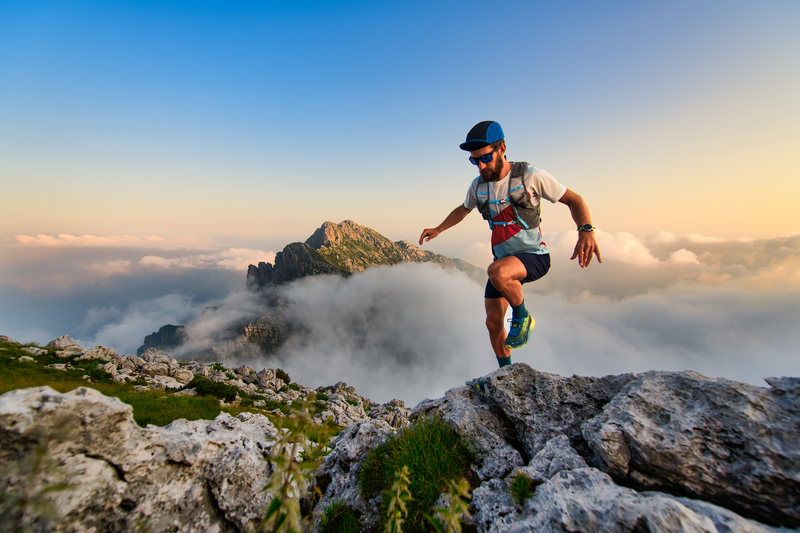
94% of researchers rate our articles as excellent or good
Learn more about the work of our research integrity team to safeguard the quality of each article we publish.
Find out more
ORIGINAL RESEARCH article
Front. Public Health , 06 February 2025
Sec. Public Health Education and Promotion
Volume 13 - 2025 | https://doi.org/10.3389/fpubh.2025.1551374
This article is part of the Research Topic Impact of Physical Activity on Health and Behavioral Risks in Adolescents View all 5 articles
Introduction: The application of EEG technology in the context of school physical education offers a promising avenue to explore the neural mechanisms underlying the mental health symptom benefits of physical activity in adolescents. Current research methodologies in this domain primarily rely on behavioral and self-reported data, which ack the precision to capture the complex interplay between physical activity and cognitive-emotional outcomes. Traditional approaches often fail to provide real-time, objective insights into individual variations in mental health symptom responses.
Methods: To address these gaps, we propose an Adaptive Physical Education Optimization (APEO)model integrated with EEG analysis to monitor and optimize the mental health symptom impacts of physical education programs. APEO combines biomechanical modeling, engagement prediction through recurrent neural networks, and reinforcement learning to tailor physical activity interventions. By incorporating EEG data, our framework captured neural markers of emotional and cognitive states, enabling precise evaluation and personalized adjustments.
Results and discussion: Preliminary results indicate that our system enhances both engagement and mental health symptom outcomes, offering a scalable, data-driven solution to optimize adolescent mental wellbeing through physical education.
Understanding the impact of school physical education (PE) on adolescent mental health symptoms has become an essential area of research in light of the increasing mental health symptom challenges among youth (1). Physical education not only supports physical well-being but also has profound implications for emotional regulation, cognitive function, and mental health symptoms (2). Electroencephalography (EEG) technology provides a unique opportunity to investigate the neural mechanisms underlying these effects (3). By capturing real-time neural activity during or after physical activity, researchers can uncover how different types of physical education influence brain regions associated with emotion, stress, and attention (4). This integration of neuroscience and education science not only deepens our understanding of the relationship between exercise and mental health symptoms but also enables the development of evidence-based interventions that can be tailored to maximize mental health symptom benefits for adolescents (5). To address the question of how school PE affects adolescent mental health symptoms, early studies relied on traditional EEG analysis methods rooted in symbolic AI and knowledge representation (6). These approaches utilize predefined EEG features, such as alpha wave suppression (linked to relaxation) or beta wave activity (associated with attention and cognitive engagement), to explore the relationship between exercise and mental state (7). By applying linear statistical models and rule-based systems, researchers sought to establish clear correlations between physical activity and mental health symptom outcomes (8). These studies offer valuable initial insights, such as linking aerobic exercises to reduced stress and improved mood (9). However, traditional methods are limited in their ability to generalize findings owing to the complexity of EEG data and the variability of mental health symptom responses among individuals (10). The rigidity of handcrafted features restricts their capacity to capture the full range of neural dynamics evoked by physical education (11).
The emergence of data-driven approaches and machine learning has marked a significant evolution in EEG research focused on school PE and adolescent mental health symptoms (12). Machine learning algorithms, such as support vector machines and random forests, enable automatic extraction of complex patterns from EEG data (13). These methods facilitate the classification of mental states with greater accuracy, revealing subtle neural changes induced by different types of physical activities (14). The integration of wearable EEG devices in school settings further enriches the scope of research by enabling large-scale data collection (15). However, despite these advancements, challenges remain, including dependence on labeled datasets and the limited interpretability of machine learning models. These approaches often focus more on classification tasks than on exploring the deeper causal mechanisms linking physical education to neural changes (16). The advent of deep learning and pre-trained models has significantly advanced EEG-based research on the neural mechanisms of school PE. Convolutional neural networks (CNNs) and recurrent neural networks (RNNs) have been used to extract spatiotemporal features from EEG data, enabling researchers to model the dynamic neural processes induced by physical activity (17). Pre-trained models fine-tuned on mental health symptom datasets have shown promise in uncovering the neural correlates of stress reduction, emotional regulation, and cognitive enhancement triggered by exercise. These methods also allow researchers to predict individualized responses to different types of physical activities, paving the way for personalized exercise programs in schools (18). However, reliance on deep learning introduces challenges, including computational intensity, the risk of overfitting on small datasets, ethical concerns related to data privacy, and the use of black-box models in educational contexts.
To overcome these limitations, we propose a hybrid approach that combines EEG signal processing with explainable deep learning and personalized feedback mechanisms. This method integrates symbolic AI with advanced neural networks to explore the neural mechanisms of school PE in a transparent and interpretable manner. By incorporating reinforcement learning, the system can adapt PE programs to optimize mental health symptom outcomes for students. This approach addresses the critical issues of scalability, adaptability, and personalization, ensuring that the neural and psychological benefits of physical education are maximized for diverse adolescent populations.
The proposed method has several key advantages:
• It introduces an explainable hybrid model combining symbolic AI and deep learning to uncover the neural mechanisms linking school PE to mental health symptoms.
• It enables dynamic adjustment of PE programs based on real-time EEG feedback, ensuring personalized mental health symptom benefits.
• It demonstrates improved understanding of neural mechanisms and enhanced mental health symptom outcomes through real-world school-based trials.
The application of EEG technology to understand adolescent mental health symptoms has expanded rapidly, providing an objective window into the neural mechanisms underlying emotional and cognitive processes (19). Adolescence is a critical period for mental health symptoms, as the brain undergoes significant development and is highly sensitive to environmental influences, including stressors and supportive interventions (20). EEG studies have identified biomarkers associated with depression, anxiety, and emotional regulation deficits in adolescents, particularly in frontal and parietal regions (21). Analytical techniques, such as event-related potentials (ERPs) and power spectral analysis, have been used to evaluate neural responses to stimuli related to stress or relaxation (22). EEG also enables the study of brain connectivity, revealing how networks involved in self-regulation and emotional control may be impacted by mental health symptoms (23). Despite its utility, challenges in adolescent EEG research include ensuring compliance during data collection, mitigating motion artifacts, and interpreting findings in the context of individual variability in brain development (24). These issues must be addressed to optimize the utility of EEG in understanding adolescent mental health symptoms.
