- 1School of Information Management, Nanjing University, Nanjing, China
- 2Department of Psychiatry, University of Illinois at Chicago, Chicago, IL, USA
Within well-established fields of biomedical science, we identify “gaps,” topical areas of investigation that might be expected to occur but are missing. We define a field by carrying out a topical PubMed query and analyze Medical Subject Headings (MeSH) by which the set of retrieved articles are indexed. MeSH terms that occur in >1% of the articles are examined pairwise to see how often they are predicted to co-occur within individual articles (assuming that they are independent of each other). A pair of MeSH terms that are predicted to co-occur in at least 10 articles, yet are not observed to co-occur in any article, are “gaps” and were studied further in a corpus of 10 disease-related article sets and 10 related to biological processes. Overall, articles that filled gaps were cited more heavily than non-gap-filling articles and were 61% more likely to be published in multidisciplinary high-impact journals. Nine different features of these “gaps” were characterized and tested to learn which, if any, correlate with the appearance of one or more articles containing both MeSH terms within the next 5 years. Several different types of gaps were identified, each having distinct combinations of predictive features: (a) those arising as a byproduct of MeSH indexing rules; (b) those having little biological meaning; (c) those representing “low hanging fruit” for immediate exploitation; and (d) those representing gaps across disciplines or subdisciplines that do not talk to each other or work together. We have built a free, open tool called “Mine the Gap!” that identifies and characterizes the “gaps” for any PubMed query, which can be accessed via the Anne O’Tate value-added PubMed search interface (http://arrowsmith.psych.uic.edu/cgi-bin/arrowsmith_uic/AnneOTate.cgi).
Introduction
Although no single theoretical framework covers all types of scientific discoveries, several scholars have pointed out that new discoveries often involve new combinations of existing concepts or ideas (Swanson and Smalheiser, 1997; Chen et al., 2009; Uzzi et al., 2013; reviewed in Chen, 2014). For example, published articles often present preexisting text terms (Packalen and Bhattacharya, 2015), Medical Subject Headings (MeSH) terms (Theodosiou et al., 2011; Mishra and Torvik, 2016) or citations to journals (Uzzi et al., 2013) that are combined in novel ways not seen before. It is not clear whether articles that combine novel pairs of MeSH terms will necessarily result in more impactful or important research as assessed by (say) patents or citations. However, pairs of MeSH terms have a certain appeal as an objective measure of information flow, since one can examine the overall number of new MeSH pairs that appear over time within a given field of investigation, as well as attempt to predict which new MeSH pairs are most likely to appear.
In previous investigations, we have studied the potential benefit of identifying disparate areas of scientific investigation that are disconnected—that is, they reside in two different sets of articles that do not share authors and are poorly cross-cited or co-cited—yet they contain information that, when connected, leads to promising and testable new hypotheses (Swanson and Smalheiser, 1997; Torvik and Smalheiser, 2007; Smalheiser et al., 2009). The presumption is that connections between disparate areas of investigation are likely to be overlooked (due to lack of reading widely enough by scientists), neglected (e.g., because they do not correspond to existing mainstream topics), or disfavored as implausible or meaningless (e.g., one might not expect much insight to emerge from connecting studies of in vitro fertilization and handgun safety) (Swanson, 1986).
Here, we consider the natural history of unconnected topics of investigation that reside WITHIN a single well-established field of study. MeSH term pairs that have expected co-occurrences of 10 or more within a given field but do not co-occur in any articles in that field are defined as “gaps.” That is, we seek to bridge a set of articles that are indexed by MeSH term 1 and those that are indexed by MeSH term 2, all within the larger set of articles represented by a topical PubMed query. Such gaps are less likely to represent neglected or overlooked relationships, and in fact, some of the unconnected topics might represent low-hanging fruit that investigators will explore with high priority in the near future.
In the present paper, we have identified gaps from a variety of topical PubMed queries, comprising 10 disease-related article sets and 10 related to biological processes. The gaps have been characterized in terms of nine different features at one initial time window (1987–2005). We then identified articles appearing in the same field in the subsequent 5-year time window (2006–2010) (Yetisgen-Yildiz and Pratt, 2009) and looked at those that did vs. did not fill one or more gaps (that is, articles dual indexed with both MeSH terms of the gap). We hoped to learn how gap-filling articles differ from those that do not fill gaps. Moreover, comparing different gaps among each other, we assessed whether we can identify features that correlate with the likelihood that the gap will be filled in the second time window and with the number of articles that fill that gap.
Materials and Methods
A total of 20 PubMed queries were carried out using the PubMed eUtils API, representing 10 diseases and 10 biological processes (Table 1). These were chosen to cover a wide range of topical areas, pathologies, and tissue systems and were divided into two time slices. The first time slice consisted of articles with publication dates 1987–2005 inclusive (we did not include articles earlier than 1987 because the number and indexing of MeSH terms has evolved substantially over time). The second time slice consisted of articles with publication dates 2006–2010 inclusive, i.e., the 5-year period following the first time slice. As shown in Table 1, the number of articles in the first time slice ranged between 7,000 and 50,000, and the new articles appearing in the second time slice increased the overall size of each literature by 1.25-fold to 2-fold.
