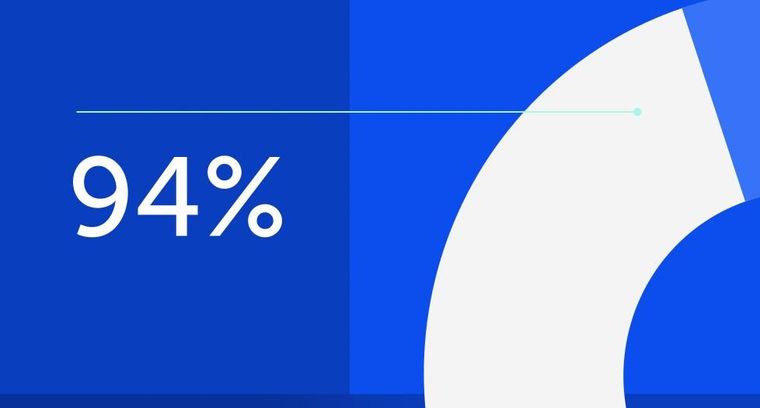
94% of researchers rate our articles as excellent or good
Learn more about the work of our research integrity team to safeguard the quality of each article we publish.
Find out more
ORIGINAL RESEARCH article
Front. Remote Sens., 08 November 2024
Sec. Remote Sensing Time Series Analysis
Volume 5 - 2024 | https://doi.org/10.3389/frsen.2024.1492534
Introduction: Factors such as (1) the number of satellite images available for a specific study and (2), the applicability of these images in terms of cloud cover, can reduce the overall accuracy of time series studies from earth observation. In this context, the Landsat 8 dataset stands out as one of the most widely used and versatile datasets for time series analysis, building on the strengths of its predecessors with its advanced features. However, despite these enhancements, there is a significant gap in the literature regarding a comprehensive assessment of Landsat 8’s performance. Specifically, there is a need for a detailed evaluation of image availability and cloud cover percentages across various global paths and rows.
Methods: To address this gap, we utilized the Landsat 8 Collection 2 dataset available through Google Earth Engine (GEE). Our approach involved filtering the dataset to focus on Landsat 8 images captured between 2014 and 2023 across all paths and rows. Using the Earth Engine Python API, we accessed and processed this data, extracting key information such as the number of available images and their associated cloud cover percentages.
Results and Discussion: Our analysis of Landsat 8 image availability revealed that regions such as Australia, parts of Africa, the Middle East, Western Asia, and Southern North America benefit from a higher frequency of Landsat imagery, while Northern Asia and Northern North America have fewer images available. Latitude-specific trends show that areas between 55 and 82 degrees receive notably fewer images. We also found that regions like central Australia, northern Africa, and the Middle East generally experience lower cloud cover, while central Africa, and northern parts of Asia, Europe, and North America have higher cloudiness. Latitudinal trends show a significant drop in cloud cover from approximately 90% at latitudes -60 to -20 degrees to below 10%, with a rise near the Equator. Cloud cover decreases again from 0 to 20 degrees latitude but increases between 20 and 60 degrees. Europe has the highest average cloud cover at 42.5%, impacting image clarity, whereas Africa has the lowest average at 23.3%, indicating clearer satellite imagery.
Identifying and describing alterations over time represents the initial instinctive action in pinpointing the catalyst behind such transformations and comprehending the process of change. Satellite remote sensing has historically served as an effective tool for detecting and categorizing alterations in the state of the Earth’s surface across periods (Orusa et al., 2023a; Verbesselt et al., 2010). Continuous Earth Observation time series provide valuable insights into the fluctuations of vegetation over time, spanning from regional to global scales. However, the estimation of trends relies on several factors, including the length of the time series, its temporal and spatial resolution, the quality of the measured data, and the statistical method employed (Forkel et al., 2013). Ensuring the availability of accurate and consistent datasets is a fundamental principle in guaranteeing reliable results for vegetation monitoring and trend analysis (Huang et al., 2021; Liu et al., 2018; Qiu et al., 2015; Rahimi, 2024; Tian et al., 2019; Verbesselt et al., 2010; Waldner et al., 2015).
The effectiveness of image classification is most clearly demonstrated by using multiple images of the same region acquired over time. Numerous studies have highlighted the value of multi-temporal satellite images in improving the accuracy of classifying various types of vegetation (Lasaponara and Lanorte, 2012; Orusa et al., 2024a; b; Verbesselt et al., 2010). Different vegetation types exhibit distinct phenologies, meaning they have different life cycles. As a result, vegetation can be more easily distinguished when using multi-temporal satellite images compared to analyzing a single image (Guyet and Nicolas, 2016). Over the past decades, satellite image series have been captured either with high temporal resolution (daily coverage at a kilometer-scale spatial resolution) or with high spatial resolution (weekly coverage at a meter-scale spatial resolution) (Petitjean et al., 2012; Simoes et al., 2021).
However, land cover mapping in regions with frequent rain and cloud cover, presents significant challenges, particularly when dealing with small agricultural parcels. Clouds and their shadows often reduce the effectiveness of optical satellite imagery and complicate time series analysis (Hu et al., 2020; Hu et al., 2019; Jain et al., 2013; Rahimi, 2024; Rahimi and Jung, 2024). Detecting and removing clouds has long been a critical concern in remote sensing image processing. Earlier methods for cloud removal primarily focused on individual or small-scale images, constrained by limited data availability and less accurate cloud detection techniques (Hu et al., 2020; Li et al., 2019; Rahimi et al., 2021; Zhao et al., 2022). Detecting clouds is, therefore, one of the initial challenges faced when attempting to automatically process optical remote sensing data.
