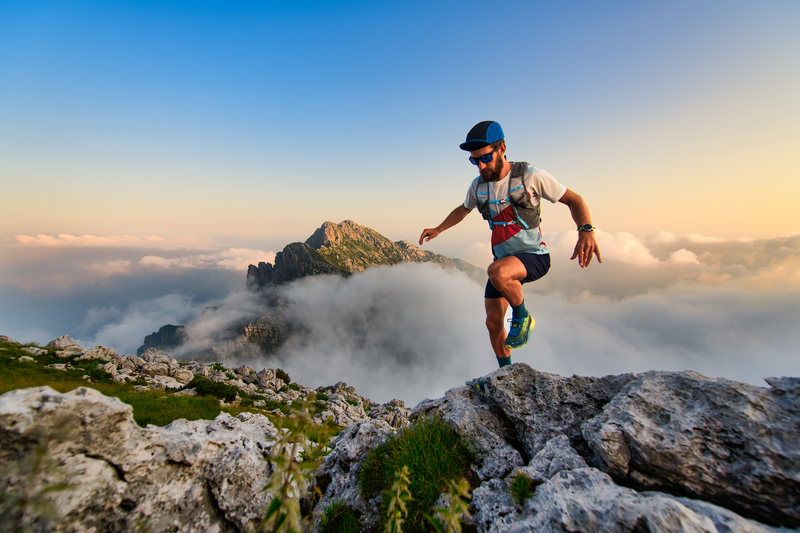
95% of researchers rate our articles as excellent or good
Learn more about the work of our research integrity team to safeguard the quality of each article we publish.
Find out more
BRIEF RESEARCH REPORT article
Front. Psychol. , 07 February 2025
Sec. Emotion Science
Volume 16 - 2025 | https://doi.org/10.3389/fpsyg.2025.1465774
Few studies have examined emotional intelligence (EI) following a person-centered approach to identify different types of EI profiles and their relationship to everyday life outcomes. Even rarer are those using an “ability” approach of EI (AEI) and related “performance-based” tests, which are considered promising. This study fills this gap by identifying AEI profiles and linking them to everyday outcomes such as health, wellbeing, and decision-making. The QEg (“QE” for Emotional Quotient - Quotient Emotional in French - and “g” for the general population), an ability-based measure of EI, along with other measures, was administered to 2,877 French adults. We then ran latent profile analysis (LPA) and identified three latent profiles within a heterogeneous population. The full emotion processing (FEP) profile outperforms the two others on key domains of life such as stress perception, home-work interaction, gratitude and satisfaction with life, emotional burnout prevention, and decision-making. Our research reveals the need for individualized AEI training programs tailored to three distinct profiles, addressing foundational skills for those with minimal or partial emotional processing while refining existing strengths for those with full emotional processing. Targeting interventions to specific profile characteristics could enhance the effectiveness of AEI training and promote improved wellbeing and life outcomes.
Emotional intelligence (EI) was originally conceptualized by Mayer and Salovey (1997) who defined it as “the ability to perceive accurately, appraise and express emotion; the ability to access and/or generate feelings when they facilitate thought; the ability to understand emotion and emotional knowledge; and the ability to regulate emotions to promote emotional and intellectual growth” (p. 10).
Furthermore, EI, now regarded as a promising form of intelligence (Bryan and Mayer, 2021), has given rise to two distinct approaches over time: (a) the “Ability” EI (AEI) model (in line with Mayer and Salovey’s original concept; Haag et al., 2023; Mayer et al., 2004; Schlegel and Mortillaro, 2019) and (b) the “Trait” EI (TEI) model (Bar-On, 1997; Goleman, 1995; Petrides and Furnham, 2000, 2003).
The AEI framework is often seen as more promising compared to TEI as it meets standards of intelligence (Haag et al., 2023, 2024a; Matthews et al., 2004; Mayer et al., 2016; Schlegel and Mortillaro, 2019). While AEI is categorized as a form of intelligence, TEI is regarded as a “personality trait,” linked to conceptual and psychometric challenges (Schlegel and Mortillaro, 2019). Furthermore, research has indicated that these two EI approaches have a weak statistical correlation (Brackett et al., 2006; Haag et al., 2023; Lopes et al., 2004).
The question of whether AEI predicts important real-life outcomes has attracted considerable attention (Robinson, 2024). In such a vein, we explore the association between AEI and real-life outcomes.
Some studies have shown that AEI predicts job performance (O’Boyle et al., 2012), the quality of relationships (Lopes et al., 2004), and overall wellbeing (Di Fabio and Kenny, 2016; Sánchez-Álvarez et al., 2016). However, these associations tend to be relatively weak and inconsistent compared to the predictive power of TEI (Robinson, 2024; Zeidner and Olnick-Shemesh, 2010).
Recently, Robinson (2024) called on researchers to provide fresh insights to know a lot more about whether and how AEI manifests itself in daily life. According to him, AEI should significantly impact decision-making, social interactions, and overall wellbeing, but further research is crucial to “forge” these links. We follow this direction by conducting a latent profile analysis of AEI to identify beneficial and risk profiles in wellbeing, mental and physical health, and decision-making.