For each set of articles retrieved from each PubMed query (which will be referred to as the query set, the retrieval set, or the topical literature), in the first time slice, we extracted all MeSH terms (ignoring subheadings) and all pairs of MeSH terms co-occurring in the same article. For MeSH terms that occurred in at least 1% of the articles in the query, we computed the co-occurrence of MeSH term pairs that would be expected if each MeSH term is assigned to articles independently, at random. Those MeSH term pairs that had expected co-occurrences of 10 or more [i.e., Frq (query AND MeSH1) × Frq (query AND MeSH2)/number of articles ≥ 10], but an observed co-occurrence of 0, were defined as “gaps.” (Note that although articles published before 1987 were not included in the first time slice, no MeSH term pair was counted as a gap if one or more articles published before 1987 were indexed by both of the MeSH terms. Thus, gaps reflect the entire MEDLINE literature from its beginnings through the end of 2005.)
Counting Citations
To compute citations for articles published in the second time slice, we used the title, publication date, and DOI when available to identify the Google Scholar (GS) record and to extract the listed GS citations as of November 2016. Of 2,418 gap-filling articles, 6 were not found in GS; for those, we used their citations in PubMed Central instead.
Within each query, each gap was characterized according to different features:
1. The expected number of co-occurrences within the query set.
2. The expected number of co-occurrences within MEDLINE as a whole (using the frequencies of each MeSH term within all of MEDLINE in the baseline 2015 release).
3. The observed number of co-occurrences within MEDLINE as a whole (baseline 2015).
4. The article odds ratio (=observed/expected number of co-occurrences within MEDLINE as a whole; Smalheiser and Bonifield, 2016).
5. The author odds ratio. This is computed as the observed/expected number of co-occurrences within the body of articles written by an individual author, summed over all authors publishing in MEDLINE (Smalheiser and Bonifield, 2016).
6. The pR score. This is an innovative measure of semantic similarity between the articles within the query set indexed by the first MeSH term vs. the articles indexed by the second MeSH term (Torvik and Smalheiser, 2007). The Arrowsmith two-node search interface (http://arrowsmith.psych.uic.edu/cgi-bin/arrowsmith_uic/start.cgi; Smalheiser et al., 2009) is utilized to carry out two separate PubMed searches, [query AND MeSH term 1] vs. [query AND MeSH term 2]. In both cases, the MeSH terms are searched without expansion to retrieve related terms. Arrowsmith software computes the words and phrases that are in common to the titles of articles in the two queries (i.e., the B-terms) and uses a quantitative model (Torvik and Smalheiser, 2007) to estimate the predicted relevance of each B-term for linking the two queries in a meaningful way. The percentage of B-terms that are predicted to be relevant is the pR score. We have hypothesized that the pR score may provide a metric to measure the overall implicit information shared by two sets of articles defined by PubMed topical queries (Torvik and Smalheiser, 2007). Because the two-node search only gives meaningful results when the two queries are of sufficient size, we only calculated pR scores when the geometric mean of Frq (query AND MeSH1) and Frq (query AND MeSH2) ≥2% of the total number of articles in the query.
7. The Common Neighbors (CN) score. This is calculated for each query by making a network graph of all MeSH terms that occurred in at least 1% of the articles in the query, where each MeSH term is a node, and nodes are linked if they co-occur in at least one article in the query. For each MeSH term pair that represents a gap, we calculate the number of CN. Python 2.7 and the Networkx-1.10 framework package were used for this and the next feature.
8. The Adamic–Adar (AA) score (Adamic and Adar, 2003). This is a normalized CN score in which the contribution of each CN is divided by the log number of links that it has. The formula is , where T(u) denotes the set of neighbors of u.
9. The MeSHSim score. This is a measure of similarity between the two MeSH terms according to path distance on the MeSH hierarchy. We used the R package by Zhou et al. (2015), using headingSim with parameters headingSim (mesh1, mesh2, method = “SP,” frame = “node”).
The outcomes analyzed for each gap in this study were
1. Number of articles appearing in the second time slice, which were indexed by both MeSH terms comprising the gap. We refer to such articles as those which fill the gap.
2. Presence or absence of articles appearing in the second time slice, which fill the gap, scored 0 or 1, regardless of the exact number of articles.
3. Citations per gap (CPG). For each gap, we computed the square root of the arithmetic mean of the number of citations across all articles filling that gap. (Since citations follow a power law approximately, the square root was taken to make the data distribution more quasi-normal.)
4. Maximum citation per month (MCM). For each gap, we identified the article having the maximum number of citations, and normalized that to citations per month, by dividing by the number of months from its publication date to November 2016.
Note that in a few cases, the same gap appeared in more than one query and was processed separately (since some of the feature scores are query-specific).