In a global analysis, King et al. (2013) collected cloud data from the Moderate Resolution Imaging Spectroradiometer (MODIS) over 12 years from Terra and over 9 years from Aqua. They found that MODIS estimated the cloud fraction at approximately 67%, with a slightly higher cloud coverage over land in the afternoon and less over the ocean during the same period globally. There was a minimal difference in global cloud cover between the Terra and Aqua satellites. Specifically, the cloud fraction over land averaged around 55% and exhibited a distinct seasonal pattern, while ocean cloudiness was notably higher at about 72% with less seasonal variation. Both Aqua and Terra showed similar zonal cloud top pressures. Therefore, in optical remote sensing studies based on time series analysis, we encounter two primary challenges: 1) the availability of data, or simply the number of satellite images for the study area, and 2) the usability of these images, which is often impacted by cloud cover or the presence of clouds.
Landsat holds the distinction of being the longest-running Earth observation satellite program at medium resolution, with its first images captured in 1972. The Landsat series offers the most extensive temporal record of space-based surface observations, with data collected from most land areas at least once per year since 1972. The release of the Landsat archive by the United States Geological Survey (USGS) in 2008 significantly increased the scientific use of Landsat data. However, Landsat missions are designed to revisit the same location every 16 days, and various factors like weather conditions and satellite-related issues can impact data collection (Orusa et al., 2023b; Rahimi et al., 2022; Tolnai et al., 2016; Zhang et al., 2022).
Landsat-8 was successfully launched on 11 February 2013. The satellite is equipped with the Operational Land Imager (OLI) and Thermal Infrared Sensors (TIRS). Landsat 8 builds upon the impressive 40-year legacy of the Landsat program, offering advanced features such as new spectral bands for blue and cirrus cloud detection, two thermal bands, enhanced sensor signal-to-noise ratio, improved radiometric resolution, and an optimized duty cycle that enables the collection of a substantially higher number of images each day (Loveland and Irons, 2016; Lymburner et al., 2016; Roy et al., 2014). Notably, Band 9, designed to assess reflectance from cirrus clouds, is included. Band 9 displays the smallest range, but it’s one of the most intriguing aspects of Landsat 8. It spans a very narrow wavelength band: just 1370 ± 10 nm. It provides information about cloud presence or absence (Shen et al., 2015).
However, there is a gap in the literature regarding the assessment of Landsat 8’s applicability in terms of the number of available images and the percentage of cloud cover for each scene across all paths and rows globally. To date, several studies have focused on using Landsat data for time series analysis, specifically examining the impact of clouds on its effectiveness at smaller scales. For instance, Holden Holden and Woodcock (2016) investigated Landsat 7 and Landsat 8 data in their analysis of time series, assessing differences in surface reflectance and cirrus cloud characteristics to determine how substitutable Landsat 8 observations are within the long-term archive. Their results revealed that although Landsat 8 provides improved radiometric resolution, better cloud detection, and enhanced geometric accuracy, any discrepancies in reflectance between the two sensors in the current Landsat Climate Data Record (CDR) need to be addressed or clarified in time series analyses to avoid adverse effects.
Xu et al. (2022) also, examined and compared the performance of three satellite sensors—PROBA-V, Landsat 8 OLI, and Sentinel-2 MSI—in tracking land cover change (LCC). Their analysis utilized a unique dataset that included 4 years of global reference data spanning from 2015 to 2018. The results revealed that, for general global LCC monitoring, Landsat 8 OLI slightly outperformed Sentinel-2, while PROBA-V was found to be the least effective. The performance differences among the sensors were consistent and were influenced by variations in data availability and the spectral regions observed. Specifically, Landsat 8 demonstrated greater accuracy in monitoring forested areas, herbaceous vegetation, and water bodies, whereas Sentinel-2 was particularly effective in monitoring wetlands.
This study aims to address a critical gap in understanding the availability and cloud cover of the Landsat 8 dataset from 2014 to 2023 on a global scale, specifically for each path and row of the satellite’s imagery. To achieve this, we systematically downloaded two key pieces of information for every Landsat path and row each year: 1) the total number of available images in the Landsat 8 Collection 2, and 2) the percentage of cloud cover associated with each image, as indicated in the image metadata. By compiling this data, we generated 20 shapefiles for each year, which illustrate both the number of available images and their corresponding cloud cover percentages for the various Landsat paths and rows. These shapefiles serve as valuable resources for researchers, enabling them to efficiently access essential information regarding any location on Earth before initiating their studies.
This study used the “LANDSAT/LC08/C02/T1_L2” dataset available in Google Earth Engine (GEE), which provides atmospherically corrected Landsat 8 Level 2, Collection 2, Tier 1 surface reflectance and surface temperature data. This collection uses data captured by the Operational Land Imager (OLI) and the Thermal Infrared Sensor (TIRS) on board the Landsat 8 satellite. The atmospheric corrections applied during Level 2 processing remove effects such as haze and atmospheric scattering from the Top of Atmosphere (TOA) reflectance, while the surface temperature retrieval algorithm provides temperature values from thermal bands. This preprocessing allows the dataset to closely represent actual surface conditions, making it ideal for applications like land cover classification, monitoring landscape changes over time, and conducting time series analyses (Crawford et al., 2023).
