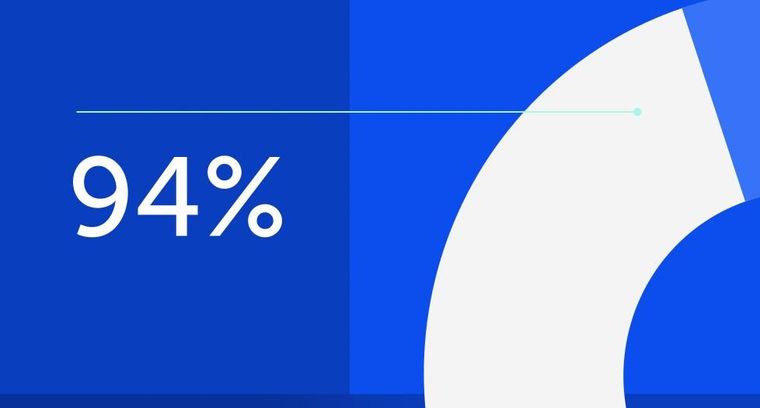
94% of researchers rate our articles as excellent or good
Learn more about the work of our research integrity team to safeguard the quality of each article we publish.
Find out more
ORIGINAL RESEARCH article
Front. Psychol.
Sec. Quantitative Psychology and Measurement
Volume 16 - 2025 | doi: 10.3389/fpsyg.2025.1474292
This article is part of the Research TopicMethodological and Statistical Advances in Educational AssessmentView all 10 articles
The final, formatted version of the article will be published soon.
Select one of your emails
You have multiple emails registered with Frontiers:
Notify me on publication
Please enter your email address:
If you already have an account, please login
You don't have a Frontiers account ? You can register here
Understanding individual cognitive profiles is crucial for developing personalized educational interventions, as cognitive differences can impact the way in which students learn. While traditional methods like Factor Mixture Modeling (FMM) have proven robust for identifying latent cognitive structures, recent deep learning advancements may offer the potential to capture more intricate and complex cognitive patterns. This study compares FMM (specifically, a FMM-1 and FMM-2 models with age as a covariate) with a Conditional Gaussian Mixture Variational Autoencoder (CGMVAE) using six cognitive dimensions obtained from the PROFFILO assessment game. Our results showed how the winner FMM-1 solution yielded two well-separated clusters (Silhouette score = 0.959) representing distinct average cognitive levels, with age significantly predicting class membership. In contrast, the CGMVAE identified ten more nuanced profiles exhibiting clear developmental trajectories across age groups. Notably, one dominant cluster (Cluster 9) increased in representation from 44% to 54% with age, highlighting a normative developmental pattern, while other clusters displayed diverse profiles. These ranged from subtle domain-specific strengths to atypical profiles characterized by significant deficits balanced by compensatory abilities. These findings highlight a trade-off: FMM provides clear, interpretable groupings suitable for broad classification, while CGMVAE reveals subtle, non-linear variations potentially reflecting complex developmental pathways. Despite practical challenges related to CGMVAE's complexity and cluster overlap, its capacity to uncover nuanced cognitive patterns shows promise for informing highly tailored educational strategies.. . .
Keywords: Variational autoencoders, clustering, machine learning, Cognitive profiles, Factor mixture modeling
Received: 01 Aug 2024; Accepted: 21 Apr 2025.
Copyright: © 2025 Orsoni, Giovagnoli, Garofalo, Mazzoni, Spinoso and Benassi. This is an open-access article distributed under the terms of the Creative Commons Attribution License (CC BY). The use, distribution or reproduction in other forums is permitted, provided the original author(s) or licensor are credited and that the original publication in this journal is cited, in accordance with accepted academic practice. No use, distribution or reproduction is permitted which does not comply with these terms.
* Correspondence: Matteo Orsoni, University of Bologna, Bologna, Italy
Disclaimer: All claims expressed in this article are solely those of the authors and do not necessarily represent those of their affiliated organizations, or those of the publisher, the editors and the reviewers. Any product that may be evaluated in this article or claim that may be made by its manufacturer is not guaranteed or endorsed by the publisher.
Supplementary Material
Research integrity at Frontiers
Learn more about the work of our research integrity team to safeguard the quality of each article we publish.