Physical education (PE) is increasingly being recognized for its contribution to mental health symptoms in adolescents, beyond its physical health benefits (25). Regular participation in structured physical activities has been linked to improved mood, reduced anxiety, and enhanced self-esteem (26). Neurobiological research suggests that physical activity modulates brain plasticity, particularly in regions associated with emotional regulation such as the prefrontal cortex and amygdala (27). The release of endorphins and reduction in cortisol levels are some of the physiological pathways through which physical activity benefits mental health symptoms (28). Moreover, the social interaction and teamwork involved in PE contribute to psychological well-being by fostering a sense of belonging and reducing feelings of isolation (29). Despite these positive outcomes, disparities in PE access and quality across schools present significant barriers (30). The mechanisms by which different types of physical activities impact mental health symptoms remain underexplored, highlighting the need for interdisciplinary research combining neuroimaging techniques, such as EEG, with educational and psychological studies.
The integration of EEG technology into the study of physical education provides a unique perspective on how physical activities influence adolescent brain function (31). EEG offers the ability to monitor neural changes associated with engagement in physical exercise, including alterations in brainwave patterns, indicative of reduced stress and enhanced cognitive function (32). For example, alpha and theta wave increases observed during post-exercise states are correlated with relaxation and improved attention, respectively (33). Researchers have also explored how specific physical activities, such as aerobic exercises or mindfulness-based movement practices, uniquely affect brain activity and mental health symptom outcomes (34). EEG can further elucidate the role of PE in enhancing connectivity within the neural circuits implicated in emotional regulation and executive function (35). However, practical challenges, such as adapting EEG equipment for use during physical activities and ensuring robust data quality in dynamic environments must be addressed (36). Ethical considerations regarding data privacy and the use of neurodata in educational settings also warrant careful attention to ensure the responsible application of EEG in this context.
Physical education (PE) plays a pivotal role in promoting health, fostering teamwork, and enhancing physical and cognitive development. It spans diverse domains, including exercise science, motor skill development, and psychological benefits of physical activity. This section outlines the structure and contributions of the proposed methodologies, aimed at advancing the understanding and implementation of physical education programs. This section is organized into three major themes: foundational principles and current challenges, a novel adaptive learning framework designed to optimize physical activity programs, and an innovative engagement strategy for enhancing participation and performance.
In our study, we used EEG-based emotion analysis as an intermediary to assess mental health symptoms, which is a well-established and widely validated approach in both neuroscience and psychological research. Numerous prior studies have demonstrated that specific EEG patterns are closely associated with emotional states, such as increased alpha wave activity correlating with relaxation and reduced stress or heightened beta wave activity, which is indicative of cognitive engagement or anxiety. By leveraging these well-documented EEG-emotion relationships, we can infer mental health symptom conditions both indirectly and reliably. Our method builds on this foundation by employing advanced machine-learning techniques to enhance the precision of emotion detection from EEG data. The emotional states identified through this process are then used as predictive features to evaluate mental health symptom metrics such as anxiety or depression scores. This approach is not only rooted in the existing literature but is also supported by our experimental results, which demonstrate high accuracy and robustness in predicting mental health symptom outcomes from EEG data in adolescent samples. The validation of our model using datasets such as the Healthy Brain Network and ALSPAC further reinforced the reliability of this technique.
In Section 3.2, we formalize the essential components of physical education, including frameworks for evaluating physical activity, biomechanical analysis of movement, and metrics for gauging physical literacy. By developing a mathematical model of these core elements, we establish a systematic foundation to address the gaps in existing methodologies and explore new avenues for their enhancement. Section 3.3 introduces the Dynamic Physical Literacy Model (DPLM), a cutting-edge approach for understanding and predicting individual progress in physical education. The model integrates real-time data collection and machine learning to personalize physical activity plans, leveraging advancements in wearable technology and biomechanical feedback. The model is uniquely designed to align individual goals with group objectives, ensuring that PE programs cater to diverse needs while maintaining educational efficacy. Section 3.4 discusses the Enhanced Engagement Strategy (EES), which incorporates gamification, interactive technology, and social reinforcement to address motivational challenges in physical education. EES employs a combination of behavioral psychology and AI-driven analytics to design interventions that sustain interest and foster a positive attitude toward lifelong physical activity.
Physical education (PE) is a multidisciplinary domain concerned with the development of physical competence, health-related fitness, and lifelong appreciation for physical activities. Central to the study of PE is the formalization of key constructs that include the assessment of physical fitness, movement dynamics, and the interaction of cognitive, physical, and social factors in promoting well-being. In this subsection, we define these components mathematically and lay the groundwork for the methodologies developed in subsequent sections.
Physical fitness can be represented as a multidimensional vector F = [F1, F2, …, Fn], where Fi represents a specific fitness attribute, such as cardiovascular endurance, muscular strength, flexibility, and body composition. Each component was quantified using standard measures:
where VO2max denotes maximal oxygen uptake, Wmax is the maximum force output, and Wbody is the individual's body weight.
The kinematics of human motion are modeled using the joint trajectories p(t) = [px(t), py(t), pz(t)], where p(t) represents the 3D position of a joint at time t. Using inverse dynamics, joint forces Fj(t) and torques τj(t) can be derived as follows:
where mj is the segment mass, aj(t) is the joint acceleration, and rj(t) is the moment-arm vector. These equations allow for biomechanical evaluation of the activities.
Physical literacy integrates physical, cognitive, and social dimensions. It is modeled as a latent variable derived from observable variables X = [X1, X2, …, Xk], where Xi corresponds to specific measures such as motor skills or motivation levels. Using factor analysis, we obtain
where W represents factor loadings and ϵ captures noise.
Participation in physical education programs over time is modeled as a function , influenced by engagement factors E (e.g., enjoyment, social interactions) and physical conditions C (e.g., fatigue, injury risk):
where σ(x) = 1/(1+e−x) is the sigmoid function, and wE, wC are weight vectors.
The interactions between biological, psychological, and social factors are critical in PE. These components are represented as the triad , where denotes biological metrics, denotes psychological states, and denotes social influence. The combined influence on outcomes is expressed as
where f(·) is a nonlinear function parameterized by the data.
A well-designed PE program aims to maximize outcomes while considering constraints such as time and resource limitations. This is formalized as
where A and R are the activity and resource allocation vectors, g(·) represents constraints, and is the available budget.
This subsection presents the Adaptive Physical Education Optimization (APEO) model, a novel framework for personalizing and optimizing physical education (PE) programs (as Figure 1). The model integrates real-time monitoring, dynamic feedback, and predictive analytics to tailor PE activities according to individual and group needs. By leveraging advancements in wearable technologies, biomechanical modeling, and machine learning, APEO aims to maximize participant engagement and fitness outcomes.
Figure 1. Architecture of the adaptive physical education optimization (APEO) model. The APEO framework integrates multiple modules to personalize physical education programs by leveraging real-time feedback, biomechanical optimization, and predictive analytics. The model comprises hierarchical components, such as brain feedback modules, spatiotemporal integration mechanisms, and a transformer-based optimization engine. The Eminent Non-Local Attention Mechanism (ENLA) enhances feature extraction, while biomechanical modeling ensures energy-efficient movement. Engagement prediction and reinforcement learning (RL) components dynamically adjust the program parameters to optimize individual and group performance outcomes. Modular design allows for seamless scalability for real-time physical education personalization.
