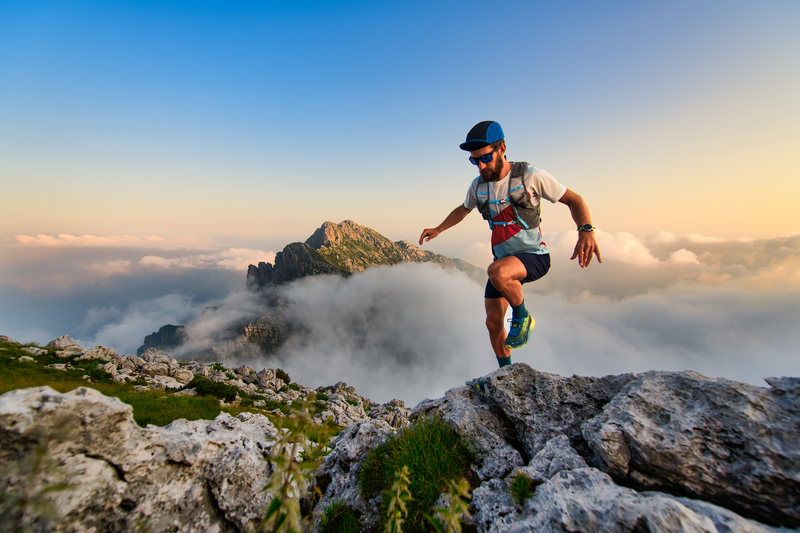
95% of researchers rate our articles as excellent or good
Learn more about the work of our research integrity team to safeguard the quality of each article we publish.
Find out more
ORIGINAL RESEARCH article
Front. Remote Sens. , 27 March 2025
Sec. Multi- and Hyper-Spectral Imaging
Volume 6 - 2025 | https://doi.org/10.3389/frsen.2025.1549119
This article is part of the Research Topic Achieving SDG 6: Remote Sensing Applications in Sustainable Water Management View all 4 articles
Satellite imagery has enabled widespread monitoring of algae in larger water bodies, however until recently, the spatial resolution of available sensors has not been sufficient to apply this to smaller lakes. Therefore, this study investigated a new dataset of high-resolution metre-scale imagery for monitoring phytoplankton at spatial and temporal scales previously impossible with satellite data. Specifically, the Planet SuperDoves constellation was used to monitor a small (0.069 km2), eutrophic lake from 2021 to 2024. Several chlorophyll-a (Chl-a) algorithms were tested on both SuperDoves and Sentinel-2 data against in situ measurements. Additionally, the suitability of citizen science data as a validation tool for widespread algal bloom monitoring was investigated by comparing reports of algal blooms in five small water bodies in central Scotland with corresponding SuperDoves Chl-a images. Chl-a was successfully retrieved using the Ocean Colour 3 algorithm (R2 = 0.64, root mean squared error (RMSE) = 0.93 g L−1), which outperformed the best performing Sentinel-2 Chl-a algorithm (R2 = 0.61, RMSE = 1.01 g L−1). Furthermore, both Sentinel-2 and SuperDoves data were equally effective for algal bloom detection, each having F1-scores of 0.89 at a Chl-a bloom threshold of 40 g L−1. This demonstrates that metre-scale satellite monitoring of algae is possible even in challenging and optically complex environments such as small, shallow water bodies. This leads towards a potential step-change in the number of remotely monitorable inland water bodies, which would be a significant advancement for global lake science, environmental management and public health protection efforts.
Water quality in lakes is a critical issue. The ecosystem services provided by lakes are innumerable, ranging from provision of drinking water, food supply, recreation, preservation of cultural heritage, and even healthcare (Alejandre et al., 2024; Heino et al., 2021; Inácio et al., 2022). However, water quality in many lakes across the globe is poor, and this is exacerbated by climate change and unsustainable industrialisation (Naderian et al., 2024). In this context, the United Nations Sustainable Development Goal 6 is focused on ensuring availability and sustainable management of water and sanitation across the globe by 2030, but unfortunately this is still out of reach for billions of people (Evaristo et al., 2023). To meet this ambitious and incredibly important goal, a massive acceleration of progress in effective and sustainable water resource management is needed, and there is therefore a need to improve the way in which we manage, monitor and understand lake ecosystems across the world (Rajapakse et al., 2023). Globally, the vast majority of lakes are very small in size (∼99% < 1 km2) (Cael and Seekell, 2016). Despite this, until recently, smaller water bodies have generally been considered of lower scientific importance than their larger counterparts, and so are somewhat less-well understood (Downing, 2010; Shen et al., 2025). Now, there is a growing recognition that smaller water bodies are critically important for biodiversity, water quality, human use, and as early indicators of environmental changes (Biggs et al., 2017). In these water bodies the aquatic primary producers, the phytoplankton, provision a large number of ecosystem services including oxygen production, nutrient cycling, climate regulation, and food supply (Naselli-Flores and Padisák, 2023). However, large accumulations of phytoplankton known as algal blooms can present severe ecological and public health risks and are thought to be increasing globally, likely due to eutrophication and climate change (Huisman et al., 2018). Therefore, there is a clear need to further our understanding of, and ability to monitor, phytoplankton dynamics, particularly in smaller lakes which have been historically less well-studied.
In recent years, satellite remote sensing has emerged as a powerful method for monitoring water quality indicators such as chlorophyll-a (chl-a), a well-established proxy for algal biomass (Zahir et al., 2024). Typically, algae monitoring with remote sensing is carried out on relatively large bodies of water such as the Great Lakes in North America (Beaulne and Fotopoulos, 2024). Historically this has been due to both the spatial limitations of satellite sensors, and the significant impact these water bodies have on regional ecosystems and economies (Sterner et al., 2020). However, the ability to monitor smaller water bodies with satellite remote sensing would provide many benefits. Firstly, this would provide valuable data which could enable widescale monitoring of algal blooms and therefore enable better management and provision of the critical services which are threatened by harmful algae (Liu et al., 2022). Additionally, there is a potentially huge benefit to the scientific community, as such data could enable global lake water quality monitoring studies of an unprecedented scale (Kutser and Soomets, 2024).
Various satellite sensors, such as Sentinel-2 Multispectral imager (MSI) (10–60 m spatial resolution, 5-day repeat cycle) and the Landsat 8/9 constellation (30 m spatial resolution, 8-day repeat cycle) have already started to further our understanding of phytoplankton dynamics and algal blooms in smaller water bodies (Zabaleta et al., 2023). SuperDoves satellite constellation, which were launched sequentially between 2019 and 2023 b y Planet Labs Public Benefit Company (PBC) offer daily 3 m imagery with eight spectral bands in the visible and near infra-red (NIR) portion of the light spectrum (Planet Labs PBC, 2018). This presents a considerable spatio-temporal advantage over the existing satellite sensors which have been used for studying phytoplankton. Previously, four-band Planet imagery has been used for chl-a estimation in a large (128 km2) lake (Niroumand-Jadidi and Bovolo, 2021), and to monitor chl-a in a number of medium to large sized (approx. 5 km2–200 km2) reservoirs (Mansaray et al., 2021). More recently, Vanhellemont (2023) evaluated the capabilities of eight-band SuperDove imagery for aquatic applications, demonstrating its potential for monitoring chl-a concentrations in a coastal environment. At present, there is one other study that has compared SuperDoves with imagery from another satellite sensor for monitoring chl-a in an inland water body. Wasehun et al. (2025) compared Sentinel-2 and SuperDoves for monitoring chl-a in a large (∼60 km2) reservoir, and found that SuperDoves generally outperformed Sentinel-2. Specifically, it was found that the higher spatial resolution of SuperDoves was beneficial for resolving narrow geometries where larger areas of the Sentinel-2 imagery displayed errors due to edge-effects. Evidently, the SuperDoves show promise for monitoring algae in inland waters, but at present there are no studies which have specifically evaluated eight-band SuperDove imagery for monitoring algae in smaller water bodies (<1 km2). Additionally, whilst the higher spatial resolution of SuperDoves may be advantageous for phytoplankton monitoring, Niroumand-Jadidi et al. (2022) found that down-sampling SuperDoves data to 15 m resolution improved the performance of river bathymetry retrieval by increasing the signal to noise ratio. This has not been investigated for chl-a monitoring, and so it is uncertain whether the higher spatial resolution of the SuperDoves is truly beneficial. Furthermore, as the SuperDoves generally cover a narrower spectral region than Landsat or Sentinel-2, they potentially lack bands which are useful for atmospheric correction (AC), such as those in the short wavelength infrared region (SWIR), which can be used for glint correction over water (Steinmetz and Ramon, 2018). Dogliotti et al. (2024) found that SuperDoves tended to have higher AC errors than Landsat and Sentinel-2 when using default “land-based” AC approaches for water reflectance retrieval. However, as there are several AC algorithms that have been developed for inland waters (e.g., Soriano-González et al., 2022; Steinmetz and Ramon, 2018; Vanhellemont and Ruddick, 2018), it would be beneficial to understand to what extent the wider range of AC algorithms available for a more well-established sensor such as Sentinel-2 MSI provide an advantage over those presently available for the SuperDoves.
