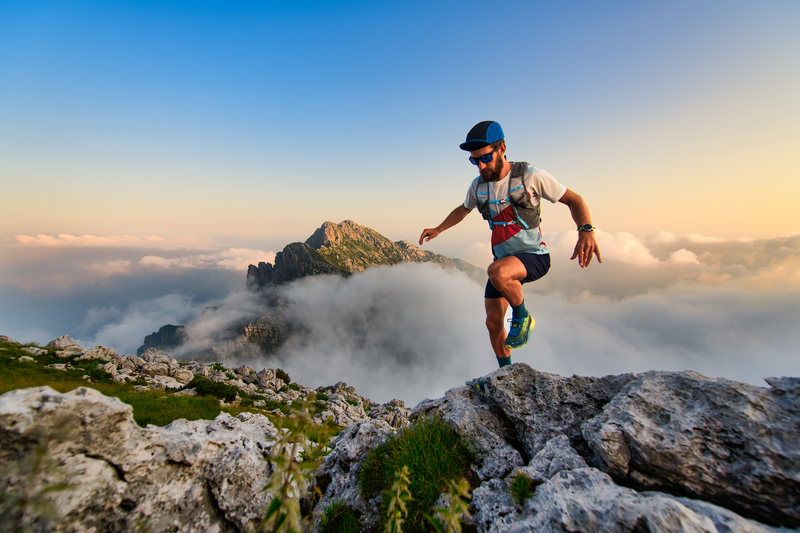
95% of researchers rate our articles as excellent or good
Learn more about the work of our research integrity team to safeguard the quality of each article we publish.
Find out more
SPECIALTY GRAND CHALLENGE article
Front. Psychol.
Sec. Cognition
Volume 16 - 2025 | doi: 10.3389/fpsyg.2025.1581160
The final, formatted version of the article will be published soon.
You have multiple emails registered with Frontiers:
Please enter your email address:
If you already have an account, please login
You don't have a Frontiers account ? You can register here
In this Editorial Grand Challenge, I will first summarize some of the multifaceted (intrinsic and extrinsic) reasons why cognitive psychology, along with many other scientific fields, has gone through a replicability crisis. Then, I will illustrate some of the proposed remedies that are bringing to a credibility revolution. I will highlight that the proposed best practices to complex epistemological problems, while necessary, must also be critically evaluated, to ward off potentially new concerns. Finally, I will advocate for a new balance to ensure methodologically sound and credible science, while also safeguarding scientific sustainability, creativity and enthusiasm.In the history of psychology, periods of vibrant discoveries and exciting research have been occasionally perturbed by troubling waves of replicability crisis, with the most recent one still ongoing (Lakens, 2024;Open Science Collaboration, 2015). While cognitive psychology seemed to be slightly less affected by the replicability crisis than social psychology (Open Science Collaboration, 2015), it has certainly not been immune to similar concerns.Firstly, publications with small sample sizes and inadequate statistical power often led to findings that could not always be reliably reproduced (Button et al., 2013). Secondly, the pressure to publish novel and positive results, coupled with distorting incentives, has fostered questionable research practices (or even fraud, in the worst cases), such as for instance selective reporting and p-hacking, where some researchers might, more or less deliberately, manipulate or select data to achieve statistically significant outcomes (e.g., Lilienfeld, 2017;Nosek et al., 2012). Additionally, complex experimental designs, researchers' degrees of freedom and variability in methodological practices have contributed to inconsistencies across studies. The prevalence of a confirmation bias, that is, the researchers' tendency to favor data that support their hypotheses, has further exacerbated this issue. Moreover, a lack of transparency in data sharing and in reporting methodological details has hindered efforts to replicate findings accurately. These challenges are currently triggering a massive cultural paradigm shift that would imply to entirely rethink the research process. It is not surprising that a growing movement, largely and meritoriously driven by the new generation of researchers, is advocating for more rigorous and transparent scientific practices. This shift is a virtuous reaction to the replicability crisis (e.g., Lindsay, 2020). These practices include for instance: (i) registered reports or at least pre-registration of studies to minimize questionable practices such as Harking (Kerr, 1998), cherry-picking and confirmation biases (e.g., Clark et al., 2022), (ii) implementing open data initiatives to increase transparency and maximization of collective scientific efforts (e.g., Mayernik, 2017;Rasti et al., 2025), and (iii) conducting replication efforts through coordinated multi-lab projects to achieve the big sample sizes necessary for sufficient statistical power. Therefore, the replication crisis is currently driving positive, praiseworthy, changes in the methods, procedures and, importantly, policies that would eventually and ideally benefit the credibility of the scientific community as a whole (e.g., Korbmacher et al., 2023). However, as I will argue throughout this Editorial, one would be naïve to think that the solutions proposed for these complex epistemological problems are always straightforward and without their own drawbacks. Any new remedy needs to be critically evaluated, and a new balance must be sought.As a first consideration, it is undeniable that the replicability crisis can be, at least partially, attributed to a series of questionable research practices that have been commonly used, tolerated, or even favored by standard practices in the field and by ill-conceived academic incentives. However, we should not overlook other potential factors that might have contributed as well to the replicability crisis in our