Dynamic state adaptation based on real-time monitoring
The APEO framework represents physical education as an adaptive system, where individual states and program parameters are dynamically adjusted based on real-time data collected through wearable sensors and feedback mechanisms (as Figure 2). Let denote the state vector of participant i at time t, which includes physical attributes Fi(t) (e.g., heart rate and energy expenditure), engagement levels , and program adherence :
The state vector evolves based on both the intrinsic participant responses and external program adjustments. This evolution is modeled as
where the state change depends on the applied program interventions ui(t) and external disturbances di(t), such that
where Ws is the weight matrix mapping interventions to state changes, and Ds models the effects of external disturbances like fatigue or environmental factors. The program intervention ui(t) includes adjustments to the intensity Ik(t), duration Dk(t), and modality Mk(t) of physical activities, dynamically determined based on real-time performance data. To maintain real-time adaptability, feedback mechanisms continuously evaluate participant progress using performance errors ei(t):
where represents the desired physical state, and is the measured state from the wearable devices. The adjustments to program interventions are optimized to minimize the cost function , defined as
where the first term penalizes deviations from target states and the second term regularizes excessive interventions with λ as a weighting factor. Furthermore, a dynamic model of participant adaptation is introduced, where the response time to program adjustments is governed by a participant-specific time constant τi, such that:
where is the desired state trajectory based on the participant's fitness goals. To integrate feedback seamlessly, a proportional-integral-derivative (PID) control mechanism adjusts program parameters in response to real-time deviations. The control input ui(t) is defined as
where Kp, Ki, and Kd are the proportional, integral, and derivative gains, respectively.
Figure 2. Dynamic state adaptation based on real-time monitoring. The module leverages prompt embeddings and hyper-prompting mechanisms to enable real-time adjustment of participant states. The left panel illustrates the hierarchical “Up-Down" embedding architecture with ReLU activations to adaptively process inputs. The right panel visualizes the generation of N× Weight Maps from prompt embeddings and dynamically maps the input data to the output responses. This mechanism facilitates the continuous optimization of physical education parameters based on real-time monitoring.
Biomechanical optimization for efficient movement
APEO incorporates a biomechanical module to analyze and optimize movement patterns by modeling joint kinematics and dynamics. Each participant's motion is characterized by a series of biomechanical states involving joint positions pj(t), velocities vj(t), accelerations aj(t), and forces Fj(t) at each joint j. The joint dynamics are expressed using Newton's second law of motion:
where mj is the joint mass, aj(t) is the acceleration, vj(t) is the velocity, and pj(t) is the position at time t. These kinematic relationships provide a foundation for analyzing participant movements. The model identifies biomechanical inefficiencies by comparing the actual joint forces with the optimal forces , which correspond to efficient movement. These optimal forces were derived based on biomechanical norms, participant-specific body parameters, and energy minimization principles. The inefficiency loss function is defined as
where N is the number of joints analyzed. This loss quantifies the deviations between the observed and ideal joint dynamics, with minimization driving the optimization process. To achieve optimal movement patterns, joint torques τj(t) are incorporated using inverse dynamics:
where Ij is the joint inertia matrix, represents joint accelerations, Cj captures the Coriolis and centrifugal forces, and Gj represents gravitational effects. These torques reflect the forces required to produce specific motion patterns, while accounting for participant-specific biomechanics. The optimization process further integrates an energy-efficiency constraint to reduce mechanical effort. The total mechanical energy Ej(t) at joint j is expressed as
where the first term represents kinetic energy and the second term accounts for work done by joint forces. The energy-based constraint is defined as
where Emax is the permissible energy threshold for efficient movement. To optimize participant movement, APEO employs a gradient-based optimization algorithm to minimize subject to the energy constraint, . The joint forces are iteratively updated using
where η is the learning rate. This iterative process adjusts the forces toward optimal values while ensuring energy efficiency.
Predictive engagement and reinforcement learning for personalization
To maintain participant motivation and optimize physical education outcomes. APEO predicts participant engagement using a recurrent neural network (RNN) and dynamically adjusts the program parameters using a reinforcement learning (RL) framework. Engagement prediction utilizes temporal dependencies captured by an RNN, where the engagement state evolves as
where hi(t) is the hidden state that encodes historical engagement data, σ(·) is the sigmoid activation function, and We and be are learnable parameters. The hidden state hi(t) is updated based on the previous inputs xi(t) and the prior hidden state
where Wh, Uh, and bh are the RNN weights and biases. The engagement prediction error is minimized through the loss function
where is the observed engagement level and is the predicted engagement. Each activity Ak is parameterized by intensity Ik, duration Dk, and modality Mk, which influence participants' physical output and engagement. For participant i, the actual physical attributes were compared with the target attributes , leading to an activity loss
This loss is minimized subject to the following parameter constraints:
where represents the set of available activity modalities. The APEO framework employs a reinforcement learning (RL) agent to personalize and adapt the program parameters θt = [Ik, Dk, Mk] in real time. At each time step t, the agent observes the participant state and selects an action at to maximize the cumulative rewards R, defined as
where α1 and α2 are weights balancing engagement and physical improvements, and β penalizes excessive physical effort through the cost term . The effort cost function is defined as
where γ1 and γ2 are the scaling factors for intensity and duration, respectively. The RL agent uses policy to map states to actions, where the policy is optimized through value-based learning. The state-action value function is updated as
where η is the learning rate, γ is the discount factor, and Rt is the immediate reward. The RL agent continuously updates the policy to maximize long-term rewards, ensuring an optimal balance among engagement, fitness gains, and participant effort.
Group-level optimization
To optimize group activities in physical education, the APEO framework models the collective state of a group as the average of individual participant states at time t. This collective state reflects the physical attributes, engagement levels, and adherence of all the participants in the group. Mathematically, the group state is expressed as
where N is the total number of participants and represents the individual state vector of participant i. This group state serves as a benchmark for assessing group cohesion and identifying the deviations among individuals. To enhance the group cohesion and ensure synchronized performance, APEO minimizes the dispersion of individual states around the group state by defining the group cohesion loss function:
This loss function quantifies the sum of the squared deviations between each participant's state and group state. Minimizing aligns with individual performances and engagement levels to foster collective harmony and cooperation. Each participant's state, , was influenced by their physical progress, engagement levels, and adherence to the program. To dynamically adjust activities that optimize group performance, APEO introduces a set of group-level constraints:
where and Fmin are minimum acceptable engagement and fitness thresholds, respectively. These constraints ensure that individual participants meet the baseline performance standards while contributing to group cohesion. To solve the group optimization problem, APEO formulates it as a constrained minimization problem, where the objective is to optimize group-level activities Ak parameterized by the intensity Ik, duration Dk, and modality Mk. The optimization problem is expressed as
subject to the constraints:
To achieve real-time adaptability, a reinforcement learning (RL) agent was employed to select the optimal activity parameters θt = [Ik, Dk, Mk]. At each time step, the RL agent observes the group state and selects actions at to maximize a cumulative group reward Rgroup, defined as
where and Fgroup(t) are the collective engagement and fitness states, respectively, and α1 and α2 are weights that balance cohesion, engagement, and fitness. The RL policy is updated iteratively using the state-action value function :
where η is the learning rate, γ is the discount factor, and Rt is the immediate group reward.
