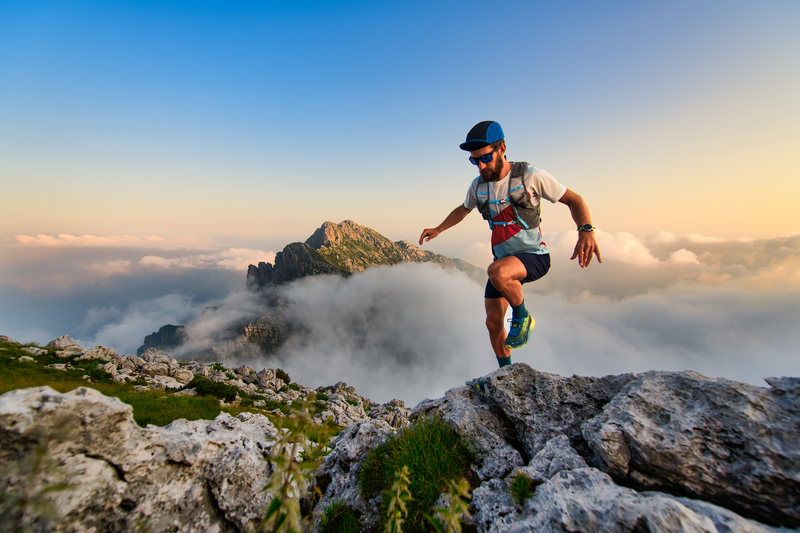
95% of researchers rate our articles as excellent or good
Learn more about the work of our research integrity team to safeguard the quality of each article we publish.
Find out more
ORIGINAL RESEARCH article
Front. Public Health , 21 March 2025
Sec. Aging and Public Health
Volume 13 - 2025 | https://doi.org/10.3389/fpubh.2025.1509458
This article is part of the Research Topic New Environmental Pollutants, Aging, and Age-Related Diseases View all 4 articles
Introduction: Epigenetic biomarkers are molecular indicators of epigenetic changes, and some studies have suggested that these biomarkers have predictive power for disease risk. This study aims to analyze the relationship between 30 epigenetic biomarkers and the risk of diabetes and cancer using machine learning modeling.
Methods: The data for this study were sourced from the NHANES database, which includes DNA methylation arrays and epigenetic biomarker datasets. Nine machine learning algorithms were used to build models: AdaBoost, GBM, KNN, lightGBM, MLP, RF, SVM, XGBoost, and logistics. Model stability was evaluated using metrics such as Accuracy, MCC, and Sensitivity. The performance and decision-making ability of the models were displayed using ROC curves and DCA curves, while SHAP values were used to visualize the importance of each epigenetic biomarker.
Results: Epigenetic age acceleration was strongly associated with cancer risk but had a weaker relationship with diabetes. In the diabetes model, the top three contributing features were logA1Mort, family income-to-poverty ratio, and marital status. In the cancer model, the top three contributing features were gender, non-Hispanic White ethnicity, and PACKYRSMort.
Conclusion: Our study identified the relationship between epigenetic biomarkers and the risk of diabetes and cancer, and used machine learning techniques to analyze the contributions of various epigenetic biomarkers to disease risk.
Diabetes and cancer are major challenges in the field of global public health, with the incidence of these diseases continuing to rise (1–3), making it urgent to develop effective early detection signals and risk prediction tools.
Epigenetic biomarkers, particularly DNA methylation, are an important direction for studying the mechanisms underlying these diseases. DNA methylation is a process in which methyl groups are added to DNA, leading to changes in gene function, such as the silencing of tumor suppressor genes, activation of oncogenes, and abnormalities in promoter regions (4–7). These changes are closely linked to the development of various chronic diseases (8–10). However, existing studies have mostly been limited to a small number of epigenetic biomarkers and lack in-depth exploration of the specific relationships between these biomarkers and diseases in different contexts. Epigenetic age clocks are constructed based on specific DNA methylation sites (CpG sites) and can accurately predict an individual’s “biological age,” which refers to the degree of aging of the body compared to its chronological age. By measuring different DNA methylation patterns, researchers have found that epigenetic age is not only highly correlated with chronological age (11), but its acceleration (the difference between epigenetic age and chronological age) is considered a potential biomarker for aging and various diseases (12). For example, accelerated epigenetic age has been found to be closely associated with several age-related diseases, such as diabetes, cancer, cardiovascular diseases, and others (8, 13, 14).
In this study, we present 30 epigenetic biomarkers that have shown potential associations with diabetes and cancer in research across multiple fields. We expanded the selection scope and considered the biological significance of these biomarkers in the context of various diseases. These include epigenetic clocks (such as HorvathAge, LinAge, PhenoAge, WeidnerAge, etc.), lifespan and mortality-related biomarkers (such as GrimAgeMort, ADMMort, B2MMort, GDF15Mort, etc.), immune system biomarkers (such as CRPMor, CD8TPP, CD4TPP, NKcel, Bcell, etc.), and metabolism-related biomarkers (such as logA1CMort, LeptinMort, PACKYRSMort), which fall into three major categories of epigenetic material. For example, biomarkers like HorvathAge and GrimAge are closely associated with the aging process and the onset of various chronic diseases. LinAge, a biomarker associated with an individual’s age, has demonstrated a strong correlation with biological aging (15). Meanwhile, biomarkers such as LeptinMort and PACKYRSMort, which are linked to metabolic processes, smoking behaviors, and nutrition, have been confirmed in multiple studies to be associated with an increased risk of diabetes (16, 17). Additionally, GDF15Mort is related to age-associated mitochondrial dysfunction (18). Therefore, selecting these biomarkers as the focus of this study aims to explore their predictive ability and underlying mechanisms in diabetes and cancer.
Machine learning (ML), as an emerging artificial intelligence technology, offers advantages over traditional statistical analyses, such as higher predictive accuracy and the ability to maintain high prediction performance even with missing data. It is capable of making accurate predictions by utilizing data from various sources. The application of machine learning techniques in the medical field for disease diagnosis and prediction can significantly contribute to disease prevention and treatment (19, 20). In recent years, machine learning has been applied across various fields of clinical disease diagnosis. For example, studies have widely applied machine learning algorithms in the monitoring of tumors and cardiovascular diseases (21). Other research has used machine learning to analyze regularly collected electronic health record data and construct predictive models for the progression from prediabetes to type 2 diabetes (22).