specific field. Replicability issues may indeed also stem, in part, from deeper epistemological and methodological problems.The social sciences, and cognitive psychology is no exception, deal with objects of investigation that cannot be directly observed but are primarily captured through latent variables and constructs. These constructs require careful, and often challenging, operationalization in order to be attacked scientifically through some measurable proxies. Since cognitive processes are inherently "fuzzier" and more ambiguous compared to more basic biological processes, there is typically no single best operationalization of a given construct to be tested, and ontological definitions require extra efforts.To take a cognitive psychology example used by Poldrack & Yarkoni (2016), performance on Nback tasks and OSPAN tasks shows weak, and often non-significant, correlations (Kane et al., 2007;Oberauer, 2005;Roberts & Gibson, 2002). This is unsettling, as both tasks were designed to assess the same cognitive construct, that is, working memory. Such discrepancies suggest issues like ambiguous operational definitions and task impurity (Kane et al., 2007), contributing to entropy in the literature. Some promising remedies have been put forward. These include the Cognitive Atlas Project (Poldrack & Yarkoni, 2016), a collaborative project to develop a knowledge base that represents the current state of cognitive science, or the Cognitive Paradigm Ontology (Turner & Laird, 2012), a systematic collection of cognitive paradigms for functional neuroimaging applications. However, although these tools are commendable, the risk could be that only global inferences could be drawn. This would happen if the tasks included in these databases are too general and lack subtask-specific manipulations. Indeed, despite their methodological idiosyncrasies, subtle experimental manipulations are often more theoretically meaningful than the common features across tasks.Additionally, the obtained findings need to be interpreted, with more than one account often being equally plausible at the same time, thus lowering internal validity. This produces uncertainty about what interpretation of findings could be the most accurate, increasing the likelihood that biased conclusions might be preferred instead. A related consequent issue is the so-called motivated research, which occurs when factors unrelated to accuracy influence how scientists engage with existing data, generate new hypotheses, collect, analyze and interpret data, and communicate research outcomes to their peers and general audience (e.g., Clark, Honeycutt, et al., 2022;Kunda, 1990). These factors include confirmation biases as well as the need for social and moral approval of specific research topics and conclusions.Furthermore, delving deeper into the core of the issue, the inherent variability of the object of investigation is another major challenge in the social sciences, also including cognitive psychology.The manifestation of cognitive processes can indeed vary considerably, not only across individuals but also intra-individually (e.g., Dhawale et al., 2017;Fiske & Rice, 1955;Kahana et al., 2018;MacPherson et al., 2019), with variability being a key aspect of cognition that cannot be (or at least not exclusively) dismissed as measurement error (Judd et al., 2024). Variability is indeed not only spuriously caused by ill-defined operationalizations of cognitive constructs, imprecise measurements of their proxies (e.g., behavior), violations of statistical assumptions, power issues, and more generally, poor experimental control and other methodological flaws (e.g., Clark & Watson, 2019;Podsakoff et al., 2012). There are also other fundamental sources of variability linked to the very nature of mental processes.Natural fluctuations characterize the manifestations of mental functions such as perception, emotion, memory, executive functions, decision-making, to cite a few. This variability arises from numerous factors, including psychobiological variables, genetic differences, developmental stages, but also situational contexts and environmental influences (e.g., epigenetics).Specifically, cognitive performance can be affected by an individual's mood (Chepenik et al., 2007), motivation (Braver et al., 2014), stress (Steinhauser et al., 2007), sleep deprivation (Killgore, 2010), and prior experience (Pastukhov & Braun, 2011). Moreover, the brain's complex neural architecture and the dynamic nature of its networks contribute to this variability, as its connectome is constantly shaped and reshaped by new experiences, providing the neural foundation for an ever-changing cognitive life (e.g., Dosenbach et al., 2010;Kolb & Gibb, 2011;Seung, 2012;Tost et al., 2015). Acknowledging, and ideally accounting for, this intrinsic variability and lability of the object of investigation in cognitive psychology (i.e., mental processes) is essential for developing robust, or at least plausible, theories and applications in cognitive psychology and neuroscience.Additionally, many findings cannot be easily generalized across different contexts (e.g., cultural, spatial, temporal) or experimental conditions (Stroebe & Strack, 2014). The environmental or experimental context can indeed affect both how and to what extent a cognitive process is activated and expressed. An example is provided in Hommel and colleagues' (2012) work. That study showed that cognitive control could be, somewhat counterintuitively, more effective when exerted in the context of the acoustic noise typical of fMRI sequences than in standard settings. This specific example raises more general considerations about the potential applicability of Heisenberg's uncertainty principle in cognitive neuroscience, where the object of investigation (the mind) is being transformed by the very tools used to study it. A more classic example of this issue is the Hawthorne effect, where individuals alter their behavior because they are aware of being observed and measured (e.g., Adair, 1984). Although, ironically enough, this famous phenomenon is probably not fully reliable, or at least not in its initial reporting (Letrud & Hernes, 2019;Levitt & List, 2011), it represents just one example of the broader (but often neglected) modulatory role of participant's motivation and demand characteristics in task performance (e.g., Orne, 1962;Weber & Cook, 1972). To summarize this section, while variability might be seen as an obstacle to advancing science, as it may lead to on-average null effects, uncovering its intrinsic (but also exogenous) sources can also have a leveraging effect on theory development. Variability could indeed be exploited to better understand inter-individual differences in cognition and advance cognitive theories (e.g., Langerock et al., 2025;Miller & Schwarz, 2017;Wang et al., 2012;cf., Rowe & Healy, 2014).A potential concern with the remedies proposed for the replicability crisis is that, while highly desirable in principle, some of them conceal other risks that should at least be acknowledged, if not actively counteracted. As the Latin writer Horace once wrote: "Est modus in rebus", which reminds us to pursue balance in life by avoiding deleterious extremes. As it shall become clear from what follows, the coveted solutions proposed to overcome the replicability crisis are also subject to this caveat. Therefore, we need to avoid applying them uncritically.The widespread availability of large open datasets, while on one side promoting reproducibility and collective efficiency, also inevitably encourages data mining and exploratory approaches. Far be it from me to want to unleash or incite a witch hunt, such a data-driven mindset (which could bring to a so-called fishing expedition attitude) might sometimes temptingly lead to the post hoc generation of hypotheses (HARKing) and, what is even worse, a lack of theoretical depth. A data-driven attitude is also encouraged by the unprecedented, exponential expansion of Machine Learning and other Artificial Intelligence (AI) algorithms. AI can undoubtedly enhance the efficient exploration and analysis of complex scientific data in different ways, such as identifying patterns and trends, allowing predictive modeling, integrating multidimensional data and so on, accelerating data-driven discoveries also in the cognitive psychology field. However, would powerful but opaque AI algorithms always help enhance our theoretical understanding of underlying cognitive mechanisms? And, in case it is achievable, could this AI-based understanding then generalize to new datasets? Although the debate in the field is currently heated with these and other open questions, it would definitely benefit from more extensive and deeper epistemological reflection, hopefully with contributions also coming from cognitive psychology.One proposed solution to the risks of a lenient data-driven attitude is the pre-registration of studies, which forces researchers to specify their a priori hypotheses truly in advance (and to clearly label as exploratory all the other hypotheses that were generated a posteriori with respect to the preregistration). However, this and other credibility-enhancing practices are still adopted on a voluntary basis and are not yet routinely applied gold standards. Additionally, pre-registration alone (as just a bureaucratic