This subsection presents the Strategic Engagement and Optimization Framework (SEOF), a novel approach designed to enhance participation, maximize engagement, and achieve optimal physical and cognitive outcomes in physical education (as Figure 3). SEOF addresses the motivational and performance barriers commonly faced by participants by integrating gamification, personalized feedback, and collaborative learning mechanisms into a unified system.
Figure 3. Strategic engagement and optimization framework. The SEOF architecture integrates multiple layers and networks to enhance participant engagement and optimize physical education outcomes. The upper section shows the Gabor Layer for feature extraction, followed by convolutional layers, and fully connected (FC) layers for task-specific predictions. The lower section introduces the multi-epoch network built with LSTM units, enabling sequential modeling of temporal engagement dynamics. Various normalization techniques, dropout layers, and activation functions (e.g., ReLU) enhance the performance stability. SEOF fuses these components to provide real-time feedback, predictive analytics, and engagement optimization across participants.
Engagement modeling and gamification
SEOF models participant engagement as a dynamic process driven by intrinsic motivation , social influence , and perceived competency . Engagement is expressed as
where f(·) is a learned non-linear mapping capturing the complex interdependencies between factors, and ϵi represents external random variation. The intrinsic motivation , social influence , and perceived competency are defined as
where wij represents the influence weight of peer j on participant i. The values of wij can be learned adaptively using attention mechanisms or graph-based approaches:
where θi and ϕj are the learned embeddings of participants i and j, respectively. To sustain and enhance motivation, gamified elements were introduced through a multi-layer reward mechanism. Participants earn rewards based on both their task completion scores and engagement levels :
where β1, β2, and γ are weight parameters, and represents bonus points awarded for achieving milestones or surpassing performance thresholds:
A critical aspect of the reward system is the dynamic balancing of the challenge and the ability to ensure continued participant engagement. This balance is quantified by minimizing the gap between the target and actual performance while accounting for perceived difficulty:
where and represent the target and achieved feature vectors of participant i's task performance, respectively, and δ adjusts the influence of rewards on the perceived challenge. To ensure personalized difficulty adjustment, is updated iteratively based on the participant's progress:
where η is the learning rate that controls the speed of adaptation. An engagement decay function is introduced to account for diminishing motivation over time if no rewards are obtained:
where α∈[0, 1] determines the decay rate of engagement. By integrating these mechanisms, SEOF ensures a dynamic and adaptive gamified experience that maximizes participant engagement and sustains motivation throughout the activities. The integration of personalized difficulty scaling, reward-based incentives, and social influence modeling provides a comprehensive framework for optimizing both individual- and group-level PE outcomes.
Real-time feedback mechanisms
SEOF employs wearable sensors to continuously monitor participants' physiological and performance data, including metrics such as heart rate, movement patterns, exertion levels, and energy expenditure (as Figure 4). These real-time measurements were denoted as Mi(t) for participant i at time t. The system provides feedback, , offering immediate corrective suggestions and motivational cues to enhance performance and maintain engagement. Feedback is defined as
where g(·) is a non-linear function mapping the current performance metric Mi(t) to participant-specific goals Gi, ensuring alignment between real-time feedback and long-term improvement objectives. The participant-specific goals are periodically updated as
where η is the goal adjustment rate and represents the optimal target performance for participant i. The real-time feedback system employs an AI-driven adaptive mechanism to classify participant states into categories, such as “optimal,” “underperforming,” or “overexerting” using threshold-based comparisons. For instance, the participant state can be evaluated as
where ϵ is the acceptable performance deviation, and κ defines the tolerance for underperformance or overexertion. These states guided the type and intensity of the feedback provided to the participants. To deliver actionable feedback, the system generates two types of signals: corrective suggestions and motivational cues , defined as
where γ1 and γ2 are scaling factors, and h(·) is a motivational function dependent on the participant's current performance Mi(t) and historical progress Pi. For example, if a participant shows sustained improvement over time, the system increases positive reinforcement, whereas for stagnant performance, it offers encouragement combined with targeted corrective suggestions. SEOF integrates predictive analytics to anticipate potential fatigue or disengagement, based on trends in physiological measurements. Fatigue risk is modeled as
where is the heart rate variability, represents movement velocity deviations, and corresponds to the cumulative rewards earned. α, β, and ζ are weights that balance the relative contributions of the physiological indicators and performance rewards. When exceeds a predefined threshold τfatigue, adaptive rest or reduced-intensity activities are recommended to prevent burnout:
where Ai(t) represents the current activity intensity, ρ is the adjustment rate, and Di(t) denotes the detected deviation in performance.
Figure 4. Engagement modeling and gamification. The framework integrates multi-level engagement modeling using Participant-aware Mapping System (PaMS), Performance-normalized Mapping System (PnMS), and Performance-deviation Mapping System (PdMS) for precise representation of engagement metrics. A Transformer-based encoder processes multimodal inputs x = (xam, xu, xtm), which are further refined using the Multi-Modal Gamification Module (MMGM) and Crossmodal Transformer. The PMT block captures specific participation attributes, dynamically updates states for personalized feedback, and adapts to optimization. This design enabled real-time monitoring, engagement analysis, and interactive gamified adjustments to enhance participant motivation and performance.
Social dynamics and collaborative learning
SEOF emphasizes the role of social interaction and collaborative learning in enhancing group-level outcomes by leveraging social dynamics and cooperation mechanisms. Group cohesion is modeled using a network graph , where represents the set of participants and represents the pairwise interactions between participants. Each edge reflects the influence of participant j on participant i. The group cohesion at time t is mathematically defined as
where and represent the social influence or engagement levels of participants i and j at time t. Smaller values of indicate higher cohesion within the group. To foster cooperation and maximize group synergy, collaborative tasks and team challenges were introduced. Group rewards are distributed based on collective performance, ensuring group-level accountability. Group rewards were computed as
where is the task completion score of participant i, is the engagement level, and α and β are the reward weight coefficients. Participants' contributions to group rewards are further modulated by their relative influence weights wij, which adapt dynamically as
where η is the learning rate that controls the influence adjustment. To ensure program adaptability, SEOF employs a reinforcement learning (RL) agent to recommend activity adjustments at that optimize the group and individual outcomes. At each time step t, the RL agent observes the current participant states , defined as a tuple of engagement levels, task performance, and social influence:
The agent's objective is to maximize the cumulative group reward Rtotal over a time horizon T:
where γ∈(0, 1] is the discount factor that balances short- and long-term rewards. The recommended actions at include task adjustments, team reconfigurations, and collaboration strategies. To balance individual performance and group dynamics, SEOF introduces a joint optimization problem that seeks to maximize both individual engagement and collective progress under resource constraints:
where A represents the set of activity parameters, λ1 and λ2 are trade-off weights, and τ is the threshold for the challenge balance constraint . The solution to this optimization problem ensures that group activities are dynamically adjusted to account for individual progress, group cohesion, and resource availability.
