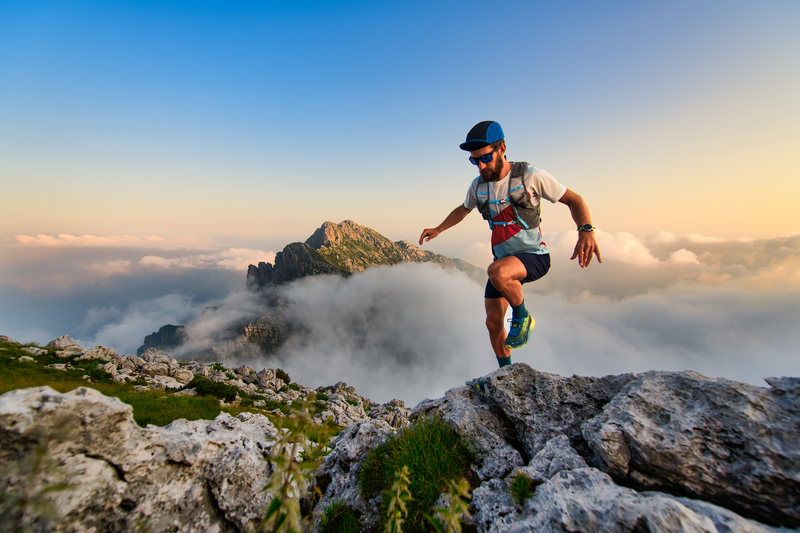
94% of researchers rate our articles as excellent or good
Learn more about the work of our research integrity team to safeguard the quality of each article we publish.
Find out more
ORIGINAL RESEARCH article
Front. Psychol.
Sec. Cognition
Volume 16 - 2025 | doi: 10.3389/fpsyg.2025.1534224
The final, formatted version of the article will be published soon.
You have multiple emails registered with Frontiers:
Please enter your email address:
If you already have an account, please login
You don't have a Frontiers account ? You can register here
Background: Effective emergency response in various industries depends on the synergy between team coordination and cognitive abilities. Industries should prioritize investing in the development of team cognition to improve readiness and ensure swift, effective responses to emergencies and crises. This study aimed to identify and model factors influencing team cognition within Emergency Re-sponse Teams (ERTs). Methods: This cross-sectional study undertook two principal phases: qualitative research using meta-synthesis and quantitative research using Best Worst Method (BWM), Interpretive Structural Model-ing (ISM), and Fuzzy Cognitive Mapping (FCM). These methods were employed to assign weights to factors, establish their hierarchy, and determine cause-and-effect relationships among team cognition shaping factors (TCSFs). Results: Through a comprehensive evaluation of the articles, 13 dimensions were identified as the primary TCSFs influencing team cognition. The reliability of the extracted factors was validated us-ing the Kappa indicator, with a value of 0.63 signifying an acceptable level of agreement. Using BWM analysis, "Team maturity (The team members' harmonization)" and "Inefficient 4Cs (commu-nication, coordination, cooperation, and collaboration)" were identified as the most influential factors shaping team cognition, with weights of 0.132 and 0.112, respectively. ISM analysis revealed "Im-proper team training programs" as a critical independent factor influencing other dimensions. FCM modeling further emphasized the significance of "Failure in decision-making" and "Leadership be-havior and performance" as pivotal contributors to team cognition, with "Team maturity" and "Ineffi-cient 4Cs" achieving the highest centrality scores of 13.44 and 13.28, respectively. Conclusion: Stakeholders can enhance team performance and effectiveness in emergency situations by understanding the relative importance of various factors, their hierarchical relationships, and the causal links between them. This allows for informed decision-making and targeted interventions, such as training programs to improve team maturity and team communication.
Keywords: team cognition1, emergency response team2, meta-synthesis3, weighting4, modelling5
Received: 09 Dec 2024; Accepted: 05 Mar 2025.
Copyright: © 2025 Esmaeili, Yazdi, Rismanchian and Shakerian. This is an open-access article distributed under the terms of the Creative Commons Attribution License (CC BY). The use, distribution or reproduction in other forums is permitted, provided the original author(s) or licensor are credited and that the original publication in this journal is cited, in accordance with accepted academic practice. No use, distribution or reproduction is permitted which does not comply with these terms.
* Correspondence:
Mahnaz Shakerian, Department of Occupational Health and Safety Engineering, School of Health, Isfahan University of Medical Sciences, Isfahan, Iran
Disclaimer: All claims expressed in this article are solely those of the authors and do not necessarily represent those of their affiliated organizations, or those of the publisher, the editors and the reviewers. Any product that may be evaluated in this article or claim that may be made by its manufacturer is not guaranteed or endorsed by the publisher.
Research integrity at Frontiers
Learn more about the work of our research integrity team to safeguard the quality of each article we publish.