- 1Jet Propulsion Laboratory, California Institute of Technology, Pasadena, CA, United States
- 2Ctrees, Pasadena, CA, United States
- 3Center for Tropical Research, Institute of the Environment and Sustainability, University of California Los Angeles, Los Angeles, CA, United States
Forests of California are undergoing large-scale disturbances from wildfire and tree mortality, caused by frequent droughts, insect infestations, and human activities. Mapping and monitoring the structure of these forests at high spatial resolution provides the necessary data to better manage forest health, mitigate wildfire risks, and improve carbon sequestration. Here, we use LiDAR measurements of top of canopy height metric (RH98) from NASA’s Global Ecosystem Dynamics Investigation (GEDI) mission to map vegetation height across the entire California for two different time periods (2019–2020 and 2021–2022) and explore the impact of disturbance. Exploring the reliability of machine learning methods for temporal monitoring of forest is still a developing field. We train a deep neural network to predict forest height metrics at 10-m resolution from radar and optical satellite imagery. Model validation against independent airborne LiDAR data showed a
1 Introduction
The contribution of forestland to emissions offset in the United States has remained relatively stable since 2005 despite steady declines in economy-wide CO2 emissions over that period (Domke et al., 2020). This suggests that the forest carbon sink in the United States, which is driven in large part by forest regrowth following harvest and natural disturbances, is slowly diminishing (Williams et al., 2016; Pugh et al., 2019; Quirion et al., 2021). This threat to the US forest sink is especially significant in California, and the Pacific Northwest where frequent droughts have caused increasingly intense wildfires and insect infestation, reducing the forest carbon stocks and productivity in the last two decades (Yu et al., 2022; Coffield et al., 2022). A history of fire suppression due to forest management has increased fuel stocks and altered fire regimes, causing catastrophic fires under cascading effects of climate change (Keane, 2002). The changes of forest carbon and health have devastating implications for California’s emission reduction strategy and threatens its future economy by directly impacting landowners, corporations, and communities living near and in forests (Badgley et al., 2022).
One of the challenges associated with understanding the threat to California forests and its inhabitants is the lack of detailed, accurate, and frequent information on changes of forest height (and the associated fuel load, and the carbon density) at the state level. Surveys of forests across California over the last century (1930s–2000s) show a significant demographic shift in forest height accompanied by a shift in composition toward increased dominance by oaks relative to pines (McIntyre et al., 2015; Hill and Field, 2021). In recent years (1990s), these surveys are performed every 10 years in a network of forest inventory and analysis (FIA) plots. However, the small number of FIA plots (about 5,300 1-acre plots) sampling more than 32 million acres of forests in California and collected over a decade, is not enough to capture widespread tree mortality and frequent disturbance that alters forest height and biomass rapidly (Yu et al., 2022). Therefore, there is an urgent need to map and frequently monitor spatial variations of forest height at the scale of disturbance and management activities across California. Widespread mapping of vegetation structure and above ground biomass at local, regional, and global scales have become possible by using airborne and space borne LiDAR (Light Detection and Ranging) sensors from the beginning of this century (Dubayah and Drake, 2000; Næsset, 2002; Lim and Treitz, 2004; Lefsky, 2010; Saatchi et al., 2011; Bruening et al., 2023). Modern LiDAR sensors measure the timing and intensity of reflected energy from forest attributes, providing relatively continuous waveforms related to the vertical distribution of plant material (branches and leaves) and accurate information on forest height and terrain elevation (Eitel et al., 2016; Dong and Chen, 2017; Kellner et al., 2019). In recent years, the applications of LiDAR have broadened in forest ecology by quantifying 3D structure, demography, and gap dynamics (Vepakomma et al., 2008; Kellner and Asner, 2009; Ferraz et al., 2016; Dalagnol et al., 2021; Jucker, 2022), carbon stocks and dynamics (Asner and Mascaro, 2014; Stark et al., 2012; Moura et al., 2020; Meyer et al., 2013), and forest disturbances (Wulder et al., 2008; García et al., 2017; Jeronimo et al., 2018).
In California, LiDAR measurements have been used to quantify structure and fuels over some of the tallest, and most complex landscapes in the world (Kelly et al., 2017; Tang et al., 2014; Su et al., 2017). Estimation of forest fuels, the organic matter available for fire ignition and combustion, are considered the most essential attributes of California forests and the key component of fire management activities (Keane et al., 2004). The fuel loads are directly linked to measurements of forest height and biomass and can be estimated from ground over small areas and from remote sensing measurements across larger landscapes (Anderson et al., 2005; Saatchi et al., 2007; Skowronski et al., 2011; Kramer et al., 2016; Garcia et al., 2017).
Although widespread airborne LiDAR data are now available over California, the measurements are acquired over multiple years with different sensor characteristics, limiting their use for the monitoring of forest height across the state. Optical and radar remote sensing observations from space may provide a reliable alternative for mapping and monitoring forest height over large areas. They can be combined with NASA’s Global Ecosystem Dynamics Investigation (GEDI) that collected LiDAR samples globally between 2019 and 2023. It provides billions of waveform observations (
Here, we want to evaluate the capacity of machine learning based model to monitor forest height changes in California. We develop a model to map forest height over the entire California, reducing the uncertainty in spatial variations compared to global maps, and improving estimation of tall forests
The first sections of this paper describe the different datasets used, and the methodologies developed to achieve the objectives. A summary of the different steps of the analysis is provided in Supplementary Figure S6. The results provide an analysis of the output maps, compared to reference data, and as a tool for change monitoring at high resolution. Finally, a discussion of the limitation of such remote sensing derived vegetation height maps is provided.