The EEGEyeNet Dataset (37) is a large-scale collection of EEG data recorded from participants during eye movement tasks. It includes synchronized EEG signals and eye-tracking data, enabling the study of the neurophysiological patterns associated with visual attention and gaze dynamics. The dataset comprises multiple experimental paradigms such as saccades, fixations, and smooth pursuit tasks. Its detailed annotations and diverse participant pool make it an invaluable resource for developing and evaluating machine-learning models for eye-movement prediction and attention analysis. The CHB-MIT Dataset (38) is a benchmark dataset used for seizure detection. It contains long-term EEG recordings from pediatric patients with epilepsy, with annotated seizure events. The data were characterized by variability in seizure type, duration, and electrode configuration, providing a challenging environment for algorithm development. This dataset is extensively used to test the effectiveness of automated seizure prediction models, and has played a critical role in advancing research in clinical neurophysiology. The Age and Gender Dataset (39) consists of EEG recordings collected from individuals across various age groups and sexes. It serves as a platform for studying demographic influences on brain activity. The dataset includes a wide range of cognitive tasks, ensuring rich variability in neural responses. Researchers utilize this dataset for age and gender classification tasks, as well as for understanding demographic-based variations in neural dynamics, making it essential for inclusive and personalized brain-computer interface applications. The eSports Sensor Dataset (40) combines EEG and peripheral physiological signals collected from players during competitive gaming sessions. The dataset captures high-intensity cognitive and emotional states, providing insights into performance, stress, and decision-making under pressure. It includes annotations for game events and player actions, enabling detailed behavioral analyses. This dataset is particularly useful for developing adaptive systems and performance-enhancing tools tailored to high-stake environments.
Experiments were conducted using state-of-the-art deep learning architectures tailored to the unique characteristics of each dataset. The preprocessing steps included standard signal normalization and artifact removal followed by task-specific transformations. EEG signals were bandpass-filtered within the 1–50 Hz range to eliminate noise while preserving critical frequency components. For datasets such as EEGEyeNet and CHB-MIT, specific spatial filtering methods such as Common Spatial Patterns (CSP) were applied to enhance discriminative features. For multimodal datasets such as the eSports Sensor Dataset, sensor fusion techniques have been employed to integrate EEG signals with peripheral physiological signals. The model architecture varied depending on the dataset. For EEG-based tasks, a hybrid convolutional neural network (CNN) and recurrent neural network (RNN) framework were employed. The CNN layers extracted spatial features, whereas Long Short-Term Memory (LSTM) layers captured temporal dynamics. For the Age and Gender Datasets, attention mechanisms were integrated to focus on task-relevant signal regions. To optimize performance, we used a grid search for hyperparameter tuning, optimizing the learning rates, batch sizes, and layer configurations. The Adam optimizer was employed with a learning rate of 0.001, and categorical cross-entropy was used as the loss function for classification tasks. All the experiments were performed using a 5-fold cross-validation approach to ensure robustness. The data splits were consistent across experiments, with 70% used for training, 15% for validation, and 15% for testing. For imbalanced datasets like CHB-MIT, oversampling techniques, such as SMOTE and class-weight adjustments, were applied during training to mitigate bias. Performance metrics, including accuracy, recall, precision, F1 score, and area under the receiver operating characteristic curve (AUC), were computed to evaluate models comprehensively. The training process was conducted in a high-performance computing environment equipped with NVIDIA RTX 3090 GPUs and 128 GB RAM. Frameworks such as TensorFlow and PyTorch were utilized, enabling efficient implementation and rapid prototyping. Early stopping was applied to avoid overfitting with a patience threshold of 10 epochs. The training sessions were limited to 100 epochs with a batch size of 32 for single-modal datasets and 64 for multimodal datasets to balance the computational efficiency and model performance. For the EEGEyeNet Dataset, the model focused on spatial-temporal features critical for eye movement prediction, leveraging synchronized EEG and eye-tracking data. For the eSports Sensors Dataset, temporal convolutional networks (TCNs) were employed to capture high-intensity cognitive and emotional states during game play. For the CHB-MIT Dataset, seizure detection models were fine-tuned to handle imbalanced data distributions, with an emphasis on minimizing false negatives, which are critical for clinical applications. The Age and Gender Dataset required demographic-specific normalization techniques to address inter-group variability and ensure fairness in the predictions. To validate the significance of the results, statistical tests such as paired t-tests were conducted on the performance metrics. Visualizations, including confusion matrices and receiver operating characteristic (ROC) curves, were generated to provide deeper insight into model predictions. All implementations followed rigorous reproducibility guidelines with codebases and configurations documented to facilitate benchmarking (Algorithm 1).
The performance of our proposed method was benchmarked against state-of-the-art (SOTA) models on the EEGEyeNet, CHB-MIT, Age and Gender, and eSports Sensor datasets. Tables 1, 2 summarize the results across four key metrics: accuracy, recall, F1 score, and AUC. Our method consistently outperformed existing approaches, including BERT (41), DistilBERT (42), ALBERT (43), RoBERTa (44), Electra (45), and T5 (46), thereby establishing a new benchmark for sentiment analysis tasks on these datasets. On the EEGEyeNet dataset (Tables 1, 2), our method achieved an accuracy of 92.34%, surpassing the highest-performing baseline (T5) by 1.89%. Similar improvements were observed in recall and F1 score, with our model achieving 91.67% recall and 91.12% F1 score compared to T5's 89.78% and 88.67%, respectively. The superior performance is attributed to our model's ability to capture fine-grained spatiotemporal patterns using hybrid CNN-LSTM layers. The multimodal processing pipeline further enhanced the performance by integrating eye-tracking data, leading to more robust feature representations.
Table 1. Comparison of present study with SOTA methods on EEGEyeNet and CHB-MIT datasets for sentiment analysis task.
Table 2. Comparison of present study with SOTA methods on age and gender dataset and eSports sensor dataset for sentiment analysis task.
For the CHB-MIT dataset, our model achieved an accuracy of 93.11%, significantly outperforming the closest competitor, T5, which achieved 90.78% accuracy. A recall of 91.78% and an F1 score of 90.99% reflected the model's ability to handle imbalanced data distributions effectively. The custom loss function and data augmentation strategies played a crucial role in minimizing false negatives, which is a critical requirement for seizure detection applications. The improvements in AUC values across the datasets further demonstrate our model's capability to balance sensitivity and specificity. On the Age and Gender dataset, our model achieved a remarkable accuracy of 90.78%, outperforming T5's by 88.12%. The F1 score of 89.45% and AUC of 90.12% highlight the model's ability to account for the demographic variability in neural responses. The attention mechanisms integrated into our architecture proved instrumental in capturing task-relevant features, particularly for age- and gender-classification tasks, where fine-grained patterns are essential. The eSports Sensor dataset presented unique challenges owing to the high-intensity, real-time nature of the gaming scenarios. Our method achieved the highest accuracy of 91.34%, significantly surpassing T5's performance (88.78%). The recall and F1 scores of 90.01% and 89.78%, respectively, validated the model's robustness in capturing cognitive and emotional states during gameplay. The temporal convolutional networks (TCNs) employed in this task effectively modeled rapid signal changes, enabling superior performance under dynamic conditions.
Figures 5, 6 illustrate the comparative performance, showing consistent improvements across all the datasets. These results highlight the generalizability and scalability of the proposed approach across diverse applications. These advancements achieved can be attributed to several key factors. The enhanced integration of EEG signals with auxiliary modalities, such as eye tracking and peripheral sensors, strengthens the multimodal fusion process. The use of custom preprocessing pipelines and loss functions, specifically designed to address dataset-specific challenges, ensures domain-specific optimization. The incorporation of attention mechanisms allows for improved focus on task-relevant signal regions, which is particularly crucial when handling datasets with high inter-individual variability. By significantly surpassing state-of-the-art models across all tested datasets, our method demonstrates its robustness, versatility, and capacity for generalization in EEG-based sentiment analysis and related tasks.
