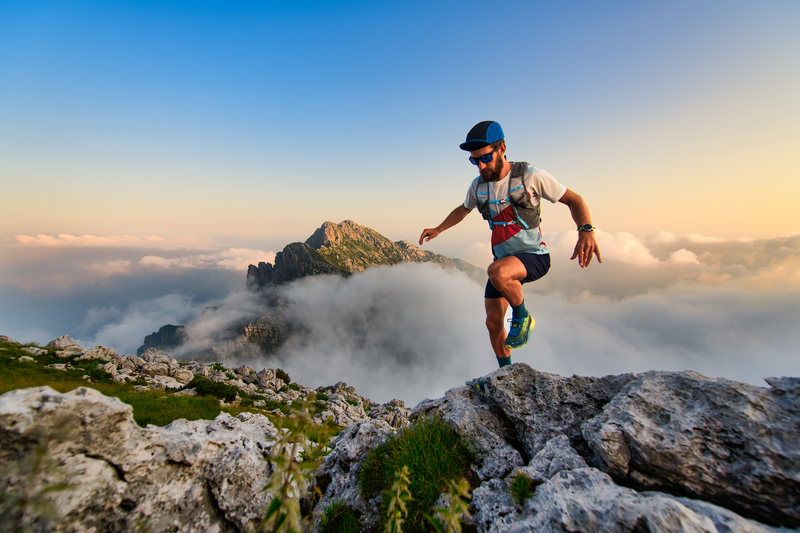
95% of researchers rate our articles as excellent or good
Learn more about the work of our research integrity team to safeguard the quality of each article we publish.
Find out more
SYSTEMATIC REVIEW article
Front. Public Health , 26 January 2023
Sec. Digital Public Health
Volume 11 - 2023 | https://doi.org/10.3389/fpubh.2023.1044059
This article is part of the Research Topic Perspectives in Digital Health and Big Data in Medicine: Current Trends, Professional Challenges, and Ethical, Legal, and Social Implications View all 24 articles
Background and objective: Hypoglycemia is a key barrier to achieving optimal glycemic control in people with diabetes, which has been proven to cause a set of deleterious outcomes, such as impaired cognition, increased cardiovascular disease, and mortality. Hypoglycemia prediction has come to play a role in diabetes management as big data analysis and machine learning (ML) approaches have become increasingly prevalent in recent years. As a result, a review is needed to summarize the existing prediction algorithms and models to guide better clinical practice in hypoglycemia prevention.
Materials and methods: PubMed, EMBASE, and the Cochrane Library were searched for relevant studies published between 1 January 2015 and 8 December 2022. Five hypoglycemia prediction aspects were covered: real-time hypoglycemia, mild and severe hypoglycemia, nocturnal hypoglycemia, inpatient hypoglycemia, and other hypoglycemia (postprandial, exercise-related).
Results: From the 5,042 records retrieved, we included 79 studies in our analysis. Two major categories of prediction models are identified by an overview of the chosen studies: simple or logistic regression models based on clinical data and data-based ML models (continuous glucose monitoring data is most commonly used). Models utilizing clinical data have identified a variety of risk factors that can lead to hypoglycemic events. Data-driven models based on various techniques such as neural networks, autoregressive, ensemble learning, supervised learning, and mathematical formulas have also revealed suggestive features in cases of hypoglycemia prediction.
Conclusion: In this study, we looked deep into the currently established hypoglycemia prediction models and identified hypoglycemia risk factors from various perspectives, which may provide readers with a better understanding of future trends in this topic.
Diabetes mellitus is a chronic disease characterized by high blood glucose caused by the inability to produce or effectively use insulin. Maintaining blood glucose within the normal range may help prevent or delay the development of diabetic microvascular or macrovascular complications (1–4). However, intensive glycemic control increases the frequency of hypoglycemia while decreasing the risk of long-term complications (5). Hypoglycemia is usually defined as a blood glucose concentration of < 70 mg/dL. Counterregulatory response and impaired cognitive function are two physical changes brought on by hypoglycemia (6–8). In addition to impaired behavioral functions such as fear of hypoglycemia (9), depression (10), and dyskinesia (11, 12), hypoglycemia can also result in fatal cardiovascular events (13).
Clinically, hypoglycemia can be classified as mild (MH) or severe (SH) depending on whether third-party assistance is required during hypoglycemia episodes or if there is a loss of consciousness (14). The frequencies of MH and SH episodes in patients with type 1 diabetes (T1D) have been estimated to be 1.6 and 0.029 episodes per person-week, respectively (15), and the incidence of SH was approximately 0.44 episodes per person-year in insulin-treated patients with type 2 diabetes (T2D) (16). Furthermore, hypoglycemia is more common in patients with diabetes who are hospitalized (17, 18). SH is extremely dangerous and is more likely to occur in patients on long-term insulin therapy (5). The counterregulatory response is critical in preventing MH from progressing to SH. However, in individuals with recurrent hypoglycemia, the frequency of hypoglycemic warning symptoms tends to decrease gradually, leading to impaired awareness of hypoglycemia (IAH) and the occurrence of asymptomatic hypoglycemia (19, 20), and IAH, in turn, increases the risk of SH (21, 22), eventually forming a vicious cycle.
Clinical attention is also given to nocturnal hypoglycemia, exercise-related hypoglycemia, and inpatient hypoglycemia. In T1D patients known as “fragile diabetes”, nocturnal hypoglycemia (NH) accounted for 55% of hypoglycemia when a blood glucose concentration < 54 mg/dL (23–25), and this proportion increased to 75% in pediatric patients (26). Patients may be unable to detect episodes of NH in time while sleeping, which may predispose them to IAH in the long run or even result in “dead-in-bed syndrome”. Aside from the benefits of physical activity, such as improving cardiopulmonary adaptability, blood lipid levels, and lowering the risk of long-term cardiovascular events in patients with diabetes (27–29), it may also cause post-exercise hypoglycemia due to increased insulin sensitivity in the short term (30), resulting in avoidance of exercise due to fear of hypoglycemia. Furthermore, symptoms of early hypoglycemia in T1D patients may be masked by physical activity, which may increase the frequency of SH in this population (31). The frequent occurrence of iatrogenic hypoglycemia in hospitalized patients is also not negligible. A systematic review has shown that intensive glucose control increases the risk of inpatient hypoglycemia (32). It was estimated that patients hospitalized for diabetes or hyperglycemia experience an average of two hypoglycemic events per week, with the majority occurring during the night (33). Worse still, inpatient hypoglycemia was associated with a variety of negative outcomes, including increased mortality and serious cardiovascular events (13).
Hypoglycemia prediction is crucial in clinical practice. Many studies have emerged in the last decade that used conventional approaches based on physiological and clinical parameters to predict hypoglycemia (34). These approaches were typically trained using retrospectively collected demographic data, laboratory test results, glucose-lowering agents, and other indicators that may be associated with hypoglycemia obtained from electronic health records in a certain period (35, 36). And the most commonly used statistics were linear regression, logistic regression, Cox hazards regression, and other operations tailored to the problem at hand.
Machine learning (ML) advances, on the other hand, and the need for more accurate hypoglycemia prediction has resulted in data-driven predictive models. Benefiting from the rapid development of continuous glucose monitoring (CGM), the risk of hypoglycemia could be simply predicted by specific parameter calculations such as low blood glucose index (LBGI) (37, 38). Complex CGM-based hypoglycemia predictions were used to develop and improve the artificial pancreas (AP) algorithm, which alerts users when blood glucose levels drop or hypoglycemia is imminent (39). Data-driven algorithms and ML models improve hypoglycemia prediction (40). CGM glucose data are frequently used for ML model establishment due to their continuity and bulkiness.
According to the prediction horizon (PH), such models can be divided into short-term (< 180 min), mid-term (180 min to 24 h), and long-term (several days, months, or even years). Undoubtedly, the prediction efficiency of CGM-based models would decrease with the extension of PH. Since glucose autocorrelation usually disappears after 30 min (41), and 30 min is the minimum time interval for effective patient intervention to prevent accidents, current CGM-based hypoglycemia prediction models set PH at 30 min or above. In brief, current hypoglycemia prediction models are mostly based on clinical parameters, CGM data, or a combination of both. The predictive accuracy varied with the study population, outcome definition, PH definition, modeling technique and model training and validation approaches (42). As for clinical data-based models, large sample size and sufficient data processing are frequently required to ensure the accuracy and reliability. Such models typically collect clinical hypoglycemic events for risk stratifying and further hypoglycemia-associated feature selection, followed by internal or external validation of model generalizability. The PH of such models tends to be broad, ranging from predicting short-term hypoglycemic events like inpatient hypoglycemia to long-term hypoglycemic events like SH events months later. It is important to note that a shorter PH may be more useful for prompt clinician intervention, whereas a larger PH increases the prevalence of an outcome and consequently model performance, but may be less useful as a decision support tool (42).
Felizardo et al. (34) conducted a systematic review of data-based algorithms and models in blood glucose prediction, which could be considered an extension of the work of Oviedo et al. (43). They only looked at ML approaches to prediction, and the relevant literature was from before June 2020. Nonetheless, no study comparing conventional and data-based hypoglycemia risk prediction has been discussed and compared from a more holistic clinical perspective to our knowledge. In this study, we investigated the currently established hypoglycemia prediction models and identified hypoglycemia risk factors from various perspectives, which may provide patients and clinicians with a better understanding of future trends in this topic. Our review will be discussed from three perspectives: (1) an overview of currently established hypoglycemia prediction models; (2) dividing the selected studies into five hypoglycemia prediction parts: real-time hypoglycemia, MH/SH, NH, inpatient hypoglycemia, and other hypoglycemia (postprandial, exercise-related), explored hypoglycemia risk factors and illustrated prediction approaches from clinical and ML perspectives, respectively; (3) a comprehensive evaluation and comparison of current hypoglycemia prediction models, with clinical implications and insights into future trends.
