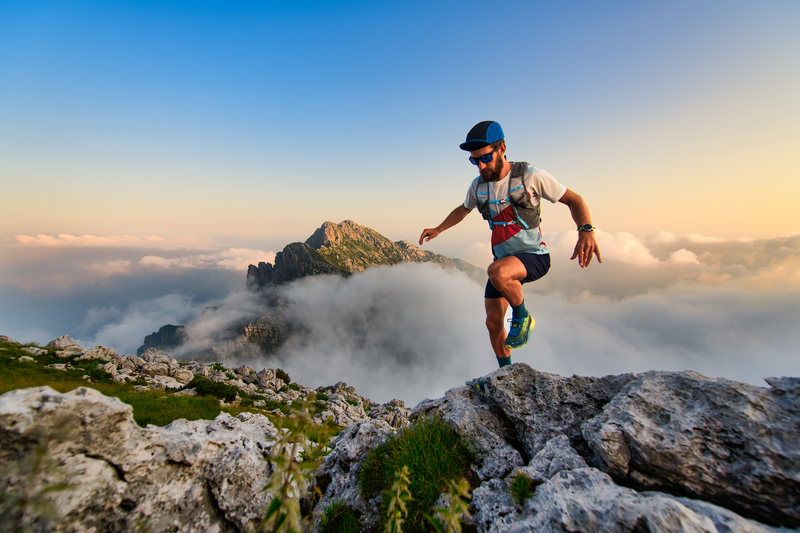
94% of researchers rate our articles as excellent or good
Learn more about the work of our research integrity team to safeguard the quality of each article we publish.
Find out more
ORIGINAL RESEARCH article
Front. Psychol.
Sec. Auditory Cognitive Neuroscience
Volume 16 - 2025 | doi: 10.3389/fpsyg.2025.1538511
The final, formatted version of the article will be published soon.
You have multiple emails registered with Frontiers:
Please enter your email address:
If you already have an account, please login
You don't have a Frontiers account ? You can register here
This study investigates the interactions between musicianship and two auditory cognitive processes: auditory working memory (AWM) and stream segregation. The primary hypothesis is that AWM could mediate the relationship between musical training and enhanced stream segregation capabilities. Two groups of listeners were tested: the first aimed to establish the relationship between the three variables, and the second aimed to replicate the effect in an independent sample. Music experience history and behavioural data were collected from a total of 145 healthy young adults with normal binaural hearing. The AWM task involved the manipulation of tonal patterns in working memory, while the Music-in-Noise Task (MINT) measured stream segregation abilities in a tonal context. The MINT expands measurements beyond traditional Speech-in-Noise assessments by capturing auditory subskills (rhythm, visual, spatial attention, prediction) relevant to stream segregation. Our results showed that musical training is associated with enhanced AWM and MINT performance and that this effect is replicable across independent samples. Moreover, we found in both samples that the enhancement of stream segregation was largely mediated by AWM capacity. The results suggest that musical training and/or aptitude enhances stream segregation by way of improved AWM capacity.
Keywords: auditory stream segregation, auditory working memory, Hearing-in-noise, musical training, music perception
Received: 02 Dec 2024; Accepted: 25 Feb 2025.
Copyright: © 2025 Liu, Arseneau-Bruneau, Farrés Franch, Latorre, Samuels, Issa, Payumo, Rahman, Loureiro, Leung, Nave, Von Handorf, Hoddinott, Coffey, Grahn and Zatorre. This is an open-access article distributed under the terms of the Creative Commons Attribution License (CC BY). The use, distribution or reproduction in other forums is permitted, provided the original author(s) or licensor are credited and that the original publication in this journal is cited, in accordance with accepted academic practice. No use, distribution or reproduction is permitted which does not comply with these terms.
* Correspondence:
Martha Liu, Montreal Neurological Institute, McGill University Health Centre, Montreal, Canada
Robert J Zatorre, Montreal Neurological Institute, McGill University Health Centre, Montreal, Canada
Disclaimer: All claims expressed in this article are solely those of the authors and do not necessarily represent those of their affiliated organizations, or those of the publisher, the editors and the reviewers. Any product that may be evaluated in this article or claim that may be made by its manufacturer is not guaranteed or endorsed by the publisher.
Research integrity at Frontiers
Learn more about the work of our research integrity team to safeguard the quality of each article we publish.