Belonging to the USGS Landsat Collection 2, the dataset has undergone significant enhancements, including better geolocation accuracy and refined radiometric calibration, ensuring high consistency and reliability across all images. This dataset spans the entire globe and has captured images regularly since the launch of Landsat 8 in 2013, offering researchers a robust foundation for examining environmental, agricultural, and urban landscapes across various timeframes and spatial scales. Given its Tier 1 classification, it meets stringent standards for calibration and georeferencing, making it the highest-quality option within the Landsat Collection 2, and thus suitable for detailed environmental assessments and other applications where precision is critical. However, users should note a known limitation in the surface temperature data, where potential inaccuracies related to cloud cover and cloud shadows can affect the thermal bands. These issues, documented by Crawford et al. (2023), are worth considering when conducting analyses involving thermal measurements, especially in regions prone to frequent cloud cover. The quality and accuracy of this dataset enable its effective use in complex analyses, providing insights into diverse surface conditions worldwide.
To work with Google Earth Engine (GEE) within Python, we use libraries like ‘geemap’ or the Earth Engine Python API (‘ee’), which facilitate a seamless connection between Python and GEE’s extensive geospatial datasets (Viani et al., 2024). The process begins by installing the Earth Engine API and authenticating access, setting up a secure link to GEE’s resources. Once connected, we initialize the library in Python, which grants access to GEE’s vast archives, including datasets like Landsat, Sentinel, and MODIS. This integration allows us to interact with these datasets directly in Python, enabling powerful geospatial analyses across large temporal and spatial scales without the need to store data locally.
To assess the availability of Landsat 8 imagery for specific geographic coordinates (path and row) from 2014 to 2023, we leveraged Google Earth Engine (GEE) and Python. First, we accessed the entire Landsat 8 collection using the ‘ee.ImageCollection’ function, a method that allows us to filter images based on multiple criteria, including date range and location. By specifying our study period (2014–2023) and defining the area of interest through path and row coordinates, we isolated all relevant images in the Landsat 8 dataset. After filtering the collection to include only images within our defined spatial and temporal boundaries, we employed the `.size ()` function to count the number of images that met these criteria. This function provided a straightforward way to quantify image availability for each path and row, offering insights into data consistency and density over the specified time frame.
Each image in the Landsat 8 collection includes a set of metadata properties, such as the percentage of cloud cover over the area, stored in the ‘CLOUD_COVER’ attribute. This information is crucial for evaluating image quality, as higher cloud cover can limit the usefulness of the imagery for analysis. We extracted the cloud cover metadata for each filtered image, providing a way to assess image quality over time. In addition to cloud cover, each image’s acquisition time (or timestamp) was recorded. This allowed us to create a timeline of images, capturing seasonal and annual variations in coverage that could inform our study. Finally, to facilitate further analysis, we organized the results into a structured dataset that included the total image count, cloud cover percentages, and acquisition dates. This dataset was exported as a CSV file, enabling easy access, sharing, and use for subsequent analyses or project documentation. By structuring the data this way, we ensured it was ready for in-depth analysis, such as temporal studies of cloud cover patterns or evaluating the frequency and distribution of clear-sky images across the study area.
To analyze global Landsat 8 data availability, we first compiled relevant metadata for each Landsat 8 image from [USGS Landsat WRS-2 Scene Boundaries] (https://www.usgs.gov/media/files/landsat-wrs-2-scene-boundaries-kml-file). This included gathering information on cloud cover and acquisition dates, which we accessed through the USGS Landsat WRS-2 Scene Boundaries, available in a downloadable KML file containing path and row identifiers for each scene globally with a reference system of WGS1984. Using these identifiers, we calculated the total number of available images in each path and row on an annual basis from 2014 to 2023. Additionally, we computed the average cloud cover percentage per year for each path and row, providing insights into image quality and availability across different regions and times.
We then integrated this calculated data with a shapefile representing global Landsat path and row boundaries, aligning the metadata for each path and row with its corresponding geographic boundaries. From this enriched dataset, we created two types of visualizations: (1) a global map displaying the number of Landsat 8 images available per path and row and (2) a second map illustrating the average cloud cover percentage for each path and row globally. These maps highlight regions with the most extensive data coverage and areas where high cloud cover might limit data usability. Finally, to explore trends related to latitude, we plotted both the number of available images and average cloud cover percentages across various latitudes, helping us understand how global image availability and cloud cover vary geographically. This analysis allows for identifying optimal data sources by region, improving our approach for selecting cloud-free Landsat imagery for various applications.
Figure 1 illustrates the number of Landsat 8 images per path and row for the year 2023 across different world regions: (a) Africa, Europe, and Asia, and (b) North America. The figure indicates that up to 23 Landsat images can be expected annually for each path and row, given that Landsat has a 16-day revisit period. The map legend shows that some paths and rows indeed have up to 23 images. This distribution highlights that certain regions, including Australia, parts of Africa, the Middle East, Western Asia, and Southern North America, receive a high frequency of Landsat imagery. Conversely, Northern Asia and Northern North America experience fewer Landsat images, indicating lower image availability in these areas.
