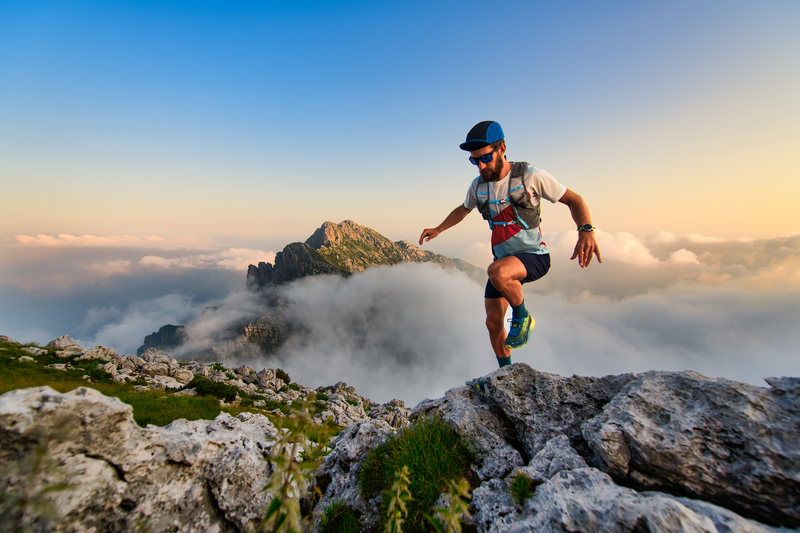
94% of researchers rate our articles as excellent or good
Learn more about the work of our research integrity team to safeguard the quality of each article we publish.
Find out more
ORIGINAL RESEARCH article
Front. Psychol.
Sec. Psychology of Aging
Volume 16 - 2025 | doi: 10.3389/fpsyg.2025.1507178
The final, formatted version of the article will be published soon.
You have multiple emails registered with Frontiers:
Please enter your email address:
If you already have an account, please login
You don't have a Frontiers account ? You can register here
Social isolation and loneliness are two of the main causes of mental health issues or suicide not only in younger adults but also in older adults. Thus, identifying an effective method to detect social isolation is important in the field of human-machine interaction. However, to our knowledge, no effective method has been developed to elicit pseudo-social isolation tasks to evaluate social isolation detection systems for older adults.This study has two research aims: 1. To develop the virtual avatar conversation cyberball task to evoke pseudo-social isolation in older adults and, 2. To identify non-verbal indicators replacing social isolation in older adults. To reach the aims, twenty-two older men were recruited as participants. They were asked to communicate with two virtual avatars on a monitor, and then to rate the follow-up questions provided in order to evaluate the level of social isolation and emotions; meanwhile, facial expressions and gaze patterns were recorded by a camera and an eye tracker. In the results, the developed virtual avatar conversation cyberball task was shown to successfully induce pseudo-social isolation in older adults, and this social isolation can be detected by the intensity of inner/outer eyebrow and eyelid movements and the blink frequency.
Keywords: older adults, Social Isolation, Virtual Avatar Interaction, Facial Recognition System, Cyber-Ball Task
Received: 08 Oct 2024; Accepted: 21 Feb 2025.
Copyright: © 2025 Takemoto, Iwamoto, Yaegashi, Yun and Takashima. This is an open-access article distributed under the terms of the Creative Commons Attribution License (CC BY). The use, distribution or reproduction in other forums is permitted, provided the original author(s) or licensor are credited and that the original publication in this journal is cited, in accordance with accepted academic practice. No use, distribution or reproduction is permitted which does not comply with these terms.
* Correspondence:
Ayumi Takemoto, Tohoku University, Sendai, Japan
Disclaimer: All claims expressed in this article are solely those of the authors and do not necessarily represent those of their affiliated organizations, or those of the publisher, the editors and the reviewers. Any product that may be evaluated in this article or claim that may be made by its manufacturer is not guaranteed or endorsed by the publisher.
Research integrity at Frontiers
Learn more about the work of our research integrity team to safeguard the quality of each article we publish.