The core objective of this study is to explore the relationship between different epigenetic age clocks and diabetes and cancer, analyzing the potential role of accelerated epigenetic age in these diseases. Secondly, machine learning techniques were used to construct risk assessment models for diabetes and cancer based on epigenetic biomarkers, and their predictive effectiveness in clinical settings was validated. Lastly, by combining clinical features with epigenetic biomarkers, the study aims to optimize risk prediction models, providing a basis for early disease screening and personalized intervention. Nine machine learning algorithms were selected to build the models, and model evaluations and comparisons were conducted to identify the best model for predicting cancer and diabetes risks. Thirty epigenetic biomarkers were used to predict the risk of diabetes and cancer and visualized for analysis. The goal is to assess the value of each epigenetic biomarker in predicting the occurrence of diabetes and cancer, especially in the early screening and prediction of these diseases. Epigenetics will undoubtedly help unravel more mysteries about aging and its associated diseases, offering strong support for the implementation of precision medicine.
The epigenetic biomarker data in this study are based on the DNA methylation array and epigenetic biomarker datasets from the NHANES database, an epidemiological survey program conducted by the National Center for Health Statistics (NCHS) at the Centers for Disease Control and Prevention (CDC) in the United States. DNA methylation array and epigenetic biomarker data were obtained from blood samples of participants. DNA was extracted from the samples and DNA methylation measurements were performed using the Illumina MethylationEPIC BeadChip. The methylation data were processed, preprocessed, and normalized to generate the epigenetic biomarker data (Table 1) (NHANES website). Sex, age, race, education level, marital status, household income, and poverty ratio were obtained from participant questionnaires and physical measurement datasets. Questionnaire data were collected using standardized questionnaires, and physical measurements were conducted at specially designed and equipped mobile centers.
In our study, race was categorized based on the questionnaire responses into five groups: Mexican American, non-Hispanic White, non-Hispanic Black, other Hispanic, and other races, with values assigned from 1 to 5. Education level was classified as high school or higher vs. less than high school. Marital status was categorized as married, cohabiting, or unmarried/not living with a partner. Household income and poverty ratio were divided into ≥300 and <300%, and BMI was categorized as ≥24 and <24.
Descriptive statistical analyses were performed using R software (version 4.4.1) (Tables 2, 3). For normally distributed continuous variables, means and standard deviations were reported, while for non-normally distributed continuous variables, medians and interquartile ranges were reported. Categorical variables were presented as percentages. Continuous variables were analyzed using t-tests or Mann–Whitney U tests, while categorical variables were analyzed using chi-square tests or Fisher’s exact tests.
The study population was randomly divided into training and validation sets at an 80:20 ratio, followed by standardization preprocessing. Nine machine learning algorithms, including AdaBoost, GBM, KNN, LightGBM, MLP, RF, SVM, XGBoost, and Logistic Regression (see Table 4 for details), were used to develop predictive models.
Oversampling was performed using SMOTE to balance the positive and negative samples, generating more positive samples to alleviate the class imbalance issue. The dataset was then divided into training and test sets. CV was set to 5, indicating 5-fold cross-validation, which was used to assess the average performance of the model under different parameter combinations. The training dataset was randomly divided into five mutually exclusive subsets (folds), with one subset used as the validation set in each iteration, and the remaining four subsets combined for training. This process allowed for 5 training and validation cycles, resulting in five validation outcomes. Grid search (GridSearchCV) was used in conjunction with cross-validation to exhaustively search the parameter grid and return the optimal parameter combination for the machine learning model, thereby achieving the best performing model. Metrics such as Positive Predictive Value, Negative Predictive Value, Sensitivity, Specificity, Matthews Correlation Coefficient, AUC, Kappa, Brier Score, accuracy, and F1 Score were used to evaluate model performance (Tables 5, 6). The Positive Predictive Value (PPV) reflects the accuracy of the model’s positive predictions, with higher values indicating fewer false positives. The F1 score ranges from 0 to 1, with higher values indicating a better balance between precision and recall, making it suitable for imbalanced class problems. The above metrics, combined with ROC curves (Figures 1, 2) and DCA curves (Figures 3, 4), were used to assess the stability and clinical decision-making ability of the model (23).
The ROC curve (Receiver Operating Characteristic Curve) measures the model’s discriminatory ability at various thresholds by plotting the trade-off between the True Positive Rate (TPR) and False Positive Rate (FPR). It is insensitive to the proportion of positive and negative samples and is ideal for evaluating binary classification models comprehensively. The DCA curve is typically used to assess the “net benefit” of a model in practical clinical applications, taking into account the risk–benefit balance at different thresholds. Net benefit is a metric that combines the risks of false positives and false negatives. A high net benefit indicates that the model effectively identifies the disease at that threshold, with a greater contribution compared to the cost of incorrect predictions. The threshold refers to the probability cutoff used by the decision model, which determines whether a sample is classified as positive. A higher threshold reduces false positives but may miss some true positives (i.e., increasing false negatives). We need to select the model that provides higher net benefit across a broader range of thresholds. The best-performing model was selected, and SHAP values were computed and plotted in summary graphs (Figures 5, 6) to report the contribution of each feature. Partial dependence plots (Figures 7–9) were used to visualize the impact of changes in aging-related genetic biomarkers on the outcomes.
Table 3 the diabetes model included a total of 2,205 participants, with 394 diabetic patients, the majority of whom were of other Hispanic descent. The average age of diabetic patients was 66.6 years (SD 8.99), significantly higher than that of non-diabetic patients, which was 65.6 years (SD 10.1), p = 0.048, indicating that age is an important risk factor for tumor occurrence. There was also a significant difference in racial distribution between the two groups (p < 0.001). The proportion of Mexican Americans (34.3%) and other Hispanics (27.1%) was significantly higher in diabetic patients compared to non-diabetic patients (26.6 and 19.7%, respectively), while the proportion of non-Hispanic Blacks was significantly lower in diabetic patients (26.9%) compared to non-diabetic patients (44.2%), indicating a significant racial difference in tumor incidence. The proportion of diabetic patients with a high school education or higher was significantly lower than that of non-diabetic patients (66.5% vs. 75.0%, p = 0.001). In addition, the prevalence of hypertension was significantly higher in diabetic patients compared to non-diabetic patients (65.0% vs. 43.8%, p < 0.001). There was no significant difference in marital status distribution between the two groups (p = 0.918). Age-related indicators: All biological age markers (such as HorvathAge, HannumAge, PhenoAge, etc.) in the diabetic group were significantly higher than those in the non-diabetic group (p < 0.05). Indicators such as HorvathAge acceleration, HannumAge acceleration, SkinBloodAge acceleration, PhenoAge acceleration, ZhangAge acceleration, and LinAge acceleration showed significant differences in diabetic patients (p < 0.05), While some age acceleration indicators, such as WeidnerAge acceleration and VidalBraloAge acceleration, showed no significant differences in diabetic patients (p > 0.05). Indicators such as GDF15Mort, B2MMort, CystatinCMort, TIMP1Mort, ADMMort, PAI1Mort, LeptinMort, CRPMort, logA1CMort, GrimAgeMort, GrimAge2Mort, and YangCell were significantly higher in diabetic patients than in non-diabetic patients (p < 0.05). Indicators such as PACKYRSMort, HorvathTelo, DunedinPoAm, CD8TPP, CD4TPP, Nkcell, Bcell, MonoPP, and NeuPP showed no significant differences between the two groups (p > 0.05).