Figure 1. Number of Landsat 8 images per path and row in (A) Africa, Europe, and Asia, and (B) North America in the year 2023. The map legend indicates that certain paths and rows contain as many as 23 available images (blue). For the paths and rows highlighted in yellow, we observe that they have the minimum number of images available.
Figure 2 displays the cloud cover percentage of Landsat 8 images per path and row for the year 2023, with separate panels for (a) Africa, Europe, and Asia, and (b) North America. The cloud cover percentage is categorized into 10 distinct classes. The figure reveals that certain regions, such as central Australia, northern Africa, and the Middle East, experience lower cloud coverage. Additionally, a few paths and rows in southern South America also show relatively low cloud cover. Conversely, areas with higher cloud coverage are evident in regions like central Africa, as well as countries such as Thailand and Indonesia. Northern parts of Asia, Europe, and North America also exhibit increased cloud coverage.
Figure 2. Cloud cover percentage of Landsat 8 images per path and row in (A) Africa, Europe, and Asia, and (B) North America in the year 2023. The map legend indicates that certain paths and rows, marked in red, have cloud cover percentages ranging from 0% to 9% in the images. The paths and rows highlighted in blue exhibit the highest cloud cover percentages, falling between 60% and 90%.
Figure 3 provides an insightful look into the global distribution of Landsat 8 imagery and cloud cover percentages relative to latitude for the year 2023. Figure 3A shows a radar plot displaying the number of Landsat 8 images available at various latitudes. The plot ranges from −55–82° latitude, with the circle’s circumference representing these latitudinal values and the radial distance from the center indicating the frequency of imagery. The data reveal that areas within latitudes of 55–82° receive a notably lower number of Landsat images compared to other latitudinal zones.
Figure 3. Global distribution of (A) Landsat 8 images and (B) Cloud cover percentage against latitude in the year 2023.
Figure 3B presents the average cloud cover percentage of Landsat images as a function of latitude. This plot demonstrates several distinct patterns in cloud coverage across different latitudinal zones. Between latitudes −60 and −20, there is a marked decrease in cloud cover percentage, dropping from approximately 90% to less than 10%. This significant reduction indicates that Southern Hemisphere mid-latitudes generally experience clearer skies compared to more equatorial regions. Conversely, from −20 to 0° latitude, the average cloud cover percentage increases, suggesting that as one approaches the Equator, cloudiness becomes more prevalent, likely due to higher moisture levels and conducive atmospheric conditions for cloud formation.
Further, from latitudes 0–20°, there is a decrease in cloud cover percentage, reflecting a reduction in cloudiness as one moves away from the Equator towards subtropical regions. This pattern might be influenced by seasonal and climatic factors that affect cloud formation. Finally, between latitudes 20 and 60°, there is a rise in cloud cover percentage, indicating that as one moves towards higher latitudes in the Northern Hemisphere, cloudiness increases. This trend could be related to the influence of mid-latitude weather systems and changing climatic conditions.
Table 1 provides an in-depth analysis of Landsat 8 imagery from 2014 to 2023, presenting three key metrics for each continent: the mean number of images per path and row, the total number of images, and the average cloud cover percentage. Overall, Table 1 illustrates significant regional variations in the availability and quality of Landsat 8 imagery. The mean number of images and the total count reflect the extent and frequency of satellite observations, while the average cloud cover percentages provide insights into the imaging conditions encountered across different continents.
Table 1. Mean number of Landsat 8 images per path and row, total number of images, and average cloud cover percentage from 2014 to 2023 for each continent.
In terms of the mean number of Landsat 8 images per path and row, Asia exhibits the highest value at 4372 images. This substantial number underscores Asia’s extensive monitoring coverage and high frequency of satellite passes, reflecting the continent’s large size and diverse environmental conditions. In stark contrast, Australia shows the lowest mean of 401 images per path and row, consistent with its smaller land area and fewer paths covered by the satellite.
The total number of images further highlights these differences. Asia leads with a total of 43,723 images, which is indicative of its vast geographic coverage and high frequency of observations. This total mirrors Asia’s high mean count per path and row. North America follows with 25,574 images, showing significant data availability but still less than Asia. South America has a total of 9,924 images, reflecting a moderate level of imagery data. Europe accumulates 11,094 images, while Africa has 19,670 images, indicating substantial but variable imagery coverage across different regions.
When examining average cloud cover percentages, Europe stands out with the highest average at 42.5%. This suggests that a significant portion of Landsat 8 images in Europe is affected by cloud cover, which may impact the clarity of the data. Conversely, Africa has the lowest average cloud cover percentage at 23.3%, indicating relatively clearer conditions during satellite passes, which could result in higher-quality imagery. South America follows with an average cloud cover of 39.8%, while Asia and North America report averages of 37.2% and 38.2%, respectively. Australia has a lower average cloud cover percentage of 20.4%, reflecting relatively favorable imaging conditions.
This study successfully evaluated the applicability of Landsat 8 Collection Level 2 for time series analysis and identified two major concerns: first, the number of available images, and second, the percentage of cloud cover for each image per path and row over a decade. First, we discuss the availability of Landsat 8 images per path and row per year, which can reach a maximum of 23 images per path and row. However, due to spatial overlap between Landsat paths and rows, users can access more images if their study area falls within these overlapping regions. Our analysis of Landsat 8 image availability revealed varying patterns across different regions of the world, including (a) Africa, Europe, and Asia, and (b) North America. This distribution highlights that certain regions, such as Australia, parts of Africa, the Middle East, Western Asia, and Southern North America, receive a higher frequency of Landsat imagery.