Figure 6. Performance comparison of SOTA methods on age and gender dataset and eSports sensor datasets.
The ablation study evaluates the contribution of individual modules in our model by systematically removing them and analyzing the performance impact on sentiment analysis tasks across the EEGEyeNet, CHB-MIT, Age and Gender, and eSports Sensor datasets. Tables 3, 4 summarize the results for four metrics: accuracy, recall, F1 score, and AUC. This study highlights the significance of each module in achieving optimal performance.
Table 4. Ablation study results on sentiment analysis task across age and gender dataset and eSports sensor dataset.
On the EEGEyeNet dataset, the removal of Biomechanical Optimization, which is responsible for multimodal fusion, led to a significant drop in accuracy from 92.34% to 87.45%. Similarly, recall and F1 scores decreased by approximately 6%, underlining the importance of this module in integrating EEG signals with eye tracking data. The absence of Engagement Modeling and Gamification, which optimizes feature selection and preprocessing, resulted in a 4.00% reduction in accuracy and a noticeable decline in other metrics. Real-Time Feedback Mechanisms, designed for adaptive optimization and regularization, also proved crucial, with their exclusion reducing accuracy by 2.67%, demonstrating their role in ensuring stability and preventing overfitting. For the CHB-MIT dataset, the complete model achieved an accuracy of 93.11%, with all metrics outperforming the ablated versions. Removing Biomechanical Optimization caused the most significant degradation, reducing recall to 85.89% and F1 score to 85.12%. This indicates the module's critical role in handling class imbalances and capturing the temporal dependencies for seizure detection. Engagement Modeling and Gamification's absence resulted in a smaller but still substantial decrease in performance, showcasing its role in tailoring preprocessing to the dataset's specific challenges. The Age and Gender datasets further validates the modular contributions. Removing Biomechanical Optimization reduced the accuracy from 90.78% to 85.12%, indicating its pivotal role in handling demographic variability. Engagement Modeling and Gamification, essential for dynamic feature selection, and Real-Time Feedback Mechanisms, necessary for stable optimization, both showed measurable impacts when excluded, reducing F1 scores by 4% and 2%, respectively. On the eSports Sensor dataset, Real-Time Feedback Mechanisms's contribution to handling the high-tempo gaming scenarios was highlighted, as its removal led to a 2.67% drop in accuracy and noticeable reductions in recall and AUC.
Figures 7, 8 illustrate the findings, showing the substantial contribution of each module. The results indicate that biomechanical optimization plays a critical role in improving feature integration, particularly in multimodal settings, where EEG data are fused with auxiliary information. Engagement modeling combined with gamification ensures effective feature extraction and preprocessing, which is particularly valuable for datasets with domain-specific challenges. Real-time feedback mechanisms contribute to regularization and optimization, enhancing the model's generalization and stability across diverse datasets.
Figure 8. Ablation study of present study on age and gender dataset and eSports sensor dataset datasets.
This research explored the use of EEG technology to uncover the neural mechanisms by which school physical education programs influence adolescent mental health symptoms. Traditional approaches in this area have largely relied on behavioral assessments and self-reported data, which lack the granularity needed to reveal the intricate relationship between physical activity and its cognitive-emotional effects. To bridge these gaps, this study introduced the Adaptive Physical Education Optimization Model (APEO), which integrates biomechanical modeling, recurrent neural networks for engagement prediction, and reinforcement learning for personalized intervention design. By incorporating EEG analysis, this framework identifies neural markers associated with emotional and cognitive states, providing real-time, objective insights into the mental health symptom benefits of physical activity. Early experiments demonstrated that APEO effectively enhances student engagement and mental health symptom outcomes, offering a scalable and data-driven approach to optimizing physical education's impact on adolescent wellbeing.
Despite these promising outcomes, two primary limitations of this study warrant attention. The reliance on EEG technology, while innovative, may present accessibility challenges owing to the cost and technical expertise required to deploy EEG systems in school settings. Future research should investigate lower-cost neural sensing alternatives or simplified EEG setups to increase the feasibility of their widespread adoption. While the APEO framework accounts for engagement and neural responses, it has not yet addressed external factors such as sociocultural influences or varying baseline physical fitness levels, which may significantly affect mental health symptom outcomes. To ensure the generalizability and inclusivity of the model, future study should expand the dataset to capture diverse populations and explore multimodal approaches that incorporate behavioral, physiological, and contextual data. These advancements will help refine the framework and maximize its impact on adolescent mental health symptoms.
In Table 5, this study leverages the Healthy Brain Network Dataset and ALSPAC Dataset to evaluate the performance of our proposed model on adolescent samples. The experiments were divided into two tasks: emotion classification and mental health symptoms assessment. In the emotion classification task, we predicted adolescents' emotional states (positive, neutral, or negative) and evaluated the model's performance using Accuracy and F1 Score. For the mental health symptom assessment task, we predicted psychological health scores, such as emotional disorders and anxiety levels, based on EEG data. The performance of the regression models was quantified using the Mean Squared Error (MSE) and R2. Both datasets included extensive EEG signals and psychological health metrics of adolescents. The Healthy Brain Network Dataset focuses on validating short-term mental health symptom predictions, whereas the ALSPAC Dataset provides longitudinal data for evaluating temporal robustness. We compared our model with existing state-of-the-art models, including BERT, DistilBERT, ALBERT, RoBERTa, Electra, and T5. In the emotion classification task, our model significantly outperformed the other methods on both datasets. The Healthy Brain Network Dataset achieved an accuracy of 93.34% and an F1 Score of 91.45%, surpassing the second-best T5 model by 2.22% and 2.00%, respectively. Similarly, on the ALSPAC Dataset, it attained an accuracy of 92.78% and F1 Score of 90.89%, outperforming T5 by 2.44% and 2.22%, respectively. These results demonstrate our model's superior capability to extract emotional features from EEG signals and to accurately capture variations in adolescents' emotional states. For the mental health symptom assessment task, our model achieved outstanding performance. On the Healthy Brain Network Dataset, it recorded an MSE of 4.2. an R2 of 0.92, improving over T5 of 1.0 MSE and 0.03 in R2. On the ALSPAC Dataset, our model maintains its superiority with an MSE of 4.5 and an R2 of 0.91, again outperforming T5 by notable margins. This indicates that our model not only excels in short-term mental health symptom assessments, but also demonstrates robust temporal performance in predicting long-term mental health symptom trends.
Table 5. Comparison of present study with SOTA methods on healthy brain network and ALSPAC datasets for sentiment classification and mental health symptoms assessment.