Table 2 the cancer model included a total of 1,291 participants, with 177 cancer patients, primarily distributed among non-Hispanic Black individuals. The average age of cancer patients (69.1 years, SD 10.00) was significantly higher than that of non-cancer patients (63.8 years, SD 9.69), p < 0.001. The proportion of cancer patients with a high school education or higher (86.2%) was significantly higher than that of non-cancer patients (79.1%), p = 0.039. There was a significant difference in racial distribution between the two groups (p < 0.001). Among non-cancer patients, the proportion of non-Hispanic Black individuals was relatively low (42.4%), while this proportion significantly increased to 68.4% in cancer patients. Additionally, the proportion of Mexican Americans, other Hispanics, and other ethnic groups was significantly lower among cancer patients. There was no significant difference between the two groups in terms of marital status (Marry), prevalence of hypertension (Hypertension), and incidence of diabetes (Diabetes). All age clock indicators (such as HorvathAge, HannumAge, SkinBloodAge, PhenoAge, ZhangAge, LinAge, WeidnerAge, VidalBraloAge, etc.) were significantly higher in cancer patients than in non-cancer patients (p < 0.001). ZhangAge acceleration: Cancer patients had significantly lower values than non-cancer patients (p < 0.001). WeidnerAge acceleration (p = 0.027) and VidalBraloAge acceleration (p = 0.020): The acceleration markers in cancer patients were significantly different from those in non-cancer patients. Other age acceleration markers (such as HorvathAge acceleration, HannumAge acceleration, etc.) showed no significant differences (p > 0.05). Indicators such as GDF15Mort, B2MMort, CystatinCMort, TIMP1Mort, ADMMort, PACKYRSMort, GrimAgeMort, GrimAge2Mort, HorvathTelo, DunedinPoAm, CD4TPP, and NeuPP were significantly higher in cancer patients than in non-cancer patients (p < 0.05). Indicators such as LeptinMort, CRPMort, logA1CMort, YangCell, CD8TPP, Nkcell, Bcell, and MonoPP showed no significant differences between the two groups (p > 0.05), suggesting that these markers may not have a direct association with tumor incidence in this study, or their mechanisms of action are complex and require further exploration with larger sample sizes or multivariate analyses.
Correlation analysis shows that the correlation coefficients between eight epigenetic ages and chronological age are all greater than 0.5 (Table 7), indicating that they are all strongly positively correlated with age, thus confirming the reliability of these clocks.
Further heatmap analysis was performed to examine the correlation between epigenetic age acceleration (epigenetic age – chronological age) and diabetes, as well as cancer (Figures 10, 11). These two heatmaps display the partial correlation coefficients between diseases (diabetes and cancer) and epigenetic age clock acceleration, illustrating the independent correlations between each disease and different age acceleration factors. Partial correlation refers to the correlation coefficient for each number, representing the partial correlation between a specific disease (diabetes or cancer) and a particular age acceleration factor. Positive correlation (red): A positive coefficient indicates that the disease is positively correlated with the acceleration factor (the higher the acceleration factor, the higher the disease risk). Negative correlation (blue): A negative coefficient indicates that the disease is negatively correlated with the acceleration factor (the higher the acceleration factor, the lower the disease risk). Close to 0 (gray): No significant correlation. In the heatmap, the colors range from red (positive correlation) to blue (negative correlation), with gray indicating weak correlations.
The partial correlation coefficients are generally close to 0, indicating that there is almost no significant independent correlation between diabetes and these epigenetic age clock accelerations. The differences in the effects of each acceleration factor on diabetes are minimal and not statistically significant.
Figure 10 LinAge acceleration and ZhangAge acceleration show a weak negative correlation with cancer (−0.19), suggesting that these two acceleration factors may be associated with a reduced risk of cancer. VidalBraloAge acceleration and HannumAge acceleration show a weak positive correlation with cancer (0.10 and 0.05, respectively), indicating that these epigenetic age clock accelerations may be associated with an increased risk of cancer. Other acceleration factors (such as HorvathAge, SkinBloodAge, etc.) show a correlation close to 0 with cancer.
After calculating evaluation metrics and plotting the ROC and DCA curves, this study assesses model performance using multiple indicators, with detailed evaluation values provided in Tables 5, 6, which present the performance evaluation of the diabetes and cancer prediction models. This study plots the ROC curves for various models of diabetes and cancer. According to the definition of the ROC curve, as the threshold changes, the curve should ideally be as “convex” as possible toward the top-left corner. Regions with high TPR and low FPR indicate better classifier performance, so LightGBM is chosen as the model with the best performance. Models with higher DCA curves indicate greater net benefit at the corresponding threshold probabilities, thus demonstrating superior performance. Different models may perform differently across different threshold ranges. For example, in the low threshold range (<0.2), some models (such as Logistic Regression and LightGBM) may have higher net benefits. In the middle range (0.2–0.6), models like Gradient Boosting and RF may outperform others. At high thresholds (>0.7), the net benefit of the models may approach that of the “Treat None” strategy (no intervention). The reference significance of “Treat All” and “Treat None”: “Treat All” is an extreme strategy where all patients are treated. “Treat None” is another extreme strategy where no patient receives intervention. When the model’s curve lies between “Treat All” and “Treat None,” it indicates that the model’s prediction lacks clinical value. Selection of the optimal model: Considering the overall threshold range, the model that consistently stays above “Treat All” and “Treat None” while covering a broader threshold probability range is the optimal choice. From this DCA curve, it is observed that Gradient Boosting and LightGBM demonstrate superior net benefits across larger threshold ranges. This study evaluates model performance using various indicators, with detailed evaluation values provided in Tables 5, 6, which present the performance evaluation of the diabetes and cancer prediction models. In the diabetes prediction, LightGBM achieved an AUC of 1.00, an MCC of 0.97, and an F1 Score of 0.98 on the validation set, while GBM had an AUC of 0.99, an MCC of 0.93, and an F1 Score of 0.95 (Table 5). LightGBM is thus selected as the best model. Similarly, in cancer prediction, LightGBM achieved an AUC of 0.98, an MCC of 0.83, and an F1 Score of 0.91 (Table 6). Therefore, it can be concluded that the LightGBM model is the best comprehensive model for assessing the risk of diabetes and cancer, and is selected for use.
