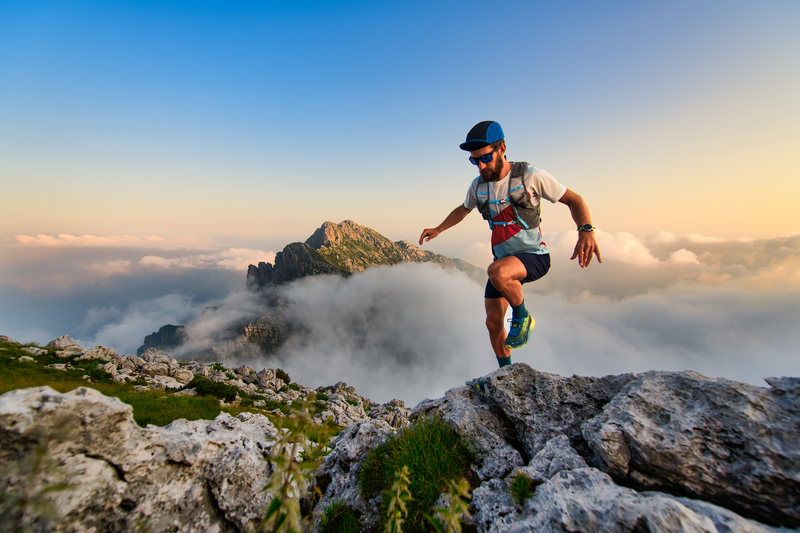
94% of researchers rate our articles as excellent or good
Learn more about the work of our research integrity team to safeguard the quality of each article we publish.
Find out more
ORIGINAL RESEARCH article
Front. Psychol.
Sec. Psychopathology
Volume 16 - 2025 | doi: 10.3389/fpsyg.2025.1500200
The final, formatted version of the article will be published soon.
You have multiple emails registered with Frontiers:
Please enter your email address:
If you already have an account, please login
You don't have a Frontiers account ? You can register here
Background: In a recent study of Norwegian children (N = 874), Halse et al. used randomintercept cross-lagged panel models (RI-CLPM) and concluded that their findings supported the assumption that deficiencies in executive functions and psychopathology are both a cause and a consequence of the other. However, it is known that RI-CLPM can give biased results.We reanalyzed data simulated to resemble the data used by Halse et al. with several complementary models, e.g., latent change score models (LCSM).The analyzed models indicated contradictory simultaneous increasing and decreasing effects between executive deficits and symptoms of psychopathology.The present contradictory findings suggested that prospective effects between executive deficits and symptoms of psychopathology may have been spurious rather than truly increasing. Consequently, conclusions by Halse et al. appear to have been premature. It is important for researchers to bear in mind that correlations, including cross-lagged effects in RI-CLPM, do not prove causality. Careful interpretation of RI-CLPM results is of utmost importance in, for example, research in clinical and developmental psychology. We recommend researchers to use, as we did here, triangulation to scrutinize findings from analyses of observational data.
Keywords: executive deficits, random-intercept cross-lagged panel models (RI-CLPM), Reanalyses, Spurious effects, Symptoms of psychopathology, Triangulation
Received: 22 Sep 2024; Accepted: 19 Feb 2025.
Copyright: © 2025 Sorjonen and Melin. This is an open-access article distributed under the terms of the Creative Commons Attribution License (CC BY). The use, distribution or reproduction in other forums is permitted, provided the original author(s) or licensor are credited and that the original publication in this journal is cited, in accordance with accepted academic practice. No use, distribution or reproduction is permitted which does not comply with these terms.
* Correspondence:
Kimmo Sorjonen, Karolinska Institutet (KI), Solna, Sweden
Disclaimer: All claims expressed in this article are solely those of the authors and do not necessarily represent those of their affiliated organizations, or those of the publisher, the editors and the reviewers. Any product that may be evaluated in this article or claim that may be made by its manufacturer is not guaranteed or endorsed by the publisher.
Research integrity at Frontiers
Learn more about the work of our research integrity team to safeguard the quality of each article we publish.