Generally, very few small water bodies are routinely monitored, highlighting the need for remote sensing approaches. However, this presents a challenge, as limited ground truth data are available for validation. A potential solution is to use data collected in a regulatory monitoring context alongside citizen science data. Therefore, this study focuses on two external sources of validation data: data collected by the Scottish Environment Protection Agency (SEPA), and citizen science data recorded through the mobile phone application Bloomin’ Algae. The use of citizen science data can foster environmental stewardship and can increase public understanding of environmental issues (Pocock et al., 2023). Alongside this, it has potential to provide wide coverage, and so can be used both to validate and complement satellite data for water quality monitoring applications (Putman et al., 2023).
Therefore, the focus of this study is to understand if SuperDoves imagery and the currently available associated algorithms are suitable for monitoring algae and algal blooms in small inland water bodies. Specifically, the key hypothesis being tested is: given that SuperDoves have a similar band configuration to Sentinel-2, it is expected that with appropriate algorithm selection they may have similar performance for algae monitoring in small water bodies. This is significant given that the spatial resolution of SuperDoves data potentially enables water quality monitoring in a vast number of lakes which previously would have been considered too small for satellite monitoring. To ascertain if this is possible, we first make a comparison of SuperDoves with Sentinel-2 and associated algorithms. This comparison enables answering the following questions for a small inland water body: (1) do the presently available atmospheric correction algorithms for SuperDoves perform similarly to the best performing algorithms for Sentinel-2? (2) using the best working algorithms, can chl-a be retrieved from SuperDoves imagery with similar or better performance to Sentinel-2? (3) using citizen science and environment agency data for validation, do Sentinel-2 and SuperDoves perform similarly for algal bloom detection? (4) Does the higher spatial resolution offered by SuperDoves resolve features in the chl-a distribution more clearly than it would at lower resolution? Finally, to test the wider applicability of the SuperDoves, we investigate whether citizen science algal bloom records in five other small water bodies in central Scotland match the corresponding SuperDoves imagery.
With an area of 0.069 km2, Airthrey Loch is a small, eutrophic lake in central Scotland (United Kingdom) with a maximum depth of less than 4.5 m. The water body is artificial, was constructed in 1788, and is now part of the University of Stirling campus (Kelly and Smith, 1996). During the spring and summer cyanobacterial blooms regularly occur which frequently result in closures to the recreational activities which the lake supports such as fishing and water sports. Whilst there is no routine water quality monitoring of Airthrey Loch, SEPA carry out phytoplankton counts a handful of times each year to assess the safety of the water for recreation upon request of the University. Airthrey Loch is large enough that most of its area is resolvable with Sentinel-2 imagery, however, it also features narrow sections which may require the higher resolution of SuperDoves to be properly resolved (Figure 1).
Figure 1. Aerial photograph of Airthrey Loch provided by Digimap®, © Getmapping Ltd. The orange triangle indicates the approximate location of the DALEC hyperspectral sensor, the orange circle the water sampling location, and the red square the 30 × 30 m area of interest (AOI) used for analysis.
An automated hyperspectral above water reflectance measurement platform (In situ Marine Optics Dynamic Above-water Radiance and Irradiance Collector (DALEC-SN0005)) was deployed for 19 days in 2022 from 28th July – 15th August, and 85 days in 2023 from 8th July – 30th September. Data were only collected over 96 days due to instrument maintenance and power supply outages. The DALEC was configured to continuously measure for 4 h each day, from 10:00–14:00 UTC to include both the SuperDoves overpass between 10:30–11:30 UTC, and Sentinel-2A/B overpass between 11:30–11:50 UTC. The DALEC automatically tracks the angle of the sun with a relative azimuth angle of 135°, and was mounted on a steel pole 1 m above the water surface at a location approximately 25 m from the shore on the western side of the lake (latitude: 56.14701°, longitude: −3.92347° - see Figure 1). It has three sensors (Figure 2) which independently measure Lu, the upwelling radiance, Lsky, the sky radiance, and Ed, the downwelling irradiance at approximately 3 nm intervals in the spectral range 380–1,020 nm. Using these measurements, remote sensing reflectances (Rrs) values from the DALEC data were calculated using Equation 1 (Mobley, 1999):
where λ is the wavelength and ρ the skylight reflectance, which for all measurements, was assumed to be the standard value for low wind speeds (<5 m s−1) of 0.028. Following the initial calculation of Rrs, the procedure established by Jiang et al. (2020) was used to remove residual skylight reflectance. Thirteen spectra with negative values, or extremely large reflectance values (Rrs (580) > 0.04 sr−1) were excluded from the data as they were assumed to be spurious measurements. The Rrs data from the DALEC sensor were then convolved using the spectral response functions of Planet SuperDoves and Sentinel-2A/B to allow for validation of the AC procedure.
Figure 2. Photograph showing the DALEC sensor deployed on Airthrey Loch. The DALEC captures downwelling irradiance (Ed), indicated by multiple arrows pointing downwards; upwelling radiance (Lu), indicated by a single angled arrow (approximately 40° off nadir) pointing up from the water to the sensor; and sky radiance (Lsky), indicated by a single angled arrow (approximately 40° off zenith) pointing down at the sensor.
In total, 25 water samples were taken from the study site over two periods: July–October 2022, and April–October 2023. Samples were taken at approximately fortnightly intervals to capture different stages of bloom development throughout the phytoplankton growing season. Where possible, clear, cloud free days were chosen for sampling to maximise the chances of suitable satellite match-up images. Samples were always collected between 10:00 and 13:00 UTC to match satellite overpass times. Sampling was done from the Southern edge of a jetty on the West bank of the loch which extends approximately 20 m away from the shore and is located close (∼30 m) to the DALEC sensor (see Figure 1). Whilst the sampling location was approximately 30 m away from the AOI, any errors arising from this would affect both SuperDoves and Sentinel-2 match-ups, meaning that the resulting performance comparison is still meaningful. On the day of sampling, samples were filtered onto 25 mm GF/F glass microfiber filters with 0.7 μm pore size and stored at −80°C for a maximum of 4 months. Subsequently, analysis for chl-a was carried out using a High-Performance Liquid Chromatography (HPLC) method based on the approach described by Hooker et al. (2009). The generated chl-a data were checked for consistency and spurious values by examining the time series to ensure that the seasonal pattern expected for eutrophic water bodies in Scotland was present. Table 1 gives details on the number of chl-a match-ups obtained for each sensor.
Table 1. Number of match-ups obtained for reflectance measurements (DALEC), and chl-a samples for both sensors.
European Space Agency (ESA) Sentinel-2 (Level 1C) and Planet SuperDoves (8-band top of atmosphere reflectance) imagery covering the full spatial extent of Airthrey Loch from January 2021 through to April 2024 was downloaded from the Planet and Copernicus Dataspace repositories. A 30 × 30 m area of interest (AOI) centred 10 m east of the DALEC sensor location was selected for comparison with the DALEC measurements (Figure 1). This corresponds to 10 × 10 pixels for the 3 m SuperDoves data, and 3 × 3 pixels for the 10 m Sentinel-2 data. The choice to offset the centre of the AOI 10 m from the location of the DALEC was done to minimise adjacency effects, whilst still including several pixels in the direct vicinity of the DALEC.
For the SuperDoves data, the Planet useable data mask (UDM) was used to identify images with clouds over the AOI. Such images were not used for further analysis. For the Sentinel-2 data, the IdePix (Identification of pixel properties) masking tool was used (Wevers et al., 2021). Sentinel-2 images with pixels in the AOI which were flagged as invalid, cloud, cloud shadow, snow/ice, bright, white, cirrus or bright white were not used for analysis.