formality) is not sufficient. It would be equally important to confront the deeper issue of the theoretical crisis: the hypotheses that are pre-registered could still be solely empirical in nature or, even worse, poorly formulated, unjustified and without strong theoretical foundations. Thus, preregistering hypotheses helps reassure the scientific community that our thinking remains unbiased but, in itself, does not substitute for theoretical and logic-based reasoning. Simply pre-registering a hypothesis, as a sort of fig leaf, without substantiating it with a solid theoretical rationale serves little purpose, and does not enhance the credibility of the hypothesis in itself (Oberauer & Lewandowsky, 2019). Moreover, it would be fine to candidly admit that a given study was exploratory, especially if at the beginning of a new research line, as long as the data obtained could inspire theory development, new hypotheses, and further research to test these hypotheses and refine the theory.Excessive focus on trying to empirically reproduce or replicate published effects or phenomena, while extremely valuable to reshape the trust and foundation of what we already know about human cognition, if an end in itself, may divert attention away from developing robust theories (Oberauer & Lewandowsky, 2019). Proposing strong theories would allow scholars to formulate credible and specific hypotheses, benefiting the scientific progress (Clark, Honeycutt, et al., 2022). Developing accurate and comprehensive theories or at least models of cognitive processes is inherently challenging due to their fuzzy nature and complexity. Thus, theories and models need to consider a broad spectrum of variables and interactions, necessitating advanced computational approaches, biological plausibility and extensive experimental testing (e.g., Busemeyer & Diederich, 2010;Turner et al., 2017). This process could benefit from collective, multidisciplinary scientific coordination toward common, challenging goals (Brignol et al., 2024;Rasti et al., 2025).A special type of scientific coordination proposed as a remedy for the lack of a theory-oriented mindset is represented by adversarial collaborations (sometimes referred to as "coopetition"; Clark et al., 2022;Ellemers et al., 2020). Although challenging, engaging with those who propose alternative theories could be scientifically rewarding in the long run (see Cowan et al., 2020;Mellers et al., 2001, for some examples). These collaborations require clear, testable hypotheses, mutual understanding of differing viewpoints, and methods that can differentiate and potentially falsify both hypotheses. This process limits researchers' biases and beliefs, enhances the integrity of methodological approaches, and advances debates effectively. By committing to methods before testing, even better when coupled with good practices such as pre-registering, open data and replication, scholars reduce the risk to post-hoc criticize or hide unwanted results. This would also make findings more credible to the scientific community. More plausible hypotheses will prevail, and incorrect ones will be falsified sooner, preventing wasted time and resources and increasing the reliability and credibility of scientific findings.Relying on increasingly sophisticated methods for analyzing rich and complex data hides similar risks. Take the field of neuroimaging as an example. While progress in the neuroimaging data analysis has provided valuable insights into brain activity, understanding how these findings are related to cognitive processes is still difficult. Explaining how complex mental functions arise from brain regions and neural networks has traditionally posed a major challenge (e.g., Coltheart, 2006;Hommel & Colzato, 2017;Niv, 2021). More specific to recent advances in the field, while connectomics approaches in cognitive neuroscience (e.g., graph theory) have significantly enhanced our ability to explain the complexity of brain organization, at least in principle, clear relationships between topological indices of network integration and segregation and cognitive processes have yet to be fully established (Litwińczuk et al., 2023). These relationships would be crucial for appreciating the biological plausibility and behavioral relevance of these highly derived, network-based measures.To make matters worse, it is acknowledged that behavioral data are not always characterized by sufficient fidelity to support robust and reliable brain-behavior correlations in the neuroimaging literature (Tiego & Fornito, 2022). Additionally, some common interpretations of cognitive neuroscience findings are unjustified, because they rely on flawed reasoning