Nearly three decades after the publication of their foundational article on AEI (Mayer and Salovey, 1997), Mayer et al. (2016) began to enhance the theoretical framework surrounding the AEI model and its assessment. Following this approach, Haag et al. (2023) introduced the QEPro model, which extends Mayer and Salovey (1997) AEI framework through theory-based item development and scoring method, integrating it within the situational judgment test (SJT) framework. While the QEPro model was specifically developed for managers, the authors later introduced the QEg model, which serves as its generalized version intended for the broader population (Haag et al., 2024a). Both (QEPro and QEg) have the same factorial structure and are composed of the same dimensions. Here, we refer to the QEg model as our target population is a general population of adults.
According to the QEg model, AEI can be defined as the ability to identify (IE), understand (UE), and regulate (SME) emotions (Haag et al., 2024a):
Identification of Emotions (IE) represent the meta-competency to accurately identify emotions in self and others (Haag et al., 2024a). It consists of three abilities:
a. Scanning Physiological Manifestations: the ability to recognize one’s own emotions through an introspective examination of the physical sensations felt.
b. Interpreting Emotional Cues: the ability to recognize emotions through their cognitive expressions; behavioral inclinations; vocal, postural, and facial cues; along with the related subjective-experiential aspect.
c. Identifying Emotional Triggers: the ability to pinpoint the specific triggers of emotions.
Understanding emotions (UE) represent the meta-competency to accurately understand emotions and anticipate their positive and negative consequences (Haag et al., 2024b). It consists of two abilities:
d. Understanding Emotional Timelines: the ability to evaluate the intensity of emotions and to predict how they will change over time.
e. Anticipating Emotional Outcomes: the ability to foresee the positive and negative effects of an emotion.
Strategic Management of Emotions (SME) represents the meta-competency to first select and then feel/express the appropriate emotions to adapt to a situation (Haag et al., 2024b). It consists of two abilities:
f. Selecting the Target Emotional State: the ability to recognize and choose the appropriate emotional state for a specific situation.
g. Emotion Regulation: the ability to apply the correct emotion regulation strategy to achieve the targeted emotional state.
Conceptualized as a set of abilities, AEI is analogous to general intelligence (Mayer and Salovey, 1997) and should thus be measured through so-called “performance-based” tests - considered the most promising way of assessing EI (Haag et al., 2023; Matthews et al., 2004) but also the most demanding for participants (cognitively and temporally effortful), which explains why few studies use this type of measurement.
New “performance-based” AEI tests emerged recently. Among them, QEg (Haag et al., 2024a), which is an online-only performance-based test that has good psychometric qualities.
The QEg questionnaire aims to measure the AEI seven dimensions of the QEg model defined earlier. The QEg questionnaire extends the framework of the QEPro questionnaire (designed only for managers and leaders) by extracting and revalidating existing items (from QEPro questionnaire), as well as by creating new items relevant to the general public and everyday contexts.
Item development and scoring of the QEg questionnaire are theory-based. Both the items and their “correct” answers are “objectively” determined by following the literature in the field of emotion and emotional intelligence framework (Haag et al., 2024a).
Furthermore, the QEg questionnaire was elaborated within the situational judgment test (SJT) framework to increase criterion-related validity and incremental validity (Lievens et al., 2008).
Although previous studies have highlighted the key role of emotional intelligence in everyday life, most of them used a variable-centered approach to investigate how individuals differed with regard to the mean of the sample but not how the specific dimensions of emotional intelligence interacted together (Pirsoul et al., 2022).
Moreover, most of these studies used a TEI (rather than AEI) approach (e.g., Martínez-Monteagudo et al., 2021; Pirsoul et al., 2022; Toyama and Mauno, 2016) that considers EI as a “trait” among other personality traits measured through self-report questionnaires (Haag et al., 2023; Matthews et al., 2004; Schlegel and Mortillaro, 2019).
As TEI and everyday life outcomes are commonly measured using self-report questionnaires, these concepts tend to show stronger correlations with each other due to their subjective nature and the consistency in how individuals evaluate their own traits or behaviors (Podsakoff et al., 2003).
In contrast, performance tests, which measure AEI more objectively, often show weaker correlations with self-report scales. This can be attributed to self-assessment biases, where individuals may overestimate or underestimate their actual abilities, leading to discrepancies between self-reported results and objectively measured performance (Kruger and Dunning, 1999; Paulhus and Reid, 1991). Adopting a different perspective to examine the relationship between AEI and life outcomes is thus required.
In this article, we explore AEI following a person-centered approach to identify—through a latent profile analysis (LPA)—AEI profiles regarding QEg’s seven dimensions and an Anova to observe their relationship to everyday life outcomes.
Recently, Haag et al. (2024b) identified two latent profiles of AEI—using the QEPro/QEg model—within a diverse population of managers. LPA, which is specifically structured to account for the presence of subpopulations characterized by different parameters (Meyer and Morin, 2016; Morin, 2016), offers a unique framework for exploring the quantity and nature of emerging AEI profiles.