2 Data and methods
2.1 GEDI waveform data
The Global Ecosystem Dynamics Investigation (GEDI) is a LiDAR mission launched in 2018 onboard the International Space Station (ISS). The waveforms are sampled along ISS orbital tracks and from different laser beams to measure waveforms returns. The variations of vegetation structure and the biomass (Dubayah et al., 2020) can then be inferred from the waveform. Since the beginning of science data collection in 2019, GEDI has been providing waveforms at approximately 25 m footprints across the Earth between
2.2 Airborne LiDAR data
Airborne Laser Scanning (ALS) data with contiguous coverage of different areas of California forests was used to validate the accuracy and spatial variations of vegetation structure of the maps created using the GEDI samples and our model. The ALS observations come from multiple sources. Three field sites within the Sierra Nevada region, Lower Teakettle (TEAK), Soaproot Saddle (SOAP), and San Joaquin Experimental Range (SJER) that are acquired as part of the National Ecological Observatory Network (NEON) funded by the National Science Foundation (NSF) (Schimel et al., 2007; National Ecological Observatory Network, 2023). The sites were surveyed during the leaf-on season in 2019 across a variety of elevations and forest types, with TEAK and SOAP sites surveyed in June and SJER surveyed in March for a total area of approximately 70
2.3 Remote sensing data
Three distinct satellite data sources are used as input to the model to predict vegetation height metrics. For optical imagery, we use Sentinel-2 imagery in the visible and infrared bands (Drusch et al., 2012). We specifically use the blue, green, red, and near-infrared bands at their native 10 m spatial resolution. A mosaic was created by taking the median values from cloud-free images captured between April and October for the years 2019–2020 and 2021–2022 over California. The second source is data from the Advanced Land Observation Satellite (ALOS-2), operated by the Japanese space agency, carrying an L-band synthetic aperture radar PALSAR 2 (Rosenqvist et al., 2007). Both polarization (HH, HV) measurements are provided in a mosaic with 25 m resolution for each year. The lower frequency of L-band measurements allows for penetration through the canopy cover, providing complementary information to the visible and infrared observations (Shimada et al., 2014). To match the time periods used from the GEDI measurement, the mean of the 2019 and 2020 mosaics is used to represent the 2019–2020 period, and the 2021 imagery only to represent the 2021–2022 period, as data for 2022 are not available from JAXA yet. The third dataset is the Copernicus digital elevation model (DEM) GLO-30 dataset (Fahrland et al., 2020). Elevation plays a significant role in determining the distribution of vegetation structure and types in California. It is a well-known factor influencing plant traits, and the correlation between tree cover and elevation profiles has been extensively described in previous studies (Critchfield, 1971; Rybansky et al., 2016). Additionally, elevation is crucial in analyzing the impact of topography on LiDAR returns (Khalefa et al., 2013). Wall-to-wall mosaics of these datasets were prepared over California at 10 m spatial resolution for the two analyzed different time periods considered using the Google Earth Engine platform (Gorelick et al., 2017).
2.4 Ancillary data
We selected a set of ancillary datasets for data analysis and validation of vegetation structure maps. The datasets are produced by the California Department of Forestry and Fire Protection (CALFire) in order to facilitate research and forest management across the state. We downloaded the map of Wildlife Habitat Relationship classes (WHRTYPE) from (Mayer and Laudenslayer, 1988; CALVEG and California Wildlife Habitat Relationship, 2022), which represents a comprehensive land cover map with more than 50 vegetation classes for the entire state of California. We also used the extents of recent large fires (
Additionally, the California Department of Water Resources (DWR) has created watershed maps, which are manageable units of land with geographic coherence, averaging around 3
Finally, existing height maps from (Potapov et al., 2021; Lang et al., 2022) were obtained for inter comparison of the height maps. These maps are available at similar resolution from 10 to 30 m and also used LiDAR data from space borne or airborne sensors to train machine learning models relying on different combinations of input data.
2.5 Model implementation