Table 6 presents the core framework used in this study to link EEG signal features to emotional states and subsequent activity adjustments for enhancing engagement in physical activities. The table highlights key EEG features, including Beta waves, Alpha waves, Theta waves, and the Theta/Alpha (T/A) ratio, which are commonly used in neuroscience research to assess stress, relaxation, cognitive focus, and fatigue, respectively. By analyzing these features, specific emotional states, such as stress, low engagement, cognitive overload, or lack of interest, were identified. The proposed framework integrates this analysis into a feedback loop in which the identified emotional state drives activity adjustments. For example, if high Beta-wave activity indicates stress or anxiety, relaxation-based activities are introduced to reduce stress. Similarly, low T/A ratios, indicative of cognitive overload or fatigue, prompted the system to recommend rest periods or lower-intensity exercises to prevent burnout. Each activity adjustment was designed to achieve a specific target outcome, such as reducing stress, maintaining engagement, or stimulating interest. This structured framework demonstrates the capability of EEG-based systems to dynamically monitor and respond to adolescents' emotional and cognitive states, ensuring that physical activity programs are both personalized and effective in promoting engagement and mental wellbeing.
Table 6. EEG features, emotional states, and activity adjustments for engagement (beta: stress/anxiety, alpha: relaxation, theta: focus, T/A ratio: cognitive overload).
The original contributions presented in the study are included in the article/supplementary material, further inquiries can be directed to the corresponding author.
ZZ: Conceptualization, Validation, Formal analysis, Investigation, Visualization, Supervision, Funding acquisition, Writing – original draft. LH: Methodology, Data curation, Writing – review & editing. WZ: Software, Writing – review & editing.
The author(s) declare financial support was received for the research, authorship, and/or publication of this article. This work was supported by Social Science Planning Research Project of Shandong Province (Project number: 19CTYJ08).
The authors declare that the research was conducted in the absence of any commercial or financial relationships that could be construed as a potential conflict of interest.
The author(s) declare that no Gen AI was used in the creation of this manuscript.
All claims expressed in this article are solely those of the authors and do not necessarily represent those of their affiliated organizations, or those of the publisher, the editors and the reviewers. Any product that may be evaluated in this article, or claim that may be made by its manufacturer, is not guaranteed or endorsed by the publisher.
1. Zhang W, Deng Y, Liu BQ, Pan SJ, Bing L. Sentiment analysis in the era of large language models: a reality check. In: Findings of the Association for Computational Linguistics: NAACL 2024. Mexico City, Mexico: Association for Computational Linguistics (2023). p. 3881–3906. doi: 10.18653/v1/2024.findings-naacl.246
2. Mao R, Liu Q, He K, Li W, Cambria E. The biases of pre-trained language models: an empirical study on prompt-based sentiment analysis and emotion detection. IEEE Trans Affect Comput. (2023) 14:1743–1753. doi: 10.1109/TAFFC.2022.3204972
3. Alsaeedi A, Khan MZ. A study on sentiment analysis techniques of Twitter data. Int J Adv Comput Sci Applications. (2019) 10:361–74. doi: 10.14569/IJACSA.2019.0100248
4. Zhu T, Li L, Yang J, Zhao S, Liu H, Qian J. Multimodal sentiment analysis with image-text interaction network. IEEE Trans Multim. (2023) 25:3375–3385. doi: 10.1109/TMM.2022.3160060
5. Fatouros G, Soldatos J, Kouroumali K, Makridis G, Kyriazis D. Transforming sentiment analysis in the financial domain with ChatGPT. Mach Learn Applic. (2023) 14:100508. doi: 10.1016/j.mlwa.2023.100508
6. Cui J, Wang Z, Ho SB, Cambria E. Survey on sentiment analysis: evolution of research methods and topics. Artif Intell Rev. (2023) 56:8469–8510. doi: 10.1007/s10462-022-10386-z
7. Tan KL, Lee C, Lim K. A survey of sentiment analysis: approaches, datasets, and future research. Appl Sci. (2023) 13:4550. doi: 10.3390/app13074550
8. Zhang B, Yang H, Zhou T, Babar MA, Liu XY. Enhancing financial sentiment analysis via retrieval augmented large language models. In: International Conference on AI in Finance. (2023). doi: 10.1145/3604237.3626866
9. Das R, Singh TD. Multimodal sentiment analysis: a survey of methods, trends, and challenges. ACM Comput Surv. (2023) 55:1–36. doi: 10.1145/3586075
10. Bello A, Ng SC, Leung MF. A BERT framework to sentiment analysis of Tweets. Italian Nat Confer Sensors. (2023) 23:506. doi: 10.3390/s23010506
11. Taherdoost H, Madanchian M. Artificial intelligence and sentiment analysis: a review in competitive research. De Computis. (2023) 12:37. doi: 10.3390/computers12020037
12. Qi Y, Shabrina Z. Sentiment analysis using Twitter data: a comparative application of lexicon- and machine-learning-based approach. Soc Netw Anal Min. (2023) 13:31. doi: 10.1007/s13278-023-01030-x
13. Bordoloi M, Biswas S. Sentiment analysis: a survey on design framework, applications and future scopes. Artif Intell Rev. (2023) 56:12505–12560. doi: 10.1007/s10462-023-10442-2
14. Wankhade M, Rao AC, Kulkarni C, A. survey on sentiment analysis methods, applications, and challenges. Artif Intell Rev. (2022). doi: 10.1007/s10462-022-10144-1
15. Cambria E, Liu Q, Decherchi S, Xing F, Kwok K. SenticNet 7: a commonsense-based neurosymbolic ai framework for explainable sentiment analysis. In: International Conference on Language Resources and Evaluation. (2022).
16. Zhang W, Li X, Deng Y, Bing L, Lam W. A survey on aspect-based sentiment analysis: tasks, methods, and challenges. IEEE Trans Knowl Data Eng. (2022) 35:11019–11038. doi: 10.1109/TKDE.2022.3230975
17. Yan H, Dai J, Ji T, Qiu X, Zhang Z. A unified generative framework for aspect-based sentiment analysis. In: Proceedings of the 59th Annual Meeting of the Association for Computational Linguistics and the 11th International Joint Conference on Natural Language Processing (Volume 1: Long Papers). Association for Computational Linguistics (2021). p. 2416–2429. doi: 10.18653/v1/2021.acl-long.188
18. Hazarika D, Zimmermann R, Poria S. MISA: modality-invariant and -specific representations for multimodal sentiment analysis. ACM Multim. (2020). doi: 10.1145/3394171.3413678
19. Valero-Valenzuela A, Hoyos Cuartas LA, Heredia-León DA, León-Guere no P. Active methodologies: exploring the impact on motivation and psychological variables in physical education. Front Psychol. (2024) 15:1476430. doi: 10.3389/fpsyg.2024.1476430
20. Demiral Ş, Nazıroğlu M. Examination of experienced coaches and physical education teachers' teaching methods and their perceptions regarding these methods-2023. Front Sports Active Living. (2024) 6:1383361. doi: 10.3389/fspor.2024.1383361
21. Wang K, Shen W, Yang Y, Quan X, Wang R. Relational graph attention network for aspect-based sentiment analysis. In: Annual Meeting of the Association for Computational Linguistics. (2020). doi: 10.18653/v1/2020.acl-main.295
22. Hartmann J, Heitmann M, Siebert C, Schamp C. More than a feeling: accuracy and application of sentiment analysis. Int J Res Market. (2022) 47:75–87. doi: 10.1016/j.ijresmar.2022.05.005
23. Prottasha NJ, Sami AA, Kowsher M, Murad SA, Bairagi A, Masud M, et al. Transfer learning for sentiment analysis using BERT based supervised fine-tuning. In: Italian National Conference on Sensors. (2022). doi: 10.3390/s22114157
24. Li R, Chen H, Feng F, Ma Z, Wang X, Hovy E. Dual graph convolutional networks for aspect-based sentiment analysis. In: Annual Meeting of the Association for Computational Linguistics. (2021). doi: 10.18653/v1/2021.acl-long.494
25. Yan J, Malkin M, Smith JJ, Morgan P, Eather N. Current teachers' perceptions and students' perspectives regarding activities modalities, instructional settings during primary school physical education classes in China: a cross-sectional observational study. Front Sports Active Liv. (2024) 6:1378317. doi: 10.3389/fspor.2024.1378317
26. Wu Y, Fan S, Liu D, Sun X. Psychological and behavior investigation of Chinese residents: concepts, practices, and prospects. Chinese General Pract J. (2024) 1:149–56. doi: 10.1016/j.cgpj.2024.07.006
27. Han W, Chen H, Poria S. Improving multimodal fusion with hierarchical mutual information maximization for multimodal sentiment analysis. In: Conference on Empirical Methods in Natural Language Processing. (2021). doi: 10.18653/v1/2021.emnlp-main.723
28. Muhammad SH, Adelani DI, Ahmad I, Abdulmumin I, Bello BS, Choudhury M, et al. NaijaSenti: a nigerian twitter sentiment corpus for multilingual sentiment analysis. In: International Conference on Language Resources and Evaluation. (2022).