We searched the PubMed, EMBASE, and Cochrane Library databases for relevant literature from 1 January 2015 to 8 December 2022. The search keywords were “diabetes”, “hypoglycemia prediction”, “hypoglycemia warning”, “hypoglycemia detection” and “hypoglycemia estimation”. A total of 5,042 records were found, with 61 of them being relevant to our topic. In addition, we manually added 18 records related to the included records after full-text reading and reference tracking. Figure 1 depicts the flow diagram of detailed literature inclusion.
To include as many relevant records as possible to increase the reliability of our review, we included the records which met the following conditions: (1) the study population were patients with abnormal glucose tolerance or diagnosed with diabetes, regardless of the age of the patients and the type of diabetes; (2) the original data used for the analysis of factors related to hypoglycemia in the records and the establishment of predictive algorithms or models were the patient basic data, medication regimen, laboratory test results and blood glucose measurement results (self-monitoring of blood glucose [SMBG] or CGM results) or other indices retrieved from the real-world studies, clinical trials or cohort studies; (3) the main results of the record were detailed and were exact correlations, algorithms or models related to hypoglycemia prediction. We excluded the following types of records: (1) the research topic was the algorithm proposal or improvement of hypoglycemia warning in AP or CGM products; (2) NCT protocols; (3) abstract/short papers; (4) reviews; (5) blood glucose concentration predictions only but not hypoglycemic events; (6) comments; (7) in silico study.
Data were extracted from the full text and supplementary information of eligible records according to pre-established literature classification criteria. For each study, the following data were carefully extracted: first author, year of publication, use of database features (study population, sample size, type of input clinical data for modeling, source of input glucose data for modeling, modeling approach), type of hypoglycemia prediction (real-time hypoglycemia, MH/SH, NH, inpatient hypoglycemia, other hypoglycemia [postprandial, exercise-related]). Then, the algorithms or models used, the performance, as well as the significance of model performance metrics were tabulated in detail according to the type of hypoglycemia prediction.
1) An overview of the currently established prediction model of hypoglycemia;
2) divided the selected studies into five hypoglycemia prediction parts: real-time hypoglycemia, MH/SH, NH, inpatient hypoglycemia, and other hypoglycemia (postprandial, exercise-related), explored the risk factors of hypoglycemia and illustrated prediction approaches from the perspective of clinical and machine learning, respectively;
3) a comprehensive evaluation and comparison of current hypoglycemia prediction models, extraction of clinical implications, and insights into the future trends in this topic.
Of the 5,042 records obtained after the initial search, 220 records remained after the primary screening of titles and abstracts and were assessed for eligibility, and 159 records were excluded after full-text review, as follows: (1) 69 records were related to the validation of algorithms in the AP or insulin pump; (2) 20 records were without full text; (3) 15 records were protocols for randomized controlled trials; (4) 22 records were reviews; (5) 28 records only predicted blood glucose concentrations but not hypoglycemic events; (6) three records were comment, and (7) two records were in silico studies (Figure 1). Furthermore, we added 18 relevant studies through reference tracking. As a result, the total number of studies included was 79: 15 studies on real-time hypoglycemia prediction (19.0%), 24 studies on MH/SH prediction (30.4%), 13 studies on NH prediction (16.5%), 17 studies on inpatient hypoglycemia prediction (21.5%), two studies on exercise-related hypoglycemia prediction (2.5%), and three studies on postprandial hypoglycemia prediction (3.8%), three studies on real-time and NH prediction (3.8%), 1 study on real-time and inpatient hypoglycemia prediction (1.3%) and 1 study on real-time, NH and postprandial hypoglycemia prediction (1.3%) (Figure 2). Details of all included articles are summarized in Table 1.
Patients with T1D and T2D made up the majority of the participants in the chosen studies, with 31 of them (39.2%) only including T1D patients. The sample sizes of among studies varied significantly: Six studies with a maximum of 10 participants (7.6%), 22 studies with 11 to 100 participants (27.8%), 19 studies with 101 to 1,000 participants (24.1%), 16 studies with 1,001–10,000 participants (20.3%), 13 studies with 10,001–100,000 participants (16.5%) and two studies more than 100,000 participants (2.5%) (Figure 3). The study subjects were from all age groups.
One of the most important strategies for successful hypoglycemia prediction is the selection of appropriate inputs. Thirty-eight of the 79 included studies (48.1%) used CGM data or CGM-derived indices to predict hypoglycemia. Demographics information, insulin use, laboratory tests results, comorbidities, and a history of hypoglycemia were all widely included in clinical hypoglycemia prediction models. Furthermore, other blood glucose-related factors such as carbohydrate intake or meal information (13/79, 16.5%) and physical activity or exercise (7/79, 8.9%) were also included. In addition to the aforementioned factors, 12 studies (15.2%) used physiological parameters such as heart rate, near-infrared light, skin impedance, skin temperature, sweating, and sleep to develop hypoglycemia prediction models (Table 2, Figure 4).
PH is the time period that the model has to forecast the outcome in the future. Prediction windows ranging from 15 min to hours, days, or even months have been reported. It is natural to anticipate a decrease in prediction power as the PH increases due to the limited number of available confounding factors in the data used to train the model. A shorter PH may be more useful for prompt clinician intervention, whereas a larger PH increases the prevalence of an outcome and consequently model performance, but may be less useful as a decision support tool (42). An increase in PH, on the other hand, improves clinical usability of prediction services by extending the time required to take the necessary action during a critical situation, but at the expense of clinical accuracy. According to the aforementioned PH division, 21 studies were short-term forecasting (26.6%), 21 studies were mid-term forecasting (26.6%) and 19 studies were long-term forecasting (24.1%).
Various classes of ML techniques have been used in general dynamic system modeling, regression, and prediction services. However, for hypoglycemia prediction in the present study, logistic regression (LR) were the most used techniques (28/79, 35.4%), as shown in Figure 5. Random forest (RF), one of the tree-based models, was the second most used approach (14/79, 17.7%). Support vector machines (SVM) was the third most used algorithm (10/79, 12.7%). Autoregressive and neural networks in various forms ranked as the fourth most used techniques (8/79, 10.1%). XGBoost and support vector regression (SVR) ranked as the fifth (6/79, 7.6%) and sixth most used technique (5/79, 6.3%). Long-short-term memory (LSTM) (4/79, 5.1%), naïve Bayes (3/79, 3.8%), Adaboost (2/79, 2.5%), k-nearest neighbors (k-nn) (2.5%) and other techniques were also used in hypoglycemia prediction.
Model validation is crucial for algorithm development and performance estimation. External validation is important since internal validation may overestimate model performance on a future cohort of patients (121). There are 12 studies utilizing external validation in the present study (15.2%). Internal validation strategies include re-substitution validation, hold-out validation, k-fold cross-validation, leave-one-out cross-validation, and repeated k-fold cross-validation (122). The most commonly used strategies in the reviewed articles are various forms of k-fold cross-validation (22/79, 27.8%). The K-fold cross-validation strategy involves randomly partitioning the datasets into k equal subsets and using one set as a validation set and the rest for training, repeating the process for each subset. Moreover, random subsampling, bootstrapping, hold-out validation, leave-one-out validation approaches are also used.
As model evaluation performance measures, the majority of studies used sensitivity, specificity, and area under the curve (AUC). For prediction models based on clinical data, correlation coefficients and c-statistics were also frequently used. In addition, accuracy, positive predictive value (PPV) and false positive rate (FPR) were used as evaluation parameters. Each metric was defined in Supplementary Table S1.
The CGM system can detect the glucose concentration in the interstitial fluid continuously and comprehensively, laying the groundwork for modern glucose monitoring and the emergence of AP. A CGM system, an insulin pump, and a dosing algorithm comprise AP (123). Algorithms are used to implement individual-based accurate blood glucose prediction. The proposal and improvement of dosing algorithms have gradually evolved into a bottleneck of AP development with the increasing maturity of CGM systems. Accurate prediction of impending hypoglycemia is difficult due to large intra- and inter-subject variability, as well as numerous exogenous factors such as diet, exercise, hypoglycemic drugs, and mood changes that can affect blood glucose levels (124). Following a review of the literature, it was discovered that the current impending hypoglycemia prediction primarily includes physiological models, data-based models, and hybrid models (43). Simply put, the development of physiological models is dependent on an understanding of glucose metabolism in the body. These models, which are often compartment models, simulate glucose metabolism and can be used to study glucose-regulated physiological processes. Data-driven models, on the other hand, rely primarily on CGM data and occasionally on additional signals to simulate the patient's physiological response without involving physiological variables. Hybrid models typically combine a physiological model with a data-driven model. Such models incorporate dietary information and insulin absorption via physiological models, as well as massive CGM data, to improve the overall prediction accuracy of this model.
This section presents the most recent research on data-based and hybrid hypoglycemia prediction models that only use CGM data as inputs (78, 79, 90, 102, 104–106). AR models were commonly used. Yang et al. (78) proposed an AR integrated moving average (ARIMA) model with an adaptive recognition algorithm. After training with CGM data from 100 subjects (50 T1D + 50 T2D), it was discovered that this model had 100.0% sensitivity in predicting hypoglycemic events, a 9.4% FPR, and an early alert with an average 25.5 min treatment time window to avoid hypoglycemia deterioration. Gadaleta et al. (79) used CGM data from 89 T1D patients to compare current common ML models (static and dynamic) and discovered that the SVR model performed best in terms of prediction accuracy as well as the speed of hypoglycemia detection, with sensitivity and PPV of 75.0 and 51.0%, respectively. Another study comparing linear and nonlinear models laterally found that at a PH of 30 min, the individualized ARIMA model outperformed all linear models in terms of hypoglycemic event detection and prediction accuracy (105). Furthermore, Marcus et al. (90) used a novel patient-specific supervised machine learning (SML) model for hypoglycemia prediction after 30 min and discovered that when the best-fit model was selected for each patient, the hypoglycemia sensitivity was 64.0%, and FPR was 4.0%. Even when only CGM glucose data below 70 mg/dL were included, similar results were obtained. Li (102) and Yu et al. (104) used the model of change detection method and the Winsorization method in conjunction with the autoregressive moving average (ARMA) model and the recursive least squares (RLS) method, respectively. The sensitivity of the former was 95.72%, and the sensitivities of the latter were 85.90, 80.36, and 78.07% when the threshold of hypoglycemia was set at 54, 70, and 79 mg/dL, respectively. Wenbo et al. (106) achieved 94.80% sensitivity for hypoglycemic event prediction at a PH of 60 min using the variational mode decomposition (VMD)-kernel extreme learning machine (KELM)-AdaBoost algorithm. As for sensitivity, the authors of (78) achieved the highest sensitivity at a PH of 30 min (Table 3).