or other unproven assumptions. A well-known example of flawed reasoning is reverse inference, that is, deducing the involvement of particular mental processes solely based on observed patterns of brain activation, without the constraints of elegant experimental manipulations that would enhance internal validity (Kriegeskorte et al., 2009;Poldrack, 2006). As another example, many compensatory cognitive interpretations of brain patterns in older adults are not fully justified unless data are analyzed longitudinally (e.g., Pudas et al., 2013).Another potential drawback of widely available open data is the inherent risk of salami slicing, which involves splitting data from a research study that should form a single publication into several smaller publishable units. Salami slicing, which has long been considered a questionable and unethical research practice in various fields (Karlsson & Beaufils, 2013;Siegel & Baveye, 2010;Spielmans et al., 2010), remains a significant concern, and even more so in the era of open science. This practice can lead to various issues, such as consuming excessive resources during the editorial and review process and overwhelming science readers. It can also lead to an inflated number of articles cluttering the literature, and additionally increase the risk of publication bias, and distort effect sizes in meta-analyses. To overcome this issue, the same or overlapping data samples should always be properly acknowledged as such across different publications (e.g., Hilgard et al., 2019;Urbanowicz & Reinke, 2018).Furthermore, also worth mentioning, the risk of wasting resources and produce flawed science becomes amplified in case the tasks used to collect behavioral data in large open datasets were not carefully designed and suffer from some bug.A well-known trade off exists between experimental control and generalizability. On one hand, more attention to well-designed experimental paradigms that take care of confounds when trying to fully characterize a psychological effect is desirable to increase internal validity (e.g., Verbruggen et al., 2019;Viviani et al., 2024), and hopefully to also enhance scientific credibility and replicability. On the other hand, excessive methodological control in research poses a significant risk, as it can lead to a decrease of generalizability, that is, external and ecological validity (Shadish et al., 2002). In other words, the more tightly controlled experiments become, the less accurately their findings reflect realworld conditions, making it difficult to apply the results to broader contexts or diverse populations. This undermines the relevance and applicability of the research, limiting its potential impact outside the highly controlled environment in which it was conducted.Another potential risk of placing too much emphasis on the remedies for the replicability crisis is a decrease in enthusiasm for exploration, creativity and discovery, that are also essential aspects of the scientific process, complementary to methodological rigor. Focusing too much on strict standards, while being undeniably vital for overcoming the pressing credibility crisis, might also lead to excessive caution, hindering divergent thinking and the serendipitous attitude that drives new discoveries (Kaufman & Glǎveanu, 2018;Ness, 2015). In principle, most researchers would subscribe to recommendations, best practices, and, more generally, to virtuous organized skepticism, in the hope the scientific community will universally adopt them (Anderson et al., 2007). However, when these begin to become imposed norms, a looming risk is that they can lead to additional paperwork in fulfilling the already hypertrophic and self-referential accountability and evaluation processes. The latter require extra time and mental energy, limited resources that researchers would prefer to devote, with much more motivation and enthusiasm, to their actual research activities. If new practices become excessively demanding due to an increased bureaucracy burden, they risk reducing the time and resources available for creative activities (Kaufman & Glǎveanu, 2018). In other words, with excessive constraints, creativity could also be suppressed (Medeiros et al., 2014).This jeopardy within the scientific field should be considered in addition to the already observed decline in creativity within the general population (Kim, 2011).In other words, while best practices in science are indispensable and non-negotiable, the burdensome bureaucracy and other possible downsides that might accompany their implementation should be kept strictly in check, and new incentives should be envisioned that could promote slower but more accurate