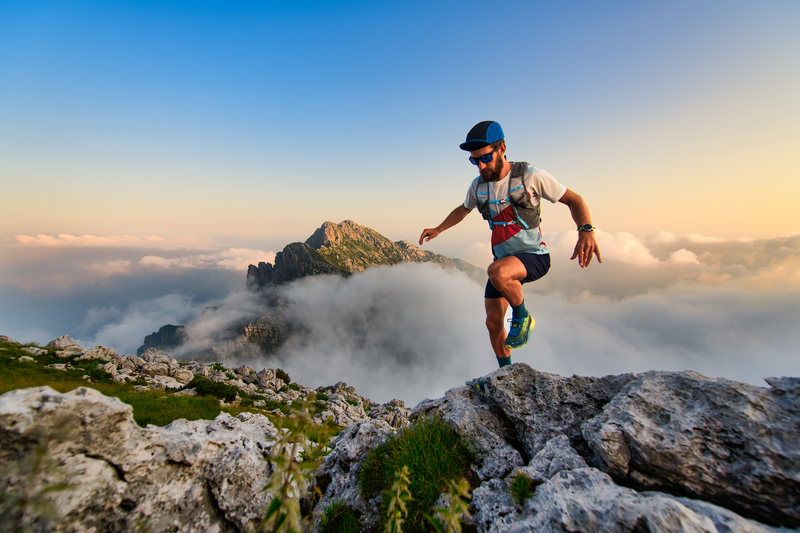
95% of researchers rate our articles as excellent or good
Learn more about the work of our research integrity team to safeguard the quality of each article we publish.
Find out more
OPINION article
Front. Psychol.
Sec. Educational Psychology
Volume 16 - 2025 | doi: 10.3389/fpsyg.2025.1550621
This article is part of the Research Topic The Convergence of Cognitive Neuroscience and Artificial Intelligence: Unraveling the Mysteries of Emotion, Perception, and Human Cognition View all articles
The final, formatted version of the article will be published soon.
You have multiple emails registered with Frontiers:
Please enter your email address:
If you already have an account, please login
You don't have a Frontiers account ? You can register here
problems in student retention and critical thinking in 206 vocational education students in the Akwa Ibom State, Nigeria. The results showed that AI posed significant threats to the male students showing more concern than their female peers. While AI aids vocational education, it has the potential to reduce cognitive engagement because the students may accept passively the information provided by AI without critical scrutiny (Ododo et al., 2024). The study emphasizes the need for AI-powered learning platforms that encourage source verification and independent thinking, ensuring that the students learn critically rather than passively.Creativity: Another area of serious concern is the impact of AI on creativity. An experiment on undergraduate students who enrolled for a Creative Thinking & Problem-Solving class at a leading Southeastern United States university studied the impact that AI tools, including ChatGPT-3, had on the divergent thinking ability of the students. Conducting a mixed-methods design using the Alternative Uses Task (AUT), the researchers found that AI-supported students scored better on fluency, flexibility, and elaboration when thinking up ideas but also found that the use of AI had liabilities, including cognitive fixation and lower creative confidence as the students over-relied on AI suggestions (Habib et al., 2024). These findings underscore the need to use AI in learning environments in a manner that maximizes its strengths and minimizes its liabilities so that AI becomes an enabler, not a constraint, on creative potential.To better understand the relationship between AI in education and key psychological and cognitive theories, the following table categorizes AI applications based on their alignment with Cognitive Load Theory, Bloom's Taxonomy, and Self-Determination Theory.Cognitive Load Theory (Schnotz & Kürschner, 2007) provides valuable insights into the manner in which AI impacts learning. AI has the potential to maximize the extraneous load through the elimination of redundant work, allowing the student to focus on more important cognitive operations. However, over-reliance on AI has the potential to reduce the germane load, which is needed for deep learning and the development of higher-order thinking skills. For instance, even as adaptive AI has the potential to personalize learning experiences and make difficult concepts easier, it must be done in a way that promotes effortful engagement rather than passive reception of information.Bloom's Taxonomy: Bloom's Taxonomy (Shaikh et al., 2021) categorizes cognitive skills from simple recall to higher-order thinking. The role of AI varies at various levels. AI applications may enhance recall and synthesis of information (lower-order skills), but the risk that AI may stifle more judgmental and analytical skills (higher-order thinking) exists. If students overuse AIprovided answers, their skill to develop independent critical thinking skills may be impaired. Therefore, AI implementation must be made in a way that enhances higher-order thinking along with the strengths of AI to automate lower-order thinking skills.Self-Determination Theory (Ryan & Deci, 2000) points to three psychological needs-autonomy, competence, and relatedness-that are critical to motivation and learning. AI's role in the academic environment has to be in harmony with these principles. While AI has the potential to create competence through customized learning, excessive dependence on AI could compromise autonomy. AI-based learning also has to provide human interaction and guidance to prevent the erosion of relatedness, keeping the students socially and emotionally engaged in the learning process.To make sure that AI enhances learning without replacing necessary thinking functions, a wellplanned implementation roadmap has to be adopted. Firstly, educators must do a needs analysis to determine where AI can be integrated and align with learning goals for cognitive functions. Secondly, selection of AI has to be thoughtful, with selected tools facilitating engagement and not dependency. Thirdly, AI has to be integrated in the classroom in a balanced manner, with space for individualized guidance but with teacher-driven conversations. And finally, monitoring and feedback mechanisms need to be instituted to measure the impact on student learning and make necessary adjustments accordingly.AI-Powered Adaptive Learning in STEM: AI tutoring has increased student performance on standardized tests. Stanford research found a 15% increase in scores for students using AI platforms compared to traditional instruction (Top 6 AI Tools Revolutionizing Math Tutoring Techniques, n.d.). However, concerns remain about the building of conceptual understanding. Research by the University of Pennsylvania with Turkish high school students found that those using ChatGPT to practice performed worse on exams when compared with those who did not use the technology. While AI-assisted students answered correctly 48% more problems, their score on a concept understanding test was 17% lower (Barshay, 2024). These findings show that AI enhances procedural skill but does not necessarily create deeper learning. For optimal benefit, AIbased tutoring must be integrated with pedagogy that encourages critical thinking and active engagement.The application of AI-based software has been found to be immensely