In contrast, Northern Asia and Northern North America experience fewer Landsat images, indicating lower image availability in these areas. Additionally, when examining the number of Landsat 8 images available across various latitudes, we observe that regions within latitudes 55–82° receive significantly fewer images compared to other latitudinal zones. Consequently, in certain regions of the world, there may be insufficient Landsat 8 images for time series analysis, even if all available images are cloud-free and atmospherically corrected. Asia has the highest mean number of Landsat 8 images per path and row, with 4,372 images, highlighting its extensive monitoring and frequent satellite passes due to its large size and diverse environments. In contrast, Australia has the lowest mean of 401 images, aligning with its smaller land area and fewer satellite paths. The total number of images reinforces these differences: Asia leads with 43,723 images, reflecting its vast coverage and high observation frequency, followed by North America with 25,574 images. South America has 9,924 images, Europe 11,094 images, and Africa 19,670 images, indicating varying levels of data coverage across these regions.
The analysis of Landsat 8 image availability across different regions reveals important challenges and opportunities for remote sensing applications. While some regions benefit from a high frequency of satellite imagery due to spatial overlap in the paths and rows, others face significant limitations. This uneven distribution of images underscores the difficulty in applying uniform remote sensing techniques globally, as regions with lower image availability might lack the necessary data density for robust analysis. Additionally, the differences in the total number of images between continents, with Asia receiving the highest number and Australia the least, highlight the varying levels of monitoring intensity. This disparity may lead to gaps in environmental monitoring and resource management, particularly in regions with fewer images, thereby affecting the accuracy and reliability of remote sensing studies in those areas. Overall, these findings emphasize the need for tailored approaches in remote sensing that account for regional differences in data availability and highlight the importance of optimizing satellite data usage in underrepresented regions.
Upon examining cloud cover percentages across different regions, distinct patterns emerge. In regions such as central Australia, northern Africa, and the Middle East, cloud cover tends to be lower, resulting in clearer skies. Some areas in southern South America also show relatively minimal cloudiness. Conversely, higher cloud cover is noted in central Africa, as well as in countries like Thailand and Indonesia. Northern regions of Asia, Europe, and North America also experience increased cloudiness. Our analysis also reveals clear latitudinal trends in cloud cover. Between latitudes −60 and −20°, there is a significant drop in cloud cover from approximately 90% to below 10%, indicating that mid-latitudes in the Southern Hemisphere generally have clearer skies compared to equatorial zones. As latitude shifts from −20 to 0°, cloud cover rises, reflecting increased cloudiness near the Equator due to higher humidity and conducive conditions for cloud formation. Moving from 0 to 20° latitude, cloud cover decreases, suggesting clearer conditions in subtropical regions. Finally, between latitudes 20 and 60°, cloud cover increases again, which may be associated with mid-latitude weather systems and varying climatic conditions.
Regarding average cloud cover percentages, Europe has the highest average at 42.5%, indicating frequent cloud cover that may affect image clarity. Conversely, Africa has the lowest average at 23.3%, suggesting clearer satellite imagery. South America has a moderate average of 39.8% cloud cover, while Asia and North America report averages of 37.2% and 38.2%, respectively. Australia, with the lowest average cloud cover of 20.4%, offers the best conditions for clear imaging, reducing the likelihood of cloud obstruction in satellite observations. The examination of cloud cover percentages across various regions and latitudinal zones reveals significant challenges for remote sensing using Landsat 8 imagery. Cloud cover, a critical factor affecting the quality of satellite data, varies considerably by region and latitude, presenting both opportunities and limitations for effective monitoring and analysis. Regions with lower cloud cover, offer clearer imaging conditions. This reduced cloudiness is beneficial for detailed observation and analysis, facilitating more accurate environmental monitoring and change detection. However, even within these regions, variability exists, and certain areas may still experience intermittent cloud cover that could affect image quality. In contrast, regions with higher cloud cover, face more challenges. Persistent cloudiness in these areas can obscure satellite observations, leading to gaps in data and potential inaccuracies in analysis. This can be particularly problematic for studies requiring continuous time series data, as frequent cloud cover may hinder the acquisition of useable images.
Latitudinal trends further complicate the situation. The notable decrease in cloud cover from latitudes −60 to −20 indicates clearer skies in mid-latitude regions of the Southern Hemisphere, which can be advantageous for satellite observations. Conversely, the increase in cloud cover near the Equator (from −20 to 0° latitude) suggests that tropical and subtropical regions experience more frequent cloudiness due to higher humidity and conducive atmospheric conditions. This trend may limit the effectiveness of remote sensing in these regions, requiring careful planning and potentially additional data processing to account for cloud interference. The reduction in cloud cover from 0 to 20° latitude, followed by an increase between 20 and 60° latitude in the Northern Hemisphere, highlights the complex interplay of seasonal and climatic factors influencing cloudiness. Mid-latitude weather systems and varying climatic conditions contribute to increased cloud cover in these higher latitudes, affecting the availability and quality of satellite imagery. Overall, the variability in cloud cover across different regions and latitudes underscores the need for strategic planning in remote sensing projects.