Table 3. Machine learning approaches for real-time hypoglycemia prediction and best results performed.
In addition to using CGM data and insulin to predict, carbohydrate intake was also used in models (Table 3). Dave et al. (103) confirmed the benefits of including blood glucose-affecting physiological factors in the model by demonstrating satisfactory sensitivity and specificity in hypoglycemia prediction. A recent study indicated a promising predictive efficacy of physiologically-based kinetic model (PKM) which integrated CGM data, carbohydrate intake and insulin usage (120). Additionally, one of the significant factors affecting blood glucose is physical activity (exercise) (125). However, the effect of physical activity (exercise) on blood glucose varies considerably based on many factors, such as the type of activity, amount and intensity of activity, and duration. When compared to studies that only used CGM data as inputs, the contribution of features (meal, insulin, and exercise) other than CGM glucose data is lower but not insignificant; their significance rises for predictions with PH of 60 min (103). Hence, adding clinical factors other than CGM data may allow for improved predictive efficacy, even though overall model performance had not improved to a satisfactory degree.
Aside from CGM data, some researchers have also used non-invasive methods to predict hypoglycemia. Cichosz et al. (47, 68) used CGM and electrocardiograph (ECG) data to predict short-term hypoglycemia events, achieving an AUC of more than 0.96 and a sensitivity of 100% when the PH was set at 20 min. However, the sensitivity and specificity of another NN model for predicting hypoglycemia based solely on ECG signals were only 78.0 and 60.0%, respectively, which could be explained in part by the limited glucose data used in modeling (53). Furthermore, Elvebakk et al. (69) collected physiological parameters such as ECG signals, near-infrared light (NIR), and skin impedance from 20 T1D patients with IAH undergoing hypoglycemic clamp, and they demonstrated that a probability model based on the physiological signals described above could identify 88% of hypoglycemia. Similarly, Tronstad et al. (75) compared the accuracy of partial least squares (PLS) and artificial NN (ANN) in predicting hypoglycemia using NIR signals, skin impedance, and skin temperature data from the same patient source. Their findings showed that the ANN model that combined NIR, skin temperature, and skin impedance outperformed. However, in general, NIR and bioimpedance-based hypoglycemia detection was not very accurate, but it could provide blood glucose trends to some extent.
In summary, current studies aimed at predicting real-time hypoglycemia were mostly short-term. As the PH increased, the prediction accuracy inevitably decreased. Hence, accurate long-term hypoglycemia prediction is extremely urgent. Furthermore, hypoglycemia prediction is gradually shifting from a single signal to a combination of signals to more accurately simulate real-life glucose changes. As shown in Table 3, there was no single technique that could be identified as the most popular model in terms of algorithms. The ML approaches trend revealed that researchers were still experimenting with a diverse set of ML techniques. It can be concluded that there have been some promising results in the field of real-time hypoglycemia prediction, but there is still much room for advancement.
Prior to the booming of ML in hypoglycemia prediction, simple predictions based on massive clinical data emerged in this field of study (Table 4). Long duration of diabetes, alcohol consumption, eating disorders, low BMI, insulin use, low LDL levels, combined diabetic retinopathy (DR) or diabetic peripheral neuropathy (DPN), depression or dementia, great glycemic variability, infection, and heart failure were identified as risk factors for overall hypoglycemic events (44–46, 49, 51, 63, 96). A long-term SH risk prediction study based on clinical parameters in 1,676, 885 adult T2D patients found that old age, female gender, smoking, alcohol abuse, low BMI, lack of exercise, history of SH, use of insulin or multiple oral hypoglycemic agents, combined hypertension and chronic kidney disease (CKD), long duration of diabetes, and a high Charlson comorbidity index were all important risk factors for the development of SH (64). Several studies have shown an association between insulin use, intensive insulin therapy, previous history of SH, and SH (44, 48, 51, 62, 91). Furthermore, black race, sulfonylurea use, low HbA1c, low serum creatinine levels, and poor cognitive function were also identified as risk factors for SH (44, 91). Hu et al. (84) performed ROC analysis on fasting insulin, fasting blood glucose, and total insulin treatment time of 257 T2D patients receiving intensive therapy and reported an AUC of 0.666 in hypoglycemia prediction. Karter et al. (57) classified 165,148 patients into high, medium, and low-risk groups for SH hospitalization by assessing six factors: number of previous hypoglycemia hospitalizations, insulin use, sulfonylurea use, previous emergency history, CKD stage, and age. Following model validation, they concluded that the proposed SH risk assessment tool was accurate and effective after 12 months of observation. Accordingly, some researchers have used another 6-parameter model (age, type of diabetes, HbA1c, eGFR and previous history of hypoglycemia) to predict the 6-month SH risk (58).
A remarkable cohort study of 27,841 T1D patients followed for an average of 7 years to model T1D health outcomes revealed that male gender, Ln (HbA1c), HDL level, and smoking were risk factors for hypoglycemia (HR = 1.32, 1.63, 1.14, and 1.40, respectively), while Ln (eGFR) was a protective factor (HR = 0.77) (94). Another SH predictive model study based on electronic health record data of 47, 280 T2D patients found that a history of previous hypoglycemia (HR = 4.44), black race (HR = 1.81), Medicaid insurance (HR = 1.35), previous history of cardiovascular disease (HR = 2.35), depression (HR = 1.28), mental disorder other than depression (HR = 1.55), alcohol consumption (HR = 1.55), and CKD (HR = 1.86) were risk factors for SH, while the relationship between sulfonylureas and SH changed with HbA1c: sulfonylurea use was a risk factor (HR = 1.61) when HbA1c was 6%, but it became a protective factor (HR = 0.69) when HbA1c was 9%. Furthermore, the effect of HbA1c levels on SH varied at some extreme values: when the reference HbA1c was 6%, the corresponding HR was 1.59 when an HbA1c level of 5%, however, HR of HbA1c changed to 0.73 when at a level of 7%, and the HR for HbA1c = 9% was 1.39 instead (92).
In addition to clinical models, there were a number of studies that used ML approaches to predict mild and severe hypoglycemia. Ma et al. (89), for example, proposed a model to predict SH that could handle missing data, and they finally included 48 risk factors associated with SH such as demographic data (age, ethnicity, education information), vital signs (diastolic blood pressure, DBP), laboratory test results (creatinine, eGFR, urine protein, UACR) and medication regimens (diuretics, potassium supplements, ACEI, α-blockers, β-blockers, anticoagulants, sulfonylureas, biguanides, thiazolidinediones, insulin, etc.) after univariate analysis for relevant variable screening and showed that the average c-statistic of their proposed SH prediction model was 0.77. Predictive modeling also made use of mathematical approaches. Samuel et al. (50) identified four clinical factors associated with MH (BMI, diabetes duration, HbA1c, and GFR) and proposed a mathematical formula for calculating the incidence of MH incorporating these four parameters, but its accuracy was highly uncertain in different populations of patients with diabetes. However, the prediction efficiency of ML was not always superior to that of traditional statistical methods. Li et al. (71) used electronic health records data from 38,780 patients with diabetes to create a prediction model for hypoglycemia and discovered that the ML method (RF) was only 1% more effective than LR in predicting hypoglycemia. A robust recursive partitioning algorithm based SH-related emergency department (ED) use prediction model was proposed based on a large sample size and external validation (57) (Table 5).
Age between 10.0 and 19.9 years, diagnosis of T1D, and initiation of insulin therapy were found to be risk factors for NH events (< 54 mg/dL, 00:00–05:59) in a study using self-monitoring of blood glucose (SMBG) values and clinical indicators from 8,190 patients with diabetes (100). For NH prediction, CGM parameters had also been adopted in addition to clinical parameters. Daytime (6:00-22:59) mean absolute glucose (MAG) and mean pre-midnight blood glucose levels had predictive value for NH events, according to a cross-sectional study of 83 insulin-treated hospitalized T2D patients (56). Sakurai et al. (61) developed an equation relating age, SMBG values, and basal insulin dose to predict NH. Besides, strenuous physical activity was found to be an important predictor of NH after adjusting for age and gender (70) (Table 6).
ML approaches based on CGM data were widely used for NH prediction in addition to clinical factors (Table 7). Tkachenko (55) and Sampath et al. (54) proposed combining predictive risk factors of NH based on CGM data, which both resulted in improved predictive performance. Based on massive nocturnal CGM raw glucose data derived from 9,800 T1D patients, a random forest (RF) model demonstrated an overall NH predictive performance of AUC up to 0.84 (AUC = 0.90 during 00:00–03:00, and AUC = 0.75 during 03:00–06:00) (76). Furthermore, a novel CGM metric-gradient and combining mean sensor glucose enabled the prediction of NH events in patients with diabetes with a sensitivity of 92.05% and a false positive rate of 7.69% (88).
