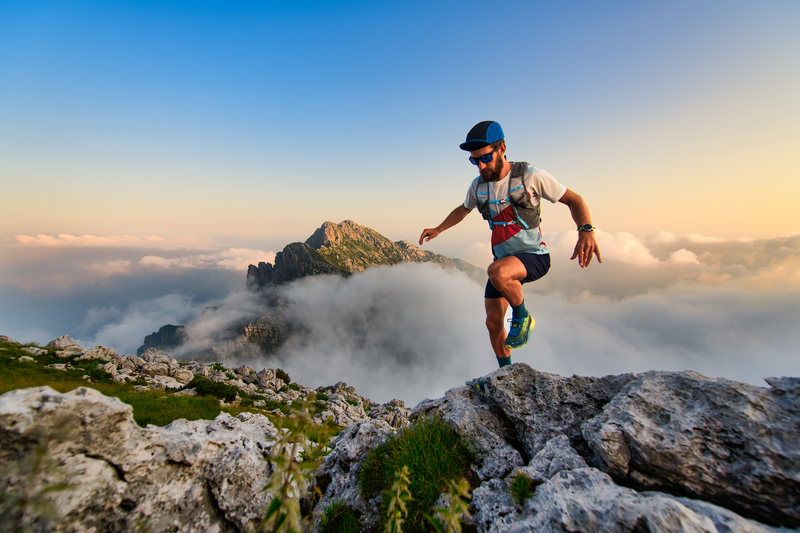
95% of researchers rate our articles as excellent or good
Learn more about the work of our research integrity team to safeguard the quality of each article we publish.
Find out more
ORIGINAL RESEARCH article
Front. Psychol.
Sec. Decision Neuroscience
Volume 16 - 2025 | doi: 10.3389/fpsyg.2025.1496013
The final, formatted version of the article will be published soon.
You have multiple emails registered with Frontiers:
Please enter your email address:
If you already have an account, please login
You don't have a Frontiers account ? You can register here
In recent years, the integration of electroencephalogram (EEG) and somatosensory data in athlete potential evaluation has garnered increasing attention. Traditional research methods mainly rely on processing EEG signals or motion sensor data independently. While these methods can provide a certain level of performance assessment, they often overlook the synergy between brain activity and physical movement, making it difficult to comprehensively capture an athlete's potential. Moreover, most existing approaches employ shallow models, which fail to fully exploit the temporal dependencies and cross-modal interactions within the data, leading to suboptimal accuracy and robustness in evaluation results. To address these issues, this paper proposes a Transformer-based model, SensoriMind-Trans Net, which combines EEG signals and somatosensory data. The model leverages a multi-layer Transformer network to capture the temporal dependencies of EEG signals and utilizes a somatosensory data feature extractor and cross-modal attention alignment mechanism to enhance the comprehensive evaluation of athletes' cognitive and motor abilities. Experiments conducted on four public datasets demonstrate that our model outperforms several existing state-of-the-art (SOTA) models in terms of accuracy, inference time, and computational efficiency, showcasing its broad applicability in athlete potential evaluation. This study offers a new solution for athlete data analysis and holds significant implications for future multimodal sports performance assessment.
Keywords: electroencephalogram (EEG), somatosensory data, transformer, athlete potential evaluation, cross-modal
Received: 13 Sep 2024; Accepted: 21 Mar 2025.
Copyright: © 2025 Huang. This is an open-access article distributed under the terms of the Creative Commons Attribution License (CC BY). The use, distribution or reproduction in other forums is permitted, provided the original author(s) or licensor are credited and that the original publication in this journal is cited, in accordance with accepted academic practice. No use, distribution or reproduction is permitted which does not comply with these terms.
* Correspondence:
Zhenying Huang, Ganzhou Vocational and Technical College, Ganzhou, China
Disclaimer: All claims expressed in this article are solely those of the authors and do not necessarily represent those of their affiliated organizations, or those of the publisher, the editors and the reviewers. Any product that may be evaluated in this article or claim that may be made by its manufacturer is not guaranteed or endorsed by the publisher.
Research integrity at Frontiers
Learn more about the work of our research integrity team to safeguard the quality of each article we publish.