We employ a deep learning model to establish the relationship between the remote sensing predictor variables and the sparsely distributed relative height metrics derived from GEDI waveform measurements. The predictors consist of seven layers: four bands from Sentinel-2 data, two bands from the ALOS2 L-band backscatter, and one band from the GLO-30 digital elevation model. Our method adopts an encoder-decoder architecture similar to the U-Net network (Ronneberger et al., 2015), using an EfficientNet encoder (Tan and Le, 2021), that has demonstrated promising performance in vegetation studies within remote sensing applications (Wagner et al., 2019; Kattenborn et al., 2021). The dataset is divided into training and evaluation sets to assess the model’s generalization capability. Issues can arise when creating the training and evaluation sets with a random sampling (Kattenborn et al., 2022; Ploton et al., 2020). To provide a geographically independent validation set, we define two distinct geographical regions. During the training phase, 10,000 patches of size 512 × 512 pixels with seven bands are randomly extracted from the training area for each epoch. The model produces a 1 × 512 × 512 prediction array of RH98. The mean squared error loss is computed for the pixels that have a value in the output GEDI patch. The loss is summed for each batch of data during training, and the Adam optimizer (Kingma and Ba, 2014) is applied to update the model parameters. The final model is selected as the one with the lowest loss on the validation data from the same year (occurring after 230 epochs). To create the final maps, the trained model is applied over the 2019–2020 data (partially used in training) and on the 2021–2022 data (not used in training). The predicted height map at 10 m resolution are computed per patch of
3 Results
3.1 Forest height maps
The produced map of top of canopy heights (RH98) detects patterns of forests height variations across the state, Figure 1. Showing high mean top canopy height across the northwest coniferous forests (
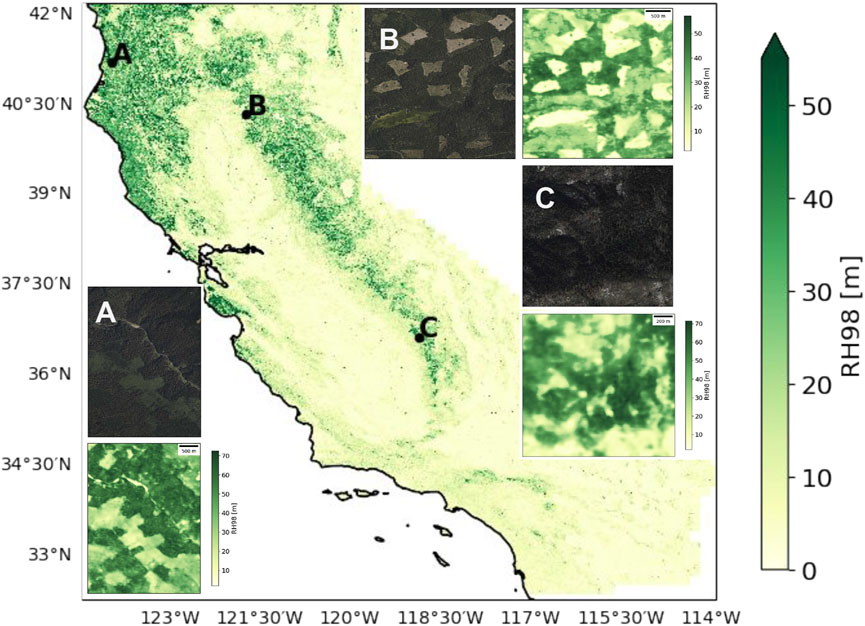
Figure 1. Map of the top canopy height (RH98) of California forests for the period 2019–2020. The three insets (A–C) show a zoom-in of selected sites with RGB satellite imagery from Bing
The heights from GEDI and the model prediction are aggregated for individual watersheds across California. By area, watershed with at least one GEDI observation cover more than 50% of the total state area. The average density of 10 m pixels with a GEDI value in these watersheds is
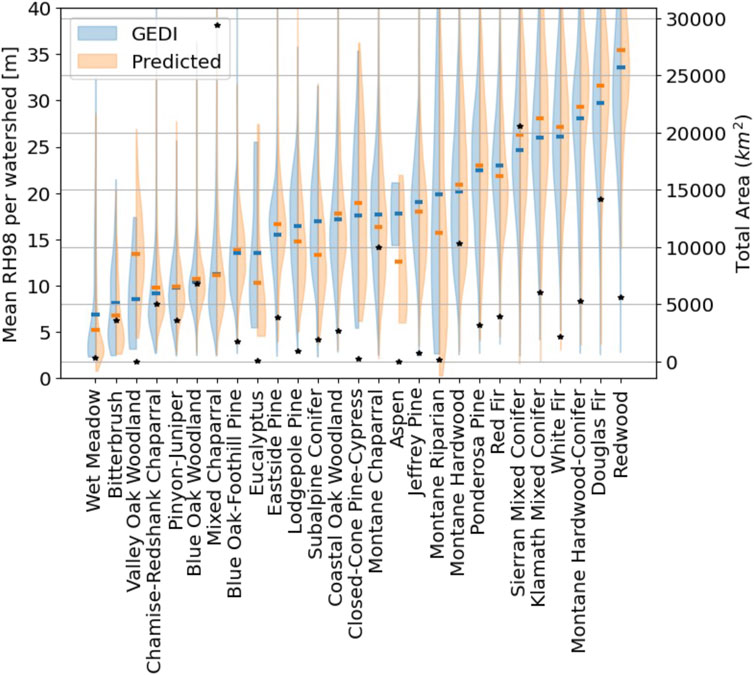
Figure 2. Variations of average top canopy height for California major vegetation types grouped by watershed, the violin plot shows the mean and distribution for all watersheds with a common dominant vegetation type, from GEDI samples (blue) and predicted by the model (orange), the total watershed area of each vegetation type is shown in the secondary axis (black dots).
3.2 Machine learning model performance
The model reached a root mean squared error of 7.1 m on the training set and 4.46 m on the GEDI data validation set. The relative root-mean-square error, and the explained variance between the GEDI pixel values and the predicted values for the training (validation) area are respectively, 43% (56%), 0.74 (0.68) for 2019–2020 and 44% (51%), 0.73 (0.63) for 2021-2022.
The highest explained variance and the lowest RMSE are obtained with the 2019–2020 data used in training by a very slight margin. The generalization of the model is good across time, as all the metrics are consistent between the different data splits. The model generalization across the unseen validation area is slightly lower than other the same area in a different year.
To further analyze the model error in representing variations of GEDI RH98, we compute the residuals between the model prediction and the observed GEDI RH98 values in 2021–2022. Supplementary Figure S2 shows the absolute and relative residuals for bins in predicted height. The absolute error is
3.3 Validation of spatial patterns on airborne LiDAR acquisitions
To further analyze the height predictions, we compare the predicted heights from 2019 to 2020 to the ones measured from ALS in prior years (up to 3 years prior). Figure 3 shows the comparison between the ALS data described in Section 2.2 and the predicted map for 2019–2020. The range of height values from GEDI observations and the predicted map is similar to the ALS height metrics, showing a range of top of canopy height from 0 to 60 m. Measured GEDI RH98 is in good agreement with the ALS top of canopy height across California (R2 = 0.72). The sampling provided by GEDI is on average correct, as shown by the black line closely following the 1:1 red line. However, we observe that the error can be very large for individual pixel values, depicted by the sparse points away from the 1:1 line. This leads to a large standard deviation of GEDI derived metric for each height bin in the ALS data. This can be explained by the original
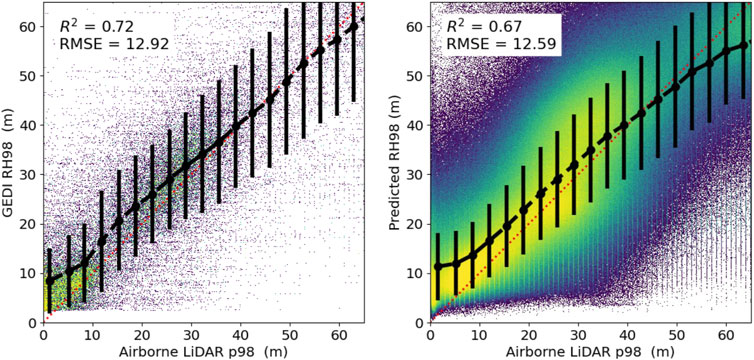
Figure 3. Pixel level comparison between GEDI measurements (first column) and model-predicted (second column) height metrics and the 98th percentile (first row) of ALS data across all the validation sites. Points are colored following the increasing density of pixels from blue to yellow, the mean and standard deviation of the y-axis variable within bins of the x-axis variable is shown in black, the red dotted line shows the 1:1 line.
The spatial patterns of height variability are similar between the predicted height maps and the observed ALS for all the different vegetation types across the sites (Figure 4). The difference between the predicted and reference height in the third column highlights the overestimation of the low values (especially for the SJER site) and some discrepancies in high vegetation, as in the northern part of the SOAP site.