Models that included blood glucose-affecting physiological factors other than CGM data were also widely used in the field of NH prediction. Jensen et al. (85), for example, performed ML feature extraction and ROC curve analysis on basic demographic data, dietary intake, and insulin use combined with CGM data from 463 T1D patients and discovered that the combined extracted CGM indices (linear regression slope of blood glucose during 21:00–24:00, lowest blood glucose value, lowest blood glucose value on the previous night) and BMI could achieve an AUC of 0.79 for NH prediction (sensitivity: 75%, specificity: 70%). Furthermore, Bertachi et al. (82) confirmed the importance of combining physical activity with CGM data to predict NH events: the specificity of predicting NH was significantly improved to 91.9% when additional information such as heart rate, number of steps, calorie expenditure, and sleep were added. Calhoun et al. (97) discovered an association between NH and bedtime BG, exercise intensity, daytime hypoglycemia, HbA1c, and active insulin (insulin on board, IOB). Vehi et al. (95) established an ANN model that included CGM data, exercise and sleep information from six T1D patients and had a 44.0% sensitivity and an 85.9% specificity in predicting NH. Another study of NH prediction using a SVR model based on CGM data found that blood glucose values at bedtime, age, and mean blood glucose 1 h before bedtime were related to the occurrence of NH, and the AUC of this model to finally predict NH events was 0.86 (sensitivity: 94.1%, specificity: 72.0%) (93).
It can be seen that the most popular approaches for NH prediction in our reviewed articles were RF and SVM. The RF model including CGM, demographics data, INS and other clinical indicators of 406 T1D patients established by Berikov et al. (112) achieved a better performance at a PH of 30 min. Whereas a SVR model taking CGM, insulin use and carbohydrate intake information into consideration showed satisfactory AUC (0.86) and sensitivity (94.1%) for a longer prediction window (93).
Seventy-five percent of hypoglycemic events associated with coma or seizures occur at night, as warning autonomic symptoms caused by hypoglycemia are frequently insufficient to awaken the patient (126). Since NH events are urgent and harmful to patients, current NH prevention focuses primarily on accurately predicting upcoming NH events and alerting using modern glucose monitoring technology and AP to urge medical staff or patients to take prompt action. The findings of our literature review also highlight recent significant advances in the field of NH prediction using ML, however, these algorithms and models must still be validated in a large sample and tested in real-world applications in the future.
A recent electronic health record-based risk prediction study for iatrogenic hypoglycemic events included 35,147 hospitalized patients (mean age, 66 years) who received at least 1 U of insulin and completed four finger stick records (35). Demographic data, diagnostic information, inpatient procedure, laboratory test results, finger blood results, and therapeutic drugs were among the 43 types of data extracted from electronic health records. They revealed that basal insulin dose, CV of finger stick blood values, previous hypoglycemic events, nadir glucose value, body weight, and mean blood glucose in the first 24 h of hospitalization were the most important predictors of hypoglycemia. The c-statistic was 0.90 of internal validation and was 0.86–0.88 of external validation (35). Stuart et al. (59) used multivariate LR to develop a prediction model from hospital admissions of 9,584 patients with diabetes, finding that ethnicity (black and Asian), older age (≥75 years), type of admission (emergency), insulin and sulfonylurea use could all predict the occurrence of hypoglycemia in hospitalized patients. A study of 9,665 patients with diabetes using modeling and validation revealed that older age, nasogastric tube or gastrostomy tube feeding, a higher Charlson comorbidity index, admission for vomiting, combined acute renal failure, and insulin use were risk factors for inpatient hypoglycemia (98). In addition to age and insulin, emergency department history in the previous 6 months, oral hypoglycemic agent use without inducing hypoglycemia, and severe CKD were all associated with inpatient hypoglycemia (74). Furthermore, a study of 21,840 adult patients with diabetes found that male patients were more prone to hypoglycemia on the first day of hospitalization, while glucose variability, low body weight, low creatinine clearance, insulin and sulfonylurea use were common risk factors for hypoglycemia (66). Frail patients treated with insulin or with insufficient nocturnal glucose monitoring were predisposed to inpatient hypoglycemia (81). Low serum albumin levels, in addition to the aforementioned risk factors, were also identified (86, 87) (Table 8).
The use of ML approaches in inpatient hypoglycemia prediction was summarized in Table 9. It can be seen that the most popular approaches for inpatient hypoglycemia prediction in our reviewed articles were LR and XGBoost. A study that used ML to mine electronic health records data from 17,658 patients with diabetes revealed the superiority of ML: when compared to the traditional LR method (AUC = 0.75), the AUC of the XGBoost model could reach 0.96 in identifying hypoglycemic events (36). However, considering of the sample size and model validation, the study carried out by Mathioudakis et al. (35) using stochastic gradient boosting (SGB) achieved the best performance in a narrow PH (24 h after each glucose measurement).
Table 9. Machine learning approaches for inpatient hypoglycemia prediction and best results performed.
Prediction of postprandial and exercise-related hypoglycemia was also included in our review. Oviedo et al. (73) used a SVM algorithm to predict postprandial hypoglycemia within 240 minutes after meal in 10 T1D patients receiving sensor-augmented pump (SAP) therapy, and they found that the sensitivity and specificity for prediction of mild hypoglycemia < 70 mg/dL were 71.0 and 79.0%, respectively, and those for severe hypoglycemia < 54 mg/dL were 77.0 and 81.0%, respectively. Seo et al. (80) compared four ML models and discovered that the RF model had 89.6% sensitivity and 91.3% specificity in predicting hypoglycemic events after 30 min of meal absorption (Table 10).
Meanwhile, our research focused on exercise-related hypoglycemia. Prevention of hypoglycemia during exercise is a major challenge in diabetes. Providing predictions of glycemic changes during and following exercise can help people with diabetes avoid hypoglycemia. Reddy et al. (77) pointed out the most important features in the RF model for exercise-related hypoglycemia prediction: an average heart rate above 121 beats/min in the first 5 min of exercise, an increase in energy expenditure and a blood glucose value below 182 mg/dL at the beginning of exercise tended to increase the likelihood of hypoglycemia. Another unique dataset representing 320 days and 50,000 + time points of glycemic measurements collecting in adults with T1D who participated in a 4-arm crossover study evaluating insulin-pump therapies was used to develop adaptive, personalized ML algorithms to predict exercise-related glucose changes (115). Their personalized algorithms based on LR algorithm achieved high accuracy (84%) and specificity (90%) in predicting hypoglycemia during and following 4-h exercise sessions.
Hypoglycemia is a huge obstacle to achieving optimal glucose control in patients with diabetes, posing a great challenge to the healthcare system while potentially harming the patient's cardiovascular system and brain. Prediction of hypoglycemia is critical in clinical practice. In this study, we comprehensively reviewed the literature on hypoglycemic risk factors or hypoglycemic prediction that have been published in recent years and elaborated on the research progress in the prediction of various types of hypoglycemic events that are most concerned in clinical practice from various perspectives. Before the explosion of machine learning for hypoglycemia prediction, simple prediction based on large sample-sized clinical data proliferated due to clinical need over the last decade. We concluded the summary table of risk factors for different types of hypoglycemic events by extracting hypoglycemic risk factors from various studies. As a result, we discovered that age, insulin dose, sulfonylurea use, prior history of hypoglycemia, and combined CKD were the main risk factors for hypoglycemic episodes in clinical practice, but that under certain conditions, advanced age or younger age, different levels of HbA1c, and sulfonylurea use had different effects on hypoglycemia. This necessitates clinicians to assess the patient's condition before making an accurate judgment and decision.
The implementation of CGM systems, insulin pumps, and AP in clinical practice provides technical support for the prevention of hypoglycemic events. With the booming field of ML, hypoglycemic prediction models incorporating several other major factors affecting blood glucose such as insulin dose, carbohydrate intake, and physical activity have sprung up based on the massive amount of blood glucose information carried by CGM. However, the PH of current studies is < 240 min. Furthermore, the predictive performance of hypoglycemia varied with sample size, training data type, and ML method used. Furthermore, any predictive model and prevention strategy must be validated in a variety of clinical settings to determine whether there will be subsequent hypoglycemia reductions and improvements in clinical outcomes. In conclusion, while there have been some promising results in the field of hypoglycemia prediction, there is still much room for improvement.
Hypoglycemia in patients with diabetes is influenced by multiple factors, such as insulin administration, carbohydrate intake, physical activity, previous blood glucose readings, stress, age, BMI, duration of diabetes, pancreatic islet function, comorbidities, alcohol consumption, and smoking. To more accurately estimate the risk of hypoglycemia, an ideal predictive model for hypoglycemia should consider all pertinent confounding factors jointly. In this review, demographics data and CGM readings were the main types of model inputs used. CGM glucose values, for instance, were the most frequently used feature when performing real-time and nocturnal hypoglycemia predictions. Systems using ML can be trained to forecast future hypoglycemic events based on a person's historical glucose levels. The majority of these models used time series prediction techniques with glucose data that contains precise timestamps corresponding to real glucose values. Additionally, some models also considered the effects of insulin, carbohydrate intake, and physical activity on hypoglycemia. In our study, patients manually recorded their dietary information using paper diaries or electronic diaries. This information included the frequency and timing of each meal and was typically estimated as carbohydrate (grams). Nevertheless, some researchers have attempted to automate the process of dietary data recording. In most cases, ML model integration was done directly using carbohydrate amounts (in grams), figuring out how many calories were in food, or using compartmental models to predict how much glucose is absorbed from the gut into the blood. Besides, the type, quantity, and intensity of physical activity, as well as its duration, all affect hypoglycemia differently. Data on physical activity can be gathered manually or automatically by wearable devices. A wide range of physical activity data, including the intensity of activity, the total energy expended over a specific period of time, a standard table of caloric use during exercise, task metabolic equivalent (MET) were taken into consideration. According to the current results, the most useful features are still the information carried by CGM, such as the original blood glucose value and hypoglycemia-related CGM parameters. When compared to studies that only used CGM data as inputs, the contribution of features (meal, insulin, and exercise) other than CGM glucose data was lower but not insignificant. In contrast to real-time hypoglycemia prediction models, which primarily relied on CGM blood glucose readings, MH/SH and inpatient hypoglycemia predictions were primarily based on the occurrence of hypoglycemic events collected from clinical datasets, and the included parameters were mostly demographics, insulin use, HbA1c or fasting blood glucose levels and previous hypoglycemic events. For such models, the most useful characteristics were those that were clinically closely related to the occurrence of hypoglycemia such as age, insulin, BMI, renal function, previous hypoglycemic events, and comorbidities.
