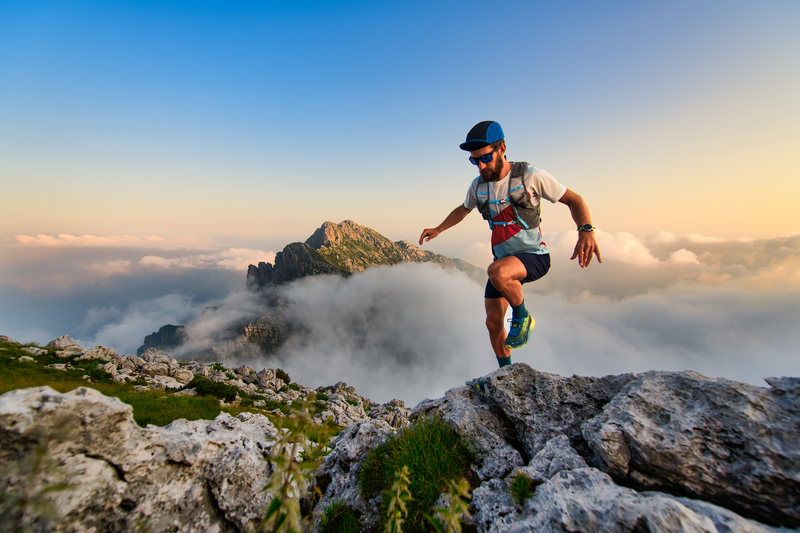
94% of researchers rate our articles as excellent or good
Learn more about the work of our research integrity team to safeguard the quality of each article we publish.
Find out more
ORIGINAL RESEARCH article
Front. Psychol.
Sec. Personality and Social Psychology
Volume 16 - 2025 | doi: 10.3389/fpsyg.2025.1500674
The final, formatted version of the article will be published soon.
You have multiple emails registered with Frontiers:
Please enter your email address:
If you already have an account, please login
You don't have a Frontiers account ? You can register here
Despite its relevance for health outcomes, only recently gender has begun to be explored in the scientific literature, typically using a rigid binary framework. To tackle this, the Stanford Gender-related Variables for Health Research (GVHR) was developed to analyze gender from a multidimensional perspective. We aim to use our Portuguese version of the GVHR and analyze its association with health outcomes, including perceived stress. To this aim, 351 participants completed the GVHR scale, sociodemographic, and health information (including the Perceived Stress Scale, PSS-10). A Confirmatory Factor Analyses (CFA) was first performed, and logistic and linear regressions were used to explore the association between gender and health-related variables. All measures of CFA showed appropriate goodness of fit. Regarding regression models, gender discrimination and higher levels of risk-taking were associated with binge drinking. Lower social support and risk-taking, and being male, were associated with being overweight. Regarding stress, it was positively associated with discrimination and work strain, while it was negatively associated with social support, emotional intelligence and risk-taking. Finally, discrimination and work strain were positively associated with mental health worsening and activity limitations, while social support was negatively associated with mental health worsening. In conclusion, by approaching gender from a multidimensional perspective we detected specific factors influencing health outcomes, showing that the relational aspects of gender are particularly relevant for mental health.
Keywords: gender, stress, Mental Health, Discrimination, social support
Received: 23 Sep 2024; Accepted: 12 Feb 2025.
Copyright: © 2025 Picó-Pérez, Coelho, Vieira, Machado-Sousa and Morgado. This is an open-access article distributed under the terms of the Creative Commons Attribution License (CC BY). The use, distribution or reproduction in other forums is permitted, provided the original author(s) or licensor are credited and that the original publication in this journal is cited, in accordance with accepted academic practice. No use, distribution or reproduction is permitted which does not comply with these terms.
* Correspondence:
Maria Picó-Pérez, Department of Basic Psychology, Clinic and Psychobiology, Jaume I University, Castelló de La Plana, Spain
Disclaimer: All claims expressed in this article are solely those of the authors and do not necessarily represent those of their affiliated organizations, or those of the publisher, the editors and the reviewers. Any product that may be evaluated in this article or claim that may be made by its manufacturer is not guaranteed or endorsed by the publisher.
Research integrity at Frontiers
Learn more about the work of our research integrity team to safeguard the quality of each article we publish.