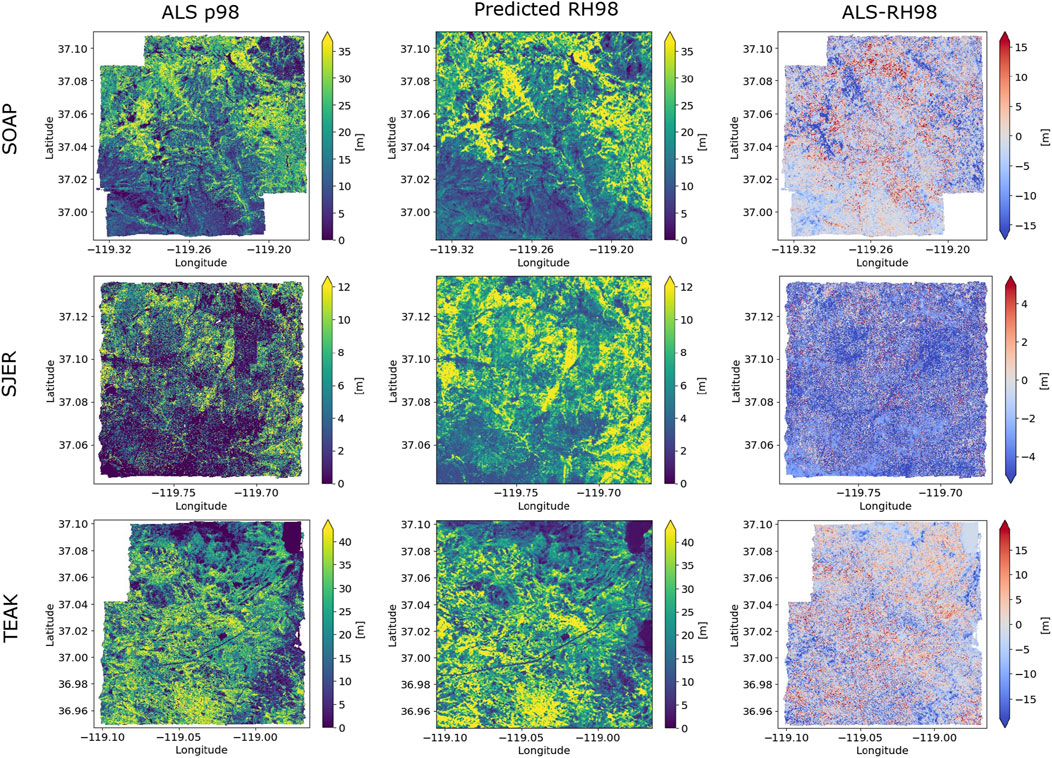
Figure 4. Maps of the model prediction of RH98 and ALS 98th percentile for the three NEON sites (top to bottom SOAP, SJER, TEAK) used for validation, the first column shows the ALS, the second the predicted value and the third one the difference between the first two columns.
3.4 Monitoring forest height
The top of canopy height difference (
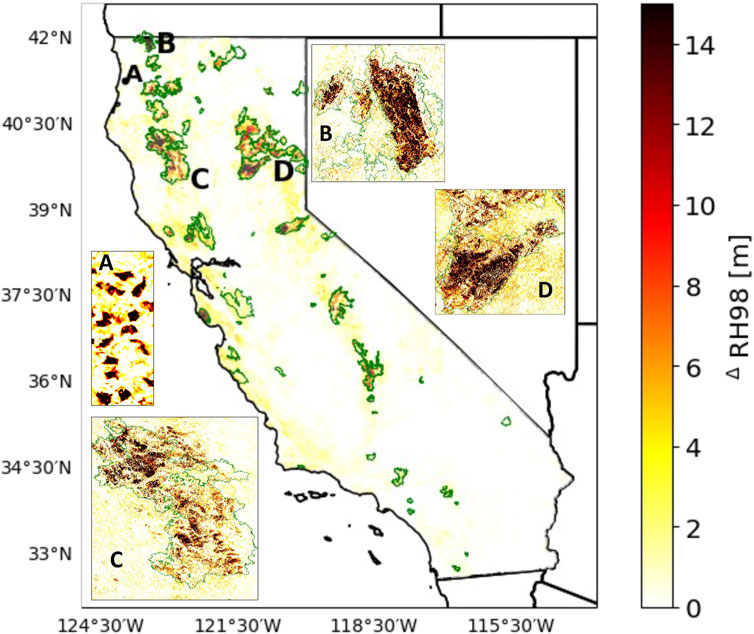
Figure 5. Decrease of canopy height between the 2019–2020 and the 2021–2022 period in the predicted RH98, on the global map at the watershed level and in the insets at the pixel level for a managed area in North-West California (A), the Slater (B), August Complex (C), and North Complex (D) fires, the area delineated in green show the extents of the large fires in California that occurred in 2020 or 2021.
The change of top of canopy height is further analyzed for fires in 2020 and 2021, and compared to the Monitoring Trends In Burn Severity (MTBS) maps output. Figure 6 shows the change of height for each class in the burn severity classification. The larger decrease in height for the increasing severity is clearly apparent. With on average
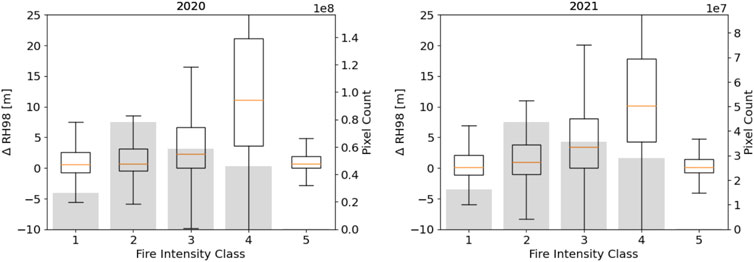
Figure 6. Boxplots of the mean height and interquartile range (rectangle) change between the two predicted RH98 maps for the MTBS maps for the 2020 (left) and 2021 (right) fires within each severity class (1–4 increasing burn intensity, 5 - increasing greenness post fire), the secondary axis shows the number of pixels in each class.