The most effective algorithm in this area is still up for debate, despite the fact that many ML techniques have been widely used for hypoglycemia prediction over the past 10 years. As was already mentioned, the study population, PH, outcome definitions, modeling techniques, and model validation strategies all affect the model performance. Accordingly, it is essential to make sure that all conditions are comparable in order to accurately determine which algorithm outperforms for forecasting a particular hypoglycemic event. The study population of almost every study, according to the literature we reviewed for this study, is unique. Additionally, the inputs used for model development vary from study to study, which makes the horizontal comparison even more challenging. Although comparing sample size and use of external validation under the same PH and outcome under a specific hypoglycemia prediction scenario can yield a relatively well-behaved algorithm, this comparison is partially empirical and lacks direct comparisons of the performance metrics. In light of the present findings of this review, it is challenging to directly compare algorithms.
With the availability of large amounts of clinical data and growing awareness of big data analysis tools, more and more accurate hypoglycemia prediction models can be developed and tested. Future research should concentrate on the discovery of novel algorithms or models to develop more medical devices or decision support systems to prevent various types of hypoglycemic events and other adverse outcomes. Clinical trials will be required before application to assess the economic efficacy and long-term benefits to patients with diabetes.
The generation of automated and continuous personal data has become possible with the proliferation of commercially available CGM, wearable, and other glucose collection devices for self-monitoring, opening up opportunities for better training ML models with more detailed data. Although integrating CGM with clinical data may improve model performance, widespread implementation of CGM devices in patients with diabetes remains an unsolved problem due to financial and human factor considerations (staff training, time, resources, and other physiological measurement tools that most patients do not have). Furthermore, despite increased research on ML-based prediction models for hypoglycemia over the last decade, achieving a generic model with accurate predictive efficacy under real-world conditions remains difficult due to the complexity of blood glucose dynamics. From the literature reviewed in this paper, most of the study samples came from retrospective datasets and were internally validated only. Thus, there are still great uncertainties in the model's accuracy and generalizability. Although advanced ML methods have been used to address these issues, the majority have yet to be invoked and tested in real-world situations. Future prospective external validation studies are urgently needed to confirm whether these models improve glycemic outcomes.
The original contributions presented in the study are included in the article/Supplementary material, further inquiries can be directed to the corresponding author.
ZZ and LY conceived and designed the analysis. LZ collected the data, performed the analysis, and wrote the paper. All authors contributed to the article and approved the submitted version.
This work was supported by the National Key R&D Program of China (2018YFC2001005).
The authors declare that the research was conducted in the absence of any commercial or financial relationships that could be construed as a potential conflict of interest.
All claims expressed in this article are solely those of the authors and do not necessarily represent those of their affiliated organizations, or those of the publisher, the editors and the reviewers. Any product that may be evaluated in this article, or claim that may be made by its manufacturer, is not guaranteed or endorsed by the publisher.
The Supplementary Material for this article can be found online at: https://www.frontiersin.org/articles/10.3389/fpubh.2023.1044059/full#supplementary-material
1. Diabetes C, Complications Trial Research G, Nathan DM, Genuth S, Lachin J, Cleary P, et al. The effect of intensive treatment of diabetes on the development and progression of long-term complications in insulin-dependent diabetes mellitus. N Engl J Med. (1993) 329:977–86. doi: 10.1056/NEJM199309303291401
2. Diabetes C. Complications Trial/Epidemiology of Diabetes I, Complications Research G, Lachin JM, Genuth S, Cleary P, et al. Retinopathy and nephropathy in patients with type 1 diabetes four years after a trial of intensive therapy. N Engl J Med. (2000) 342:381–9. doi: 10.1056/NEJM200002103420603
3. Holman RR, Paul SK, Bethel MA, Matthews DR, Neil HA. 10-year follow-up of intensive glucose control in type 2 diabetes. N Engl J Med. (2008) 359:1577–89. doi: 10.1056/NEJMoa0806470
4. Polak JF, Backlund JY, Cleary PA, Harrington AP, O'Leary DH, Lachin JM, et al. Progression of carotid artery intima-media thickness during 12 years in the diabetes control and complications trial/epidemiology of diabetes interventions and complications (Dcct/Edic) study. Diabetes. (2011) 60:607–13. doi: 10.2337/db10-0296
5. Shaefer C, Hinnen D, Sadler C. Hypoglycemia and diabetes: increased need for awareness. Curr Med Res Opin. (2016) 32:1479–86. doi: 10.1185/03007995.2016.1163255
6. Amiel SA, Gale E. Physiological responses to hypoglycemia. Counterregulation and cognitive function. Diabetes care. (1993) 16 Suppl 3:48–55. doi: 10.2337/diacare.16.3.48
7. Flatt AJS, Little SA, Speight J, Leelarathna L, Walkinshaw E, Tan HK, et al. Predictors of recurrent severe hypoglycemia in adults with type 1 diabetes and impaired awareness of hypoglycemia during the hypocompass study. Diabetes Care. (2020) 43:44–52. doi: 10.2337/dc19-0630
8. Graveling AJ, Deary IJ, Frier BM. Acute hypoglycemia impairs executive cognitive function in adults with and without type 1 diabetes. Diabetes Care. (2013) 36:3240–6. doi: 10.2337/dc13-0194
9. Nefs G, Bevelander S, Hendrieckx C, Bot M, Ruige J, Speight J, et al. Fear of hypoglycaemia in adults with type 1 diabetes: results from diabetes miles–the Netherlands. Diabetic Med. (2015) 32:1289–96. doi: 10.1111/dme.12739
10. Kikuchi Y, Iwase M, Fujii H, Ohkuma T, Kaizu S, Ide H, et al. Association of severe hypoglycemia with depressive symptoms in patients with type 2 diabetes: the Fukuoka diabetes registry. BMJ Open Diabetes Res care. (2015) 3:e000063. doi: 10.1136/bmjdrc-2014-000063
11. Kelly D, Hamilton JK, Riddell MC. Blood glucose levels and performance in a sports camp for adolescents with type 1 diabetes mellitus: a field study. Int J Pediatr. (2010) 2010:216167. doi: 10.1155/2010/216167
12. Sherin A, Peeyush KT, Naijil G, Chinthu R, Paulose CS. Hypoglycemia induced behavioural deficit and decreased gaba receptor, creb expression in the cerebellum of streptozoticin induced diabetic rats. Brain Res Bull. (2010) 83:360–6. doi: 10.1016/j.brainresbull.2010.09.004
13. Yakubovich N, Gerstein HC. Serious cardiovascular outcomes in diabetes: the role of hypoglycemia. Circulation. (2011) 123:342–8. doi: 10.1161/CIRCULATIONAHA.110.948489
14. Seaquist ER, Anderson J, Childs B, Cryer P, Dagogo-Jack S, Fish L, et al. Hypoglycemia and diabetes: a report of a workgroup of the american diabetes association and the endocrine society. Diabetes Care. (2013) 36:1384–95. doi: 10.2337/dc12-2480
15. Pramming S, Thorsteinsson B, Bendtson I, Binder C. Symptomatic hypoglycaemia in 411 Type 1 Diabetic Patients. Diabetic Med. (1991) 8:217–22. doi: 10.1111/j.1464-5491.1991.tb01575.x
16. Akram K, Pedersen-Bjergaard U, Carstensen B, Borch-Johnsen K, Thorsteinsson B. Frequency and risk factors of severe hypoglycaemia in insulin-treated type 2 diabetes: a cross-sectional survey. Diabetic Med. (2006) 23:750–6. doi: 10.1111/j.1464-5491.2006.01880.x
17. Turchin A, Matheny ME, Shubina M, Scanlon JV, Greenwood B, Pendergrass ML. Hypoglycemia and clinical outcomes in patients with diabetes hospitalized in the general ward. Diabetes Care. (2009) 32:1153–7. doi: 10.2337/dc08-2127
18. Ulmer BJ, Kara A, Mariash CN. Temporal occurrences and recurrence patterns of hypoglycemia during hospitalization. Endocr Pract. (2015) 21:501–7. doi: 10.4158/EP14355.OR
19. Dagogo-Jack SE, Craft S, Cryer PE. Hypoglycemia-associated autonomic failure in insulin-dependent diabetes mellitus. recent antecedent hypoglycemia reduces autonomic responses to, symptoms of, and defense against subsequent hypoglycemia. J Clin Investig. (1993) 91:819–28. doi: 10.1172/JCI116302
20. Veneman T, Mitrakou A, Mokan M, Cryer P, Gerich J. Induction of hypoglycemia unawareness by asymptomatic nocturnal hypoglycemia. Diabetes. (1993) 42:1233–7. doi: 10.2337/diab.42.9.1233