For each query, to compare gap-filling vs. non-gap-filling articles, the PMIDs corresponding to each set of articles were entered into our value-added PubMed search interface Anne O’Tate (http://arrowsmith.psych.uic.edu/cgi-bin/arrowsmith_uic/AnneOTate.cgi; Smalheiser et al., 2008). We extracted the top 20 journals, MeSH terms, and author names for the articles that did vs. did not fill at least one gap. We also tabulated the percentage of articles that were published in a multidisciplinary high-impact journal (defined as either Science, Nature, Proc. Natl. Acad. Sci. U.S.A., Cell, New England Journal of Medicine, the Lancet, or JAMA). Finally, to compare citations of gap-filling vs. non-gap-filling articles, for each of the 20 queries, we randomly chose non-gap-filling articles (the number to equal the gap-filling articles for that query). That is, an equal number of gap-filling and non-gap-filling articles were compared for each query.
Results
Prevalence and Fate of Gaps across Queries
Gaps—defined here as pairs of MeSH terms that never co-occurred within any article in the query set despite an expected co-occurrence of 10 or more based on their individual frequencies—were surprisingly prevalent within the PubMed query sets. The number of gaps ranged widely from 10 to 889 across different queries (Table 2). This variability was only partially accounted for by differences in the sizes of the query sets, since queries ranged from 0.13 to 2.78 gaps per 100 articles (Table 2). Nonetheless, the mean number of gaps per query was 261, representing a great number of potential “loose ends” that one might potentially attempt to tie up in the near future!
In fact, across all queries, 30.7% of the gaps present at the end of 2005 were filled by one or more articles published during the subsequent 5-year period of 2006–2010. The high rate at which gaps were filled suggests that they do not generally represent neglected backwaters or research dead-ends. We compared articles that do vs. do not fill gaps in the second time slice, to see which types of journals they were published in. We found that the vast majority of gap-filling articles appeared in journals sharing the topical scope of the top 20 journals where non-gap-filling articles appeared, and only about 10% of the journals were topically divergent. For example, for the acute myocardial infarction query, 27 of the 30 articles that filled gaps were published in cardiology journals; plus one published in a psychiatry journal, one in an oncology journal, and one in a toxicology journal. Similarly, on average, the top 20 MeSH terms indexing the gap-filling articles shared 12 MeSH terms with the top 20 MeSH terms that indexed the non-gap-filling articles. Moreover, there was some overlap of authors between the two sets: across all queries, an average of 1.45 author names (listed on 2 or more gap-filling articles) were also in the top 20 author names in the list of those publishing non-gap-filling articles.
Impact of Gap-Filling Articles
Gap-filling articles were more highly cited, overall, than non-gap-filling articles (65.11 vs. 52.44 citations on average), a difference that was highly significant and consistent across the dataset as a whole (non-parametric two-tailed Mann–Whitney U test, p = 4.11 × 10−14; unpaired two-tailed t-test performed on square roots of citations, p = 7.82 × 10−11). Thus, insofar as citations are indicative of scientific impact, gap-filling articles were more prominent than a random cross section of articles published on the same topics.
Perhaps most tellingly, gap-filling articles were significantly more likely to appear in the most prestigious, multidisciplinary high-impact journals (defined here as including Science, Nature, Proc. Natl. Acad. Sci. U.S.A., Cell, New England Journal of Medicine, the Lancet, and JAMA). Overall, 3.34% of gap-filling articles were published in high-impact journals compared to 2.08% of non-gap-filling articles (3.34 ± 3.19 SD vs. 2.08 ± 1.22, N = 20, p = 0.016 by paired two-tailed t-test; p = 0.0026 by sign test). Stated another way, gap-filling articles are 61% more likely to appear in high-impact journals than non-gap-filling articles (3.334/2.08 = 1. 606). The set of gap-filling articles is enriched in findings deemed particularly novel and significant at the time of publication (i.e., as assessed by reviewers and editors of high-impact journals). Together, these findings suggest that most of the gap-filling articles reside in, and may help redirect, the mainstream of the field.
Features of Gaps in Disease vs. Biological Processes Query Sets
The 10 disease query sets and 10 biological processes query sets were comparable in terms of number of articles per query. The number of gaps and the percentage of gaps filled in the second time slice were not significantly different between the two types of queries. However, the disease-related vs. biological processes article sets differed significantly in their structure. For example, the biological processes queries had almost twice the number of MeSH pairs per article as the disease queries (Table 2), suggesting that they are more topically diverse. The article odds ratios of the gaps found in the disease query sets were less than half the mean value in the biological processes queries (Table 3). The MeSH terms comprising disease gaps had significantly lower similarities as judged by author odds ratios, CN and AA network scores, and MeSHSim as well (Table 3). Interestingly, the distribution of pR scores was nearly the same in the two types of queries (mean = 0.252, SD = 0.082 vs. mean = 0.254, SD = 0.086; Table 3). Yet only 56% of the disease gaps were bridging MeSH terms that were frequent enough to calculate pR scores (see Materials and Methods), whereas 72% of biological processes gaps were scored. We had not initially anticipated that these parameters would differ across the board so strikingly, especially since both article sets are biomedical in nature. This finding presumably reflects the fact that biological processes are studied across multiple model systems and multiple levels of integration (from molecules to organisms), whereas the disease-related studies are more narrowly focused on humans and issues such as diagnosis, pathogenesis, and treatment. In any case, this led us to analyze the features of gaps within the article sets both separately and as a combined dataset.