To analyze the agreement between the predicted change and the one measured by GEDI sampling, Supplementary Figure S5 shows the change from the GEDI data averaged at the watershed level. The very sparse nature of the GEDI data makes the analysis at high resolution impossible, as there are very few pixels with repeated observations. However, it is possible to find watersheds with repeated observations and at least 100 pixels with values per square kilometer. The analysis of these repeatedly observed watersheds shows a good agreement between the change in height at the watershed level measured from GEDI and from the maps produced (Supplementary Figure S5, upper right). The largest changes in fire affected areas show up to
Overall, we computed the total area with more than 10 m top of canopy height in 2019-2020 whose height decreased by more than 30% in 2021-2022, and found that 8,874
4 Discussion
4.1 Model of canopy height based on GEDI in California
In California, our model mapped the top of canopy height for two different time periods with an explained variance of about 0.7. This shows the performance of our methodology to predict canopy heights across time and over a large range of values, up to 70 m in redwood forests. Multiple studies have attempted to derive canopy height from remote sensing predictors (Potapov et al., 2021; Lang et al., 2022) at the pixel level or using scene level features with deep learning. All models face challenges to represent the full range of vegetation heights in different parts of the world. This limited sensitivity can be explained by multiple factors: (1) the lack of information in the underlying satellite imagery (optical or radar) to predict height variations in forests. (2) The rare occurrence of very tall trees: in California, only
The global models employ different strategies such as training data rebalancing, or model localization to alleviate the lack of sensitivity to variation of height in different regions. This study shows how a locally trained model (e.g., at the state level), helps improve the predictions compared to global approaches as shown by the reduction in RMSE (
A second challenge faced by remote sensing derived height maps is to achieve the highest spatial resolution possible. The effective resolution of the output maps is coarser than the 10 m resolution of Sentinel-2 observations. Different factors can explain this apparent decrease in resolution. First, the large footprint (
Multi-temporal monitoring of heights requires precise and accurate estimations of vegetation height on different time steps. Although the results agree well with the distribution of heights measured by GEDI, the remaining uncertainties are still too large to accurately estimate growth of different forest types. In addition to the model uncertainties, the measurements available from GEDI have large uncertainties, Figure 3 and overestimate vegetation heights
The current results already provide valuable information for decision makers. They can be used as tools to improve assessment of damages following fires, or integrated in existing fire probability models (Faivre et al., 2014; Li and Banerjee, 2021). Regarding the impact of wildfires on the Californian forests, we find that about 8,874
5 Conclusion
In this paper, we show how remote sensing observations and sparse GEDI LiDAR observations can be used to create a wall-to-wall maps of vegetation structure in California. A deep learning model is trained using ALOS2-PALSAR mosaics and Sentinel-2 data from the 2019–2020 period to estimate the 98th percentile (RH98) of the GEDI waveform return. The model robustness is also evaluated on the 2021–2022 period, with similar performance. It can therefore be applied to create maps on two separate dates and measure changes in vegetation height caused by degradation. The changes estimated by our method have a very good agreement with existing fire areas in California and provide a finer understanding of the degradation severity at high resolution compared to existing fire intensity maps. We find that between those years, more than 60% of forest (RH98
Data availability statement
The raw data used in this study are available from the original data providers. Derived products supporting the conclusions of this article will be made available by the authors upon reasonable request and without undue restrictions.
Author contributions
SF: Conceptualization, Data curation, Formal Analysis, Investigation, Methodology, Software, Validation, Visualization, Writing–original draft, Writing–review and editing, Resources. JL: Methodology, Software, Writing–review and editing. YY: Formal Analysis, Investigation, Writing–review and editing. RD: Investigation, Validation, Writing–review and editing. FW: Data curation, Supervision, Validation, Writing–review and editing. LS: Conceptualization, Investigation, Methodology, Writing–review and editing. SS: Funding acquisition, Investigation, Project administration, Resources, Supervision, Validation, Writing–review and editing.
Funding
The author(s) declare that financial support was received for the research, authorship, and/or publication of this article. Research was carried out at the Jet Propulsion Laboratory, California Institute of Technology, under a contract (19-ACCESS19-0066) with the National Aeronautics and Space Administration.
Conflict of interest
The authors declare that the research was conducted in the absence of any commercial or financial relationships that could be construed as a potential conflict of interest.
Publisher’s note
All claims expressed in this article are solely those of the authors and do not necessarily represent those of their affiliated organizations, or those of the publisher, the editors and the reviewers. Any product that may be evaluated in this article, or claim that may be made by its manufacturer, is not guaranteed or endorsed by the publisher.
Supplementary material
The Supplementary Material for this article can be found online at: https://www.frontiersin.org/articles/10.3389/frsen.2024.1459524/full#supplementary-material
References
Adam, M., Urbazaev, M., Dubois, C., and Schmullius, C. (2020). Accuracy assessment of gedi terrain elevation and canopy height estimates in european temperate forests: influence of environmental and acquisition parameters. Remote Sens. 12, 3948. doi:10.3390/rs12233948
Anderson, M. C., Norman, J. M., Kustas, W. P., Li, F., Prueger, J. H., and Mecikalski, J. R. (2005). Effects of vegetation clumping on two–source model estimates of surface energy fluxes from an agricultural landscape during smacex. J. Hydrometeorol. 6, 892–909. doi:10.1175/jhm465.1
Asner, G. P., and Mascaro, J. (2014). Mapping tropical forest carbon: calibrating plot estimates to a simple lidar metric. Remote Sens. Environ. 140, 614–624. doi:10.1016/j.rse.2013.09.023
Badgley, G., Freeman, J., Hamman, J. J., Haya, B., Trugman, A. T., Anderegg, W. R., et al. (2022). Systematic over-crediting in California’s forest carbon offsets program. Glob. Change Biol. 28, 1433–1445. doi:10.1111/gcb.15943
Boucher, E., and Aires, F. (2023). Improving remote sensing of extreme events with machine learning: land surface temperature retrievals from iasi observations. Environ. Res. Lett. 18, 024025. doi:10.1088/1748-9326/acb3e3
Bruening, J. M., May, P. B., Armston, J. D., and Dubayah, R. O. (2023). Precise and unbiased biomass estimation from gedi data and the us forest inventory. Front. For. Glob. Change 6, 1149153. doi:10.3389/ffgc.2023.1149153
CALVEG and California Wildlife Habitat Relationship (CWHR) (2022). California integrated assessment of watershed health. USFS, CA.gov: USDA.
Coffield, S. R., Vo, C. D., Wang, J. A., Badgley, G., Goulden, M. L., Cullenward, D., et al. (2022). Using remote sensing to quantify the additional climate benefits of California forest carbon offset projects. Glob. Change Biol. 28, 6789–6806. doi:10.1111/gcb.16380
Critchfield, W. B. (1971). Profiles of California vegetation, 76. Pacific Southwest Research Station.