21. Pedersen-Bjergaard U, Pramming S, Heller SR, Wallace TM, Rasmussen AK, Jorgensen HV, et al. Severe hypoglycaemia in 1076 adult patients with type 1 diabetes: influence of risk markers and selection. Diabetes Metab Res Rev. (2004) 20:479–86. doi: 10.1002/dmrr.482
22. Braak EW, Appelman AM. van de Laak M, Stolk RP, van Haeften TW, Erkelens DW. Clinical characteristics of type 1 diabetic patients with and without severe hypoglycemia. Diabetes Care. (2000) 23:1467–71. doi: 10.2337/diacare.23.10.1467
23. Epidemiology of Severe Hypoglycemia in the Diabetes Control and Complications Trial. The Dcct Research Group. Am J Med. (1991) 90:450–9. doi: 10.1016/0002-9343(91)80085-Z
24. Nathan DM, Cleary PA, Backlund JY, Genuth SM, Lachin JM, Orchard TJ, et al. Intensive diabetes treatment and cardiovascular disease in patients with type 1 diabetes. N Engl J Med. (2005) 353:2643–53. doi: 10.1056/NEJMoa052187
25. Nathan DM, Group DER. The diabetes control and complications trial/epidemiology of diabetes interventions and complications study at 30 years: overview. Diabetes Care. (2014) 37:9–16. doi: 10.2337/dc13-2112
26. Davis EA, Keating B, Byrne GC, Russell M, Jones TW. Hypoglycemia: incidence and clinical predictors in a large population-based sample of children and adolescents with Iddm. Diabetes Care. (1997) 20:22–5. doi: 10.2337/diacare.20.1.22
27. Lumb A. Diabetes and exercise. Clin Med (Lond). (2014) 14:673–6. doi: 10.7861/clinmedicine.14-6-673
28. McCarthy MM, Funk M, Grey M. Cardiovascular health in adults with type 1 diabetes. Prev Med. (2016) 91:138–43. doi: 10.1016/j.ypmed.2016.08.019
29. Tikkanen-Dolenc H, Waden J, Forsblom C, Harjutsalo V, Thorn LM, Saraheimo M, et al. Frequent and intensive physical activity reduces risk of cardiovascular events in type 1 diabetes. Diabetologia. (2017) 60:574–80. doi: 10.1007/s00125-016-4189-8
30. Riddell MC, Gallen IW, Smart CE, Taplin CE, Adolfsson P, Lumb AN, et al. Exercise management in type 1 diabetes: a consensus statement. Lancet Diab Endocrinol. (2017) 5:377–90. doi: 10.1016/S2213-8587(17)30014-1
31. Younk LM, Mikeladze M, Tate D, Davis SN. Exercise-related hypoglycemia in diabetes mellitus. Expert Rev Endocrinol Metab. (2011) 6:93–108. doi: 10.1586/eem.10.78
32. Murad MH, Coburn JA, Coto-Yglesias F, Dzyubak S, Hazem A, Lane MA, et al. Glycemic control in non-critically ill hospitalized patients: a systematic review and meta-analysis. J Clin Endocrinol Metab. (2012) 97:49–58. doi: 10.1210/jc.2011-2100
33. Bailon RM, Cook CB, Hovan MJ, Hull BP, Seifert KM, Miller-Cage V, et al. Temporal and geographic patterns of hypoglycemia among hospitalized patients with diabetes mellitus. J Diabetes Sci Technol. (2009) 3:261–8. doi: 10.1177/193229680900300206
34. Felizardo V, Garcia NM, Pombo N, Megdiche I. Data-based algorithms and models using diabetics real data for blood glucose and hypoglycaemia prediction - a systematic literature review. Artif Intell Med. (2021) 118:102120. doi: 10.1016/j.artmed.2021.102120
35. Mathioudakis NN, Abusamaan MS, Shakarchi AF, Sokolinsky S, Fayzullin S, McGready J, et al. Development and validation of a machine learning model to predict near-term risk of iatrogenic hypoglycemia in hospitalized patients. JAMA Network Open. (2021) 4:e2030913. doi: 10.1001/jamanetworkopen.2020.30913
36. Ruan Y, Bellot A, Moysova Z, Tan GD, Lumb A, Davies J, et al. Predicting the risk of inpatient hypoglycemia with machine learning using electronic health records. Diabetes Care. (2020) 43:1504–11. doi: 10.2337/dc19-1743
37. Crenier L, Abou-Elias C, Corvilain B. Glucose variability assessed by low blood glucose index is predictive of hypoglycemic events in patients with type 1 diabetes switched to pump therapy. Diabetes Care. (2013) 36:2148–53. doi: 10.2337/dc12-2058
38. Kovatchev BP, Cox DJ, Gonder-Frederick LA, Young-Hyman D, Schlundt D, Clarke W. Assessment of risk for severe hypoglycemia among adults with iddm: validation of the low blood glucose index. Diabetes Care. (1998) 21:1870–5. doi: 10.2337/diacare.21.11.1870
39. Boughton CK, Hovorka R. Advances in artificial pancreas systems. Sci Transl Med. (2019) 11:484. doi: 10.1126/scitranslmed.aaw4949
40. Mujahid O, Contreras I, Vehi J. Machine learning techniques for hypoglycemia prediction: trends and challenges. Sensors (Basel, Switzerland). (2021) 21:2. doi: 10.3390/s21020546
41. Kovatchev B, Clarke W. Peculiarities of the continuous glucose monitoring data stream and their impact on developing closed-loop control technology. J Diabetes Sci Technol. (2008) 2:158–63. doi: 10.1177/193229680800200125
42. Zale A, Mathioudakis N. Machine learning models for inpatient glucose prediction. Curr Diab Rep. (2022) 22:353–64. doi: 10.1007/s11892-022-01477-w
43. Oviedo S, Vehi J, Calm R, Armengol J. A review of personalized blood glucose prediction strategies for T1dm patients. Int J Numer Method Biomed Eng. (2017) 33:6. doi: 10.1002/cnm.2833
44. Investigators OT. Predictors of nonsevere and severe hypoglycemia during glucose-lowering treatment with insulin glargine or standard drugs in the origin trial. Diabetes Care. (2015) 38:22–8. doi: 10.2337/dc14-1329
45. Bordier L, Buysschaert M, Bauduceau B, Doucet J, Verny C, Lassmann Vague V, et al. Predicting factors of hypoglycaemia in elderly type 2 diabetes patients: contributions of the gerodiab study. Diabetes Metab. (2015) 41:301–3. doi: 10.1016/j.diabet.2015.03.001
46. Cariou B, Fontaine P, Eschwege E, Lievre M, Gouet D, Huet D, et al. Frequency and predictors of confirmed hypoglycaemia in type 1 and insulin-treated type 2 diabetes mellitus patients in a real-life setting: results from the dialog study. Diabetes Metab. (2015) 41:116–25. doi: 10.1016/j.diabet.2014.10.007
47. Cichosz SL, Frystyk J, Tarnow L, Fleischer J. Combining information of autonomic modulation and Cgm measurements enables prediction and improves detection of spontaneous hypoglycemic events. J Diabetes Sci Technol. (2015) 9:132–7. doi: 10.1177/1932296814549830
48. Ganz ML Li Q, Wintfeld NS, Lee YC, Sorli C, Huang JC. The dynamic relationship between current and previous severe hypoglycemic events: a lagged dependent variable analysis among patients with type 2 diabetes who have initiated basal insulin. Curr Med Res Opin. (2015) 31:1809–15. doi: 10.1185/03007995.2015.1074891
49. Inzucchi SE, Umpierrez G, DiGenio A, Zhou R, Kovatchev B. How well do glucose variability measures predict patient glycaemic outcomes during treatment intensification in type 2 diabetes? Diabetes Res Clin Pract. (2015) 108:179–86. doi: 10.1016/j.diabres.2014.12.020
50. Samuel S, Goswami D, Shum K, Boye KS, Rengarajan B, Curtis B, et al. A model of mild hypoglycemia. Curr Med Res Opin. (2015) 31:633–41. doi: 10.1185/03007995.2015.1020363
51. Sonoda N, Morimoto A, Ugi S, Morino K, Sekine O, Nemoto K, et al. Predictors for mild and severe hypoglycemia in insulin-treated japanese diabetic patients. PLoS ONE. (2015) 10:e0130584. doi: 10.1371/journal.pone.0130584
52. Sudharsan B, Peeples M, Shomali M. Hypoglycemia prediction using machine learning models for patients with type 2 diabetes. J Diabetes Sci Technol. (2015) 9:86–90. doi: 10.1177/1932296814554260
53. Ling SH, San PP, Nguyen HT. Non-invasive hypoglycemia monitoring system using extreme learning machine for type 1 diabetes. ISA Trans. (2016) 64:440–6. doi: 10.1016/j.isatra.2016.05.008
54. Sampath S, Tkachenko P, Renard E, Pereverzev SV. Glycemic control indices and their aggregation in the prediction of nocturnal hypoglycemia from intermittent blood glucose measurements. J Diabetes Sci Technol. (2016) 10:1245–50. doi: 10.1177/1932296816670400
55. Tkachenko P, Kriukova G, Aleksandrova M, Chertov O, Renard E, Pereverzyev SV. Prediction of nocturnal hypoglycemia by an aggregation of previously known prediction approaches: proof of concept for clinical application. Comput Methods Programs Biomed. (2016) 134:179–86. doi: 10.1016/j.cmpb.2016.07.003
56. Klimontov VV, Myakina NE. Glucose variability indices predict the episodes of nocturnal hypoglycemia in elderly type 2 diabetic patients treated with insulin. Diabetes Metab Syndr. (2017) 11:119–24. doi: 10.1016/j.dsx.2016.08.023
57. Karter AJ, Warton EM, Lipska KJ, Ralston JD, Moffet HH, Jackson GG, et al. Development and validation of a tool to identify patients with type 2 diabetes at high risk of hypoglycemia-related emergency department or hospital use. JAMA Intern Med. (2017) 177:1461–70. doi: 10.1001/jamainternmed.2017.3844
58. Schroeder EB, Xu S, Goodrich GK, Nichols GA, O'Connor PJ, Steiner JF. Predicting the 6-month risk of severe hypoglycemia among adults with diabetes: development and external validation of a prediction model. J Diabetes Complications. (2017) 31:1158–63. doi: 10.1016/j.jdiacomp.2017.04.004
59. Stuart K, Adderley NJ, Marshall T, Rayman G, Sitch A, Manley S, et al. Predicting inpatient hypoglycaemia in hospitalized patients with diabetes: a retrospective analysis of 9584 admissions with diabetes. Diabetic Med. (2017) 34:1385–91. doi: 10.1111/dme.13409
