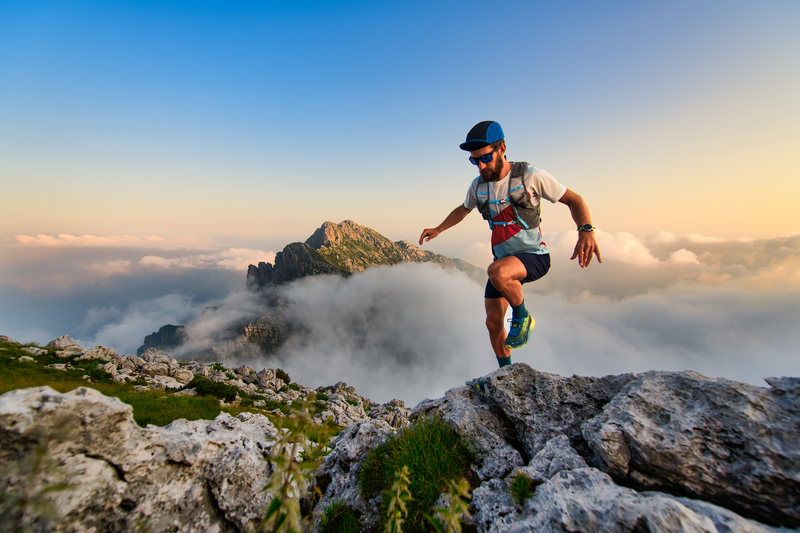
94% of researchers rate our articles as excellent or good
Learn more about the work of our research integrity team to safeguard the quality of each article we publish.
Find out more
ORIGINAL RESEARCH article
Front. Psychol.
Sec. Quantitative Psychology and Measurement
Volume 16 - 2025 | doi: 10.3389/fpsyg.2025.1517623
The final, formatted version of the article will be published soon.
You have multiple emails registered with Frontiers:
Please enter your email address:
If you already have an account, please login
You don't have a Frontiers account ? You can register here
Although several factor analytic studies have investigated the factor structure of the Personality Assessment Inventory (PAI), no consensus has been reached regarding the best factor solution, either considering the 11 clinical scales or the 22 full scales. Whereas some studies have found that a two-factor solution for the clinical scales and a four-factor solution for the 22 scales were the most parsimonious factor structures, other studies suggested different factor models (e.g., a three-factor solution for the 11 clinical scales, and a three-or five-factor solution for the 22 full scales). The present study aimed to investigate the factor structure of the Portuguese version of the PAI in a sample of 900 participants (aged 18-74 years, 57.7% female). Several factor models (uncorrelated, correlated, hierarchical, and bifactor) were examined, and the results supported a bifactor model with two first-order factors for the 11 clinical scales (the bifactor model with three first-order factor also revealed an adequate fit) and a three-factor model for the 22 full scales. Comparisons between the Portuguese factor structure and other international versions of the PAI were examined. Implications and directions for future research on PAI's dimensionality are discussed.
Keywords: Personality Assessment Inventory (PAI), confirmatory factor analysis, Principal Component Analysis, Portuguese version, psychometric properties
Received: 20 Nov 2024; Accepted: 24 Feb 2025.
Copyright: © 2025 Paulino, Moniz, Moura, Rijo, Morey and Simões. This is an open-access article distributed under the terms of the Creative Commons Attribution License (CC BY). The use, distribution or reproduction in other forums is permitted, provided the original author(s) or licensor are credited and that the original publication in this journal is cited, in accordance with accepted academic practice. No use, distribution or reproduction is permitted which does not comply with these terms.
* Correspondence:
Mauro Paulino, Center for Research in Neuropsychology and Cognitive and Behavioral Intervention, University of Coimbra, Coimbra, Portugal
Disclaimer: All claims expressed in this article are solely those of the authors and do not necessarily represent those of their affiliated organizations, or those of the publisher, the editors and the reviewers. Any product that may be evaluated in this article or claim that may be made by its manufacturer is not guaranteed or endorsed by the publisher.
Research integrity at Frontiers
Learn more about the work of our research integrity team to safeguard the quality of each article we publish.