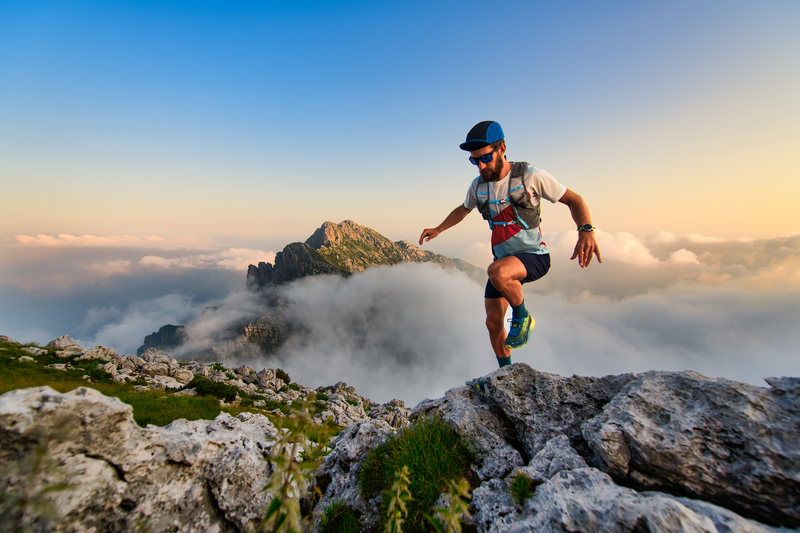
94% of researchers rate our articles as excellent or good
Learn more about the work of our research integrity team to safeguard the quality of each article we publish.
Find out more
ORIGINAL RESEARCH article
Front. Public Health
Sec. Infectious Diseases: Epidemiology and Prevention
Volume 13 - 2025 | doi: 10.3389/fpubh.2025.1544351
The final, formatted version of the article will be published soon.
You have multiple emails registered with Frontiers:
Please enter your email address:
If you already have an account, please login
You don't have a Frontiers account ? You can register here
Objective: Early assessment and intervention of Acquired Immune Deficiency Syndrome (AIDS) patients at high risk of mortality is critical. This study aims to develop an optimally performing mortality risk prediction model for AIDS patients with comorbid AIDS-related diseases or symptoms to facilitate early intervention.Methods: The study included 478 first-time hospital-admitted AIDS patients with related diseases or symptoms. Eight predictors were screened using lasso regression, followed by building eight models and using SHAP values (Shapley's additive explanatory values) to identify key features in the best models. The accuracy and discriminatory power of model predictions were assessed using variable importance plots, receiver operating characteristic curves, calibration curves, and confusion matrices. Clinical benefits were evaluated through decision-curve analyses, and validation was performed with an external set of 48 patients. Results: Lasso regression identified eight predictors, including hemoglobin, infection pathway, Sulfamethoxazole-Trimethoprim, expectoration, headache, persistent diarrhea, Pneumocystis jirovecii pneumonia, and bacterial pneumonia. The optimal model, XGBoost, yielded an Area Under Curve (AUC) of 0.832, a sensitivity of 0.703, and a specificity of 0.799 in the training set. In the test set, the AUC was 0.729, the sensitivity was 0.717, and the specificity was 0.636. In the external validation set, the AUC was 0.873, the sensitivity was 0.852, and the specificity was 0.762. Furthermore, the calibration curves showed a high degree of fit, and the DCA curves demonstrated the overall high clinical utility of the model.Conclusion: In this study, an XGBoost-based mortality risk prediction model is proposed, which can effectively predict the mortality risk of patients with co-morbid AIDS-related diseases or symptomatic AIDS, providing a new reference for clinical decision-making.
Keywords: machine learning, XGBoost, aids, HIV, Prediction model
Received: 12 Dec 2024; Accepted: 17 Feb 2025.
Copyright: © 2025 Chen, Pan, Wubuli, Lu and Maimaiti. This is an open-access article distributed under the terms of the Creative Commons Attribution License (CC BY). The use, distribution or reproduction in other forums is permitted, provided the original author(s) or licensor are credited and that the original publication in this journal is cited, in accordance with accepted academic practice. No use, distribution or reproduction is permitted which does not comply with these terms.
* Correspondence:
Erxiding Maimaiti, Xinjiang Medical University, Ürümqi, China
Disclaimer: All claims expressed in this article are solely those of the authors and do not necessarily represent those of their affiliated organizations, or those of the publisher, the editors and the reviewers. Any product that may be evaluated in this article or claim that may be made by its manufacturer is not guaranteed or endorsed by the publisher.
Research integrity at Frontiers
Learn more about the work of our research integrity team to safeguard the quality of each article we publish.