Table S1 in Supplementary Material shows the non-parametric Spearman rank correlation rho values for the features and outcomes all considered pairwise, for the combined dataset and separately for disease and biological processes queries. (Note that Pearson linear correlation values are not appropriate here since many of the features and outcomes are not normally distributed.) Considering, first, how different gap features are correlated among themselves, it is apparent that the CN and AA scores are almost perfectly correlated (0.994), suggesting that they are redundant, whereas the MeSHSim score shows a very low correlation with any other feature (<0.20), suggesting that it measures a very different and non-redundant type of MeSH term similarity. The article odds and author odds show an intermediate correlation (0.5–0.6) as expected (Smalheiser and Bonifield, 2016), indicating that they are related but that each gives information on its own. The article odds measures how often the two MeSH terms co-occur in MEDLINE as a whole (not just within the given query), relative to what is expected by chance, whereas the author odds measures how often the two MeSH terms co-occur in the body of articles written by the same individual, relative to the level expected by chance.
Gaps are Heterogeneous
We identified several types of gaps that arise from different scientific scenarios, each associated with a different combination of features:
1. Gaps arising as a byproduct of MeSH indexing. A lack of co-occurrence of two MeSH terms could potentially be a trivial consequence of MeSH indexing rules. For example, few articles are indexed with both “Fatal Outcome” and “Mortality,” despite the very similar nature of these two MeSH terms. This is because MEDLINE indexers are instructed to index an article with “Fatal Outcome” if it is concerned with death of an individual, whereas an article is indexed with “Mortality” if it discusses death at a population level. Another example is “Tumor Cells, Cultured” vs. “Cell Line, Tumor.” These are closely related; in fact, they are adjacent on the MeSH hierarchy and have a very high MeSHSim score = 0.92, but the former concept is used to index articles that describe culturing tumor cells acutely whereas the latter term is used if the tumor cells are established as a cell line. Across the combined dataset of 5,221 gaps, only 3 gaps had MeSHSim scores above 0.9, and all were examples of MeSH indexing rules.
2. Gaps that lack biological meaning. Another reason that two MeSH terms might not co-occur within the same article is because there is truly no meaningful relationship between them. For example, separate articles on suicide discuss “Suicide, Assisted” and discuss the enzyme “Thymidine Kinase,” but arguably there is no information gain from combining these apparently unrelated concepts. Note that the article odds is very low for this gap (=0), as is the author odds (=0.063). The pR score for these two MeSH terms is only 0.045, similar to the value observed when pairs of MeSH terms are chosen at random (Torvik and Smalheiser, 2007). Gaps of this nature are much less likely to be filled during the second time slice: across the combined dataset, 862 gaps satisfied the criteria (article odds < 0.2, author odds < 0.5, pR < 0.2), of which only 9.3% were filled during the second time slice, with a mean of 1.27 articles per filled gap (compared to 28.2% across all other gaps with a mean of 1.89 articles per filled gap). Using stricter criteria (article odds < 0.1, author odds < 0.2, pR < 0.2), there were 71 gaps of which only 2.8% were filled (by one article each).
3. Gaps that represent “low hanging fruit.” Conversely, a gap may represent “low hanging fruit”—pairs of MeSH terms that have not previously been studied together yet both lie at the research frontier of the field. For example, “Angina, Unstable” and “Heart Rupture, Post-Infarction” did not co-occur in any article within the acute MI literature by the end of the first time slice, but these two topics did co-occur within Medline as a whole as often as expected by chance (i.e., article odds = 1.06), and they had an extremely high author odds score (=36.42), indicating that the same investigators had a strong tendency to discuss both topics (albeit in different articles). Two articles in the second time slice were indexed by both of these MeSH terms, and the concepts were explicitly related to each other (i.e., heart rupture was a complication of unstable angina-associated infarction). Across the combined dataset, we observed 185 gaps satisfying the criteria (article odds > 1, author odds > 1), of which 47.6% were filled during the second time slice, with a mean of 3.4 articles per filled gap.
4. Gaps in communication. A final (and perhaps the most interesting) reason that gaps may exist in the first time slice is because the two MeSH terms are associated with different disciplines, groups of investigators, geographical regions, or other sub-groups of the field that are either not aware of each other, or do not collaborate together. For example, in the apoptosis query set, the pair of MeSH terms “Liver Neoplasms” and “Neurons” remained unfilled in the second time slice, and has very low article odds (=0.0065) and low author odds (=0.40). Nevertheless, since liver cells and neurons share many biochemical and cellular pathways, it is plausible that they share information that might be useful to bridge. Note that the pR score for this pair is 0.499, similar to the highest values observed for pairs of article sets that are closely topically related (Torvik and Smalheiser, 2007). Such a gap may be intrinsically promising, yet remain unexplored during the second time slice, due to reasons that may be cultural or pragmatic rather than scientific. Across the combined dataset, we observed 45 gaps satisfying the criteria (article odds < 0.2, author odds < 0.5, pR > 0.35), of which 22.2% were filled during the second time slice, and a mean of 1.2 articles published per filled gap (compared to 25.1% filled across all other gaps, and 1.85 articles published per filled gap). Thus, this type of gap is not entirely neglected, but the number of articles published per gap is relatively low, especially compared to the “low hanging fruit.” As indicated above, the influence of pR was restricted to the diseases queries: 36.4% of gaps fulfilling the criteria for this type were filled during the second time slice, and a mean of 1.2 articles published per filled gap, in contrast to the biological processes queries where only 8.7% of these gaps were filled and 1.0 articles per filled gap.