The SHAP value summary plot reports that the top three contributing features in the diabetes model are logA1Mort, family income-to-poverty ratio, and marital status (Figure 5). In the cancer model, the top three contributing features are gender, non-Hispanic white, and PACKYRSMort (Figure 6). Based on the above SHAP value summary plot, partial dependence plots for important features were drawn. An increase in logA1Mort is positively correlated with the risk of diabetes (Figure 9). When the economic status of the subjects is poorer (such as when the family income-to-poverty ratio is close to 0 or 1), the probability of developing diabetes is higher. When the family income-to-poverty ratio is between 1 and 4, the partial dependence values stabilize, and there is no significant fluctuation in diabetes risk. When the poverty ratio exceeds 4, the partial dependence values sharply decrease, suggesting that a higher poverty ratio (better economic status) may be associated with a lower risk of diabetes (Figure 8). On the other hand, Figure 7 shows that PACKYRSMort is non-linearly related to cancer risk. When the cumulative smoking burden is less than 10, the risk of cancer is relatively low. When the cumulative smoking burden is between 10 and 30, the risk of cancer significantly increases. When the cumulative smoking burden exceeds 30, the risk of cancer shows a declining trend.
Epigenetic clocks rely on DNA methylation (DNAm) analysis at specific CpG sites to predict an individual’s chronological age with high accuracy (11). Additionally, epigenetic age acceleration—the discrepancy between chronological age and epigenetic age—is increasingly recognized as a marker of aging, capable of predicting premature onset of diseases and early mortality (12). When applied to large-scale human epidemiological datasets, research has shown that the gap between predicted epigenetic age and actual chronological age is linked to various age-related diseases, particularly when epigenetic age surpasses chronological age. There is growing evidence supporting the notion that accelerated epigenetic aging serves as a novel biomarker for cancer risk. A study by Plonski et al. (13) found that epigenetic age acceleration correlates with late-stage mortality in both cancer and chronic diseases. Similarly, research by Zheng et al. (24) demonstrated a statistically significant positive correlation between epigenetic age acceleration and overall mortality, highlighting its role as a key predictor of colorectal cancer survival. Levine et al. (25) further suggested that accelerated epigenetic aging could serve as a useful biomarker for assessing lung cancer susceptibility. In the present study, univariate analysis revealed that all epigenetic clocks (including HorvathAge, HannumAge, PhenoAge, and others) were significantly elevated in cancer patients compared to non-cancer controls. Heatmap analysis also identified a weak negative correlation (−0.19) between LinAge acceleration and ZhangAge acceleration with cancer risk, suggesting that these two forms of epigenetic age acceleration might be associated with a lower cancer risk. Conversely, VidalBraloAge acceleration and HannumAge acceleration showed weak positive correlations (0.10 and 0.05, respectively) with cancer, indicating that these epigenetic age accelerations may be linked to an increased cancer risk. These findings support the conclusion that epigenetic age acceleration is potentially associated with cancer risk. Consistent with previous studies (14), which have suggested that epigenetic age accelerations, such as Horvath acceleration, Hannum acceleration, and Grim acceleration, can predict cancer mortality, the results reinforce the role of epigenetic age acceleration in cancer outcomes.
In this study, various epigenetic age acceleration markers, including HorvathAge accelerations, HannumAge accelerations, SkinBloodAge accelerations, PhenoAge accelerations, ZhangAge accelerations, and LinAge accelerations, exhibited significant differences in diabetic patients (p < 0.05). However, there was little to no significant independent correlation between diabetes and these accelerations of epigenetic age clocks. Previous research (8) has also shown that epigenetic age is linked to the mortality risk in individuals with type 2 diabetes. Vetter et al. (26) noted that after adjusting for covariates, no significant cross-sectional association was found between epigenetic age acceleration and the diagnosis of diabetes. On the other hand, longitudinal analysis indicated that in male diabetic participants, each additional year of 7-CpG DNAm acceleration at baseline was associated with an 11% increased likelihood of developing new diabetes-related complications or exacerbating pre-existing complications during the follow-up period. A separate longitudinal study conducted among twins (27) found a positive correlation between blood glucose levels and epigenetic age markers. As this study is cross-sectional, it did not perform longitudinal comparisons, suggesting that future research should explore the relationship between epigenetic age and diabetes in more depth through longitudinal studies. Furthermore, due to sample limitations in the database, diabetes was not categorized in this study, highlighting the need for more detailed research with categorized data and larger sample sizes in future investigations.
In this study, epigenetic markers such as GDF15Mort, B2MMort, CystatinCMort, TIMP1Mort, ADMMort, PACKYRSMort, GrimAgeMort, GrimAge2Mort, HorvathTelo, DunedinPoAm, CD4TPP, and NeuPP were significantly higher in cancer patients compared to non-cancer patients (p < 0.05). These biomarkers are involved in key cellular processes, such as cell cycle regulation, inflammatory response, apoptosis, and gene expression regulation, all of which play crucial roles in cancer development and progression. GDF15 promotes tumor cell invasion and metastasis by regulating cell migration and apoptosis (28). High expression of GDF15 in various cancer types is associated with tumor invasiveness and poor prognosis (29). TIMP1 as a matrix metalloproteinase inhibitor, TIMP1 regulates matrix remodeling in the tumor microenvironment and promotes tumor metastasis (30, 31). Elevated levels of TIMP1 are linked to poor prognosis in multiple cancers (32–34).
This study employed machine learning techniques to analyze the relationship between 30 epigenetic biomarkers and the risks of diabetes and cancer, and it visualized the association between the most influential biomarkers and the outcomes. In the diabetes risk assessment model, the top three most important features identified were logA1Mort, the household income to poverty ratio, and marital status. Among these, logA1Mort demonstrated a linear relationship with the onset of diabetes, where its increase was positively correlated with an elevated risk of diabetes. LogA1Mort refers to the log-transformed glycated hemoglobin value derived from DNA methylation (35), and evidence indicates that it is strongly correlated with insulin, triglyceride, and glucose levels, as well as with insulin sensitivity and glucose metabolism (35). Importantly, studies have shown that for each standard deviation increase in logA1Mort, the risk ratio for coronary heart disease rises by approximately 30% (35). These findings suggest that logA1Mort could serve as a valuable biomarker for predicting diabetes, particularly in the context of an aging population. Integrating age with epigenetic biomarkers could facilitate the identification of high-risk groups, enabling earlier interventions. This study is the first to quantify the contribution of logA1Mort in diabetes prediction using machine learning techniques. By incorporating machine learning models alongside 30 epigenetic biomarkers, we were able to more accurately assess the contribution of these biomarkers to diabetes risk, offering a novel approach for early diabetes screening.
