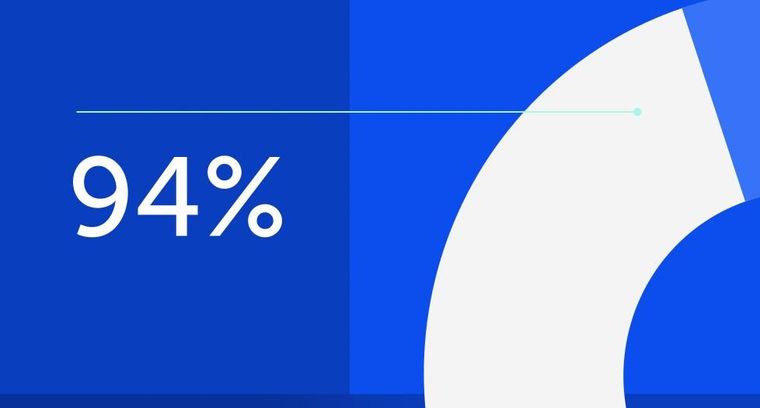
94% of researchers rate our articles as excellent or good
Learn more about the work of our research integrity team to safeguard the quality of each article we publish.
Find out more
ORIGINAL RESEARCH article
Front. Psychol.
Sec. Cognition
Volume 16 - 2025 | doi: 10.3389/fpsyg.2025.1574809
The final, formatted version of the article will be published soon.
Select one of your emails
You have multiple emails registered with Frontiers:
Notify me on publication
Please enter your email address:
If you already have an account, please login
You don't have a Frontiers account ? You can register here
Due to the application of Artificial Intelligence (AI) in high-risk domains like law or medicine,trustworthy AI and trust in AI are of increasing scientific and public relevance. A typical conception,for example in the context of medical diagnosis, is that a knowledgeable user receives AI-generated classification as advice. Research to improve such interactions often aims to foster theuser’s trust, which in turn should improve the combined human-AI performance. Given that AImodels can err, we argue that the possibility to critically review, thus to distrust, an AI decision isan equally interesting target of research.We created two image classification scenarios in which the participants received mock-upAI advice. The quality of the advice decreases for a phase of the experiment. We studied thetask performance, trust and distrust of the participants, and tested whether an instruction toremain skeptical and review each piece of advice led to a better performance compared to aneutral condition. Our results indicate that this instruction does not improve but rather worsensthe participants’ performance. Repeated single-item self-report of trust and distrust shows anincrease in trust and a decrease in distrust after the drop in the AI’s classification quality, with nodifference between the two instructions. Furthermore, via a Bayesian Signal Detection Theoryanalysis, we provide a procedure to assess appropriate reliance in detail, by quantifying whetherthe problems of under- and over-reliance have been mitigated. We discuss implications of ourresults for the usage of disclaimers before interacting with AI, as prominently used in currentLLM-based chatbots, and for trust and distrust research.
Keywords: Trust in AI, Trust, distrust, Human-AI interaction, Signal detection theory, Bayesian parameter estimation, image classification
Received: 11 Feb 2025; Accepted: 08 Apr 2025.
Copyright: © 2025 Peters and Scharlau. This is an open-access article distributed under the terms of the Creative Commons Attribution License (CC BY). The use, distribution or reproduction in other forums is permitted, provided the original author(s) or licensor are credited and that the original publication in this journal is cited, in accordance with accepted academic practice. No use, distribution or reproduction is permitted which does not comply with these terms.
* Correspondence: Tobias Martin Peters, University of Paderborn, Paderborn, Germany
Disclaimer: All claims expressed in this article are solely those of the authors and do not necessarily represent those of their affiliated organizations, or those of the publisher, the editors and the reviewers. Any product that may be evaluated in this article or claim that may be made by its manufacturer is not guaranteed or endorsed by the publisher.
Supplementary Material
Research integrity at Frontiers
Learn more about the work of our research integrity team to safeguard the quality of each article we publish.