Several AC procedures for the generation of Level 2 surface reflectance products were tested. For SuperDoves data there are presently only two available AC procedures, and both were used for testing: the default Planet surface reflectance (SR) product, and Atmospheric Correction for Operational Land Imager (OLI) “lite” (ACOLITE), which is designed specifically for aquatic applications (Vanhellemont, 2023). ACOLITE works by automatically selecting bands that are minimally influenced by surface reflectance and uses these for “dark spectrum fitting” to correct for atmospheric scattering and absorption effects (Vanhellemont and Ruddick, 2018). For Sentinel-2, three AC approaches were tested: ACOLITE; Polymer, which uses a spectral matching approach to separate and correct atmospheric and sun glint signals from water leaving reflectance using polynomial and bio-optical modelling (Steinmetz and Ramon, 2018); and Case 2 Regional Coast Colour (C2RCC) which is a deep learning approach trained on a large database of simulated water leaving reflectances and corresponding top of atmosphere radiances (Soriano-González et al., 2022). C2RCC and Polymer were selected as these have been shown to consistently outperform other Sentinel-2 atmospheric methods over inland waters (Warren et al., 2019). ACOLITE was selected for comparison as it is the only procedure available for both SuperDoves and Sentinel-2. For C2RCC, three different neural network configurations were tested: case-2 waters in general (C2RCC_nets), extreme case-2 waters (C2X_nets), and complex extreme case-2 waters (C2X_COMPLEX_nets). All AC models were tested using the default settings, asides from the salinity parameters which were set to 0.3 ppt, a typical value for freshwaters in proximity to urban developments (Dugan et al., 2017).
For both satellite imagery datasets, the median Rrs value over the AOI was calculated for each image and matched with the corresponding DALEC data. A matching window of ±1 day was used to accommodate days with DALEC outages or those immediately before or after DALEC recording started. Matching windows of this size have been previously shown to be effective in obtaining a larger number of inland water match-ups without introducing significant error (Schröder et al., 2024). Table 1 gives details on the number of match-ups obtained for each sensor. To evaluate the performance of the AC, the corrected satellite data were linearly regressed against the convolved DALEC reflectances. Slope, coefficient of determination (R2), root mean squared error (RMSE), median absolute percentage difference (MAPD), and mean average difference (MAD), were used as performance indicators. All algorithms were ranked according to each of these metrics and a combined total rank computed for each algorithm. The highest-ranking AC algorithms for both SuperDoves and Sentinel-2 data were identified and used for subsequent analyses.
All of the algorithms in Neil et al. (2019) and Vanhellemont (2023) which use bands available in both the Planet SuperDoves and Sentinel-2 data were chosen for testing. In Neil et al. (2019), several algorithms make use of the near infrared ratio in linear, quadratic and power functions. Preliminary tests found that the linear versions of these algorithms performed best and so only these were considered for the formal analysis. This resulted in six separate algorithms (Equations 2-9) which were used for formal testing:
1. Near infrared ratio (Gitelson, 1992):
2. Normalised difference chlorophyll index (NDCI) (Mishra and Mishra, 2012):
3. NASA Ocean Colour 3 band algorithm (OC3) (O’Reilly et al., 1998):
where
4. NASA Ocean Colour 2 band algorithm (OC2) (O’Reilly et al., 1998):
where
5. Red band difference (RBD) (Freitas and Dierssen, 2019):
6. Adapted version of the red band ratio (RBR3) (Vanhellemont, 2023):
where a, b, c, d, and e are the tuneable coefficients associated with each algorithm, and Rrs(λ) is the remote sensing reflectance at the wavelength λ.
All in situ chl-a match-ups were used for tuning the coefficients. Tuning was done using least squares regression in log-space as log-transformed chl-a is generally normally distributed, and therefore more suitable for regression (Seegers et al., 2018). Preliminary investigation found that after fitting, the two-fourth order polynomial ocean colour algorithms often produced extremely large or negative chl-a values when applied to new imagery, suggesting overfitting (Lever et al., 2016). Therefore, simplified linear versions (c, d, e = 0) of OC2 and OC3 were used to avoid this. As with the AC evaluation, the median Rrs values from the same 30 × 30 m grid were used. Algorithm performance was evaluated using root mean squared error (RMSE), median absolute percentage difference (MAPD), bias (mean average difference, MAD), slope and R2. All metrics were first calculated in log-space and then converted back to linear space as recommended by Seegers et al. (2018).
To test the capability of the algorithms to detect algal blooms, records were compiled from data recorded by SEPA and the citizen science application Bloomin’ Algae. Both sources included binary ‘bloom’/‘no bloom’ data. Specifically, SEPA record algal cell counts, where they define blooms to be cyanobacterial concentrations of more than 105 cells mL-1. On request of the University of Stirling, SEPA generally do this analysis on samples taken in the aftermath of a large bloom, to check if recreational activities can be restarted. Therefore, the majority of the SEPA records were in the “no bloom” category. Bloomin’ Algae is a mobile phone application developed by the United Kingdom Centre for Ecology & Hydrology (UKCEH) which enables members of the public to report sightings of algal blooms. Blooms are reported by uploading photos with some brief contextual notes to an online database. All bloom reports are regularly reviewed by experts at UKCEH, and categorised as either ‘confirmed’, ‘plausible’, or ‘incorrect’. For this study, only reports of blooms categorised as ‘confirmed’ were used. Together, these bloom reports allowed for testing of the ability of the SuperDoves and Sentinel-2 sensors to detect blooms in Airthrey Loch. Specifically, each of the bloom records was matched with the nearest satellite image, allowing ±1 day for match-ups. The chl-a values were calculated for each of these images using the best performing chl-a algorithms previously identified for Sentinel-2 MSI and SuperDoves. The median value from the Western basin of the lake was used as all the bloom records used for this study were located at that side of the lake, as well as this being the in situ sampling location. This chl-a data was classified as either “bloom” or ‘no bloom’ based on a threshold. For Airthrey Loch, there has been no work which defines an algal bloom threshold based on chl-a levels, and so the threshold was varied from 0 to 55 μg L−1. For each of these thresholds, the F1 score, a standard measure of classification performance, was calculated. Class imbalances were accounted for through weighting (Christen et al., 2023).
To directly investigate the effect of spatial resolution on the retrieval of chl-a across the water body we followed a similar procedure to Li et al. (2024). This consisted of a comparison between the 3 m resolution SuperDoves chl-a image from the 29th of May 2023 with down-sampled images of 10 m and 20 m resolution generated using the same data. Histograms were used to compare the overall distribution of chl-a values in each scenario.
To investigate the capability of SuperDoves to detect algal blooms in other small water bodies, five small water bodies in central Scotland within a 35 km radius of Airthrey Loch were selected for additional analysis (Table 2). All water bodies selected were less than 1 km2, and had at least one confirmed Bloomin’ Algae bloom record. For each water body, the closest available SuperDoves image to a bloom report from either 2023 or 2024 was compared with an image from the end of January 2023. Algal blooms very rarely occur in Scottish water bodies at this time of year, and so these images act as a low-chl-a baseline (May et al., 2022). For each image, the OC3 algorithm with the coefficients derived earlier in the study was used to visualise the chl-a distribution over each water body, and lake-median chl-a values calculated. Qualitative comparisons were made between the baseline and bloom images to examine the correspondence between Bloomin’ Algae records and high chl-a concentrations measured using SuperDoves.
Table 2. Location, area and mean depth of water bodies used for additional analysis (Hughes et al., 2004).
Most AC models performed better for the bands located between 490 and 666 nm for both MSI and SuperDoves sensors (Figure 3). In the majority of SuperDoves bands, ACOLITE products showed consistently higher slopes and biases than those derived from the Planet SR product, whereas the Planet SR product generally had lower RMSE and MAD (Figures 3, 4). On average, these two AC algorithms ranked equally and so both were considered for subsequent analyses.
Figure 3. Atmospheric correction performance metrics: R2, slope, RMSE, MAPD, and MAD plotted against wavelength for both SuperDoves (right column) and Sentinel-2 (left column) match-up data. For clarity, only the best performing of the C2RCC algorithms (C2X Complex Nets) is shown. Blue lines indicate errors associated with ACOLITE, orange lines C2X Complex Nets, green lines Polymer, and red lines the planet surface reflectance product. (a) S2. (b) SD. (c) S2. (d) SD. (e) S2. (f) SD. (g) S2. (h) SD. (i) S2. (j) SD.