In this study, a poorer economic status, as indicated by a household income-to-poverty ratio close to 0 or 1, was associated with a higher likelihood of developing diabetes. When the household income-to-poverty ratio ranged from 1 to 4, the partial dependence values stabilized, showing no significant fluctuations in diabetes risk. However, when the poverty ratio exceeded 4, the partial dependence values sharply decreased, suggesting that a higher poverty ratio (which reflects better economic status) may be linked to a reduced risk of developing diabetes. The household income-to-poverty ratio, as a proxy for socioeconomic status, has been demonstrated in several studies to significantly influence both the incidence and prognosis of diabetes (36). This study further highlights the predictive role of income and poverty ratio in assessing diabetes risk, particularly in low-income groups, where both the incidence and mortality rates of diabetes are higher. Socioeconomic factors can elevate the risk of diabetes by influencing behaviors related to health, access to healthcare, and other factors. Therefore, future diabetes prevention and intervention strategies should take these socioeconomic variables into account, with a particular focus on enhancing early screening and health education for low-income populations.
In this study, marital status is also one of the important features in the diabetes model. Studies have pointed out (37) that for minority men and non-Hispanic white men, divorce/separation status is significantly associated with diabetes mortality, while for minority women and non-Hispanic white women, widowhood status is significantly associated with diabetes mortality. Some studies have shown (38, 39) that the impact of marital status on diabetes mortality differs by gender.
In the cancer risk assessment model, gender, non-Hispanic White ethnicity, and PACKYRSMort were identified as the top three important features. Among these, gender was found to be one of the most significant contributing factors, which aligns with existing literature, underscoring gender as a critical element in cancer risk prediction. The incidence of various cancers shows notable differences between males and females. Several studies have indicated (40) that men generally exhibit a higher incidence of various cancer types compared to women, particularly cancers that are strongly linked to smoking and alcohol consumption, such as lung cancer, esophageal cancer, and metastatic cancers. Due to differences in endocrine functions, immune system responses, and other factors such as physical activity, men tend to have higher susceptibility to these cancers. For instance, in the case of lung cancer (41), men face a significantly higher risk compared to women, especially among smokers. Although smoking rates have decreased among women, the incidence of lung cancer in women has risen in recent years, which may be attributed to factors such as women’s smoking levels, genetic predispositions, and gender-specific differences in smoking behaviors. Conversely, breast cancer incidence is substantially higher in women than in men (42, 43), a difference closely tied to the role of estrogen in the female body. Estrogen promotes the development and lactation of breast tissue, and prolonged exposure to this hormone may increase the risk of developing breast cancer.
There is substantial evidence that habitual smoking is a major risk factor for immune-mediated inflammatory diseases and various cancers (44). Specifically, smoking is a major risk factor for head and neck cancers and lung cancer (45). Approximately 90% of lung cancer cases are directly related to smoking (46). Smoking is also closely linked to bladder cancer, pancreatic cancer, and renal pelvic cancer. Smoking is also associated with the etiology of colon cancer, liver cancer, and stomach cancer (45, 46). Existing data clearly indicate that smoking increases the risk of cancer in multiple organs (47) and often leads to premature death. The five-year survival rate for lung cancer is very low, only 15%. Other smoking-related cancers, such as liver cancer and pancreatic cancer, also have low five-year survival rates (18 and 9%, respectively). PACKYRSMort is a composite biomarker for estimating pack-years of smoking, proposed by Ake T. Lu based on DNA methylation (17). Our study shows that PACKYRSMort has a nonlinear relationship with cancer risk. When the cumulative smoking burden is <10, the risk of cancer is relatively low. When the cumulative smoking burden is between 10 and 30, the risk of cancer significantly increases. When the cumulative smoking burden is >30, the risk of cancer begins to decline. Our results differ from other studies. Rota et al. (48) quantified the dose–response relationship between smoking and gastric cancer risk and found that smoking is not only an independent risk factor for gastric cancer, but the risk increases with the number of cigarettes smoked daily, from a small amount to 20, and the risk increases in a dose-dependent manner with the duration of smoking. A meta-analysis by Lugo et al. showed that the risk of gallbladder cancer increases linearly with smoking intensity and duration (49). In addition, the risk of breast cancer (50) and cervical cancer (51) has also been reported to be linearly associated with smoking intensity. Our study is consistent with previous research when the cumulative smoking burden is ≤30, but when the cumulative smoking burden is >30, cancer risk shows a certain degree of decline. This phenomenon requires further biological explanation. A possible explanation is that the carcinogenic risk brought by long-term smoking has pushed the individual’s health status to the limit, and other health factors (such as immune decline, organ failure, etc.) may begin to significantly affect their cancer risk. Additionally, long-term smoking may alter DNA methylation patterns (52, 53), leading to the suppression or activation of certain gene expressions, thus affecting the mechanisms of cancer development.
In the cancer prediction model, the non-Hispanic White population emerged as a key sociodemographic factor, underscoring the significant role of race and cultural background in cancer risk prediction. Previous studies have shown (54) that, compared to non-Hispanic White individuals, Hispanic men and women experienced 25–30% lower cancer incidence (2014–2018) and mortality rates (2015–2019), with a notably lower incidence of common cancers. This study further substantiates the relevance of this group in the cancer model, highlighting the potential influence of racial differences on cancer susceptibility. These findings not only enhance our understanding of cancer risk prediction but also lay the groundwork for future personalized risk assessments. By integrating epigenetic biomarkers (such as PACKYRSMort) with sociodemographic factors (such as gender and non-Hispanic White ethnicity), we can more accurately identify high-risk populations, particularly in contexts where gender, race, and socioeconomic factors intersect. These results offer both theoretical support for personalized cancer prevention strategies and data-driven insights that can inform public health policy development.
Although this study has made significant progress in exploring the relationship between epigenetic biomarkers and the risk of diabetes and cancer, and has successfully developed relevant predictive models, several limitations remain. These limitations highlight important areas for improvement and provide a clear direction for future research.
Firstly, although this study is based on a large amount of epigenetic data, the data is sourced from a single NHANES database. The types of diabetes and cancer are not further categorized, and the data is geographically limited to North America. Additionally, there are missing data points within the dataset, leading to sample imbalances. While standardization and other balancing techniques were applied during data processing, some bias remains inevitable. Therefore, future validation with external cohorts and additional cohort studies would be helpful for further analysis. Expanding data collection and analysis to include diverse regions, races, and cultural backgrounds would improve the model’s applicability and external validity.
