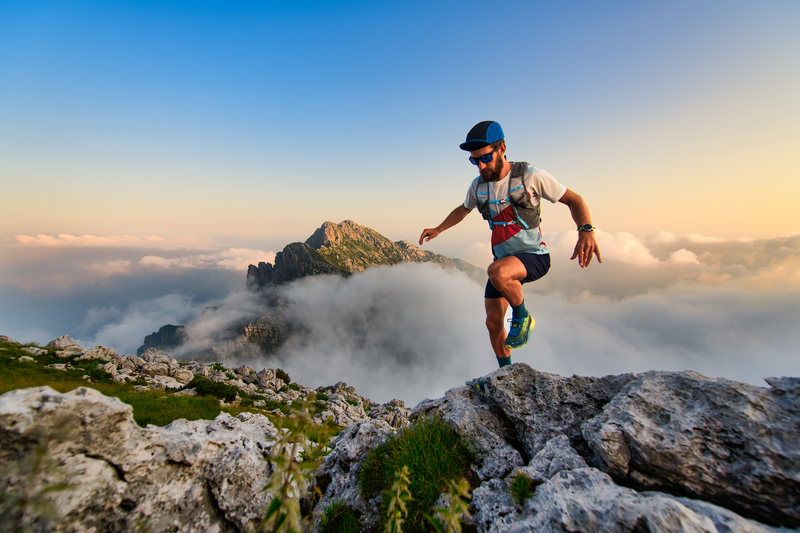
94% of researchers rate our articles as excellent or good
Learn more about the work of our research integrity team to safeguard the quality of each article we publish.
Find out more
ORIGINAL RESEARCH article
Front. Psychol.
Sec. Psychology of Language
Volume 16 - 2025 | doi: 10.3389/fpsyg.2025.1520111
The final, formatted version of the article will be published soon.
You have multiple emails registered with Frontiers:
Please enter your email address:
If you already have an account, please login
You don't have a Frontiers account ? You can register here
To develop AI tools that can communicate on par with human speakers and listeners, we need a deeper understanding of the factors that affect their perception and production of spoken language. Thus, the goal of this study was to examine to what extent two AI tools, Amazon Alexa and Polly, are impacted by factors that are known to modulate speech perception and production in humans. In particular, we examined the role of lexical (word frequency, phonological neighborhood density) and stylistic (speaking rate) factors. In the domain of perception, high-frequency words and slow speaking rate significantly improved Alexa's recognition of words produced in real time by native speakers of American English (n=21). Alexa also recognized words with low neighborhood density with greater accuracy, but only at fast speaking rates. In contrast to human listeners, Alexa showed no evidence of adaptation to the speaker over time. In the domain of production, Polly's vowel duration and formants were unaffected by the lexical characteristics of words, unlike human speakers. Overall, these findings suggest that, despite certain patterns that humans and AI tools share, AI tools lack some of the flexibility that is the hallmark of human speech perception and production.
Keywords: artificial intelligence, speech recognition, word frequency, neighborhood density, Speaking rate, adaptation 1. Introduction
Received: 30 Oct 2024; Accepted: 25 Feb 2025.
Copyright: © 2025 Song, Rojas and Pycha. This is an open-access article distributed under the terms of the Creative Commons Attribution License (CC BY). The use, distribution or reproduction in other forums is permitted, provided the original author(s) or licensor are credited and that the original publication in this journal is cited, in accordance with accepted academic practice. No use, distribution or reproduction is permitted which does not comply with these terms.
* Correspondence:
Anne Pycha, University of Wisconsin–Milwaukee, Milwaukee, United States
Disclaimer: All claims expressed in this article are solely those of the authors and do not necessarily represent those of their affiliated organizations, or those of the publisher, the editors and the reviewers. Any product that may be evaluated in this article or claim that may be made by its manufacturer is not guaranteed or endorsed by the publisher.
Research integrity at Frontiers
Learn more about the work of our research integrity team to safeguard the quality of each article we publish.