Figure 4. SuperDoves remote sensing reflectance plotted against in situ remote sensing reflectance measurements for the two tested atmospheric correction algorithms. Each marker represents a separate acquisition. Blue circles represent ACOLITE corrected data and orange crosses the Planet surface reflectance data. The solid coloured lines plotted were generated from ordinary least squares linear regression, and the black dashed line is the 1:1 line. (a) 444 nm. (b) 492 nm. (c) 533 nm. (d) 566 nm. (e) 612 nm. (f) 666 nm. (g) 707 nm. (h) 866 nm.
For the Sentinel-2 data, on average across all wavebands the highest-ranking algorithm was C2X_COMPLEX_nets, the C2RCC algorithm designed for complex waters. Whilst Polymer did outperform this algorithm at certain wavebands, C2X_COMPLEX_nets performed more consistently across all wavebands, and had very few outliers (Figures 3, 5).
Figure 5. Sentinel-2 remote sensing reflectance plotted against in situ remote sensing reflectance measurements for the tested atmospheric correction algorithms. Each marker represents a separate acquisition. Blue circles represent ACOLITE corrected data, orange squares C2X Complex Nets corrected data, and green crosses are data corrected with Polymer. The solid coloured lines plotted were generated from ordinary least squares linear regression, and the black dashed line is the 1:1 line. For clarity, only the best performing of the C2RCC algorithms (C2X_COMPLEX_NETS) is shown. (a) 443 nm. (b) 492 nm. (c) 560 nm. (d) 665 nm. (e) 704 nm. (f) 865 nm.
For the Sentinel-2 data, all algorithms had statistically significant fits (p ≤ 0.01) when regressed to the in situ data (Figure 6). In contrast, none of the chl-a algorithms evaluated with the Planet surface reflectance product had statistically significant fits (p > 0.05). For this reason, only the SuperDoves data corrected with ACOLITE was considered subsequently. Tuning to in situ data gave statistically significant fits (p ≤ 0.01) and moderately high R2 values (>0.5) for all algorithms with Sentinel-2 data, whereas the only statistically significant tuned SuperDoves algorithms were OC3 (p ≤ 0.001) and RBR3 (p ≤ 0.05) (Figure 6). Out of these, only OC3 had R2 > 0.5. The best performing algorithm overall was the SuperDoves OC3, which had the lowest RMSE and MAPD of 0.93 μg L−1 and 17.99%, highest R2 of 0.64, and slope of 0.64. The best performing Sentinel-2 algorithm was NDCI, which had the second highest R2 of 0.61, slope of 0.61, and second lowest RMSE of 1.01 μg L-1 overall.
Figure 6. Satellite chl-a plotted against in situ measurements. Satellite chl-a was generated with the following algorithms: (a) OC3, (b) OC2, (c) NIR, (d) NDCI, (e) RBD, and (f) RBR3. Sentinel-2 data are indicated by orange crosses and SuperDoves by blue circles. Black dashed lines are the 1:1 lines, and the solid lines are the lines of best fit generated from log-log least squares regression. RMSE, MAPD and R2 values from log-space linear regression are displayed for each sensor, alongside an indication of the statistical significance level (ns) - p > 0.05; * - p ≤ 0.05; ** - p ≤ 0.01; *** - p ≤ 0.001.
The algal bloom history of Airthrey Loch since 2021 was visualised using the full timeseries of SuperDoves OC3 and Sentinel-2 NDCI chl-a data (Figure 7). As expected of the higher temporal imagery, SuperDoves had more cloud free images during the study period than Sentinel-2. Between January 2021 and May 2024 there were a total of 191 cloud free SuperDoves images of the loch which equates to, on average, 56 per year. For Sentinel-2 there were 87 cloud free images, equating to approximately 25 per year. In 2021 there was some discrepancy between Sentinel-2 and SuperDoves, which is particularly obvious in July. However, from 2022 onwards both sensors show similar trends. The combined Bloomin’ Algae and SEPA bloom records consisted of 10 reports on separate dates, six of which were labelled as blooms. All six of these dates correspond to peaks in the chl-a time series generated from SuperDoves and Sentinel-2 data (Figure 7). Likewise, all the ‘no bloom’ records corresponded with lower chl-a values.
Figure 7. Median chl-a time series from Western basin of Airthrey loch generated with SuperDoves OC3 (solid blue line) and Sentinel-2 NDCI (dashed orange line). Purple triangles (SEPA records) and green circles (Bloomin’ Algae data) indicate bloom presence if placed in the upper section of the plot, and bloom absence if placed in the lower section.
The clear separation in chl-a values between “bloom” and “no bloom” events is reflected in the F1 scores (Figure 8). For SuperDoves, a perfect score of 1.0 was obtained with the bloom threshold set between 20–30 μg L−1, and for Sentinel-2 this was obtained in the 10–30 μg L−1 range. Performance is still very strong with F1 score >0.85 if the threshold is set between 10–45 μg L−1. This indicates that both sensors, with their associated best-performing atmospheric correction and chl-a algorithms, could detect the presence and absence of algal blooms reported by SEPA and citizen scientists in the Western basin of the lake.
Figure 8. F1 Scores from bloom detection task for SuperDoves OC3 (blue solid line) and Sentinel-2 NDCI (orange dashed line) across a range of chlorophyll-a bloom thresholds.
The difference between low and high chl-a states is evident when comparing images from mid-May to early June (Figure 9). All sensors clearly depict this variation, with the early June images showing higher chl-a concentrations. There is a noticeable correlation in the spatial pattern of the bloom observed in early June, where the highest concentrations, excluding shorelines, tend to be in the northwest part of the lake. However, estimates from Sentinel-2 NDCI imagery using the C2X_COMPLEX_nets algorithm are significantly lower compared to those from the SuperDoves OC3 imagery. In the images from the 4th June, the chl-a distribution in the narrow section of the Eastern side of the lake is very different in all three of the chl-a maps. The Sentinel-2 NDCI image corrected with C2X_COMPLEX_nets shows extremely low chl-a values, whereas the Sentinel-2 OC3 ACOLITE-corrected image is dominated by very high chl-a in this part of the water body. In contrast, the corresponding SuperDoves image shows a more discernible pattern: higher chl-a concentrations largely accumulated on the Western banks of the narrow section of the loch, with lower chl-a on the opposite bank. Notably, at the narrowest points, this section of the lake is approximately only 30 m wide, which corresponds to ten SuperDoves pixels, but only three 10 m Sentinel-2 pixels. Furthermore, the Sentinel-2,443 nm and 705 nm bands have lower resolutions of 60 and 20 m respectively. This has the consequence that parts of this narrow section are likely not properly resolvable with Sentinel-2 using NDCI and OC3 as the lower resolution bands are used for chl-a retrieval. In addition to the apparent issues with the narrow section of the loch, the Sentinel-2 NDCI image corrected with C2X_COMPLEX_nets from the 4th of June has a distinctive adjacency-effect in the Western basin. The perimeter of the basin has a narrow ring of very high chl-a values which are encircled by a ring of very low chl-a values around the perimeter of the basin.
Figure 9. Satellite chl-a maps generated with SuperDoves ACOLITE corrected OC3 (top row), Sentinel-2 C2X_COMPLEX_nets corrected NDCI (second row from top), Sentinel-2 ACOLITE corrected OC3 (second row from bottom). The bottom row shows the difference images between the first two rows. Image median (M) values are given for each of the chl-a plots and RMSE values are given for the difference images.
Analysis of the SuperDoves image from 2023 to 05-29 showed clear differences between the distribution of chl-a values when the image was down-sampled from 3 m to 10 m and 20 m spatial resolutions (Figure 10). Notably, at 10 m resolution there are clear peaks at approximately 15 and 20 μg L-1, whereas in the 3 m resolution image, the distribution in this region is more even. Similarly, the high values of chl-a at 20 m resolution are exclusively located at approximately 40 and 60 μg L−1, whereas high values in the 3 m image are distributed throughout this region.
Figure 10. Comparison of SuperDoves chl-a image from 2023 to 05-29 at three different spatial resolutions (top: 3.0 m, middle: 10.0 m, bottom: 20.0 m). Histograms show the frequency of chl-a values over the entire image in each scenario.
For all five water bodies there was a clear distinction between the baseline images from January 2023 and those acquired either shortly before or after confirmed Bloomin’ Algae bloom records (Figure 11). Castlehill Reservoir and Linlithgow Loch showed the largest median chl-a differences of 25 and 27 μgL−1 between the bloom and no-bloom images. In contrast, the differences between bloom and no-bloom images in Loch Fitty and Gartmorn Dam were lowest - both 12 μgL−1. Nonetheless, all bloom images show localised chl-a features of above 30 μgL−1, which are not present the baseline images.