Secondly, this study mainly relies on existing epigenetic clocks and machine learning techniques for risk prediction. While these methods have demonstrated their effectiveness in certain aspects, there is still significant potential for improvement in terms of algorithm complexity and predictive accuracy. For instance, training machine learning models requires large volumes of high-quality data, and challenges such as reducing data bias, addressing data loss, and improving feature selection accuracy remain critical areas for improvement. Additionally, the interpretability of these models is another key concern, particularly in the medical field, where it is essential to understand and explain the model’s predictions for clinical applications. Therefore, future research should place greater emphasis on enhancing the interpretability of these models, with a particular focus on integrating biological contexts to clarify the roles of various epigenetic biomarkers in disease prediction.
Thirdly, while epigenetic biomarkers offer promising new biological indicators for early regulation and risk assessment of diabetes and cancer, the genetic mechanisms involved are highly complex and operate across multiple regulatory levels. Single markers, such as specific DNA methylation sites or epigenetic age clocks, may not fully capture the underlying biological processes. To address this limitation, future research should incorporate multidimensional analyses that combine epigenetic biomarkers with other molecular markers, such as genetic mutations, proteomics, and transcriptomics. This multi-omics approach would provide a more comprehensive understanding of an individual’s aging process and enhance the accuracy of disease risk predictions.
Additionally, as a cross-sectional study, this research is limited in its ability to establish causal relationships and may be influenced by age-related effects or selection bias. To overcome these limitations, future research should consider conducting longitudinal studies to track changes in epigenetic biomarkers over time. This approach would help clarify causal relationships between epigenetic changes and disease development, providing a more dynamic understanding of how these biomarkers contribute to the progression of diseases such as diabetes and cancer.
This study is the first to combine epigenetic age acceleration with risk assessment of diabetes and cancer, and conducted a comprehensive analysis using machine learning models. The results indicate that epigenetic age acceleration is closely associated with cancer risk, but has a weaker relationship with diabetes. Through machine learning methods, the top three contributing features in the diabetes model are logA1Mort, household income-to-poverty ratio, and marital status, with an increase in logA1Mort being positively correlated with diabetes risk. In the cancer model, the top three contributing features are gender, non-Hispanic White ethnicity, and PACKYRSMort, with PACKYRSMort showing a nonlinear relationship with cancer risk.
Publicly available datasets were analyzed in this study. The data can be accessed from the NHANES Database at: https://www.cdc.gov/nchs/nhanes/index.htm.
This study was based on publicly available datasets. Ethical review and approval was not required for the study, in accordance with the local legislation and institutional requirements.
SZ: Conceptualization, Data curation, Formal analysis, Writing – review & editing. JJ: Conceptualization, Data curation, Writing – original draft. BX: Writing – review & editing, Software. QZ: Investigation, Methodology, Validation, Writing – original draft. HM: Data curation, Visualization, Writing – original draft.
The author(s) declare that no financial support was received for the research and/or publication of this article.
We express our gratitude to the participants and staff of the National Health and Nutrition Examination Survey (NHANES) from 2011 to 2018 for their valuable contributions.
The authors declare that the research was conducted in the absence of any commercial or financial relationships that could be construed as a potential conflict of interest.
The authors declare that no Gen AI was used in the creation of this manuscript.
All claims expressed in this article are solely those of the authors and do not necessarily represent those of their affiliated organizations, or those of the publisher, the editors and the reviewers. Any product that may be evaluated in this article, or claim that may be made by its manufacturer, is not guaranteed or endorsed by the publisher.
1. GBD 2021 Diabetes Collaborators. Global, regional, and national burden of diabetes from 1990 to 2021, with projections of prevalence to 2050: a systematic analysis for the global burden of disease study 2021. Lancet. (2023) 402:203–34. doi: 10.1016/S0140-6736(23)01301-6
2. Bray, F, Laversanne, M, Sung, H, Ferlay, J, Siegel, RL, Soerjomataram, I, et al. Global cancer statistics 2022: Globocan estimates of incidence and mortality worldwide for 36 cancers in 185 countries. CA Cancer J Clin. (2024) 74:229–63. doi: 10.3322/caac.21834
3. GBD 2021 Diseases and Injuries Collaborators. Global incidence, prevalence, years lived with disability (Ylds), disability-adjusted life-years (Dalys), and healthy life expectancy (Hale) for 371 diseases and injuries in 204 countries and territories and 811 subnational locations, 1990-2021: a systematic analysis for the global burden of disease study 2021. Lancet. (2024) 403:2133–61. doi: 10.1016/S0140-6736(24)00757-8
4. Huang, Q, Han, L, Liu, Y, Wang, C, Duan, D, Lu, N, et al. Elevation of Ptpn 1 promoter methylation is a significant risk factor of type 2 diabetes in the Chinese population. Exp Ther Med. (2017) 14:2976–82. doi: 10.3892/etm.2017.4924
5. Sheaffer, KL, Elliott, EN, and Kaestner, KH. Dna Hypomethylation contributes to genomic instability and intestinal Cancer initiation. Cancer Prev Res (Phila). (2016) 9:534–46. doi: 10.1158/1940-6207.CAPR-15-0349
6. Sergeev, AV, Loiko, AG, Genatullina, AI, Petrov, AS, Kubareva, EA, Dolinnaya, NG, et al. Crosstalk between G-Quadruplexes and Dnmt3a-mediated methylation of the c-Myc oncogene promoter. Int J Mol Sci. (2023) 25:45. doi: 10.3390/ijms25010045