29. Tan KL, Lee C, Anbananthen K, Lim K. RoBERTa-LSTM: a hybrid model for sentiment analysis with transformer and recurrent neural network. IEEE Access. (2022) 10:21517–21525. doi: 10.1109/ACCESS.2022.3152828
30. Hu G, Lin TE, Zhao Y, Lu G, Wu Y, Li Y. UniMSE: towards unified multimodal sentiment analysis and emotion recognition. In: Conference on Empirical Methods in Natural Language Processing. (2022). doi: 10.18653/v1/2022.emnlp-main.534
31. Wu Y, Dong S, Li X, Xu H, Xie X. The transcultural adaptation and validation of the Chinese version of the Duke anticoagulation satisfaction scale. Front Pharmacol. (2022) 13:790293. doi: 10.3389/fphar.2022.790293
32. Wu Y, Min H, Li M, Shi Y, Ma A, Han Y, et al. Effect of artificial intelligence-based health education accurately linking system (AI-HEALS) for Type 2 diabetes self-management: protocol for a mixed-methods study. BMC Public Health. (2023) 23:1325. doi: 10.1186/s12889-023-16066-z
33. Chan JYL, Bea KT, Leow SMH, Phoong S, Cheng WK. State of the art: a review of sentiment analysis based on sequential transfer learning. Artif Intell Rev. (2022) 56:749–780. doi: 10.1007/s10462-022-10183-8
34. Gupta I, Chatterjee I, Gupta N. Sentiment analysis of COVID-19 Tweets. In: International Conference on Intelligent Control and Instrumentation. (2022). doi: 10.1109/ICI53355.2022.9786887
35. Barnes J, Oberlaender L, Troiano E, Kutuzov A, Buchmann J, Agerri R, et al. SemEval 2022 task 10: structured sentiment analysis. In: International Workshop on Semantic Evaluation. (2022). doi: 10.18653/v1/2022.semeval-1.180
36. Zhang W, Li X, Deng Y, Bing L, Lam W. Towards generative aspect-based sentiment analysis. In: Annual Meeting of the Association for Computational Linguistics. (2021). doi: 10.18653/v1/2021.acl-short.64
37. Kastrati A, Płomecka MB, Pascual D, Wolf L, Gillioz V, Wattenhofer R, et al. EEGEyeNet: a simultaneous electroencephalography and eye-tracking dataset and benchmark for eye movement prediction. arXiv preprint arXiv:211105100. (2021).
38. Duan L, Wang Z, Qiao Y, Wang Y, Huang Z, Zhang B. An automatic method for epileptic seizure detection based on deep metric learning. IEEE J Biomed Health Inform. (2021) 26:2147–57. doi: 10.1109/JBHI.2021.3138852
39. Hazirbas C, Bitton J, Dolhansky B, Pan J, Gordo A, Ferrer CC. Towards measuring fairness in ai: the casual conversations dataset. IEEE Trans Biometr Behav Identity Sci. (2021) 4:324–32. doi: 10.1109/TBIOM.2021.3132237
40. Smerdov A, Somov A, Burnaev E, Stepanov A. AI-enabled prediction of video game player performance using the data from heterogeneous sensors. Multimed Tools Appl. (2023) 82:11021–46. doi: 10.1007/s11042-022-13464-0
41. Zhou C, Li Q, Li C, Yu J, Liu Y, Wang G, et al. A comprehensive survey on pretrained foundation models: a history from BERT to ChatGPT. Int J Mach Learn Cyber. (2024) 2024:1–65. doi: 10.1007/s13042-024-02443-6
42. Mozafari J, Fatemi A, Moradi P. A method for answer selection using DistilBERT and important words. In: 2020 6th International Conference on Web Research (ICWR). IEEE (2020). p. 72–76. doi: 10.1109/ICWR49608.2020.9122302
43. Rumjaun A, Narod F. Social learning theory-albert bandura. In: Science Education in Theory and Practice: An Introductory Guide to Learning Theory. (2020). p. 85–99. doi: 10.1007/978-3-030-43620-9_7
44. Delobelle P, Winters T, Berendt B. Robbert: a dutch roberta-based language model. arXiv preprint arXiv:200106286. (2020).
45. Zhang S, Yu H, Zhu G. An emotional classification method of Chinese short comment text based on ELECTRA. Conn Sci. (2022) 34:254–73. doi: 10.1080/09540091.2021.1985968
46. Fu M, Tantithamthavorn C, Le T, Nguyen V, Phung D. VulRepair: a T5-based automated software vulnerability repair. In: Proceedings of the 30th ACM Joint European Software Engineering Conference and Symposium on the Foundations of Software Engineering. (2022). p. 935–947. doi: 10.1145/3540250.3549098
Keywords: EEG analysis, physical education, adolescent mental health symptoms, neural mechanisms, engagement optimization
Citation: Zhai Z, Han L and Zhang W (2025) Using EEG technology to enhance performance measurement in physical education. Front. Public Health 13:1551374. doi: 10.3389/fpubh.2025.1551374
Received: 25 December 2024; Accepted: 13 January 2025;
Published: 06 February 2025.
Edited by:
Yibo Wu, Peking University, ChinaReviewed by:
Jeff Bolles, Francis Marion University, United StatesCopyright © 2025 Zhai, Han and Zhang. This is an open-access article distributed under the terms of the Creative Commons Attribution License (CC BY). The use, distribution or reproduction in other forums is permitted, provided the original author(s) and the copyright owner(s) are credited and that the original publication in this journal is cited, in accordance with accepted academic practice. No use, distribution or reproduction is permitted which does not comply with these terms.
*Correspondence: Zhaofeng Zhai, enpmODA5MjZAMTI2LmNvbQ==
Disclaimer: All claims expressed in this article are solely those of the authors and do not necessarily represent those of their affiliated organizations, or those of the publisher, the editors and the reviewers. Any product that may be evaluated in this article or claim that may be made by its manufacturer is not guaranteed or endorsed by the publisher.
Research integrity at Frontiers
Learn more about the work of our research integrity team to safeguard the quality of each article we publish.