60. Ena J, Gaviria AZ, Romero-Sanchez M, Carretero-Gomez J, Carrasco-Sanchez FJ, Segura-Heras JV, et al. Derivation and validation model for hospital hypoglycemia. Eur J Intern Med. (2018) 47:43–8. doi: 10.1016/j.ejim.2017.08.024
61. Sakurai K, Kawai Y, Yamazaki M, Komatsu M. Prediction of lowest nocturnal blood glucose level based on self-monitoring of blood glucose in Japanese patients with type 2 diabetes. J Diabetes Complications. (2018) 32:1118–23. doi: 10.1016/j.jdiacomp.2018.09.007
62. Chow LS, Zmora R, Ma S, Seaquist ER, Schreiner PJ. Development of a model to predict 5-year risk of severe hypoglycemia in patients with type 2 diabetes. BMJ Open Diabetes Res Care. (2018) 6:e000527. doi: 10.1136/bmjdrc-2018-000527
63. Torimoto K, Okada Y, Hajime M, Tanaka K, Tanaka Y. Risk Factors of hypoglycemia in patients with type 2 diabetes mellitus: a study based on continuous glucose monitoring. Diabetes Technol Ther. (2018) 20:603–12. doi: 10.1089/dia.2018.0017
64. Han K, Yun JS, Park YM, Ahn YB, Cho JH, Cha SA, et al. Development and validation of a risk prediction model for severe hypoglycemia in adult patients with type 2 diabetes: a nationwide population-based cohort study. Clin Epidemiol. (2018) 10:1545–59. doi: 10.1093/med/9780190238308.003.0004
65. Elvebakk O, Tronstad C, Birkeland KI, Jenssen TG, Bjorgaas MR, Froslie KF, et al. Evaluation of hypoglycaemia with non-invasive sensors in people with type 1 diabetes and impaired awareness of hypoglycaemia. Sci Rep. (2018) 8:14722. doi: 10.1038/s41598-018-33189-1
66. Winterstein AG, Jeon N, Staley B, Xu D, Henriksen C, Lipori GP. Development and validation of an automated algorithm for identifying patients at high risk for drug-induced hypoglycemia. Am J Health Syst Pharm. (2018) 75:1714–28. doi: 10.2146/ajhp180071
67. Mathioudakis NN, Everett E, Routh S, Pronovost PJ, Yeh HC, Golden SH, et al. Development and validation of a prediction model for insulin-associated hypoglycemia in non-critically ill hospitalized adults. BMJ Open Diabetes Res Care. (2018) 6:e000499. doi: 10.1136/bmjdrc-2017-000499
68. Cichosz SL, Henriksen MM, Tarnow L, Thorsteinsson B, Pedersen-Bjergaard U, Fleischer J. Validation of an algorithm for predicting hypoglycemia from continuous glucose measurements and heart rate variability data. J Diabetes Sci Technol. (2019) 13:1178–9. doi: 10.1177/1932296819864625
69. Elvebakk O, Tronstad C, Birkeland KI, Jenssen TG, Bjorgaas MR, Gulseth HL, et al. A multiparameter model for non-invasive detection of hypoglycemia. Physiol Meas. (2019) 40:085004. doi: 10.1088/1361-6579/ab3676
70. Jaggers JR, King KM, Watson SE, Wintergerst KA. Predicting nocturnal hypoglycemia with measures of physical activity intensity in adolescent athletes with type 1 diabetes. Diabetes Technol Ther. (2019) 21:406–8. doi: 10.1089/dia.2019.0048
71. Li X, Yu S, Zhang Z, Radican L, Cummins J, Engel SS, et al. Predictive modeling of hypoglycemia for clinical decision support in evaluating outpatients with diabetes mellitus. Curr Med Res Opin. (2019) 35:1885–91. doi: 10.1080/03007995.2019.1636016
72. Oviedo S, Contreras I, Bertachi A, Quiros C, Gimenez M, Conget I, et al. Minimizing postprandial hypoglycemia in type 1 diabetes patients using multiple insulin injections and capillary blood glucose self-monitoring with machine learning techniques. Comput Methods Programs Biomed. (2019) 178:175–80. doi: 10.1016/j.cmpb.2019.06.025
73. Oviedo S, Contreras I, Quiros C, Gimenez M, Conget I, Vehi J. Risk-based postprandial hypoglycemia forecasting using supervised learning. Int J Med Inform. (2019) 126:1–8. doi: 10.1016/j.ijmedinf.2019.03.008
74. Shah BR, Walji S, Kiss A, James JE, Lowe JM. Derivation and validation of a risk-prediction tool for hypoglycemia in hospitalized adults with diabetes: the hypoglycemia during hospitalization (hydho) score. Can J Diabetes. (2019) 43:278–82 e1. doi: 10.1016/j.jcjd.2018.08.061
75. Tronstad C, Elvebakk O, Staal OM, Kalvoy H, Hogetveit JO, Jenssen TG, et al. Non-invasive prediction of blood glucose trends during hypoglycemia. Anal Chim Acta. (2019) 1052:37–48. doi: 10.1016/j.aca.2018.12.009
76. Vu L, Kefayati S, Ide T, Pavuluri V, Jackson G, Latts L, et al. Predicting nocturnal hypoglycemia from continuous glucose monitoring data with extended prediction horizon. AMIA Annu Symp Proc. (2019) 2019:874–82.
77. Reddy R, Resalat N, Wilson LM, Castle JR, El Youssef J, Jacobs PG. Prediction of hypoglycemia during aerobic exercise in adults with type 1 diabetes. J Diabetes Sci Technol. (2019) 13:919–27. doi: 10.1177/1932296818823792
78. Yang J, Li L, Shi Y, Xie X. An arima model with adaptive orders for predicting blood glucose concentrations and hypoglycemia. IEEE J Biomed Health Inform. (2019) 23:1251–60. doi: 10.1109/JBHI.2018.2840690
79. Gadaleta M, Facchinetti A, Grisan E, Rossi M. Prediction of adverse glycemic events from continuous glucose monitoring signal. IEEE J Biomed Health Inform. (2019) 23:650–9. doi: 10.1109/JBHI.2018.2823763
80. Seo W, Lee YB, Lee S, Jin SM, Park SM. A machine-learning approach to predict postprandial hypoglycemia. BMC Med Inform Decis Mak. (2019) 19:210. doi: 10.1186/s12911-019-0943-4
81. Choi Y, Staley B, Soria-Saucedo R, Henriksen C, Rosenberg A, Winterstein AG. Common inpatient hypoglycemia phenotypes identified from an automated electronic health record-based prediction model. Am J Health Syst Pharm. (2019) 76:166–74. doi: 10.1093/ajhp/zxy017
82. Bertachi A, Vinals C, Biagi L, Contreras I, Vehi J, Conget I, et al. Prediction of nocturnal hypoglycemia in adults with type 1 diabetes under multiple daily injections using continuous glucose monitoring and physical activity monitor. Sensors (Basel, Switzerland). (2020) 20:1705. doi: 10.3390/s20061705
83. Elhadd T, Mall R, Bashir M, Palotti J, Fernandez-Luque L, Farooq F, et al. Artificial intelligence (Ai) based machine learning models predict glucose variability and hypoglycaemia risk in patients with type 2 diabetes on a multiple drug regimen who fast during Ramadan (the profast–It Ramadan Study). Diabetes Res Clin Pract. (2020) 169:108388. doi: 10.1016/j.diabres.2020.108388
84. Hu X, Xu W, Lin S, Zhang C, Ling C, Chen M. Development and validation of a hypoglycemia risk model for intensive insulin therapy in patients with type 2 diabetes. J Diabetes Res. (2020) 2020:7292108. doi: 10.1155/2020/7292108
85. Jensen MH, Dethlefsen C, Vestergaard P, Hejlesen O. Prediction of nocturnal hypoglycemia from continuous glucose monitoring data in people with type 1 diabetes: a proof-of-concept study. J Diabetes Sci Technol. (2020) 14:250–6. doi: 10.1177/1932296819868727
86. Khanimov I, Ditch M, Adler H, Giryes S, Felner Burg N, Boaz M, et al. Prediction of hypoglycemia during admission of non-critically ill patients: results from the menu study. Horm Metab Res. (2020) 52:660–8. doi: 10.1055/a-1181-8781
87. Khanimov I, Wainstein J, Boaz M, Shimonov M, Leibovitz E. Reduction of serum albumin in non-critically ill patients during hospitalization is associated with incident hypoglycaemia. Diabetes Metab. (2020) 46:27–32. doi: 10.1016/j.diabet.2019.03.003
88. Li J, Ma X, Tobore I, Liu Y, Kandwal A, Wang L, et al. A novel Cgm metric-gradient and combining mean sensor glucose enable to improve the prediction of nocturnal hypoglycemic events in patients with diabetes. J Diabetes Res. (2020) 2020:8830774. doi: 10.1155/2020/8830774
89. Ma S, Schreiner PJ, Seaquist ER, Ugurbil M, Zmora R, Chow LS. Multiple predictively equivalent risk models for handling missing data at time of prediction: with an application in severe hypoglycemia risk prediction for type 2 diabetes. J Biomed Inform. (2020) 103:103379. doi: 10.1016/j.jbi.2020.103379
90. Marcus Y, Eldor R, Yaron M, Shaklai S, Ish-Shalom M, Shefer G, et al. Improving blood glucose level predictability using machine learning. Diabetes Metab Res Rev. (2020) 36:e3348. doi: 10.1002/dmrr.3348
91. Misra-Hebert AD Ji X, Pantalone KM, Hu B, Dey T, Milinovich A, et al. Risk prediction for severe hypoglycemia in a type 2 diabetes population with previous non-severe hypoglycemia. J Diabetes Complications. (2020) 34:107490. doi: 10.1016/j.jdiacomp.2019.107490
92. Misra-Hebert AD, Milinovich A, Zajichek A, Ji X, Hobbs TD, Weng W, et al. Natural language processing improves detection of nonsevere hypoglycemia in medical records versus coding alone in patients with type 2 diabetes but does not improve prediction of severe hypoglycemia events: an analysis using the electronic medical record in a large health system. Diabetes Care. (2020) 43:1937–40. doi: 10.2337/dc19-1791
93. Mosquera-Lopez C, Dodier R, Tyler NS, Wilson LM, El Youssef J, Castle JR, et al. Predicting and preventing nocturnal hypoglycemia in type 1 diabetes using big data analytics and decision theoretic analysis. Diabetes Technol Ther. (2020) 22:801–11. doi: 10.1089/dia.2019.0458