7. Chu, AY, Tin, A, Schlosser, P, Ko, YA, Qiu, C, Yao, C, et al. Epigenome-wide association studies identify Dna methylation associated with kidney function. Nat Commun. (2017) 8:1286. doi: 10.1038/s41467-017-01297-7
8. Sabbatinelli, J, Giuliani, A, Kwiatkowska, KM, Matacchione, G, Belloni, A, Ramini, D, et al. Dna methylation-derived biological age and long-term mortality risk in subjects with type 2 diabetes. Cardiovasc Diabetol. (2024) 23:250. doi: 10.1186/s12933-024-02351-7
9. Jones, AC, Patki, A, Srinivasasainagendra, V, Hidalgo, BA, Tiwari, HK, Limdi, NA, et al. A methylation risk score for chronic kidney disease: a hypergen study. Sci Rep. (2024) 14:17757. doi: 10.1038/s41598-024-68470-z
10. Morales Berstein, F, Mccartney, DL, Lu, AT, Tsilidis, KK, Bouras, E, Haycock, P, et al. Assessing the causal role of epigenetic clocks in the development of multiple cancers: a Mendelian randomization study. eLife. (2022) 11:11. doi: 10.7554/eLife.75374
11. Lee, HS, and Park, T. The influences of DNA methylation and epigenetic clocks, on metabolic disease, in middle-aged Koreans. Clin Epigenetics. (2020) 12:148. doi: 10.1186/s13148-020-00936-z
12. Gialluisi, A, Santoro, A, Tirozzi, A, Cerletti, C, Donati, MB, de Gaetano, G, et al. Epidemiological and genetic overlap among biological aging clocks: new challenges in biogerontology. Ageing Res Rev. (2021) 72:101502. doi: 10.1016/j.arr.2021.101502
13. Plonski, NM, Chen, C, Dong, Q, Qin, N, Song, N, Parikh, HM, et al. Epigenetic age in peripheral blood among children, adolescent, and adult survivors of childhood cancer. JAMA Netw Open. (2023) 6:e2310325. doi: 10.1001/jamanetworkopen.2023.10325
14. Mendy, A, and Mersha, TB. Epigenetic age acceleration and mortality risk prediction in U.S. adults. medRxiv. [Preprint]. (2024) 2024.08.21.24312373. doi: 10.1101/2024.08.21.24312373
15. Lin, Q, Weidner, CI, Costa, IG, Marioni, RE, Ferreira, MRP, Deary, IJ, et al. DNA methylation levels at individual age-associated CpG sites can be indicative for life expectancy. Aging (Albany NY). (2016) 8:394–401. doi: 10.18632/aging.100908
16. Ofori, EK, Adekena, CN, Boima, V, Asare-Anane, H, Yorke, E, Nyarko, ENY, et al. Serum leptin levels in patients with chronic kidney disease and hypertensive heart disease: an observational cross-sectional study. Health Sci Rep. (2023) 6:e1053. doi: 10.1002/hsr2.1053
17. Lu, AT, Quach, A, Wilson, JG, Reiner, AP, Aviv, A, Raj, K, et al. DNA methylation GrimAge strongly predicts lifespan and healthspan. Aging (Albany NY). (2019) 11:303–27. doi: 10.18632/aging.101684
18. Fujita, Y, Taniguchi, Y, Shinkai, S, Tanaka, M, and Ito, M. Secreted growth differentiation factor 15 as a potential biomarker for mitochondrial dysfunctions in aging and age-related disorders. Geriatr Gerontol Int. (2016) 16:17–29. doi: 10.1111/ggi.12724
19. Han, K, Wang, J, Chu, Y, Liao, Q, Ding, Y, Zheng, D, et al. Deep learning based method for predicting DNA N6-methyladenosine sites. Methods. (2024) 230:91–8. doi: 10.1016/j.ymeth.2024.07.012
20. Baviera-Martineza, C, Martinez-Millana, A, and Lopez-Casanova, FB. Integrating automation, interactive visualization, and unsupervised learning for enhanced diabetes management. Stud Health Technol Inform. (2024) 316:1699–703. doi: 10.3233/SHTI240750
21. Zhou, D, Tian, F, Tian, X, Sun, L, Huang, X, Zhao, F, et al. Diagnostic evaluation of a deep learning model for optical diagnosis of colorectal cancer. Nat Commun. (2020) 11:2961. doi: 10.1038/s41467-020-16777-6
22. Anderson, JP, Parikh, JR, Shenfeld, DK, Ivanov, V, Marks, C, Church, BW, et al. Reverse engineering and evaluation of prediction models for progression to type 2 diabetes: an application of machine learning using electronic health records. J Diabetes Sci Technol. (2015) 10:6–18. doi: 10.1177/1932296815620200
23. Guo, J, He, Q, and Li, Y. Machine learning-based prediction of vitamin D deficiency: NHANES 2001-2018. Front Endocrinol (Lausanne). (2024) 15:1327058. doi: 10.3389/fendo.2024.1327058
24. Zheng, C, Li, L, and Xu, R. Association of epigenetic clock with consensus molecular subtypes and overall survival of colorectal cancer. Cancer Epidemiol Biomarkers Prev. (2019) 28:1720–4. doi: 10.1158/1055-9965.EPI-19-0208
25. Levine, ME, Hosgood, HD, Chen, B, Absher, D, Assimes, T, and Horvath, S. DNA methylation age of blood predicts future onset of lung cancer in the women's health initiative. Aging (Albany NY). (2015) 7:690–700. doi: 10.18632/aging.100809
26. Vetter, VM, Spieker, J, Sommerer, Y, Buchmann, N, Kalies, CH, Regitz-Zagrosek, V, et al. DNA methylation age acceleration is associated with risk of diabetes complications. Commun Med. (2023) 3:21. doi: 10.1038/s43856-023-00250-8
27. Miao, K, Hong, X, Cao, W, Lv, J, Yu, C, Huang, T, et al. Association between epigenetic age and type 2 diabetes mellitus or glycemic traits: a longitudinal twin study. Aging Cell. (2024) 23:e14175. doi: 10.1111/acel.14175
28. Joo, M, Kim, D, Lee, MW, Lee, HJ, and Kim, JM. Gdf15 promotes cell growth, migration, and invasion in gastric cancer by inducing Stat3 activation. Int J Mol Sci. (2023) 24:2925. doi: 10.3390/ijms24032925
29. Siddiqui, JA, Pothuraju, R, Khan, P, Sharma, G, Muniyan, S, Seshacharyulu, P, et al. Pathophysiological role of growth differentiation factor 15 (Gdf15) in obesity, cancer, and cachexia. Cytokine Growth Factor Rev. (2022) 64:71–83. doi: 10.1016/j.cytogfr.2021.11.002
30. Dantas, E, Murthy, A, Ahmed, T, Ahmed, M, Ramsamooj, S, Hurd, MA, et al. Timp1 is an early biomarker for detection and prognosis of lung cancer. Clin Transl Med. (2023) 13:e1391. doi: 10.1002/ctm2.1391
31. Tian, Z, Ou, G, Su, M, Li, R, Pan, L, Lin, X, et al. Timp1 derived from pancreatic cancer cells stimulates Schwann cells and promotes the occurrence of perineural invasion. Cancer Lett. (2022) 546:215863. doi: 10.1016/j.canlet.2022.215863
32. Guccini, I, Revandkar, A, D'ambrosio, M, Colucci, M, Pasquini, E, Mosole, S, et al. Senescence reprogramming by Timp1 deficiency promotes prostate cancer metastasis. Cancer Cell. (2021) 39:68–82.e9. doi: 10.1016/j.ccell.2020.10.012
