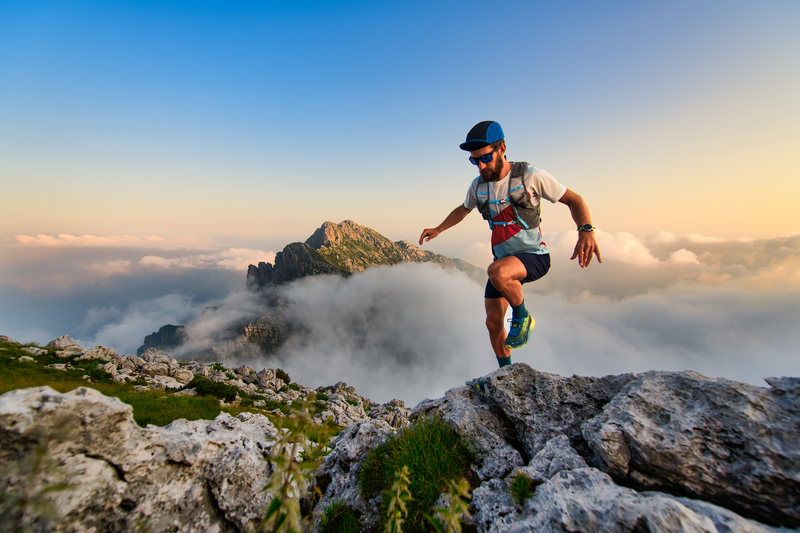
95% of researchers rate our articles as excellent or good
Learn more about the work of our research integrity team to safeguard the quality of each article we publish.
Find out more
ORIGINAL RESEARCH article
Front. Psychol.
Sec. Quantitative Psychology and Measurement
Volume 16 - 2025 | doi: 10.3389/fpsyg.2025.1487111
The final, formatted version of the article will be published soon.
You have multiple emails registered with Frontiers:
Please enter your email address:
If you already have an account, please login
You don't have a Frontiers account ? You can register here
The handling of missing data in cognitive diagnostic assessment is an important issue. You et al. (2023) proposed a Random Forest Threshold Imputation (RFTI) method specifically designed for cognitive diagnostic models (CDMs) and built on the random forest imputation. However, in RFTI, the threshold for determining imputed values to be 0 is fixed at 0.5, which may result in uncertainty in this imputation. To address this issue, we proposed an improved method, Random Forest Dynamic Threshold Imputation (RFDTI), which possess two dynamic thresholds for dichotomous imputed values. A simulation study showed that the classification of attribute profiles when using RFDTI to impute missing data was always better than the four commonly used traditional methods (i.e., person mean imputation, two-way imputation, expectation-maximization algorithm, and multiple imputation). Compared with RFTI, RFDTI was slightly better for MAR or MCAR data, but slightly worse for MNAR or MIXED data, especially with a larger missingness proportion. An empirical example with MNAR data demonstrates the applicability of RFDTI, which performed similarly as RFTI and much better than the other four traditional methods. An R package is provided to facilitate the application of the proposed method.
Keywords: missing data, cognitive diagnosis assessment, random forest threshold imputation, machine learning, Dynamic thresholds
Received: 28 Aug 2024; Accepted: 20 Mar 2025.
Copyright: © 2025 Xiaofeng, Xiao, Yang and Liu. This is an open-access article distributed under the terms of the Creative Commons Attribution License (CC BY). The use, distribution or reproduction in other forums is permitted, provided the original author(s) or licensor are credited and that the original publication in this journal is cited, in accordance with accepted academic practice. No use, distribution or reproduction is permitted which does not comply with these terms.
* Correspondence:
Hongyun Liu, Faculty of Psychology, Beijing Normal University, Beijing, 100875, Beijing, China
Disclaimer: All claims expressed in this article are solely those of the authors and do not necessarily represent those of their affiliated organizations, or those of the publisher, the editors and the reviewers. Any product that may be evaluated in this article or claim that may be made by its manufacturer is not guaranteed or endorsed by the publisher.
Research integrity at Frontiers
Learn more about the work of our research integrity team to safeguard the quality of each article we publish.