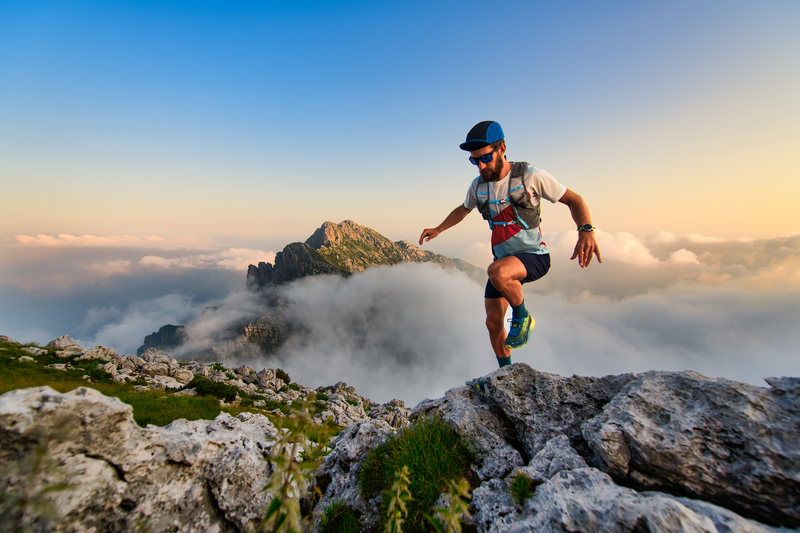
94% of researchers rate our articles as excellent or good
Learn more about the work of our research integrity team to safeguard the quality of each article we publish.
Find out more
REVIEW article
Front. Psychol.
Sec. Educational Psychology
Volume 16 - 2025 | doi: 10.3389/fpsyg.2025.1542652
The final, formatted version of the article will be published soon.
You have multiple emails registered with Frontiers:
Please enter your email address:
If you already have an account, please login
You don't have a Frontiers account ? You can register here
Since the onset of the COVID-19 pandemic in early 2020, online learning has gained widespread adoption as a learning mode in both K-12 and higher education. Learning engagement serves as a crucial indicator of learning quality and is highly correlated with students' persistence, satisfaction, and academic performance. Numerous researchers have conducted investigations into the factors that influence online learning engagement. This study employs a systematic literature review methodology to synthesize 55 empirical studies published between January 2020 and July 2023. The research findings reveal the following: (1
Keywords: ) Community of Inquiry Theory, Selfdetermination theory, Social cognition theory, Transaction Distance Theory, Digital Experience and Literacy, Emotions and Regulatory Strategies, Psychology, self-perception
Received: 10 Dec 2024; Accepted: 03 Mar 2025.
Copyright: © 2025 HU and Wen. This is an open-access article distributed under the terms of the Creative Commons Attribution License (CC BY). The use, distribution or reproduction in other forums is permitted, provided the original author(s) or licensor are credited and that the original publication in this journal is cited, in accordance with accepted academic practice. No use, distribution or reproduction is permitted which does not comply with these terms.
* Correspondence:
Juan HU, Anhui Institute of Information Technology, Wuhu, China
Disclaimer: All claims expressed in this article are solely those of the authors and do not necessarily represent those of their affiliated organizations, or those of the publisher, the editors and the reviewers. Any product that may be evaluated in this article or claim that may be made by its manufacturer is not guaranteed or endorsed by the publisher.
Research integrity at Frontiers
Learn more about the work of our research integrity team to safeguard the quality of each article we publish.