33. Lin, X, Zhao, R, Bin, Y, Huo, R, Xue, G, and Wu, J. Timp1 promotes thyroid cancer cell progression through macrophage phenotypic polarization via the Pi3K/Akt signaling pathway. Genomics. (2024) 116:110914. doi: 10.1016/j.ygeno.2024.110914
34. Yang, L, Jiang, Q, Li, DZ, Zhou, X, Yu, DS, and Zhong, J. Timp1 mRNA in tumor-educated platelets is diagnostic biomarker for colorectal cancer. Aging (Albany NY). (2019) 11:8998–9012. doi: 10.18632/aging.102366
35. Lu, AT, Binder, AM, Zhang, J, Yan, Q, Reiner, AP, Cox, SR, et al. DNA methylation GrimAge version 2. Aging (Albany NY). (2022) 14:9484–549. doi: 10.18632/aging.204434
36. Larkin, HD. AAP recommends immediate, intensive treatment for child obesity. JAMA. (2023) 329:364. doi: 10.1001/jama.2022.24498
37. Kposowa, AJ, Aly Ezzat, D, and Breault, K. Diabetes mellitus and marital status: evidence from the national longitudinal mortality study on the effect of marital dissolution and the death of a spouse. Int J Gen Med. (2021) 14:1881–8. doi: 10.2147/IJGM.S307436
38. Escolar-Pujolar, A, Córdoba Doña, JA, Goicolea Julían, I, Rodríguez, GJ, Santos Sánchez, V, Mayoral Sánchez, E, et al. The effect of marital status on social and gender inequalities in diabetes mortality in Andalusia. Endocrinol Diabetes Nutr (Engl Ed). (2018) 65:21–9. doi: 10.1016/j.endinu.2017.10.006
39. Ramezankhani, A, Azizi, F, and Hadaegh, F. Associations of marital status with diabetes, hypertension, cardiovascular disease and all-cause mortality: a long term follow-up study. PLoS One. (2019) 14:e0215593. doi: 10.1371/journal.pone.0215593
40. Haupt, S, Caramia, F, Klein, SL, Rubin, JB, and Haupt, Y. Sex disparities matter in cancer development and therapy. Nat Rev Cancer. (2021) 21:393–407. doi: 10.1038/s41568-021-00348-y
41. Siegfried, JM. Sex and gender differences in lung cancer and chronic obstructive lung disease. Endocrinology. (2022) 163:bqab254. doi: 10.1210/endocr/bqab254
42. Bhardwaj, PV, Gupta, S, Elyash, A, and Teplinsky, E. Male breast cancer: a review on diagnosis, treatment, and survivorship. Curr Oncol Rep. (2024) 26:34–45. doi: 10.1007/s11912-023-01489-z
43. Coelingh Bennink, HJT, Egberts, JFM, Mol, JA, Roes, KCB, and van Diest, PJ. Breast cancer and major deviations of genetic and gender-related structures and function. J Clin Endocrinol Metab. (2020) 105:e3065–74. doi: 10.1210/clinem/dgaa404
44. Caliri, AW, Tommasi, S, and Besaratinia, A. Relationships among smoking, oxidative stress, inflammation, macromolecular damage, and cancer. Mutat Res Rev Mutat Res. (2021) 787:108365. doi: 10.1016/j.mrrev.2021.108365
45. National Center for Chronic Disease Prevention and Health Promotion (US) Office on Smoking and Health. The health consequences of smoking—50 years of progress: a report of the surgeon general. Atlanta (GA): Centers for Disease Control and Prevention (US) (2014).
46. Viale, PH. The American Cancer Society's facts & figures: 2020 edition. J Adv Pract Oncol. (2020) 11:135–6. doi: 10.6004/jadpro.2020.11.2.1
47. Larsson, SC, and Burgess, S. Appraising the causal role of smoking in multiple diseases: a systematic review and meta-analysis of Mendelian randomization studies. EBioMedicine. (2022) 82:104154. doi: 10.1016/j.ebiom.2022.104154
48. Didovyk, A, and Tsimring, LS. Synthetic gene circuits learn to classify. Cell Syst. (2017) 4:151–3. doi: 10.1016/j.cels.2017.02.001
49. Lugo, A, Peveri, G, and Gallus, S. Should we consider gallbladder cancer a new smoking-related cancer? A comprehensive meta-analysis focused on dose-response relationships. Int J Cancer. (2020) 146:3304–11. doi: 10.1002/ijc.32681
50. Scala, M, Bosetti, C, Bagnardi, V, Possenti, I, Specchia, C, Gallus, S, et al. Dose-response relationships between cigarette smoking and breast cancer risk: a systematic review and meta-analysis. J Epidemiol. (2023) 33:640–8. doi: 10.2188/jea.JE20220206
51. Malevolti, MC, Lugo, A, Scala, M, Gallus, S, Gorini, G, Lachi, A, et al. Dose-risk relationships between cigarette smoking and cervical cancer: a systematic review and meta-analysis. Eur J Cancer Prev. (2023) 32:171–83. doi: 10.1097/CEJ.0000000000000773
52. Zeilinger, S, Kühnel, B, Klopp, N, Baurecht, H, Kleinschmidt, A, Gieger, C, et al. Tobacco smoking leads to extensive genome-wide changes in DNA methylation. PLoS One. (2013) 8:e63812. doi: 10.1371/journal.pone.0063812
53. Su, D, Wang, X, Campbell, MR, Porter, DK, Pittman, GS, Bennett, BD, et al. Distinct epigenetic effects of tobacco smoking in whole blood and among leukocyte subtypes. PLoS One. (2016) 11:e0166486. doi: 10.1371/journal.pone.0166486
Keywords: epigenetic biomarkers, epigenetic clocks, epigenetic age acceleration, diabetes, cancer, machine learning
Citation: Zhang S, Jin J, Xu B, Zheng Q and Mou H (2025) The relationship between epigenetic biomarkers and the risk of diabetes and cancer: a machine learning modeling approach. Front. Public Health. 13:1509458. doi: 10.3389/fpubh.2025.1509458
Received: 11 October 2024; Accepted: 24 February 2025;
Published: 21 March 2025.
Edited by:
Lai Xuefeng, Huazhong University of Science and Technology, ChinaReviewed by:
Giovanni Fiorito, IRCCS Giannina Gaslini Institute, ItalyCopyright © 2025 Zhang, Jin, Xu, Zheng and Mou. This is an open-access article distributed under the terms of the Creative Commons Attribution License (CC BY). The use, distribution or reproduction in other forums is permitted, provided the original author(s) and the copyright owner(s) are credited and that the original publication in this journal is cited, in accordance with accepted academic practice. No use, distribution or reproduction is permitted which does not comply with these terms.
*Correspondence: Jianan Jin, eHp1Z2ZibWFwZkBvdXRsb29rLmNvbQ==; Haibo Mou, aGFpYm8ubW91QHNodWxhbi5jb20=
†These authors have contributed equally to this work and share first authorship
Disclaimer: All claims expressed in this article are solely those of the authors and do not necessarily represent those of their affiliated organizations, or those of the publisher, the editors and the reviewers. Any product that may be evaluated in this article or claim that may be made by its manufacturer is not guaranteed or endorsed by the publisher.
Research integrity at Frontiers
Learn more about the work of our research integrity team to safeguard the quality of each article we publish.