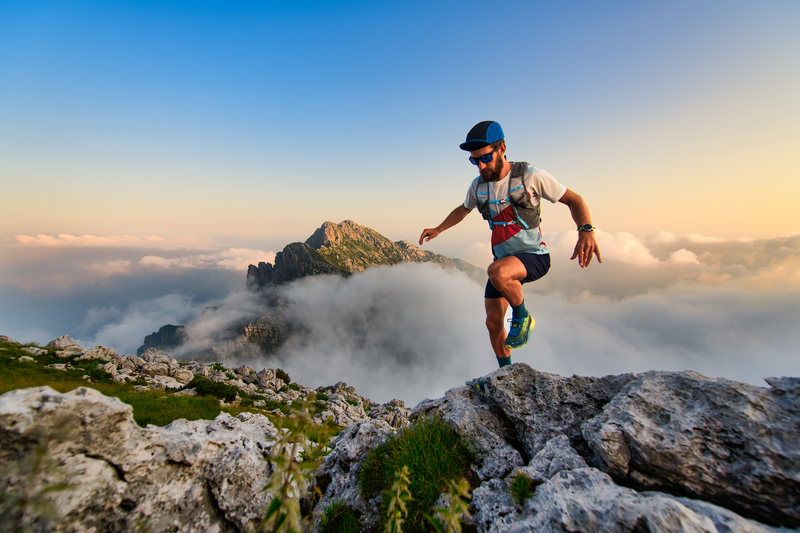
94% of researchers rate our articles as excellent or good
Learn more about the work of our research integrity team to safeguard the quality of each article we publish.
Find out more
ORIGINAL RESEARCH article
Front. Psychol.
Sec. Organizational Psychology
Volume 16 - 2025 | doi: 10.3389/fpsyg.2025.1557898
The final, formatted version of the article will be published soon.
You have multiple emails registered with Frontiers:
Please enter your email address:
If you already have an account, please login
You don't have a Frontiers account ? You can register here
factors at Time 2 and Time 4. Sample: A total of 272 employees from several Norwegian organisations participated in the study. Results: The results provided evidence of a reciprocal longitudinal negative path coefficient between sickness and experienced job resources, while there was a small and non-significant positive path coefficient on job demands. Evidence of a cyclical reciprocal effect with a sickness absence -> job resources -> burnout -> sickness absence pathway was also found thus supporting the JD-R model's ability to predict sickness absenteeism. This implies that sickness absence may lead to an experienced loss of job resources which again exacerbates burnout thus resulting in sickness absence at a later point in time. Contribution: This paper contributes to psychological theory by adding knowledge of the longitudinal and reciprocal effects of sickness absence on the experience job characteristics, as well as adding to the longitudinal data base showing the longitudinal predictive effect of burnout on sickness absence.
Keywords: sickness absenteeism, job demands, Job resources, exhaustion, Longitudinal, Path analysis
Received: 09 Jan 2025; Accepted: 19 Mar 2025.
Copyright: © 2025 Gottenborg, Hoff, Johnsen, Rydstedt and Øvergård. This is an open-access article distributed under the terms of the Creative Commons Attribution License (CC BY). The use, distribution or reproduction in other forums is permitted, provided the original author(s) or licensor are credited and that the original publication in this journal is cited, in accordance with accepted academic practice. No use, distribution or reproduction is permitted which does not comply with these terms.
* Correspondence:
Kjell Ivar Øvergård, Department of Health-, Social-, and Welfare Studies, Faculty of Health and Social Sciences, University of South-Eastern Norway, Kongsberg, Norway
Disclaimer: All claims expressed in this article are solely those of the authors and do not necessarily represent those of their affiliated organizations, or those of the publisher, the editors and the reviewers. Any product that may be evaluated in this article or claim that may be made by its manufacturer is not guaranteed or endorsed by the publisher.
Research integrity at Frontiers
Learn more about the work of our research integrity team to safeguard the quality of each article we publish.