Table 4 shows the gaps of this type within the biological processes queries. Most of these gaps are bridging very different disciplines (e.g., infertility, Male::Saccharomyces cerevisiae) and—at first glance, at least—might be thought to share no interesting information, except that their high pR score points to interesting information that might possibly have been overlooked.
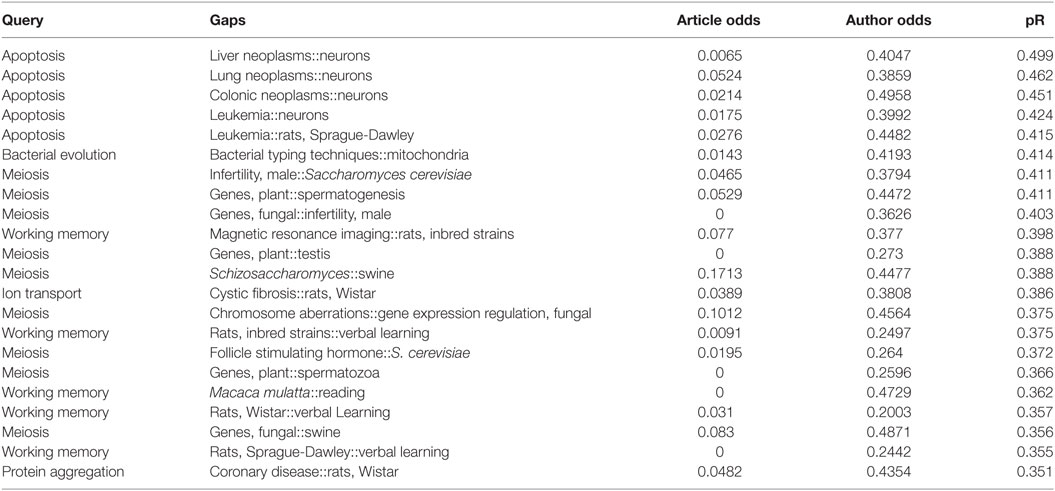
Table 4. Gaps across disciplines in biological processes article sets showing some of their relevant features.
Features That Correlate with the Likelihood That a Gap Will Be Filled in the Near Future
Putting aside the heterogeneity of gaps, we next analyzed how individual gap features were predictive of gap-filling across the combined queries and in disease-related vs. biological processes queries. We were able to identify certain gap features that correlated with the likelihood that a given gap will be filled in the next 5 years and with the number of articles filling the gap during that period. The best single predictor overall was the article odds, rho = 0.35 (0.38 in disease queries, 0.32 in biological queries). Stated another way, MeSH pairs that have co-occurred frequently elsewhere in MEDLINE are also the most likely to appear in the given query literature in the near future. This is likely to reflect gaps that are “low hanging fruit.” Other individual features that correlated with the subsequent number of gap-filling articles in the disease queries were pR (=0.34); CN/AA (=0.34); observed co-occurrence in Medline (=0.32); and author odds (= 0.23). The rho correlations were generally lower in the biological processes queries [observed co-occurrence in Medline (=0.27); CN/AA (=0.24); author odds (=0.10); and pR (=0.02)].
In order to see which features were most important after holding all other factors constant and to see which combinations of features were most predictive across all gaps, we used the Weka 3.8.0 software environment (Frank et al., 2016) to explore multiple linear regression models. Features that had skewed distributions were converted to square root values to improve quasi-normality, and the software was set to adjust for feature scaling and collinearity. Cross-validation (10-fold) was applied to avoid overfitting. The goal was not to optimize model predictive performance, but to understand the relative weights and independent influences of each feature. As shown in Table 5, over the entire dataset of 20 queries, the pR score was the most important predictor of the number of gap-filling articles that will appear in the second time slice, followed by the article odds. The relative importance of features was the same in both groups for classifying gaps simply as filled or not filled in the second time slice (0 vs. 1), regardless of the number of articles (data not shown). Relative importance of features was also the same when gaps whose article odds = 0 were removed from the set (i.e., by definition, none of those can have publications in the second time slice; not shown). A random forest model with the same features produced about the same performance as the multiple linear regression model (not shown).
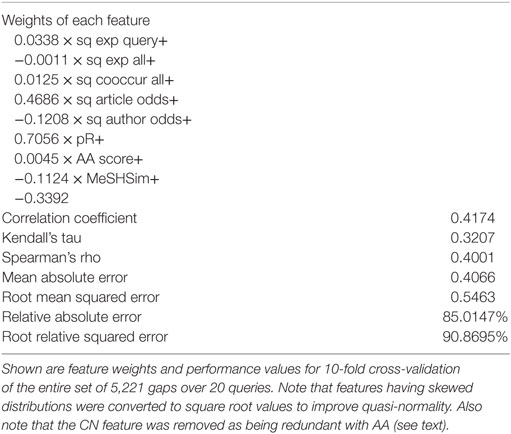
Table 5. Multiple linear regression to predict the number of gap-filling articles appearing in the second time slice in the combined dataset.
