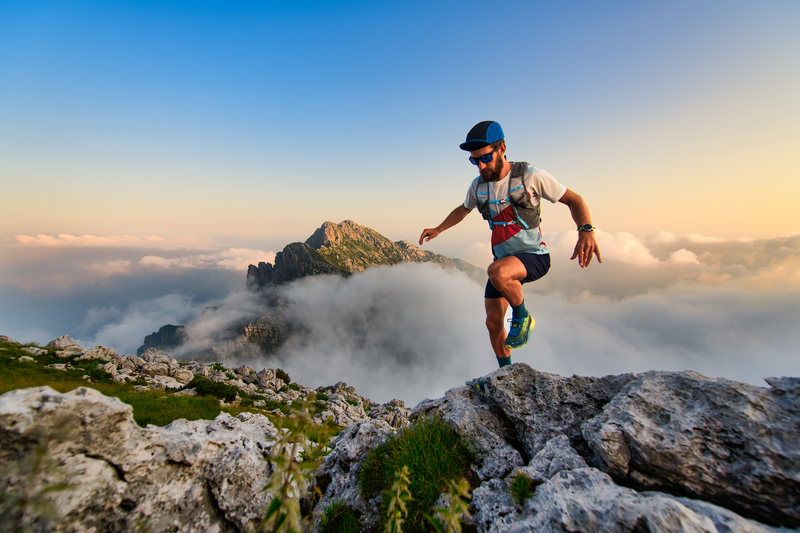
94% of researchers rate our articles as excellent or good
Learn more about the work of our research integrity team to safeguard the quality of each article we publish.
Find out more
ORIGINAL RESEARCH article
Front. Public Health
Sec. Digital Public Health
Volume 13 - 2025 | doi: 10.3389/fpubh.2025.1479095
This article is part of the Research Topic Extracting Insights from Digital Public Health Data using Artificial Intelligence, Volume III View all 10 articles
The final, formatted version of the article will be published soon.
You have multiple emails registered with Frontiers:
Please enter your email address:
If you already have an account, please login
You don't have a Frontiers account ? You can register here
Ovarian Cancer (OC) is one of the leading cancers with a significant impact on women. Despite recent advances in the medical field, such as surgeries, chemotherapy, and radiotherapy interventions, there are only marginal improvements in diagnosis using clinical parameters, as the symptoms at the early stages are very nonspecific. Due to advances in computational algorithms, such as ensemble machine learning, it is now possible to identify complex patterns in clinical parameters. However, these complex patterns do not provide deeper insights into prediction and diagnoses. Explainable Artificial Intelligence models, such as LIME and SHAPLEY Kernels, can provide insights into the decisionmaking process of ensemble models, thus increasing their applicability. The main aim of this research work is to design a computer-aided diagnostic system that accurately classifies and detects ovarian cancer. To achieve this objective, a 3-stage ensemble model and a game-theoretic approach based on SHAPLEY values were built to evaluate and visualize the results, thus analyzing the important features responsible for the prediction. The results demonstrate the efficacy of the proposed model with an accuracy of 98.66%. The proposed model's consistency and advantages are compared with single classifiers. The SHAPLEY values of the proposed model are validated by conventional statistical methods such as the p-test and Cohen's d-test to highlight the efficacy of the proposed method. To further validate the ranking of the features, we compared the p-values and Cohen's d-values of the top five and bottom five features. Proposing and validating an AI-based OC detection, diagnosis, and prognosis method on multi-modal real-life data, which mimics the move of a clinician approach with a demonstration of high performance, is the contribution of this study. The proposed strategy can lead to reliable, accurate, and consistent AI solutions for the detection and management of OC with higher patient experience and outcome at low cost, low morbidity, and low mortality; it can help millions of women living in resource-constrained and challenging economies.
Keywords: Interpretable AI, Ensemble models, bagging, boosting, machine learning, p-value, Cohen's, Shap
Received: 11 Aug 2024; Accepted: 11 Feb 2025.
Copyright: © 2025 Kodipalli, Devi, Guruvare and Ismail. This is an open-access article distributed under the terms of the Creative Commons Attribution License (CC BY). The use, distribution or reproduction in other forums is permitted, provided the original author(s) or licensor are credited and that the original publication in this journal is cited, in accordance with accepted academic practice. No use, distribution or reproduction is permitted which does not comply with these terms.
* Correspondence:
Ashwini Kodipalli, Indian Institute of Science (IISc), Bangalore, India
Shyamala Guruvare, Department of Obstetrics & Gynaecology, Kasturba Medical College, Manipal, India
Disclaimer: All claims expressed in this article are solely those of the authors and do not necessarily represent those of their affiliated organizations, or those of the publisher, the editors and the reviewers. Any product that may be evaluated in this article or claim that may be made by its manufacturer is not guaranteed or endorsed by the publisher.
Research integrity at Frontiers
Learn more about the work of our research integrity team to safeguard the quality of each article we publish.