Dalagnol, R., Wagner, F. H., Galvão, L. S., Streher, A. S., Phillips, O. L., Gloor, E., et al. (2021). Large-scale variations in the dynamics of amazon forest canopy gaps from airborne lidar data and opportunities for tree mortality estimates. Sci. Rep. 11, 1388. doi:10.1038/s41598-020-80809-w
Dhargay, S., Lyell, C. S., Brown, T. P., Inbar, A., Sheridan, G. J., and Lane, P. N. (2022). Performance of gedi space-borne lidar for quantifying structural variation in the temperate forests of south-eastern Australia. Remote Sens. 14, 3615. doi:10.3390/rs14153615
Domke, G. M., Oswalt, S. N., Walters, B. F., and Morin, R. S. (2020) “Tree planting has the potential to increase carbon sequestration capacity of forests in the United States. Proc. Natl. Acad. Sci. U. S. A. 117, 24649–24651. doi:10.1073/pnas.2010840117
Drusch, M., Del Bello, U., Carlier, S., Colin, O., Fernandez, V., Gascon, F., et al. (2012). Sentinel-2: esa’s optical high-resolution mission for gmes operational services. Remote Sens. Environ. 120, 25–36. doi:10.1016/j.rse.2011.11.026
Dubayah, R., Blair, J. B., Goetz, S., Fatoyinbo, L., Hansen, M., Healey, S., et al. (2020). The global ecosystem dynamics investigation: high-resolution laser ranging of the earth’s forests and topography. Sci. remote Sens. 1, 100002. doi:10.1016/j.srs.2020.100002
Dubayah, R. O., and Drake, J. B. (2000). Lidar remote sensing for forestry. J. For. 98, 44–46. doi:10.1093/jof/98.6.44
Eitel, J. U., Höfle, B., Vierling, L. A., Abellán, A., Asner, G. P., Deems, J. S., et al. (2016). Beyond 3-d: the new spectrum of lidar applications for earth and ecological sciences. Remote Sens. Environ. 186, 372–392. doi:10.1016/j.rse.2016.08.018
Fahrland, E., Jacob, P., Schrader, H., and Kahabka, H. (2020). “Copernicus dem product handbook,” in Airbus defence and space.
Faivre, N., Jin, Y., Goulden, M. L., and Randerson, J. T. (2014). Controls on the spatial pattern of wildfire ignitions in southern California. Int. J. Wildland Fire 23, 799–811. doi:10.1071/wf13136
Fayad, I., Ciais, P., Schwartz, M., Wigneron, J.-P., Baghdadi, N., de Truchis, A., et al. (2024). Hy-tec: a hybrid vision transformer model for high-resolution and large-scale mapping of canopy height. Remote Sens. Environ. 302, 113945. doi:10.1016/j.rse.2023.113945
Ferraz, A., Saatchi, S., Mallet, C., and Meyer, V. (2016). Lidar detection of individual tree size in tropical forests. Remote Sens. Environ. 183, 318–333. doi:10.1016/j.rse.2016.05.028
Finco, M., Quayle, B., Zhang, Y., Lecker, J., Megown, K. A., and Brewer, C. K. (2012). Monitoring trends and burn severity (mtbs): monitoring wildfire activity for the past quarter century using landsat data. Mov. status trends For. Inventory Analysis (FIA) symposium 2012, 4–6.
Garcia, M., Saatchi, S., Casas, A., Koltunov, A., Ustin, S., Ramirez, C., et al. (2017). Quantifying biomass consumption and carbon release from the California rim fire by integrating airborne lidar and landsat oli data. J. Geophys. Res. Biogeosciences 122, 340–353. doi:10.1002/2015jg003315
García, M., Saatchi, S., Casas, A., Koltunov, A., Ustin, S. L., Ramirez, C., et al. (2017). Extrapolating forest canopy fuel properties in the California rim fire by combining airborne lidar and landsat oli data. Remote Sens. 9, 394. doi:10.3390/rs9040394
Gorelick, N., Hancher, M., Dixon, M., Ilyushchenko, S., Thau, D., and Moore, R. (2017). Google earth engine: planetary-scale geospatial analysis for everyone. Remote Sens. Environ. 202, 18–27. doi:10.1016/j.rse.2017.06.031
Hakkenberg, C. R., Tang, H., Burns, P., and Goetz, S. J. (2023). Canopy structure from space using gedi lidar. Front. Ecol. Environ. 21, 55–56. doi:10.1002/fee.2585
He, S., Xue, H., Cheng, J., Wang, L., Wang, Y., and Zhang, Y. (2022). Tackling the over-smoothing problem of cnn-based hyperspectral image classification. J. Appl. Remote Sens. 16. doi:10.1117/1.jrs.16.048506
Hill, A. P., and Field, C. B. (2021). Forest fires and climate-induced tree range shifts in the western us. Nat. Commun. 12, 6583. doi:10.1038/s41467-021-26838-z
Jeronimo, S. M., Kane, V. R., Churchill, D. J., McGaughey, R. J., and Franklin, J. F. (2018). Applying lidar individual tree detection to management of structurally diverse forest landscapes. J. For. 116, 336–346. doi:10.1093/jofore/fvy023
Jucker, T. (2022). Deciphering the fingerprint of disturbance on the three-dimensional structure of the world’s forests. New Phytol. 233, 612–617. doi:10.1111/nph.17729
Kattenborn, T., Leitloff, J., Schiefer, F., and Hinz, S. (2021). Review on convolutional neural networks (cnn) in vegetation remote sensing. ISPRS J. Photogrammetry Remote Sens. 173, 24–49. doi:10.1016/j.isprsjprs.2020.12.010
Kattenborn, T., Schiefer, F., Frey, J., Feilhauer, H., Mahecha, M. D., and Dormann, C. F. (2022). Spatially autocorrelated training and validation samples inflate performance assessment of convolutional neural networks. ISPRS Open J. Photogrammetry Remote Sens. 5, 100018. doi:10.1016/j.ophoto.2022.100018
Keane, R. E. (2002). Cascading effects of fire exclusion in rocky mountain ecosystems: a literature review
