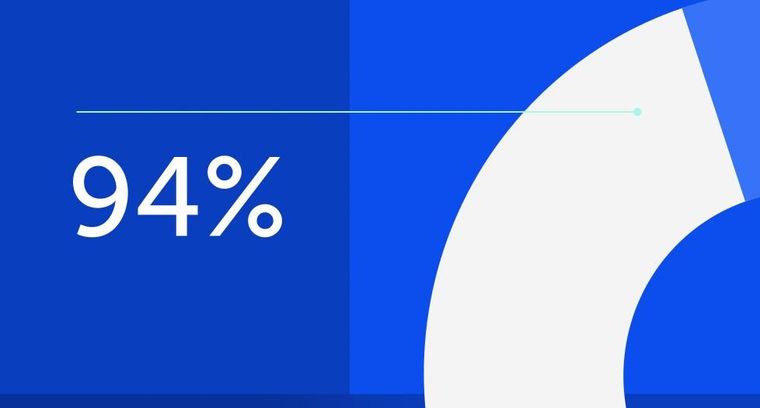
94% of researchers rate our articles as excellent or good
Learn more about the work of our research integrity team to safeguard the quality of each article we publish.
Find out more
ORIGINAL RESEARCH article
Front. Psychol.
Sec. Cognitive Science
Volume 16 - 2025 | doi: 10.3389/fpsyg.2025.1591618
This article is part of the Research TopicAdvancing Generative AI in WorldbuildingView all articles
The final, formatted version of the article will be published soon.
Select one of your emails
You have multiple emails registered with Frontiers:
Notify me on publication
Please enter your email address:
If you already have an account, please login
You don't have a Frontiers account ? You can register here
The surge in the capabilities of large language models (LLMs) has propelled the development of Artificial General Intelligence (AGI), highlighting generative agents as pivotal components for emulating complex AI behaviors. Given the high costs associated with individually training LLMs for each AI agent, there is a critical need for advanced memory retrieval mechanisms to maintain the unique characteristics and memories of individual AI agents. In this research, we developed a text-based simulation of a generative agent world, constructing a community with multiple agents and locations in which certain levels of interaction were enabled. Within this framework, we introduced a novel memory retrieval system using an Auxiliary Cross Attention Network (ACAN).This system calculates and ranks attention weights between an agent's current state and stored memories, selecting the most relevant memories for any given situation. In a novel approach, we incorporated LLM assistance, comparing memories retrieved by our model with those extracted using a base method during training, and constructing a novel loss function based on these comparisons to optimize the training process effectively. To our knowledge, this is the first study to utilize LLMs to train a dedicated agent memory retrieval network. Our empirical evaluations demonstrate that this approach substantially enhances the quality of memory retrieval, thereby increasing the adaptability and behavioral consistency of agents in fluctuating environments.Our findings not only introduce new perspectives and methodologies for memory retrieval in generative agents but also extend the utility of LLMs in memory management across varied AI agent applications.
Keywords: Artificial intelligence(AI), large language Models(LLMs), generative agents, memory retrieval, attention mechanism
Received: 11 Mar 2025; Accepted: 11 Apr 2025.
Copyright: © 2025 Chuanyang and He. This is an open-access article distributed under the terms of the Creative Commons Attribution License (CC BY). The use, distribution or reproduction in other forums is permitted, provided the original author(s) or licensor are credited and that the original publication in this journal is cited, in accordance with accepted academic practice. No use, distribution or reproduction is permitted which does not comply with these terms.
* Correspondence: Qingyun He, School of Finance and Economics, Anhui Science and Technology University, Bengbu, China
Disclaimer: All claims expressed in this article are solely those of the authors and do not necessarily represent those of their affiliated organizations, or those of the publisher, the editors and the reviewers. Any product that may be evaluated in this article or claim that may be made by its manufacturer is not guaranteed or endorsed by the publisher.
Supplementary Material
Research integrity at Frontiers
Learn more about the work of our research integrity team to safeguard the quality of each article we publish.