science and allow for more quality time. Scientists are therefore compelled to closely monitor the tendency toward hyper-bureaucratization of the scientific process and continually seek a new balance between the apparently conflicting, yet equally essential needs of methodological rigor and scientific creativity (Ness, 2015;Scheffer, 2014;Simonton, 2004), while moving away from purely quantity-based institutional evaluation processes and incentives.The awareness of a massive replicability crisis has been a slap in the face for the scientific community. It is undeniable that we can no longer continue with business as usual, and remedies to address this crisis must be implemented. However, as I have tried to argue here, this cannot be done naively or uncritically, as any new practice could also bring its own drawbacks, including changes in how we value scientific research. It has been shown, for instance, that awareness of replication failures and criticism of questionable research practices can diminish trust in past research (Anvari & Lakens, 2018). What is worse, being informed about potential solutions, such as increased transparency, could be, somewhat paradoxically, associated to reduced trust in future research (Anvari & Lakens, 2018). This highlights a significant issue with research trustworthiness, intensified by a lack of enthusiasm.In conclusion, as discussed in this Editorial, the proposed remedies aimed at fostering the credibility revolution are highly desirable and non-negotiable. However, these remedies (along with others yet to be envisaged) cannot, sic et simpliciter, be seen as a panacea for the replicability crisis without first being critically evaluated for their own hidden risks or costs. To avoid throwing the baby out with the bathwater, the scientific community desperately needs to find a new balance in the scientific process between imperative methodological rigor and best practices on one hand, and enthusiastic theory-advancing creativity and sustainability on the other. Both types of needs require more quality time for researchers, free from ill-conceived (and only apparently objective) institutional academic incentives and excessive bureaucratic burden. We need to fine-tune best practices that ensure methodological thoroughness, without renouncing greasing the wheels of scientific creativity, while minimizing the overproduction of non-replicable results. Although I am certainly not the first to propose this sort of call to action, I hope that our journal, Frontiers in Psychology: Cognition, can serve (through specific Research Topics or other formats) as an inspiring receptacle for new ways of conceiving the entire scientific process in the pursuit of this new balance, free from any chauvinistic preconceptions. The author confirms being the sole contributor of this work and has approved it for publication. 7 Funding AV is partially supported by the Italian Ministry of University and Research (MUR) and European Union in the framework of PNRR NextGenerationEU with projects: MNESYS (CUP #B83C22004960002; PE0000006) and ORCA (CUP #J93C24000190007; PE0000013). 8 Acknowledgments Part of this work was inspired by fruitful discussions with Dr. Antonino Visalli and other esteemed colleagues. ChatGPT (OpenAI, 2024) was occasionally used for language editing purposes. 9 References
Keywords: replicability crisis, credibility revolution, Scientific Discovery, creativity, psychological theories, open science 1 Introduction: replicability crisis
Received: 21 Feb 2025; Accepted: 28 Mar 2025.
Copyright: © 2025 Vallesi. This is an open-access article distributed under the terms of the Creative Commons Attribution License (CC BY). The use, distribution or reproduction in other forums is permitted, provided the original author(s) or licensor are credited and that the original publication in this journal is cited, in accordance with accepted academic practice. No use, distribution or reproduction is permitted which does not comply with these terms.
* Correspondence:
Antonino Vallesi, Dipartimento di Neuroscienze: Scienze NPSRR, Department of Neuroscience, School of Medicine and Surgery, University of Padua, Padua, 35128, Italy
Disclaimer: All claims expressed in this article are solely those of the authors and do not necessarily represent those of their affiliated organizations, or those of the publisher, the editors and the reviewers. Any product that may be evaluated in this article or claim that may be made by its manufacturer is not guaranteed or endorsed by the publisher.
Research integrity at Frontiers
Learn more about the work of our research integrity team to safeguard the quality of each article we publish.