Keane, R. E., Cary, G. J., Davies, I. D., Flannigan, M. D., Gardner, R. H., Lavorel, S., et al. (2004). A classification of landscape fire succession models: spatial simulations of fire and vegetation dynamics. Ecol. Model. 179, 3–27. doi:10.1016/j.ecolmodel.2004.03.015
Kellner, J. R., Armston, J., Birrer, M., Cushman, K., Duncanson, L., Eck, C., et al. (2019). New opportunities for forest remote sensing through ultra-high-density drone lidar. Surv. Geophys. 40, 959–977. doi:10.1007/s10712-019-09529-9
Kellner, J. R., and Asner, G. P. (2009). Convergent structural responses of tropical forests to diverse disturbance regimes. Ecol. Lett. 12, 887–897. doi:10.1111/j.1461-0248.2009.01345.x
Kelly, M., Su, Y., Di Tommaso, S., Fry, D. L., Collins, B. M., Stephens, S. L., et al. (2017). Impact of error in lidar-derived canopy height and canopy base height on modeled wildfire behavior in the sierra Nevada, California, USA. Remote Sens. 10, 10. doi:10.3390/rs10010010
Khalefa, E., Smit, I. P., Nickless, A., Archibald, S., Comber, A., and Balzter, H. (2013). Retrieval of savanna vegetation canopy height from icesat-glas spaceborne lidar with terrain correction. IEEE Geoscience Remote Sens. Lett. 10, 1439–1443. doi:10.1109/lgrs.2013.2259793
Kingma, D. P., and Ba, J. (2014). Adam: a method for stochastic optimization. arXiv Prepr. arXiv:1412.6980. doi:10.48550/arXiv.1412.6980
Kramer, H. A., Collins, B. M., Lake, F. K., Jakubowski, M. K., Stephens, S. L., and Kelly, M. (2016). Estimating ladder fuels: a new approach combining field photography with lidar. Remote Sens. 8, 766. doi:10.3390/rs8090766
Lang, N., Jetz, W., Schindler, K., and Wegner, J. D. (2022). A high-resolution canopy height model of the earth
LaRue, E. A., Downing, A. G., Saucedo, S., Rocha, A., Zesati, S. A. V., Mata-Silva, V., et al. (2023). Diversity–volume relationships: adding structural arrangement and volume to species–area relationships across forest macrosystems. Ecography 2023, e06723. doi:10.1111/ecog.06723
Lefsky, M. A. (2010). A global forest canopy height map from the moderate resolution imaging spectroradiometer and the geoscience laser altimeter system. Geophys. Res. Lett. 37. doi:10.1029/2010gl043622
Li, S., and Banerjee, T. (2021). Spatial and temporal pattern of wildfires in California from 2000 to 2019. Sci. Rep. 11, 8779. doi:10.1038/s41598-021-88131-9
Lim, K. S., and Treitz, P. M. (2004). Estimation of above ground forest biomass from airborne discrete return laser scanner data using canopy-based quantile estimators. Scand. J. For. Res. 19, 558–570. doi:10.1080/02827580410019490
Mayer, K. E., and Laudenslayer, W. F. (1988). A guide to wildlife habitats of California (The Department)
McIntyre, P. J., Thorne, J. H., Dolanc, C. R., Flint, A. L., Flint, L. E., Kelly, M., et al. (2015). Twentieth-century shifts in forest structure in California: denser forests, smaller trees, and increased dominance of oaks. Proc. Natl. Acad. Sci. U. S. A. 112, 1458–1463. doi:10.1073/pnas.1410186112
Meyer, V., Saatchi, S., Chave, J., Dalling, J., Bohlman, S., Fricker, G., et al. (2013). Detecting tropical forest biomass dynamics from repeated airborne lidar measurements. Biogeosciences 10, 5421–5438. doi:10.5194/bg-10-5421-2013
Moura, Y. M. d., Balzter, H., Galvão, L. S., Dalagnol, R., Espírito-Santo, F., Santos, E. G., et al. (2020). Carbon dynamics in a human-modified tropical forest: a case study using multi-temporal lidar data. Remote Sens. 12, 430. doi:10.3390/rs12030430
Næsset, E. (2002). Predicting forest stand characteristics with airborne scanning laser using a practical two-stage procedure and field data. Remote Sens. Environ. 80, 88–99. doi:10.1016/s0034-4257(01)00290-5
National Ecological Observatory Network (NEON) (2023). Discrete return lidar point cloud. doi:10.48443/XXBY-5A18
Ploton, P., Mortier, F., Réjou-Méchain, M., Barbier, N., Picard, N., Rossi, V., et al. (2020). Spatial validation reveals poor predictive performance of large-scale ecological mapping models. Nat. Commun. 11, 4540. doi:10.1038/s41467-020-18321-y
Potapov, P., Li, X., Hernandez-Serna, A., Tyukavina, A., Hansen, M. C., Kommareddy, A., et al. (2021). Mapping global forest canopy height through integration of gedi and landsat data. Remote Sens. Environ. 253, 112165. doi:10.1016/j.rse.2020.112165
Pugh, T. A., Lindeskog, M., Smith, B., Poulter, B., Arneth, A., Haverd, V., et al. (2019). Role of forest regrowth in global carbon sink dynamics. Proc. Natl. Acad. Sci. 116, 4382–4387. doi:10.1073/pnas.1810512116
Quirion, B. R., Domke, G. M., Walters, B. F., Lovett, G. M., Fargione, J. E., Greenwood, L., et al. (2021). Insect and disease disturbances correlate with reduced carbon sequestration in forests of the contiguous United States. Front. For. Glob. Change 4, 716582. doi:10.3389/ffgc.2021.716582
Ronneberger, O., Fischer, P., and Brox, T. (2015) “U-net: convolutional networks for biomedical image segmentation,” in Medical image computing and computer-assisted intervention–MICCAI 2015: 18th international conference, Munich, Germany, october 5-9, 2015, proceedings, Part III, 18. Springer International Publishing.