As suspected, the fitted regression models were quite different for the disease queries vs. the biological processes queries (Table 6 vs. Table 7). Interestingly, both article odds and pR scores were the top two predictors in both groups, although pR was far more important in the disease queries whereas article odds dominated in the biological processes queries. The MeSHSim score had an appreciable negative weight in the disease queries only, whereas the author odds ratio had an appreciable negative weight only in the biological processes queries. The network similarity scores (CN and AA) contributed significantly when examined as single features in both sets of articles (Table 5; cf. Kastrin et al., 2016) but had little effect in the fitted models; that is, their influence could be “explained away” by the influence of other correlated features.
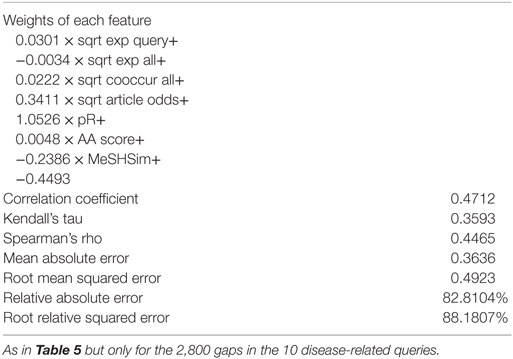
Table 6. Multiple linear regression to predict the number of gap-filling articles appearing in the second time slice in the 10 disease queries only.
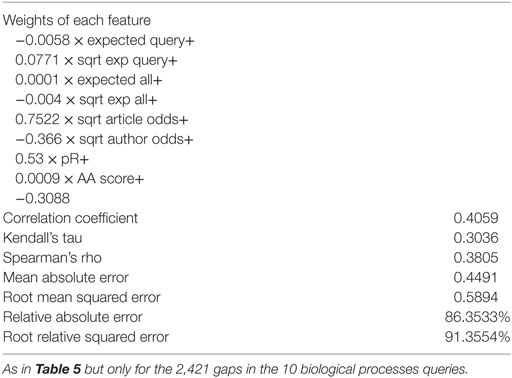
Table 7. Multiple linear regression to predict the number of gap-filling articles appearing in the second time slice in the 10 biological processes queries only.
Relation of Gap Features to Subsequent Citations
Surprisingly, none of the features that were examined showed any large rank correlations with the number of citations garnered by gap-filling articles, either measured as an average of the articles filling a particular gap or the paper having the maximum number of citations (Table S1 in Supplementary Material). This lack of correlation was observed for both disease-related queries and biological processes queries (Table S1 in Supplementary Material) and for the subset of “low hanging fruit” (not shown). Normalizing the number of citations by topic (i.e., dividing the citations for each gap by the mean number of citations observed for gaps in that query) did not affect these negative results (Radicchi and Castellano, 2012). Thus, although gap-filling articles as a group tended to garner more citations than non-gap-filling articles, we were not able to identify features that predicted which gaps, once filled, were more likely to be highly cited. These findings are possibly limited by the fact that only one time slice and duration were examined, and some transformational research may only garner citations after substantial time has passed (e.g., van Raan, 2004).
Discussion
The present paper identified and characterized “gaps,” i.e., pairs of MeSH terms that never co-occurred within any article in a given topical biomedical literature, despite an expected co-occurrence of 10 or more based on their individual frequencies. Ten disease queries and 10 biological processes queries were conducted in PubMed to generate a test bed in the range of 7,000–50,000 articles each for study. We found that each query set (containing articles through 2005) is associated with multiple gaps. As a whole, these are relatively dynamic, since almost a third of them were filled within the next 5 years by the publication of one or more articles that were dual indexed with both MeSH terms. The gap-filling articles are published in journals that are topically similar to non-gap-filling articles, are more highly cited than non-gap-filling articles on the same topic, and are 61% more likely to be published in multidisciplinary high-impact journals.
Gaps fell into several categories that have very different origins, characteristic features, and implications for scientists. A total of nine gap features were employed to characterize gaps, which were used to divide them into several categories:
(a) Gaps arising as a byproduct of MeSH indexing rules that constrain dual indexing of very similar MeSH terms. The signature of these infrequently observed gaps was a very high MeSHSim score, which measures path closeness on the MeSH hierarchy.
(b) Gaps that lack any obvious biological meaning. Such gaps had very low scores on multiple features.
(c) Gaps that represent “low hanging fruit.” Such gaps had relatively high article odds scores, meaning that the pair of MeSH terms has previously been well studied in other fields and is now poised to contribute to the given query literature. This gap category appears to be most likely to generate gap-filling articles in the next 5 years.