Researchers must consider cloud cover when selecting study areas and interpreting satellite imagery, as excessive cloudiness can significantly degrade image quality and usability. To tackle these challenges, advancements in cloud detection, image processing, and the integration of supplementary data sources are essential for enhancing satellite-based monitoring and analysis. One notable development is the United States Geological Survey’s (USGS) Landsat Analysis Ready Data (ARD), which aims to facilitate time series analysis. Qiu et al. (2018) assessed the temporal consistency of this dataset and proposed several processing improvements, focusing on data resampling, cloud and shadow detection, Bidirectional Reflectance Distribution Function (BRDF) correction, and topographic correction. Their findings indicated that the updated cloud and shadow detection method (e.g., Fmask 4.0 versus version 3.3) moderately improved data consistency.
Additionally, Zhu and Helmer (2018) introduced Automatic Time-Series Analysis (ATSA), a method for detecting clouds and cloud shadows in multi-temporal optical imagery. The ATSA process involves five key steps: (1) calculating cloud and shadow indices for enhanced detection; (2) generating an initial cloud mask through unsupervised classification; (3) refining the mask by analyzing the time series of the cloud index; (4) estimating a potential shadow mask based on geometric relationships; and (5) further refining the shadow mask using the time series of the shadow index. This method was validated with Landsat-8 OLI, Landsat-4 MSS, and Sentinel-2 imagery across three sites, comparing results to the established Function of Mask (Fmask) technique used by the USGS.
Qiu et al. (2020) also developed a Cmask (Cirrus Cloud Mask) to detect cirrus clouds in Landsat 8 imagery using time series data from the Cirrus Band (1.36–1.39 μm). This algorithm identifies pixels influenced by cirrus clouds by comparing model predictions to actual Top-Of-Atmosphere (TOA) reflectance data. The study aimed to clarify the definition of cirrus clouds within Landsat observations, establishing criteria for classifying pixels affected by cirrus clouds, which can significantly impact reflectance in other spectral bands. The Cmask algorithm demonstrated a significant reduction in errors, achieving an 8% error rate in distinguishing cirrus clouds from clear observations, compared to 15% with prior methods. Cao et al. (2020) introduced the AutoRegression to Remove Clouds (ARRC) method, which innovatively utilizes the autocorrelation present in Landsat time-series data. This technique employs multi-year Landsat images, including partially cloud-contaminated ones, during the cloud-removal process. ARRC effectively addresses situations where substantial land cover changes over several years could negatively affect the autocorrelation in Landsat time series data.
This study provides a comprehensive evaluation of the Landsat 8 Collection Level 2 data for time series analysis, revealing key insights into image availability and cloud cover issues. Our findings highlight two primary concerns that affect the utility of Landsat 8 imagery: the number of available images and the percentage of cloud cover. We found an uneven distribution in the number of available images which poses challenges for comprehensive global monitoring, especially in regions with lower image availability, potentially leading to gaps in environmental data and analysis. Our analysis shows that certain regions, experience relatively high cloud cover, complicating having clearer satellite observations. Latitudinal patterns further complicate this issue, with notable variations in cloud cover from the Southern Hemisphere to the Northern Hemisphere. This study underscores the importance of considering both image availability and cloud cover in remote sensing applications. Tailoring remote sensing approaches to account for regional differences and optimizing the use of satellite data is crucial for improving the accuracy and reliability of environmental monitoring and analysis. Improved cloud detection methods and integration with other data sources can help mitigate the impact of cloud cover and enhance the effectiveness of Landsat 8 imagery for time series studies.
The datasets presented in this study can be found in online repositories. The names of the repository/repositories and accession number(s) can be found in the article/supplementary material.
ER: Conceptualization, Data curation, Methodology, Software, Writing–original draft. CJ: Conceptualization, Formal Analysis, Funding acquisition, Project administration, Resources, Validation, Visualization, Writing–review and editing.
The author(s) declare that financial support was received for the research, authorship, and/or publication of this article. This work was supported by the Basic Science Research Program through the National Research Foundation of Korea (NRF) funded by the Ministry of Education (grant no.: NRF-2018R1A6A1A03024862), and Rural Development Administration, Agenda project on climate change monitoring RS-2024-00397542.
The authors declare that the research was conducted in the absence of any commercial or financial relationships that could be construed as a potential conflict of interest.
All claims expressed in this article are solely those of the authors and do not necessarily represent those of their affiliated organizations, or those of the publisher, the editors and the reviewers. Any product that may be evaluated in this article, or claim that may be made by its manufacturer, is not guaranteed or endorsed by the publisher.
