation. Available online at: https://books.google.de/books?id=yp34HAAACAAJ
Teufel, S., and Kan, M.-Y. (2011). “Robust argumentative zoning for sensemaking in scholarly documents,” in Advanced Language Technologies for Digital Libraries, eds R. Bernardi, S. Chambers, B. Gottfried, F. Segond, and I. Zaihrayeu, Vol. 6699 (Berlin; Heidelberg: Springer), 154–170. doi: 10.1007/978-3-642-23160-5_10
Teufel, S., Siddharthan, A., and Batchelor, C. (2009). “Towards discipline-independent argumentative zoning: evidence from chemistry and computational linguistics,” in EMNLP ′09 Proceedings of the 2009 Conference on Empirical Methods in Natural Language Processing, Vol. 3 (Stroudsburg, PA), 1493–1502. doi: 10.3115/1699648.1699696
Timmer, A., Sutherland, L. R., and Hilsden, R. J. (2003). Development and evaluation of a quality score for abstracts. BMC Med. Res. Methodol. 3:2. doi: 10.1186/1471-2288-3-2
Westergaard, D., Stærfeldt, H.-H., Tønsberg, C., Jensen, L. J., and Brunak, S. (2017). Text mining of 15 million full-text scientific articles. BioRxiv. Available online at: http://www.biorxiv.org/content/early/2017/07/11/162099
Keywords: abstract, full text, generosity, environmental sciences, measure, metric, scientific articles, text-mining
Citation: Ermakova L, Bordignon F, Turenne N and Noel M (2018) Is the Abstract a Mere Teaser? Evaluating Generosity of Article Abstracts in the Environmental Sciences. Front. Res. Metr. Anal. 3:16. doi: 10.3389/frma.2018.00016
Received: 31 January 2018; Accepted: 23 April 2018;
Published: 15 May 2018.
Edited by:
Iana Atanassova, Université Bourgogne Franche-Comté, FranceReviewed by:
Chengzhi Zhang, Nanjing University of Science and Technology, ChinaKevin Boyack, SciTech Strategies, Inc., United States
Copyright © 2018 Ermakova, Bordignon, Turenne and Noel. This is an open-access article distributed under the terms of the Creative Commons Attribution License (CC BY). The use, distribution or reproduction in other forums is permitted, provided the original author(s) and the copyright owner are credited and that the original publication in this journal is cited, in accordance with accepted academic practice. No use, distribution or reproduction is permitted which does not comply with these terms.
*Correspondence: Liana Ermakova, liana.ermakova@univ-brest.fr