(d) Gaps in communication. These have not been brought together in any article because the two MeSH terms are associated with different disciplines, groups of investigators, geographical regions, or other subgroups of the field that are either not aware of each other, or do not collaborate together. Such gaps had low article odds (that is, they had not been well studied together anywhere in MEDLINE) and low author odds scores (that is, the same investigators had rarely written on both topics, even in separate articles), yet such gaps showed evidence that the MeSH terms generate some useful information when brought together, as reflected by high implicit similarity pR scores (Torvik and Smalheiser, 2007). We have hypothesized that the pR score measures the amount of implicit information shared between two MeSH terms (Torvik and Smalheiser, 2007), and a high pR score ought to imply that significant new knowledge can be gained from bridging the two MeSH terms (Swanson and Smalheiser, 1997). Thus, in contrast to “low hanging fruit,” the “gaps in communication” may point to discoveries that involve more unexpected or surprising new connections.
Although this initial categorization of gap types appears to hold generally, the relative prevalence of different types of gaps varied extensively across biomedical article sets on different topics which are structured in different ways (e.g., some are topically narrow and focused, others are a mix of separate communities). Multiple linear regression modeling was carried out to learn which gap features, if any, correlated with the number of gap-filling articles appearing in the next 5 years. However, we learned that fitting a single quantitative model was of limited value, because of the heterogeneity in types of gaps and types of queries. For example, the pR score was the strongest predictor of gap-filling articles when tested in disease-related query sets, yet the article odds ratio was a much stronger predictor in biological processes query sets. When considering all gaps as a single collection, the features studied here explained only about 16% of the variability in the number of articles published in the second time slice (Table 5).
The initial study reported here provides the starting point for much further research. For example: what is the function and relative importance of articles that fill gaps—that is, articles newly combining MeSH terms that are both already well represented in a field—relative to articles that introduce MeSH terms appearing in the disciplinary field for the first time, and relative to articles that are indexed by MeSH terms which are entirely novel and newly added to the MeSH hierarchy?
• How important are different types of gaps, particularly “low hanging fruit” vs. “gaps in communication,” for driving the mainstream of a field in new directions? Which types of gaps, if any, are most likely to lead to findings that transform a field radically?
• Which types of gaps, once filled, will be associated with articles that are cited highly? None of the gap features that we examined were useful in predicting the number of citations that a gap-filling article will garner, but we only examined the set of gaps as a whole. This analysis needs to be repeated using a larger dataset of queries that examines different types of gaps separately.
• As well, when comparing gap-filling vs. non-gap-filling articles on the same topic, it will be interesting to see how they compare on other features that reflect research strategies and approaches, such as journal, country of origin, size of collaborative author team, extent of interdisciplinarity (Larivière et al., 2015), and so on.
• The present study only examined one 5-year follow-up time slice (2005–2010). However, that time frame was chosen rather pragmatically. It will be worth examining gaps filled during the next 5 years (2010–2015) to see if the results are similar. Also, the time of delay in filling a gap is itself a feature that may have significance.
• What is the significance of gaps that have never co-occurred in any articles in MEDLINE to date, i.e., those whose article odds = 0? Filling such gaps requires truly novel combinations of MeSH terms. Although most of these gaps may not be biologically meaningful, those that do bridge useful information may have the potential to be especially surprising and innovative.
• Finally, there are inherent limitations to the use of MeSH terms for identifying gaps, due to their manual assignment to articles, partial redundancy in some cases, granularity, incomplete coverage, relatively slow updating of new terms into the hierarchy, restriction to articles indexed in MEDLINE, and so on. The Arrowsmith project (Torvik and Smalheiser, 2007; Smalheiser et al., 2009) employed shared title words and phrases, rather than shared MeSH terms, for bridging pairs of articles, for these and other reasons. It will be worth exploring how gaps identified using pairs of title terms (or pairs of terms appearing in abstract or full text) will compare to those identified using pairs of MeSH terms.
Implementation
To provide a public test bed for studying gaps, we have implemented a tool called “Mine the Gap!” as part of the Anne O’Tate suite of value-added PubMed search tools (accessible with no login or passwords at http://arrowsmith.psych.uic.edu/cgi-bin/arrowsmith_uic/AnneOTate.cgi). The user carries out any topical PubMed query, after which a panel of options are displayed on the left hand of the page. One of these, the “Mine the Gap!” tool, identifies and characterizes the gaps with regard to a number of features (especially, article odds and author odds scores) and displays them in ranked form on the website. Optionally, the user can then click a button to automatically carry out Arrowsmith two-node searches on each gap, calculate the pR scores, and view the resulting output from each two-node search if desired. The dataset can be re-ranked on the website or exported as a comma-delimited text file for further study by informaticians, domain scientists, or policy researchers.
The master sheet of gaps, features, and outcomes is attached to this article as Table S2 in Supplementary Material, and the list of gap-filling and non-gap-filling articles with their citations is attached as Table S3 in Supplementary Material.
Author Contributions
Conceived and designed the study; performed regression analyses: NS. Provided data on PMIDs and feature scores; programmed the website: GB. Calculated network features and citations: YP. Carried out statistical analyses: NS and GB. Wrote and approved the manuscript: NS, YP, and GB.
Conflict of Interest Statement
The authors declare that the research was conducted in the absence of any commercial or financial relationships that could be construed as a potential conflict of interest.
Funding
This study was supported by NIH P01AG039347. YP received a short-term fellowship from Nanjing University.
Supplementary Material
The Supplementary Material for this article can be found online at http://journal.frontiersin.org/article/10.3389/frma.2017.00003/full#supplementary-material.