Figure 11. Comparison of Superdoves OC3 chl-a images from five small water bodies in central Scotland. The left column shows images from late January, and the right column shows images captured on dates close to Bloomin’ Algae bloom reports. Median (M) chl-a values for each image are also shown. (a) Image date: 2023-01-26. (b) Image date:2023-09 08 Bl oom report: 2023-09-10. (c) Image date:2023-0125. (d) Image date:2024-07-31 Bloom n!JIOrl:: 2024-07-31. (e) lmilge date: 2023-01-26. (f) Image date: 2023·10 23 Bloom report: 2023-10-28. (g) Image date: 2023 01-25. (h) Image dm:2023-08-07 Bloom n!JIC)rt:: 2023 08-10. (i) Image date: 2023-01-25. (j) Image date: 2023 08-07 Bloom report: 2023-08-08.
At present, algorithms for AC over water and retrieval of water quality parameters such as chl-a have not been widely tested for eight-band SuperDoves imagery. At the time of writing, this has only been done in the present study and by Vanhellemont (2023) for a coastal environment. There are currently only two available AC algorithms for SuperDoves, and of these, only ACOLITE is intended for aquatic applications. Thus, there were notable differences in the performance of AC algorithms available for Sentinel-2 and SuperDoves. Notably, where ACOLITE suffered from elevated errors at higher wavebands above 700 nm, the C2X Complex Nets algorithm generally performed more consistently across the spectrum (Figure 3). This discrepancy in error across wavelengths provides a plausible explanation as to why the NIR, RBD and NDCI algorithms (that use the ratio or difference between the 666 and 707 nm bands) were not sensitive to changes in chl-a. The finding that the ACOLITE-corrected Sentinel-2 data also showed a similar error pattern across wavebands suggests that this issue may be partly due to AC limitations. However, it is likely that this error may have other sources such as the relatively low signal-to-noise ratio of SuperDoves (Valenzuela et al., 2024). This is a general issue for microsatellite constellations given their small size and limited power (Siddique, 2024). Furthermore, the correspondence between in situ and satellite Rrs in the 443/444 nm band was poor, with negative correlations in both SuperDoves and Sentinel-2 data. This is not unusual - it is very common for AC procedures to perform poorly over coastal and inland waters at the blue-end of the visible spectrum (Lv et al., 2024). Typically this is due to difficulties in estimating the contribution of strongly absorbing aerosols at these wavelengths (Kahn et al., 2016). In comparison, Vanhellemont (2023) found that the ACOLITE correction was effective across all eight wavebands. Notably, the errors in the 444 nm, 707 nm and 866 nm bands were significantly lower than those found in the present study. This discrepancy could be due to dissimilarities between the study sites (coastal compared with inland water), and possibly differences in the atmospheric conditions associated with each of these (Pahlevan et al., 2021). However, for another coastal environment, Dogliotti et al. (2024) also found issues with AC of SuperDoves in the 444 and 866 nm bands. A likely source of this error is sun glint, which can be corrected using SWIR (900–1700 nm) bands which SuperDoves lacks (Lavigne et al., 2023). Whilst NIR bands have been used successfully for glint correction of SuperDoves imagery over clear waters, further developments are required to improve this capability for turbid waters (Vanhellemont, 2023).
Despite challenges associated with AC, with the best algorithms, the SuperDoves chl-a retrieval error was generally lower than that of Sentinel-2, indicating the suitability of this data for phytoplankton monitoring in small water bodies such as Airthrey Loch. Retrieval of chl-a in this study was inevitably affected by errors arising from various sources. Firstly, there was a consistent discrepancy between the location of in situ sampling and the satellite pixels that this was compared with. Furthermore, the timing of sampling did not consistently match with satellite acquisition times–many chl-a samples were taken approximately 24 h before or after the corresponding satellite acquisition. Whilst this is an important error source, both sensors had similar numbers of same-day and 1-day difference match-ups (Table 1). Therefore, whilst this has the consequence of a chl-a retrieval uncertainty comparable, if not greater, in magnitude to that of the average variation in ±24 h, the sensor comparison is nonetheless valid. Furthermore, using this approach, we were able to use both sensors to detect algal blooms recorded by SEPA and Bloomin’ algae in a reliable way across a range of chl-a thresholds. An obvious issue with defining algal blooms using a simple metric such as a chl-a threshold is that this does not necessarily correlate well with qualitative records such as the Bloomin’ Algae records which are verified using user-uploaded photos of cyanobacterial scums. Given that these scums can sometimes be relatively small in area, it is possible for reports of blooms to correspond with images with a relatively low median chl-a. Whilst this did not appear to be an issue for the Airthrey Loch data that was analysed, this was apparent for some of the images of other water bodies. Specifically, Loch Fitty and Gartmorn Dam both had relatively low median chl-a measurements close to dates corresponding to Bloomin’ Algae bloom reports (Figure 11). Reliably detecting smaller scums near to water body edges using satellite imagery is challenging, because emergent vegetation are commonly found in marginal areas, and can contribute to the chl-a signal detected by a satellite sensor (Jiang et al., 2023; Qing et al., 2020). For cyanobacteria monitoring applications, it can be beneficial to make use of the phycocyanin absorption peak at ∼ 620 nm, a spectral feature specific to cyanobacteria (Beck et al., 2017). This enables distinguishing cyanobacterial scums from emergent vegetation or other chl-a sources such as green algae. Whilst Sentinel-2 does not have a band configuration which supports this, SuperDoves have a band centred at 612 nm and so could potentially be used for high resolution cyanobacteria-specific monitoring.
The spatial resolution analysis of the SuperDoves chl-a data over Airthrey Loch showed that the difference in resolution between SuperDoves and Sentinel-2 is important (Figure 10). Specifically, down-sampling SuperDoves from 3 m to 10 m or 20 m, resulted in chl-a distributions with notable differences, including higher occurrences of lower to moderate values. Visually, this appears as a blurring of the patches of higher chl-a concentration, which could prevent detection of smaller scums, pointing to a clear benefit of the higher resolution imagery for algal bloom monitoring applications. Whilst higher resolution imagery typically has a lower signal to noise ratio (Niroumand-Jadidi et al., 2022), this is likely less important for detecting high concentrations of algae, where the chl-a signal is typically strong. Therefore, for algal bloom detection and tracking, the higher spatial resolution offered by the SuperDoves is beneficial. Furthermore, the lower resolution Sentinel-2 NDCI data showed some apparent adjacency-effects in the wider basin. The very low chl-a values at the edge of the water body in the Sentinel-2 NDCI images likely included 20 m NIR pixels (705 nm) which overlap both water and land. For such pixels, high NIR reflectance would be expected as the shoreline of the lake has significant vegetation cover (Huang et al., 2021). With the NDCI algorithm, high NIR reflectance should produce high chl-a values, and therefore the low chl-a values indicate an over-correction in the NIR by the C2X_COMPLEX_nets algorithm. Whilst these are only issues for a relatively small portion of Airthrey Loch, they would likely be more prevalent for smaller lakes where narrow geometries, edge-adjacent pixels, and mixed pixels tend to make up a larger portion of the total area (Jiang et al., 2023). Therefore, our results corroborate and extend upon those of Wasehun et al. (2025), indicating that a key advantage of the higher spatial resolution SuperDoves data is that this reduces distortion in the distribution of measured chl-a values and reduces the extent of adjacency-effects.
The finding that gross changes in water quality associated with the presence and absence of algal blooms were detectable in several small water bodies indicates promise for the wider applicability of SuperDoves data for algae monitoring. Still, there are various sources of uncertainty associated with this analysis which are difficult to quantify without additional in situ data. For example, in shallow water bodies bottom effects may contribute an unwanted component to the chl-a signal (Ma et al., 2011). Given that most of the water bodies considered in this study were relatively shallow, this was likely a source of error for them all (Table 2). With sufficient in situ chl-a data, it is possible to correct for bottom effects and other errors (Zhang et al., 2018), however this poses a specific challenge when considering using high resolution satellite imagery for widespread water quality monitoring: given the sheer number of small water bodies, gathering sufficient data for validating phytoplankton monitoring schemes and algal bloom detection systems is potentially difficult. Furthermore, routine monitoring programs tend to be reserved for larger, more economically significant lakes (Biggs et al., 2017). However, the efficacy of using irregular records from reactive environmental monitoring and citizen science to validate satellite monitoring (Figures 7, 11) suggests that this approach could be used more widely than currently for comparing with remotely sensed data. Satellite imagery can suffer from errors and missing data due to atmospheric effects, cloud cover, confounding (non-algal) chl-a sources, and adjacency effects (Zahir et al., 2024). Likewise, citizen science data are likely to be collected somewhat irregularly, possibly with inconsistencies, and uncertainty arising from human error and biases (Pocock et al., 2023). However, as their sources of error are very much independent from each other, together these data complement each other very well. Therefore, further exploring ways to use these data alongside each other would offer a richer, more complete way of monitoring and understanding aquatic ecosystems than either would be capable of alone (Putman et al., 2023).