useful in language learning. At the University of Baghdad, research centered on the contribution provided by AI towards the teaching of the English language, with the focus being on its contribution towards the performances of the students. The findings indicated that AI-based software, including speech recognition, chatbots, and virtual tutors, contributed immensely towards the communicative competence and fluency of the students. Additionally, more than 80% of the respondents believed that AI would be a major contributor towards language teaching in the future. While AI provided individualized teaching and real-time feedback, the research also indicated the need for human interaction for enhanced concept formation and engagement. Some students believed that additional training would be necessary to ensure the maximum utilization of AI in language learning. These findings reflect the reality that even as AI might be a value addition to language acquisition, it must be an addition to the traditional learning process and not a replacement for human teaching.While AI has much to offer the learning process, it also presents real threats that must be addressed in order to use it responsibly. Most significant among these threats is the danger of over-reliance on AI, which lowers the quality and extent of mental work as well as the quality of the ability to solve problems. If students over-rely on AI-based answers, they might bypass necessary mental operations like critical thinking and self-reasoning. In order to counter this threat, instructors must incorporate reflection points, where students must describe AI-given answers in their own language. Periodic AI-free solving sessions and class discussions with fellow classmates also ensure active mental engagement, making sure that AI becomes an add-on, and not a replacement, for self-reasoning.Another vital challenge is algorithmic bias, where AI models trained on unbalanced datasets could perpetuate educational inequalities unwittingly. This becomes a major issue when AI-based assessments or grading software favor some populations based on unbalanced data. To counter this, designers must include transparency mechanisms in the creation of AI models and diversify the datasets through the addition of multilingual and multicultural inputs. Furthermore, the implementation of fairness-aware AI algorithms has the potential to reduce bias, with the effect that AI-based educational software increases inclusiveness and does not perpetuate inequalities.A third concern is the risk that students who are highly reliant on AI-based learning experiences may lose motivation. If the thinking is done by AI, the students may lose motivation and engagement as well as the intrinsic motivation to learn and solve problems independently. To counter this, AI should be designed to develop autonomy through interactive, self-directed challenges. Gamification modules, adaptive problem-solving, and decision-making and creative input-requiring AI simulations can ensure motivation with the promise that the students are active agents in their learning process. AI should be a cognitive enabler that encourages deeper engagement and not a passive repository of knowledge.The application of AI in learning has a paradoxical character because it has the capability to be both a cognitive amplifier and inhibitor. In order to be maximally beneficial, AI must be designed as an enabler, rather than a substitute, fostering intellectual autonomy, problem-solving, and deep learning. According to Cognitive Load Theory, AI must decrease cognitive overload but sustain active cognitive engagement. According to Bloom's Taxonomy, AI must aid higher-order thinking and not enhance recall alone. Additionally, according to Self-Determination Theory, AI must generate autonomy and competence and not dependency. AI should complement and not replace human instruction, with students doing higher-order thinking as the efficiency and personalization strengths of AI are leveraged. This calls on teachers to implement AI in a way that enhances self-directed learning and metacognitive thinking. AI designers and policymakers must ensure equity, transparency, and explainability to prevent biases in algorithms and make AI accessible to all students on the same level playing field.Future research should consider the long-term impact of AI on thinking development, specifically on the storage of memories, critical thinking, and motivation in students. As AI reshapes the learning process, it becomes imperative to create pedagogically informed AI integration strategies that balance the efficiency provided by technology with the thinking skills that are necessary for meaningful learning. Maintaining AI-based learning as a force for empowerment, rather than dependency, will be crucial in the development of the future of learning.
Keywords: Artificial intelligence in education, Cognitive Load Theory, AI and Critical Thinking, self-determination theory, AI-driven Learning Strategies
Received: 23 Dec 2024; Accepted: 31 Mar 2025.
Copyright: © 2025 Jose, Cherian, Verghis, Varghise, S and Joseph. This is an open-access article distributed under the terms of the Creative Commons Attribution License (CC BY). The use, distribution or reproduction in other forums is permitted, provided the original author(s) or licensor are credited and that the original publication in this journal is cited, in accordance with accepted academic practice. No use, distribution or reproduction is permitted which does not comply with these terms.
* Correspondence:
Binny Jose, Department of Health and Wellness, Marian College Kuttikkanam Autonomous, Kuttikkanam, India
Disclaimer: All claims expressed in this article are solely those of the authors and do not necessarily represent those of their affiliated organizations, or those of the publisher, the editors and the reviewers. Any product that may be evaluated in this article or claim that may be made by its manufacturer is not guaranteed or endorsed by the publisher.
Research integrity at Frontiers
Learn more about the work of our research integrity team to safeguard the quality of each article we publish.