Cao, R., Chen, Y., Chen, J., Zhu, X., and Shen, M. (2020). Thick cloud removal in Landsat images based on autoregression of Landsat time-series data. Remote Sens. Environ. 249, 112001. doi:10.1016/j.rse.2020.112001
Crawford, C. J., Roy, D. P., Arab, S., Barnes, C., Vermote, E., Hulley, G., et al. (2023). The 50-year Landsat collection 2 archive. Sci. Remote Sens. 8, 100103. doi:10.1016/j.srs.2023.100103
Forkel, M., Carvalhais, N., Verbesselt, J., Mahecha, M. D., Neigh, C. S., and Reichstein, M. (2013). Trend change detection in NDVI time series: effects of inter-annual variability and methodology. Remote Sens. 5 (5), 2113–2144. doi:10.3390/rs5052113
Guyet, T., and Nicolas, H. (2016). Long term analysis of time series of satellite images. Pattern Recognit. Lett. 70, 17–23. doi:10.1016/j.patrec.2015.11.005
Holden, C. E., and Woodcock, C. E. (2016). An analysis of Landsat 7 and Landsat 8 underflight data and the implications for time series investigations. Remote Sens. Environ. 185, 16–36. doi:10.1016/j.rse.2016.02.052
Hu, C., Huo, L.-Z., Zhang, Z., and Tang, P. (2020). Multi-temporal landsat data automatic cloud removal using Poisson blending. IEEE Access 8, 46151–46161. doi:10.1109/access.2020.2979291
Hu, Q., Sulla-Menashe, D., Xu, B., Yin, H., Tang, H., Yang, P., et al. (2019). A phenology-based spectral and temporal feature selection method for crop mapping from satellite time series. Int. J. Appl. Earth Observation Geoinformation 80, 218–229. doi:10.1016/j.jag.2019.04.014
Huang, S., Tang, L., Hupy, J. P., Wang, Y., and Shao, G. (2021). A commentary review on the use of normalized difference vegetation index (NDVI) in the era of popular remote sensing. J. For. Res. 32 (1), 1–6. doi:10.1007/s11676-020-01155-1
Jain, M., Mondal, P., DeFries, R. S., Small, C., and Galford, G. L. (2013). Mapping cropping intensity of smallholder farms: a comparison of methods using multiple sensors. Remote Sens. Environ. 134, 210–223. doi:10.1016/j.rse.2013.02.029
King, M. D., Platnick, S., Menzel, W. P., Ackerman, S. A., and Hubanks, P. A. (2013). Spatial and temporal distribution of clouds observed by MODIS onboard the Terra and Aqua satellites. IEEE Trans. geoscience remote Sens. 51 (7), 3826–3852. doi:10.1109/tgrs.2012.2227333
Lasaponara, R., and Lanorte, A. (2012). Satellite time-series analysis. England & Wales: Taylor & Francis, 4649–4652.
Li, Z., Shen, H., Cheng, Q., Liu, Y., You, S., and He, Z. (2019). Deep learning based cloud detection for medium and high resolution remote sensing images of different sensors. ISPRS J. Photogrammetry Remote Sens. 150, 197–212. doi:10.1016/j.isprsjprs.2019.02.017
Liu, J., Zhu, W., Atzberger, C., Zhao, A., Pan, Y., and Huang, X. (2018). A phenology-based method to map cropping patterns under a wheat-maize rotation using remotely sensed time-series data. Remote Sens. 10 (8), 1203. doi:10.3390/rs10081203
Loveland, T. R., and Irons, J. R. (2016). Landsat 8: the plans, the reality, and the legacy. Remote Sens. Environ. 185, 1–6. doi:10.1016/j.rse.2016.07.033
Lymburner, L., Botha, E., Hestir, E., Anstee, J., Sagar, S., Dekker, A., et al. (2016). Landsat 8: providing continuity and increased precision for measuring multi-decadal time series of total suspended matter. Remote Sens. Environ. 185, 108–118. doi:10.1016/j.rse.2016.04.011
Orusa, T., Cammareri, D., Freppaz, D., Vuillermoz, P., and Borgogno Mondino, E. (2023a). “Sen4MUN: a prototypal service for the distribution of contributions to the European municipalities from copernicus satellite imagery. A case in aosta valley (NW Italy),” in Italian conference on geomatics and geospatial technologies (Springer), 109–125.
Orusa, T., Viani, A., and Borgogno-Mondino, E. (2024a). Earth observation data and geospatial deep learning AI to assign contributions to European municipalities Sen4MUN: an empirical application in Aosta Valley (NW Italy). Land 13 (1), 80. doi:10.3390/land13010080
Orusa, T., Viani, A., and Borgogno-Mondino, E. (2024b). IRIDE, the euro-Italian earth observation program: overview, current progress, global expectations, and recommendations. Environ. Sci. Proc. 29 (1), 74. doi:10.3390/ECRS2023-16839
Orusa, T., Viani, A., Moyo, B., Cammareri, D., and Borgogno-Mondino, E. (2023b). Risk assessment of rising temperatures using landsat 4–9 LST time series and Meta® population dataset: an application in aosta valley, NW Italy. Remote Sens. 15 (9), 2348. doi:10.3390/rs15092348
Petitjean, F., Kurtz, C., Passat, N., and Gançarski, P. (2012). Spatio-temporal reasoning for the classification of satellite image time series. Pattern Recognit. Lett. 33 (13), 1805–1815. doi:10.1016/j.patrec.2012.06.009
Qiu, B., Li, W., Tang, Z., Chen, C., and Qi, W. (2015). Mapping paddy rice areas based on vegetation phenology and surface moisture conditions. Ecol. Indic. 56, 79–86. doi:10.1016/j.ecolind.2015.03.039
Qiu, S., Lin, Y., Shang, R., Zhang, J., Ma, L., and Zhu, Z. (2018). Making Landsat time series consistent: evaluating and improving Landsat analysis ready data. Remote Sens. 11 (1), 51. doi:10.3390/rs11010051