Rosenqvist, A., Shimada, M., Ito, N., and Watanabe, M. (2007). Alos palsar: a pathfinder mission for global-scale monitoring of the environment. IEEE Trans. Geoscience Remote Sens. 45, 3307–3316. doi:10.1109/tgrs.2007.901027
Roussel, J.-R., Auty, D., Coops, N. C., Tompalski, P., Goodbody, T. R., Meador, A. S., et al. (2020). lidr: an r package for analysis of airborne laser scanning (als) data. Remote Sens. Environ. 251, 112061. doi:10.1016/j.rse.2020.112061
Rybansky, M., Brenova, M., Cermak, J., van Genderen, J., and Sivertun, Å. (2016). Vegetation structure determination using lidar data and the forest growth parameters. IOP Conf. Ser. Earth Environ. Sci. 37, 012031, doi:10.1088/1755-1315/37/1/012031
Saatchi, S., Halligan, K., Despain, D. G., and Crabtree, R. L. (2007). Estimation of forest fuel load from radar remote sensing. IEEE Trans. geoscience remote Sens. 45, 1726–1740. doi:10.1109/tgrs.2006.887002
Saatchi, S. S., Harris, N. L., Brown, S., Lefsky, M., Mitchard, E. T., Salas, W., et al. (2011). Benchmark map of forest carbon stocks in tropical regions across three continents. Proc. Natl. Acad. Sci. 108, 9899–9904. doi:10.1073/pnas.1019576108
Schimel, D., Hargrove, W., Hoffman, F., and MacMahon, J. (2007). Neon: a hierarchically designed national ecological network. Front. Ecol. Environ. 5, 59. doi:10.1890/1540-9295(2007)5[59:nahdne]2.0.co;2
Schwartz, M., Ciais, P., De Truchis, A., Chave, J., Ottlé, C., Vega, C., et al. (2023). Forms: forest multiple source height, wood volume, and biomass maps in France at 10 to 30 m resolution based on sentinel-1, sentinel-2, and global ecosystem dynamics investigation (gedi) data with a deep learning approach. Earth Syst. Sci. Data 15, 4927–4945. doi:10.5194/essd-15-4927-2023
Shimada, M., Itoh, T., Motooka, T., Watanabe, M., Shiraishi, T., Thapa, R., et al. (2014). New global forest/non-forest maps from alos palsar data (2007–2010). Remote Sens. Environ. 155, 13–31. doi:10.1016/j.rse.2014.04.014
Skowronski, N. S., Clark, K. L., Duveneck, M., and Hom, J. (2011). Three-dimensional canopy fuel loading predicted using upward and downward sensing lidar systems. Remote Sens. Environ. 115, 703–714. doi:10.1016/j.rse.2010.10.012
Stark, S. C., Leitold, V., Wu, J. L., Hunter, M. O., de Castilho, C. V., Costa, F. R., et al. (2012). Amazon forest carbon dynamics predicted by profiles of canopy leaf area and light environment. Ecol. Lett. 15, 1406–1414. doi:10.1111/j.1461-0248.2012.01864.x
Su, Y., Ma, Q., and Guo, Q. (2017). Fine-resolution forest tree height estimation across the sierra Nevada through the integration of spaceborne lidar, airborne lidar, and optical imagery. Int. J. Digital Earth 10, 307–323. doi:10.1080/17538947.2016.1227380
Tan, M., and Le, Q. (2021). “Efficientnetv2: smaller models and faster training,” in International Conference on machine learning (PMLR).
Tang, H., Brolly, M., Zhao, F., Strahler, A. H., Schaaf, C. L., Ganguly, S., et al. (2014). Deriving and validating leaf area index (lai) at multiple spatial scales through lidar remote sensing: a case study in sierra national forest, ca. Remote Sens. Environ. 143, 131–141. doi:10.1016/j.rse.2013.12.007
The Cadmus Group, Inc. (2013). California integrated assessment of watershed health. EPA Report. U.S. Environmental Protection Agency.
Vepakomma, U., St-Onge, B., and Kneeshaw, D. (2008). Spatially explicit characterization of boreal forest gap dynamics using multi-temporal lidar data. Remote Sens. Environ. 112, 2326–2340. doi:10.1016/j.rse.2007.10.001
Vogeler, J. C., Fekety, P. A., Elliott, L., Swayze, N. C., Filippelli, S. K., Barry, B., et al. (2023). Evaluating gedi data fusions for continuous characterizations of forest wildlife habitat. Front. Remote Sens. 4, 1196554. doi:10.3389/frsen.2023.1196554
Wagner, F. H., Sanchez, A., Tarabalka, Y., Lotte, R. G., Ferreira, M. P., Aidar, M. P. M., et al. (2019). Using the u-net convolutional network to map forest types and disturbance in the atlantic rainforest with very high resolution images. Remote Sens. Ecol. Conservation 5, 360–375. doi:10.1002/rse2.111
Wang, J. A., Randerson, J. T., Goulden, M. L., Knight, C. A., and Battles, J. J. (2022). Losses of tree cover in California driven by increasing fire disturbance and climate stress. AGU Adv. 3, e2021AV000654. doi:10.1029/2021av000654
Williams, C. A., Gu, H., MacLean, R., Masek, J. G., and Collatz, G. J. (2016). Disturbance and the carbon balance of us forests: a quantitative review of impacts from harvests, fires, insects, and droughts. Glob. Planet. Change 143, 66–80. doi:10.1016/j.gloplacha.2016.06.002
Wulder, M. A., Bater, C. W., Coops, N. C., Hilker, T., and White, J. C. (2008). The role of lidar in sustainable forest management. For. Chron. 84, 807–826. doi:10.5558/tfc84807-6
Keywords: forest height, remote sensing, machine learning, California, wildfire, change detection, GEDI
Citation: Favrichon S, Lee J, Yang Y, Dalagnol R, Wagner F, Sagang LB and Saatchi S (2025) Monitoring changes of forest height in California. Front. Remote Sens. 5:1459524. doi: 10.3389/frsen.2024.1459524
Received: 04 July 2024; Accepted: 23 December 2024;
Published: 22 January 2025.
Edited by:
Saeedeh Eskandari, Research Institute of Forests and Rangelands, IranReviewed by:
Qin Ma, Nanjing Normal University, ChinaYuanhui Zhu, Arizona State University, United States
Copyright © 2025 Favrichon, Lee, Yang, Dalagnol, Wagner, Sagang and Saatchi. This is an open-access article distributed under the terms of the Creative Commons Attribution License (CC BY). The use, distribution or reproduction in other forums is permitted, provided the original author(s) and the copyright owner(s) are credited and that the original publication in this journal is cited, in accordance with accepted academic practice. No use, distribution or reproduction is permitted which does not comply with these terms.
*Correspondence: Samuel Favrichon, c2FtdWVsLmZhdnJpY2hvbkBnbWFpbC5jb20=