References
Adamic, L. A., and Adar, E. (2003). Friends and neighbors on the web. Soc. Networks 25, 211–230. doi: 10.1016/S0378-8733(03)00009-1
Chen, C. (2014). The Fitness of Information: Quantitative Assessments of Critical Evidence. New York, NY: John Wiley & Sons.
Chen, C., Chen, Y., Horowitz, M., Hou, H., Liu, Z., and Pellegrino, D. (2009). Towards an explanatory and computational theory of scientific discovery. J. Informetr. 3, 191–209. doi:10.1016/j.joi.2009.03.004
Frank, E., Hall, M. A., and Witten, I. H. (2016). “The WEKA workbench,” in Online Appendix for Data Mining: Practical Machine Learning Tools and Techniques, 4th Edn (Burlington, MA: Morgan Kaufmann).
Kastrin, A., Rindflesch, T. C., and Hristovski, D. (2016). Link prediction on a network of co-occurring MeSH terms: towards literature-based discovery. Methods Inf. Med. 55, 340–346. doi:10.3414/ME15-01-0108
Larivière, V., Haustein, S., and Börner, K. (2015). Long-distance interdisciplinarity leads to higher scientific impact. PLoS ONE 10:e0122565. doi:10.1371/journal.pone.0122565
Mishra, S., and Torvik, V. I. (2016). Quantifying conceptual novelty in the biomedical literature. Dlib Mag. 22, 9–10. doi:10.1045/september2016-mishra
Packalen, M., and Bhattacharya, J. (2015). Neophilia ranking of scientific journals. NBER Working Papers No. 21579. doi:10.3386/w21579
Radicchi, F., and Castellano, C. (2012). Testing the fairness of citation indicators for comparison across scientific domains: the case of fractional citation counts. J. Informetr. 6, 121–130. doi:10.1016/j.joi.2011.09.002
Smalheiser, N. R., and Bonifield, G. (2016). Two similarity metrics for medical subject headings (MeSH): an aid to biomedical text mining and author name disambiguation. J. Biomed. Discov. Collab. 7, e1. doi:10.5210/disco.v7i0.6654
Smalheiser, N. R., Torvik, V. I., and Zhou, W. (2009). Arrowsmith two-node search interface: a tutorial on finding meaningful links between two disparate sets of articles in MEDLINE. Comput. Methods Programs Biomed. 94, 190–197. doi:10.1016/j.cmpb.2008.12.006
Smalheiser, N. R., Zhou, W., and Torvik, V. I. (2008). Anne O’Tate: a tool to support user-driven summarization, drill-down and browsing of PubMed search results. J. Biomed. Discov. Collab. 3, 2. doi:10.1186/1747-5333-3-2
Swanson, D. R., and Smalheiser, N. R. (1997). An interactive system for finding complementary literatures: a stimulus to scientific discovery. Artif. Intell. 91, 183–203. doi:10.1016/S0004-3702(97)00008-8
Theodosiou, T., Vizirianakis, I. S., Angelis, L., Tsaftaris, A., and Darzentas, N. (2011). MeSHy: mining unanticipated PubMed information using frequencies of occurrences and concurrences of MeSH terms. J. Biomed. Inform. 44, 919–926. doi:10.1016/j.jbi.2011.05.009
Torvik, V. I., and Smalheiser, N. R. (2007). A quantitative model for linking two disparate sets of articles in MEDLINE. Bioinformatics 23, 1658–1665. doi:10.1093/bioinformatics/btm161
Uzzi, B., Mukherjee, S., Stringer, M., and Jones, B. (2013). Atypical combinations and scientific impact. Science 342, 468–472. doi:10.1126/science.1240474
van Raan, A. F. J. (2004). Sleeping beauties in science. Scientometrics 59, 461–466. doi:10.1023/B:SCIE.0000018543.82441.f1
Yetisgen-Yildiz, M., and Pratt, W. (2009). A new evaluation methodology for literature-based discovery systems. J. Biomed. Inform. 42, 633–643. doi:10.1016/j.jbi.2008.12.001
Keywords: scientific discovery, link prediction, Medical Subject Headings, text mining, literature-based discovery
Citation: Peng Y, Bonifield G and Smalheiser NR (2017) Gaps within the Biomedical Literature: Initial Characterization and Assessment of Strategies for Discovery. Front. Res. Metr. Anal. 2:3. doi: 10.3389/frma.2017.00003
Received: 23 January 2017; Accepted: 23 March 2017;
Published: 22 May 2017
Edited by:
Min Song, Yonsei University, South KoreaReviewed by:
Karin Verspoor, University of Melbourne, AustraliaRaf Guns, University of Antwerp, Belgium
Copyright: © 2017 Peng, Bonifield and Smalheiser. This is an open-access article distributed under the terms of the Creative Commons Attribution License (CC BY). The use, distribution or reproduction in other forums is permitted, provided the original author(s) or licensor are credited and that the original publication in this journal is cited, in accordance with accepted academic practice. No use, distribution or reproduction is permitted which does not comply with these terms.
*Correspondence: Neil R. Smalheiser, neils@uic.edu