94. Tran-Duy A, Knight J, Palmer AJ, Petrie D, Lung TWC, Herman WH, et al. A patient-level model to estimate lifetime health outcomes of patients with type 1 diabetes. Diabetes Care. (2020) 43:1741–9. doi: 10.2337/dc19-2249
95. Vehi J, Contreras I, Oviedo S, Biagi L, Bertachi A. Prediction and prevention of hypoglycaemic events in type-1 diabetic patients using machine learning. Health Informatics J. (2020) 26:703–18. doi: 10.1177/1460458219850682
96. Weiner M, Cummins J, Raji A, Ofner S, Iglay K, Teal E, et al. A randomized study on the usefulness of an electronic outpatient hypoglycemia risk calculator for clinicians of patients with diabetes in a safety-net institution. Curr Med Res Opin. (2020) 36:583–93. doi: 10.1080/03007995.2020.1717451
97. Calhoun P, Levine RA, Fan J. Repeated measures random forests (rmrf): identifying factors associated with nocturnal hypoglycemia. Biometrics. (2021) 77:343–51. doi: 10.1111/biom.13284
98. Elbaz M, Nashashibi J, Kushnir S, Leibovici L. Predicting hypoglycemia in hospitalized patients with diabetes: a derivation and validation study. Diabetes Res Clin Pract. (2021) 171:108611. doi: 10.1016/j.diabres.2020.108611
99. Wang W, Wang S, Wang X, Liu D, Geng Y, Wu T, et al. Glucose-insulin mixture model and application to short-term hypoglycemia prediction in the night time. IEEE Trans Biomed Eng. (2021) 68:834–45. doi: 10.1109/TBME.2020.3015199
100. Jermendy G, Kecskes A, Nagy A. Nocturnal hypoglycaemia in patients with diabetes mellitus: database analysis of a cohort using telemedicine support for self-monitoring of blood glucose over a 10-year-long period. Medicina (Kaunas, Lithuania). (2021) 57:167. doi: 10.3390/medicina57020167
101. Kyi M, Gorelik A, Reid J, Rowan LM, Wraight PR, Colman PG, et al. Clinical prediction tool to identify adults with type 2 diabetes at risk for persistent adverse glycemia in hospital. Can J Diabetes. (2021) 45:114–21 e3. doi: 10.1016/j.jcjd.2020.06.006
102. Li L, Xie X, Yang J. A Predictive model incorporating the change detection and winsorization methods for alerting hypoglycemia and hyperglycemia. Med Biol Eng Comput. (2021) 59:2311–24. doi: 10.1007/s11517-021-02433-8
103. Dave D, DeSalvo DJ, Haridas B, McKay S, Shenoy A, Koh CJ, et al. Feature-based machine learning model for real-time hypoglycemia prediction. J Diabetes Sci Technol. (2021) 15:842–55. doi: 10.1177/1932296820922622
104. Yu X, Ma N, Yang T, Zhang Y, Miao Q, Tao J, et al. A multi-level hypoglycemia early alarm system based on sequence pattern mining. BMC Med Inform Decis Mak. (2021) 21:22. doi: 10.1186/s12911-021-01389-x
105. Prendin F, Del Favero S, Vettoretti M, Sparacino G, Facchinetti A. Forecasting of glucose levels and hypoglycemic events: head-to-head comparison of linear and nonlinear data-driven algorithms based on continuous glucose monitoring data only. Sensors (Basel, Switzerland). (2021) 21:1647. doi: 10.3390/s21051647
106. Wenbo W, Yang S, Guici C. Blood glucose concentration prediction based on Vmd-Kelm-Adaboost. Med Biol Eng Comput. (2021) 59:2219–35. doi: 10.1007/s11517-021-02430-x
107. Han H, Lai J, Yan C, Li X, Hu S, He Y, et al. Development and validation of a prediction model of perioperative hypoglycemia risk in patients with type 2 diabetes undergoing elective surgery. BMC Surg. (2022) 22:167. doi: 10.1186/s12893-022-01601-3
108. Witte H, Nakas C, Bally L, Leichtle AB. Machine learning prediction of hypoglycemia and hyperglycemia from electronic health records: algorithm development and validation. JMIR. (2022) 6:e36176. doi: 10.2196/36176
109. Yang H, Li J, Liu S, Yang X, Liu J. Predicting risk of hypoglycemia in patients with type 2 diabetes by electronic health record-based machine learning: development and validation. JMIR. (2022) 10:e36958. doi: 10.2196/36958
110. Yun JS, Han K, Choi SY, Cha SA, Ahn YB, Ko SH. External validation and clinical application of the predictive model for severe hypoglycemia. Front Endocrinol. (2022) 13:1006470. doi: 10.3389/fendo.2022.1006470
111. Wright AP, Embi PJ, Nelson SD, Smith JC, Turchin A, Mize DE. Development and validation of inpatient hypoglycemia models centered around the insulin ordering process. J Diabetes Sci Technol. (2022) 2022:19322968221119788. doi: 10.1177/19322968221119788
112. Berikov VB, Kutnenko OA, Semenova JF, Klimontov VV. Machine learning models for nocturnal hypoglycemia prediction in hospitalized patients with type 1 diabetes. J Pers Med. (2022) 12:1262. doi: 10.3390/jpm12081262
113. Parcerisas A, Contreras I, Delecourt A, Bertachi A, Beneyto A, Conget I, et al. A machine learning approach to minimize nocturnal hypoglycemic events in type 1 diabetic patients under multiple doses of insulin. Sensors (Basel, Switzerland). (2022) 22:4. doi: 10.3390/s22041665
114. Wang S, Tan Z, Wu T, Shen Q, Huang P, Wang L, et al. Largest amplitude of glycemic excursion calculating from self-monitoring blood glucose predicted the episodes of nocturnal asymptomatic hypoglycemia detecting by continuous glucose monitoring in outpatients with type 2 diabetes. Front Endocrinol. (2022) 13:858912. doi: 10.3389/fendo.2022.858912
115. Tyler NS, Mosquera-Lopez C, Young GM, El Youssef J, Castle JR, Jacobs PG. Quantifying the impact of physical activity on future glucose trends using machine learning. iScience. (2022) 25:103888. doi: 10.1016/j.isci.2022.103888
116. Duckworth C, Guy MJ, Kumaran A, O'Kane AA, Ayobi A, Chapman A, et al. Explainable machine learning for real-time hypoglycemia and hyperglycemia prediction and personalized control recommendations. J Diabetes Sci Technol. (2022):19322968221103561. doi: 10.1177/19322968221103561
117. Faccioli S, Prendin F, Facchinetti A, Sparacino G, Del Favero S. Combined use of glucose-specific model identification and alarm strategy based on prediction-funnel to improve online forecasting of hypoglycemic events. J Diabetes Sci Technol. (2022) 2022:19322968221093665. doi: 10.1177/19322968221093665
118. Park JU, Kim Y, Lee Y, Urtnasan E, Lee KJ, A. Prediction algorithm for hypoglycemia based on support vector machine using glucose level and electrocardiogram. J Med Syst. (2022) 46:68. doi: 10.1007/s10916-022-01859-3
119. Zhu T, Li K, Herrero P, Georgiou P. Personalized Blood Glucose Prediction for Type 1 Diabetes Using Evidential Deep Learning and Meta-Learning. In: IEEE Transactions on Bio-Medical Engineering (2022).
120. Zhu T, Uduku C, Li K, Herrero P, Oliver N, Georgiou P. Enhancing self-management in type 1 diabetes with wearables and deep learning. NPJ Digital Med. (2022) 5:78. doi: 10.1038/s41746-022-00626-5
121. Bleeker SE, Moll HA, Steyerberg EW, Donders AR, Derksen-Lubsen G, Grobbee DE, et al. External validation is necessary in prediction research: a clinical example. J Clin Epidemiol. (2003) 56:826–32. doi: 10.1016/S0895-4356(03)00207-5
122. Yadav S, Shukla S. Analysis of K-Fold Cross-Validation over Hold-out Validation on Colossal Datasets for Quality Classification. (2016) p. 78–83. doi: 10.1109/IACC.2016.25
123. Hovorka R. Closed-loop insulin delivery: from bench to clinical practice. Nat Rev Endocrinol. (2011) 7:385–95. doi: 10.1038/nrendo.2011.32
124. Cobelli C, Renard E, Kovatchev B. Artificial pancreas: past, present, future. Diabetes. (2011) 60:2672–82. doi: 10.2337/db11-0654
125. Roy A, Parker RS. Dynamic modeling of exercise effects on plasma glucose and insulin levels. J Diabetes Sci Technol. (2007) 1:338–47. doi: 10.1177/193229680700100305
Keywords: diabetes mellitus, hypoglycemia, prediction, data-based algorithms or models, machine learning
Citation: Zhang L, Yang L and Zhou Z (2023) Data-based modeling for hypoglycemia prediction: Importance, trends, and implications for clinical practice. Front. Public Health 11:1044059. doi: 10.3389/fpubh.2023.1044059
Received: 14 September 2022; Accepted: 10 January 2023;
Published: 26 January 2023.
Edited by:
João Valente Cordeiro, New University of Lisbon, PortugalReviewed by:
Nestoras Mathioudakis, Johns Hopkins University, United StatesCopyright © 2023 Zhang, Yang and Zhou. This is an open-access article distributed under the terms of the Creative Commons Attribution License (CC BY). The use, distribution or reproduction in other forums is permitted, provided the original author(s) and the copyright owner(s) are credited and that the original publication in this journal is cited, in accordance with accepted academic practice. No use, distribution or reproduction is permitted which does not comply with these terms.
*Correspondence: Lin Yang, eWFuZ2xpbl9uZm1AY3N1LmVkdS5jbg==
Disclaimer: All claims expressed in this article are solely those of the authors and do not necessarily represent those of their affiliated organizations, or those of the publisher, the editors and the reviewers. Any product that may be evaluated in this article or claim that may be made by its manufacturer is not guaranteed or endorsed by the publisher.
Research integrity at Frontiers
Learn more about the work of our research integrity team to safeguard the quality of each article we publish.