Qiu, S., Zhu, Z., and Woodcock, C. E. (2020). Cirrus clouds that adversely affect Landsat 8 images: what are they and how to detect them? Remote Sens. Environ. 246, 111884. doi:10.1016/j.rse.2020.111884
Rahimi, E. (2024). The efficiency of long short-term memory (LSTM) in phenology-based crop classification. Journal of the Korean Society of Remote Sensing 40 (1), 57–69. doi:10.7780/kjrs.2024.40.1.6
Rahimi, E., Barghjelveh, S., and Dong, P. (2021). Quantifying how urban landscape heterogeneity affects land surface temperature at multiple scales. J. Ecol. Environ. 45 (1), 22–13. doi:10.1186/s41610-021-00203-z
Rahimi, E., Barghjelveh, S., and Dong, P. (2022). A comparison of discrete and continuous metrics for measuring landscape changes. J. Indian Soc. Remote Sens. 50 (7), 1257–1273. doi:10.1007/s12524-022-01526-7
Rahimi, E., and Jung, C. (2024). Comparative analysis of supervised and phenology-based approaches for crop mapping: a case study in South Korea. Journal of the Korean Society of Remote Sensing 40 (2), 179–190. doi:10.7780/kjrs.2024.40.2.5
Roy, D. P., Wulder, M. A., Loveland, T. R., Woodcock, C. E., Allen, R. G., Anderson, M. C., et al. (2014). Landsat-8: Science and product vision for terrestrial global change research. Remote Sens. Environ. 145, 154–172. doi:10.1016/j.rse.2014.02.001
Shen, Y., Wang, Y., Lv, H., and Li, H. (2015). Removal of thin clouds using cirrus and QA bands of Landsat-8. Photogrammetric Eng. & Remote Sens. 81 (9), 721–731. doi:10.14358/pers.81.9.721
Simoes, R., Camara, G., Queiroz, G., Souza, F., Andrade, P. R., Santos, L., et al. (2021). Satellite image time series analysis for big earth observation data. Remote Sens. 13 (13), 2428. doi:10.3390/rs13132428
Tian, H., Huang, N., Niu, Z., Qin, Y., Pei, J., and Wang, J. (2019). Mapping winter crops in China with multi-source satellite imagery and phenology-based algorithm. Remote Sens. 11 (7), 820. doi:10.3390/rs11070820
Tolnai, M., Nagy, J. G., and Bakó, G. (2016). Spatiotemporal distribution of Landsat imagery of Europe using cloud cover-weighted metadata. J. Maps 12 (5), 1084–1088. doi:10.1080/17445647.2015.1125308
Verbesselt, J., Hyndman, R., Newnham, G., and Culvenor, D. (2010). Detecting trend and seasonal changes in satellite image time series. Remote Sens. Environ. 114 (1), 106–115. doi:10.1016/j.rse.2009.08.014
Viani, A., Orusa, T., Borgogno-Mondino, E., and Orusa, R. (2024). A one health google earth engine web-GIS application to evaluate and monitor water quality worldwide. Euro-Mediterranean J. Environ. Integration, 1–14. doi:10.1007/s41207-024-00528-w
Waldner, F., Canto, G. S., and Defourny, P. (2015). Automated annual cropland mapping using knowledge-based temporal features. ISPRS J. Photogrammetry Remote Sens. 110, 1–13. doi:10.1016/j.isprsjprs.2015.09.013
Xu, L., Herold, M., Tsendbazar, N.-E., Masiliūnas, D., Li, L., Lesiv, M., et al. (2022). Time series analysis for global land cover change monitoring: a comparison across sensors. Remote Sens. Environ. 271, 112905. doi:10.1016/j.rse.2022.112905
Zhang, Y., Woodcock, C. E., Arévalo, P., Olofsson, P., Tang, X., Stanimirova, R., et al. (2022). A global analysis of the spatial and temporal variability of useable Landsat observations at the pixel scale. Front. Remote Sens. 3, 894618. doi:10.3389/frsen.2022.894618
Zhao, F., Xia, L., Kylling, A., Shang, H., and Yang, P. (2022). Mapping global flying aircraft activities using Landsat 8 and cloud computing. ISPRS J. Photogrammetry Remote Sens. 184, 19–30. doi:10.1016/j.isprsjprs.2021.12.003
Keywords: cloud, phenological studies, latitude, optical, path and row, google earth engine
Citation: Rahimi E and Jung C (2024) Evaluating the applicability of landsat 8 data for global time series analysis. Front. Remote Sens. 5:1492534. doi: 10.3389/frsen.2024.1492534
Received: 07 September 2024; Accepted: 28 October 2024;
Published: 08 November 2024.
Edited by:
Tommaso Orusa, University of Turin, ItalyReviewed by:
Riccardo Orusa, Liguria and Valle d’Aosta (IZSTO), ItalyCopyright © 2024 Rahimi and Jung. This is an open-access article distributed under the terms of the Creative Commons Attribution License (CC BY). The use, distribution or reproduction in other forums is permitted, provided the original author(s) and the copyright owner(s) are credited and that the original publication in this journal is cited, in accordance with accepted academic practice. No use, distribution or reproduction is permitted which does not comply with these terms.
*Correspondence: Chuleui Jung, Y2p1bmdAYW5kb25nLmFjLmty
Disclaimer: All claims expressed in this article are solely those of the authors and do not necessarily represent those of their affiliated organizations, or those of the publisher, the editors and the reviewers. Any product that may be evaluated in this article or claim that may be made by its manufacturer is not guaranteed or endorsed by the publisher.
Research integrity at Frontiers
Learn more about the work of our research integrity team to safeguard the quality of each article we publish.