Alongside spatial resolution, another clear advantage of the SuperDoves data is that of temporal resolution. Given that SuperDoves images are acquired daily, this imagery could be expected to give approximately a five-fold increase in the number of useable images compared with Sentinel-2. However, only slightly more than twice as many useable images were obtained with SuperDoves. This is largely a reflection of a challenge associated with using optical remote sensing data at high latitudes (e.g., central Scotland) where significant cloud cover is common throughout the year (McGrath et al., 2023). On average, approximately one cloud-free SuperDoves image was obtained each week. Although a significant improvement on the Sentinel-2 data, this is far from the daily observations which Dubelaar et al. (2004) suggest are required to follow ecosystem dynamics of phytoplankton in detail. Therefore, for regions like Scotland with consistent and regular cloud cover, higher frequency monitoring data would likely be best obtained using automated in situ measurement platforms (Marcé et al., 2016). However, even in areas where satellite temporal coverage is limited by cloud cover, there is still the potential to collect far more data than would be feasible with manual sampling efforts. Whilst there were some errors and discrepancies between the SuperDoves and Sentinel-2 chl-a estimates, we were still able to detect gross changes in water quality. Therefore, if applied on a larger scale, this capability could be of significant use to assist regulatory agencies with targeting sampling efforts to reduce operational costs.
Finally, it is worth noting that Planet SuperDoves data are not freely available, except for small-scale research projects, such as the present study (Planet Labs PBC, 2018). Whilst Planet have various pricing structures available, including discounts for non-profit organizations, it is still possible that certain projects such as global lake studies or implementation of widespread algal bloom monitoring programs might be prohibited by cost. It is therefore very likely that freely available products such as Sentinel-2 and Landsat will continue to be widely used for water quality monitoring applications even as higher resolution imagery becomes increasingly attractive.
Our study highlights several key findings and challenges associated with using the Planet SuperDoves satellite constellation for monitoring phytoplankton in a small, eutrophic lake. Despite some challenges, it was found that SuperDoves data are competitive with Sentinel-2 for this application and have distinct advantages arising from higher spatial and temporal resolution. We have shown that the higher spatial resolution offered by SuperDoves can enable monitoring of a vast number of smaller lakes, which has important implications for the field of limnology, environmental monitoring, and public health protection efforts. Furthermore, it has been demonstrated that alongside irregular environmental agency records, citizen science data can be a valuable complement to satellite imagery. This points to a low-cost means of validating widespread monitoring data, whilst also generating an increased sense of shared environmental stewardship in society. Ultimately, while high-resolution satellite data like SuperDoves offer great potential for monitoring phytoplankton dynamics, they should complement, rather than replace other data collection methods. Such a combined approach could enable monitoring of a huge number of unstudied lakes, and therefore provide critical data to improve the management and conservation of small, sensitive aquatic ecosystems at a scale previously impossible.
The datasets presented in this article are not readily available because Data underlying the results presented in this paper are not publicly available but may be obtained from the author after agreement with the original data provider. Requests to access the datasets should be directed toZGFhNUBzdGlyLmFjLnVr.
DA: Conceptualization, Data curation, Investigation, Methodology, Software, Validation, Visualization, Writing–original draft, Writing–review and editing. ES: Conceptualization, Funding acquisition, Methodology, Project administration, Resources, Supervision, Writing–review and editing. PH: Conceptualization, Funding acquisition, Methodology, Project administration, Resources, Supervision, Writing–review and editing. IJ: Conceptualization, Funding acquisition, Methodology, Project administration, Resources, Supervision, Writing–review and editing.
The author(s) declare that financial support was received for the research, authorship, and/or publication of this article. This work was supported by the Hydro Nation Scholars Programme funded by the Scottish Government and managed by the Hydro Nation International Centre.
We thank the European Space Agency, Planet Labs PBC, the United Kingdom Centre for Ecology and Hydrology, and the Scottish Environment Protection Agency for the provision of data necessary for this study.
The authors declare that the research was conducted in the absence of any commercial or financial relationships that could be construed as a potential conflict of interest.
The author(s) declare that no Generative AI was used in the creation of this manuscript.
All claims expressed in this article are solely those of the authors and do not necessarily represent those of their affiliated organizations, or those of the publisher, the editors and the reviewers. Any product that may be evaluated in this article, or claim that may be made by its manufacturer, is not guaranteed or endorsed by the publisher.
Alejandre, J. C. P., Irvine, K. N., Chastin, S., Pfleger, S., Rendall, J., and Helwig, K. (2024). Scotland’s model of a blue-green prescribing programme for primary mental healthcare: participatory co-creation research with Q-Methodology. Lancet 404, S95. doi:10.1016/S0140-6736(24)02040-3
Beaulne, D., and Fotopoulos, G. (2024). Development of an algal bloom satellite and in situ metadata hub with case studies in Canada. Ecol. Inf. 79, 102447. doi:10.1016/j.ecoinf.2023.102447
Beck, R., Xu, M., Zhan, S., Liu, H., Johansen, R. A., Tong, S., et al. (2017). Comparison of satellite reflectance algorithms for estimating phycocyanin values and cyanobacterial total biovolume in a temperate reservoir using coincident hyperspectral aircraft imagery and dense coincident surface observations. Remote Sens. 9, 538. doi:10.3390/rs9060538
Biggs, J., von Fumetti, S., and Kelly-Quinn, M. (2017). The importance of small waterbodies for biodiversity and ecosystem services: implications for policy makers. Hydrobiologia 793, 3–39. doi:10.1007/s10750-016-3007-0
Cael, B. B., and Seekell, D. A. (2016). The size-distribution of Earth’s lakes. Sci. Rep. 6, 29633. doi:10.1038/srep29633
Christen, P., Hand, D. J., and Kirielle, N. (2023). A review of the F-measure: its history, properties, criticism, and alternatives. ACM Comput. Surv. 56 (73), 1–24. doi:10.1145/3606367
Dogliotti, A. I., Piegari, E., Rubinstein, L., Perna, P., and Ruddick, K. G. (2024). Using the automated HYPERNETS hyperspectral system for multi-mission satellite ocean colour validation in the Río de la Plata, accounting for different spatial resolutions. Front. Remote Sens. 5. doi:10.3389/frsen.2024.1354662
Downing, J. A. (2010). Emerging global role of small lakes and ponds: little things mean a lot. Limnetica 29, 9–24. doi:10.23818/limn.29.02
Dubelaar, G. B. J., Geerders, P. J. F., and Jonker, R. R. (2004). High frequency monitoring reveals phytoplankton dynamics. J. Environ. Monit. 6, 946–952. doi:10.1039/B409350J
Dugan, H. A., Bartlett, S. L., Burke, S. M., Doubek, J. P., Krivak-Tetley, F. E., Skaff, N. K., et al. (2017). Salting our freshwater lakes. Proc. Natl. Acad. Sci. 114, 4453–4458. doi:10.1073/pnas.1620211114
Evaristo, J., Jameel, Y., Tortajada, C., Wang, R. Y., Horne, J., Neukrug, H., et al. (2023). Water woes: the institutional challenges in achieving SDG 6. Sustain. Earth Rev. 6, 13. doi:10.1186/s42055-023-00067-2
Freitas, F. H., and Dierssen, H. M. (2019). Evaluating the seasonal and decadal performance of red band difference algorithms for chlorophyll in an optically complex estuary with winter and summer blooms. Remote Sens. Environ. 231, 111228. doi:10.1016/j.rse.2019.111228
Gitelson, A. (1992). The peak near 700 nm on radiance spectra of algae and water: relationships of its magnitude and position with chlorophyll concentration. Int. J. Remote Sens. 13, 3367–3373. doi:10.1080/01431169208904125
Heino, J., Alahuhta, J., Bini, L. M., Cai, Y., Heiskanen, A.-S., Hellsten, S., et al. (2021). Lakes in the era of global change: moving beyond single-lake thinking in maintaining biodiversity and ecosystem services. Biol. Rev. Camb Philos. Soc. 96, 89–106. doi:10.1111/brv.12647
Hooker, S., Van Heuklem, L., Thomas, C., Claustre, H., Ras, J., Schluter, L., et al. (2009). The third SeaWiFS HPLC analysis round-robin experiment (SeaHARRE-3). Greenbelt, Maryland (United States of America).
Huang, S., Tang, L., Hupy, J.P., Wang, Y., and Shao, G. (2021). A commentary review on the use of normalized difference vegetation index (NDVI) in the era of popular remote sensing. J. For. Res. 32, 1–6. doi:10.1007/s11676-020-01155-1
Hughes, M., Hornby, D. D., Bennion, H., Kernan, M., Hilton, J., Phillips, G., et al. (2004). The development of a GIS-based inventory of standing waters in great britain together with a risk-based prioritisation protocol. Water, Air, and Soil Pollut. Focus 4, 73–84. doi:10.1023/B:WAFO.0000028346.27904.83
Huisman, J., Codd, G. A., Paerl, H. W., Ibelings, B. W., Verspagen, J. M. H., and Visser, P. M. (2018). Cyanobacterial blooms. Nat. Rev. Microbiol. 16, 471–483. doi:10.1038/s41579-018-0040-1
Inácio, M., Barceló, D., Zhao, W., and Pereira, P. (2022). Mapping lake ecosystem services: a systematic review. Sci. Total Environ. 847, 157561. doi:10.1016/j.scitotenv.2022.157561
Jiang, D., Matsushita, B., and Yang, W. (2020). A simple and effective method for removing residual reflected skylight in above-water remote sensing reflectance measurements. ISPRS J. Photogrammetry Remote Sens. 165, 16–27. doi:10.1016/j.isprsjprs.2020.05.003
Jiang, D., Scholze, J., Liu, X., Simis, S. G. H., Stelzer, K., Müller, D., et al. (2023). A data-driven approach to flag land-affected signals in satellite derived water quality from small lakes. Int. J. Appl. Earth Observation Geoinformation 117, 103188. doi:10.1016/j.jag.2023.103188
Kahn, R. A., Sayer, A. M., Ahmad, Z., and Franz, B. A. (2016). The sensitivity of SeaWiFS ocean color retrievals to aerosol amount and type. J. Atmos. Ocean. Technol. 33, 1185–1209. doi:10.1175/JTECH-D-15-0121.1
Kelly, L. a., and Smith, S. (1996). The nutrient budget of a small eutrophic loch and the effectiveness of straw bales in controlling algal blooms. Freshw. Biol. 36, 411–418. doi:10.1046/j.1365-2427.1996.00059.x
Kutser, T., and Soomets, T. (2024). Satellite data is revealing long time changes in the world largest lakes. Sci. Rep. 14, 14391. doi:10.1038/s41598-024-65250-7
Lavigne, H., Vanhellemont, Q., Ruddick, K., and Vansteenwegen, D. (2023). Turbid water sun glint removal for high resolution sensors without SWIR, in: remote sensing of the Ocean, sea ice, coastal waters, and large water regions 2023. Present. A. T. Remote Sens. Ocean, Sea Ice, Coast. Waters, Large Water Regions 2023, SPIE, 30–45. doi:10.1117/12.2683912
Lever, J., Krzywinski, M., and Altman, N. (2016). Model selection and overfitting. Nat. Methods 13, 703–704. doi:10.1038/nmeth.3968
Li, J., Li, Y., Yu, Y., Li, J., Cai, X., Lyu, L., et al. (2024). Evaluating the capabilities of China’s new satellite HJ-2 for monitoring chlorophyll a concentration in eutrophic lakes. Int. J. Appl. Earth Observation Geoinformation 126, 103618. doi:10.1016/j.jag.2023.103618
Liu, S., Glamore, W., Tamburic, B., Morrow, A., and Johnson, F. (2022). Remote sensing to detect harmful algal blooms in inland waterbodies. Sci. Total Environ. 851, 158096. doi:10.1016/j.scitotenv.2022.158096
Lv, J., Feng, L., and Zhao, D. (2024). Validation of global gridded aerosol models in inland/coastal water atmospheric correction for MODIS, VIIRS, and Landsat. IEEE Trans. Geoscience Remote Sens. 62, 1–10. doi:10.1109/TGRS.2024.3427836
Ma, R., Duan, H., Liu, Q., and Loiselle, S. A. (2011). Approximate bottom contribution to remote sensing reflectance in Taihu Lake, China. J. Gt. Lakes. Res. 37, 18–25. doi:10.1016/j.jglr.2010.12.002
Mansaray, A. S., Dzialowski, A. R., Martin, M. E., Wagner, K. L., Gholizadeh, H., and Stoodley, S. H. (2021). Comparing PlanetScope to landsat-8 and sentinel-2 for sensing water quality in reservoirs in agricultural watersheds. Remote Sens. 13, 1847. doi:10.3390/rs13091847
Marcé, R., George, G., Buscarinu, P., Deidda, M., Dunalska, J., de Eyto, E., et al. (2016). Automatic high frequency monitoring for improved lake and reservoir management. Environ. Sci. Technol. 50, 10780–10794. doi:10.1021/acs.est.6b01604
May, L., Taylor, P., Gunn, I. D. M., Thackeray, S. J., Carvalho, L., Corr, M., et al. (2022). “Assessing climate change impacts on the water quality of Scottish standing waters,” in CRW2020_01 (Scotland’s Centre of Expertise for Waters CREW).
McGrath, C. N., Cowley, D. C., Hood, S., Clarke, S., and Macdonald, M. (2023). An assessment of high temporal frequency satellite data for historic environment applications. A case study from Scotland. Archaeol. Prospect. 30, 267–282. doi:10.1002/arp.1890
Mishra, S., and Mishra, D. R. (2012). Normalized difference chlorophyll index: a novel model for remote estimation of chlorophyll-a concentration in turbid productive waters. Remote Sens. Environ. Remote Sens. Urban Environ. 117, 394–406. doi:10.1016/j.rse.2011.10.016
Mobley, C. D. (1999). Estimation of the remote-sensing reflectance from above-surface measurements. Appl. Opt. AO 38, 7442–7455. doi:10.1364/AO.38.007442
Naderian, D., Noori, R., Heggy, E., Bateni, S. M., Bhattarai, R., Nohegar, A., et al. (2024). A water quality database for global lakes. Resour. Conservation Recycl. 202, 107401. doi:10.1016/j.resconrec.2023.107401
Naselli-Flores, L., and Padisák, J. (2023). Ecosystem services provided by marine and freshwater phytoplankton. Hydrobiologia 850, 2691–2706. doi:10.1007/s10750-022-04795-y
Neil, C., Spyrakos, E., Hunter, P. D., and Tyler, A. N. (2019). A global approach for chlorophyll-a retrieval across optically complex inland waters based on optical water types. Remote Sens. Environ. 229, 159–178. doi:10.1016/j.rse.2019.04.027
Niroumand-Jadidi, M., and Bovolo, F. (2021). Water quality retrieval and algal bloom detection using high-resolution cubesat imagery. isprs annals of the photogrammetry. Remote Sens. Spatial Inf. Sci. V-3–2021, 191–195. doi:10.5194/isprs-annals-V-3-2021-191-2021
Niroumand-Jadidi, M., Legleiter, C. J., and Bovolo, F. (2022). River bathymetry retrieval from landsat-9 images based on neural networks and comparison to SuperDove and sentinel-2. IEEE J. Sel. Top. Appl. Earth Observations Remote Sens. 15, 5250–5260. doi:10.1109/JSTARS.2022.3187179
O’Reilly, J. E., Maritorena, S., Mitchell, B. G., Siegel, D. A., Carder, K. L., Garver, S. A., et al. (1998). Ocean color chlorophyll algorithms for SeaWiFS. J. Geophys. Res. Oceans 103, 24937–24953. doi:10.1029/98JC02160
Pahlevan, N., Mangin, A., Balasubramanian, S. V., Smith, B., Alikas, K., Arai, K., et al. (2021). ACIX-Aqua: a global assessment of atmospheric correction methods for Landsat-8 and Sentinel-2 over lakes, rivers, and coastal waters. Remote Sens. Environ. 258, 112366. doi:10.1016/j.rse.2021.112366
Pocock, M. J. O., Hamlin, I., Christelow, J., Passmore, H.-A., and Richardson, M. (2023). The benefits of citizen science and nature-noticing activities for well-being, nature connectedness and pro-nature conservation behaviours. People Nat. 5, 591–606. doi:10.1002/pan3.10432
Putman, N. F., Beyea, R. T., Iporac, L. A. R., Triñanes, J., Ackerman, E. G., Olascoaga, M. J., et al. (2023). Improving satellite monitoring of coastal inundations of pelagic Sargassum algae with wind and citizen science data. Aquat. Bot. 188, 103672. doi:10.1016/j.aquabot.2023.103672
Qing, S., A, R., Shun, B., Zhao, W., Bao, Y., and Hao, Y. (2020). Distinguishing and mapping of aquatic vegetations and yellow algae bloom with Landsat satellite data in a complex shallow Lake, China during 1986–2018. Ecol. Indic. 112, 106073. doi:10.1016/j.ecolind.2020.106073
Rajapakse, J., Otoo, M., and Danso, G. (2023). Progress in delivering SDG6: safe water and sanitation. Camb. Prisms Water 1, e6. doi:10.1017/wat.2023.5
Schröder, T., Schmidt, S. I., Kutzner, R. D., Bernert, H., Stelzer, K., Friese, K., et al. (2024). Exploring spatial aggregations and temporal windows for water quality match-up analysis using sentinel-2 MSI and sentinel-3 OLCI data. Remote Sens. 16, 2798. doi:10.3390/rs16152798
Seegers, B. N., Stumpf, R. P., Schaeffer, B. A., Loftin, K. A., and Werdell, P. J. (2018). Performance metrics for the assessment of satellite data products: an ocean color case study. Opt. Express, OE 26, 7404–7422. doi:10.1364/OE.26.007404
Shen, W., Zhang, L., Ury, E. A., Li, S., Xia, B., and Basu, N. B. (2025). Restoring small water bodies to improve lake and river water quality in China. Nat. Commun. 16, 294. doi:10.1038/s41467-024-55714-9
Soriano-González, J., Urrego, E. P., Sòria-Perpinyà, X., Angelats, E., Alcaraz, C., Delegido, J., et al. (2022). Towards the combination of C2RCC processors for improving water quality retrieval in inland and coastal areas. Remote Sens. 14, 1124. doi:10.3390/rs14051124
Steinmetz, F., and Ramon, D. (2018). Sentinel-2 MSI and sentinel-3 OLCI consistent ocean colour products using POLYMER, in: remote sensing of the open and coastal ocean and inland waters. Present. A. T. Remote Sens. Open Coast. Ocean Inland Waters, SPIE, 46–55. doi:10.1117/12.2500232
Sterner, R. W., Keeler, B., Polasky, S., Poudel, R., Rhude, K., and Rogers, M. (2020). Ecosystem services of Earth’s largest freshwater lakes. Ecosyst. Serv. 41, 101046. doi:10.1016/j.ecoser.2019.101046
Valenzuela, A., Reinke, K., and Jones, S. (2024). Assessing the spatial resolution distance of satellite images: SuperDove versus Landsat 8. Int. J. Remote Sens. 45, 4120–4159. doi:10.1080/01431161.2024.2357839
Vanhellemont, Q. (2023). Evaluation of eight band SuperDove imagery for aquatic applications. Opt. Express, OE 31, 13851–13874. doi:10.1364/OE.483418
Vanhellemont, Q., and Ruddick, K. (2018). Atmospheric correction of metre-scale optical satellite data for inland and coastal water applications. Remote Sens. Environ. 216, 586–597. doi:10.1016/j.rse.2018.07.015
Warren, M. A., Simis, S. G. H., Martinez-Vicente, V., Poser, K., Bresciani, M., Alikas, K., et al. (2019). Assessment of atmospheric correction algorithms for the Sentinel-2A MultiSpectral Imager over coastal and inland waters. Remote Sens. Environ. 225, 267–289. doi:10.1016/j.rse.2019.03.018
Wasehun, E. T., Beni, L. H., Di Vittorio, C. A., Zarzar, C. M., and Young, K. R. L. (2025). Comparative analysis of Sentinel-2 and PlanetScope imagery for chlorophyll-a prediction using machine learning models. Ecol. Inf. 85, 102988. doi:10.1016/j.ecoinf.2024.102988
Wevers, J., Müller, D., Scholze, J., Kirches, G., Quast, R., and Brockmann, C. (2021). IdePix for sentinel-2 MSI algorithm theoretical basis document. Zenodo. doi:10.5281/zenodo.5788067
Zabaleta, B., Aubriot, L., Olano, H., and Achkar, M. (2023). Satellite assessment of eutrophication hot spots and algal blooms in small and medium-sized productive reservoirs in Uruguay’s main drinking water basin. Environ. Sci. Pollut. Res. 30, 43604–43618. doi:10.1007/s11356-023-25334-9
Zahir, M., Su, Y., Shahzad, M. I., Ayub, G., Rehman, S. U., and Ijaz, J. (2024). A review on monitoring, forecasting, and early warning of harmful algal bloom. Aquaculture 593, 741351. doi:10.1016/j.aquaculture.2024.741351
Zhang, Y., Duan, H., Xi, H., Huang, Z., Tsou, J., Jiang, T., et al. (2018). Evaluation of the influence of aquatic plants and lake bottom on the remote-sensing reflectance of optically shallow waters. Atmosphere-Ocean 56, 277–288. doi:10.1080/07055900.2018.1454295
AC Atmospheric correction
ACOLITE Atmospheric Correction for Operational Land Imager ‘lite’
AOI Area of interest
chl-a Chlorophyll-a
C2RCC Case 2 Regional Coast Colour
C2RCC_nets General case-2 waters neural network
C2X_COMPLEX_nets Complex extreme case-2 waters neural network
C2X_nets Extreme case-2 waters neural network
DALEC Dynamic Above-water Radiance and Irradiance Collector
ESA European Space Agency
HPLC High performance liquid chromatography
MAD Mean average difference
MAPD Median absolute percentage difference
MSI Multi spectral imager
NASA National Aeronautics and Space Administration
NDCI Normalised difference chlorophyll index
NIR Near infrared
OC Ocean colour
OC2 Ocean colour 2 (NASA chl-a algorithm)
OC3 Ocean colour 3 (NASA chl-a algorithm)
OLI Operational land imager
PBC Public benefit company
POLYMER POLYnomial based algorithm applied to MERIS
RBD Red band difference
RBR3 Red band ratio (3-band chl-a algorithm)
RMSE Root mean squared error
Rrs Remote sensing reflectance
SD SuperDoves
SDG Sustainable development goal
SEPA Scottish Environment Protection Agency
SR Surface Reflectance
SWIR Short wavelength infrared region
S2 Sentinel-2
UDM Useable data mask
UK United Kingdom
UKCEH United Kingdom Centre for Ecology and Hydrology
UTC Coordinated universal time
Keywords: algal blooms, cyanobacteria, sentinel-2, freshwater, planetscope, citizen science
Citation: Atton Beckmann D, Spyrakos E, Hunter P and Jones ID (2025) Widespread phytoplankton monitoring in small lakes: a case study comparing satellite imagery from planet SuperDoves and ESA sentinel-2. Front. Remote Sens. 6:1549119. doi: 10.3389/frsen.2025.1549119
Received: 20 December 2024; Accepted: 07 March 2025;
Published: 27 March 2025.
Edited by:
Zhigang Cao, Chinese Academy of Sciences (CAS), ChinaReviewed by:
Shaohua Lei, Nanjing Hydraulic Research Institute, ChinaCopyright © 2025 Atton Beckmann, Spyrakos, Hunter and Jones. This is an open-access article distributed under the terms of the Creative Commons Attribution License (CC BY). The use, distribution or reproduction in other forums is permitted, provided the original author(s) and the copyright owner(s) are credited and that the original publication in this journal is cited, in accordance with accepted academic practice. No use, distribution or reproduction is permitted which does not comply with these terms.
*Correspondence: D. Atton Beckmann, ZGFuaWVsLmF0dG9uLmJlY2ttYW5uQHN0aXIuYWMudWs=
Disclaimer: All claims expressed in this article are solely those of the authors and do not necessarily represent those of their affiliated organizations, or those of the publisher, the editors and the reviewers. Any product that may be evaluated in this article or claim that may be made by its manufacturer is not guaranteed or endorsed by the publisher.
Research integrity at Frontiers
Learn more about the work of our research integrity team to safeguard the quality of each article we publish.