Their analysis yielded two distinct profiles: The full emotional processing (FEP) and the minimal emotional processing (MEP). The FEP profile significantly outperformed the MEP profile on the following five AEI dimensions: Identifying Emotional Triggers, Understanding Emotional Timelines, Anticipating Emotional Outcomes, Selecting the Target Emotional State, and Emotion Regulation. The authors concluded that the FEP displays “the most complete processing of emotion” (p4).
Our approach, in line with Haag et al. (2024b), uses LPA to identify emerging AEI profiles, based on the QEg model and its questionnaire, within a general population, and subsequently examines their associations with real-life outcomes.
All participants (N = 2,877; Table 1), French native, volunteered in the study without financial incentives and being aware of the confidentiality of their answers. All participants were recruited with the support of a French popular science magazine and a French television program devoted to science which have published on their respective websites a call to participate in our study. They completed QEg and other questionnaires online (via the Qualtrics software package). Test administration was extended over 4 weeks.
QEg was administered online to all participants. All other measures were French adaptations of self-report questionnaires and were also online administered. We confirmed the psychometric qualities of each of these scales through confirmatory factorial analyses (CFAs) and verification of internal consistency using Cronbach’s alpha. All the CFA results showed a good fit with the presupposed models, and the alpha values were in line with those expected (see Table 2; Nunnally and Bernstein, 1994):
• Perceived Stress Scale 10 (PSS-10; Bellinghausen et al., 2009): χ2 (35) = 345.4, CFI = 0.98, RMSEA = 0.056 [0.050, 0.061], TLI = 0.98 et SRMR = 0.045
• Shirom-Melamed Burnout Measure (SMBM; Sassi and Neveu, 2010): χ2 (74) = 1216.8, CFI = 96, RMSEA = 0.073 [0.070, 0.077], TLI = 0.96 et SRMR = 0.049
• Satisfaction with Life Scale (SWLS; Bacro et al., 2020): χ2 (5) = 48.79, CFI = 0.99, RMSEA = 0.055 [0.042, 0.070], TLI = 0.98 et SRMR = 0.013
• Gratitude Questionnaire-6 (GQ-6; Shankland and Martin-Krumm, 2012): χ2 (9) = 134.6, CFI = 0.96, RMSEA = 0.07 [0.060, 0.080], TLI = 0.93 et SRMR = 0.031
• Survey Work–Home Interaction-Nijmegen (SWING; Lourel et al., 2005): χ2 (203) =2899.01, CFI = 0.91, RMSEA = 0.068 [0.066, 0.070], TLI = 0.89 et SRMR = 0.057
• Consideration of Future Consequences Scale (CFC-14; Camus et al., 2014): χ2 (76) = 818.9, CFI = 0.96, RMSEA = 0.058 [0.055, 0.062], TLI = 0.95 et SRMR = 0.055
LPA is designed to determine sub-groups in an extent sample, called a profile. LPA was run with the open-source software Mclust package (Scrucca et al., 2016) to investigate one to five profiles. The optimal number of profiles has been selected in regard to the statistics criterion (see Table 3), substantive meaningfulness, and theoretical conformity (Gillet et al., 2019).
The three-profile to the five-profile solutions were examined more closely. This examination demonstrated that the three-profile solution was the most proper solution regarding its statistical and theoretical conformity. The three-profile solution was retained for further analyses (BIC = 294; Figure 1).
We named the three profiles full emotional processing (FEP), minimal emotional processing (MEP), and partial emotional processing (PEP). The FEP outperforms the two other profiles on all EI abilities measured by QEg (the subscales appear in Figure 1). These results suggest that the FEP profile captures and processes emotional information in a broader and deeper way than the two other profiles (Scanning Physiological Manifestations, Interpreting Emotional Cues, Identifying Emotional Triggers, Understanding Emotional Timelines, Anticipating Emotional Outcomes, Selecting the Target Emotional State, and Emotion Regulation).
We also ran ANOVA in order to understand more deeply the benefits of full emotional processing on real-world outcomes such as perceived stress, home–work interaction, mental health, wellbeing, and decision-making.
We test differences between the three identified profiles (FEP, PEP, and MEP) on several variables through ANOVA. We then compare profiles means two by two with pairwise t-test. Our results show that FEP significantly outperforms the two other profiles (MEP performs the worst each time) on all key life variables measured (see Table 2).
FEP is associated with the lowest levels of perceived stress which is consistent with the literature (Shahin, 2020). High perceived stress has a negative impact on mental and physical health (Lu et al., 2019).
FEP is characterized by the lowest score on the home–work negative interaction. Previous research pointed out associations between negative HWI and fatigue (Geurts et al., 2003) and decreased psychological health (Van Hooff et al., 2005).
FEP exhibits and experiences greater gratitude, an emotion that helps individuals to deal with adversity, anxiety, and depression, and to build strong interpersonal relationships (Cunha et al., 2019; Diniz et al., 2023).
FEP has the highest scores on the satisfaction with life scale. Satisfaction with life is associated with better health, higher self-esteem, stronger resilience, lower depression, and anxiety (Diener et al., 2018; Cerezo et al., 2022).
FEP has the lowest score on the Emotional Exhaustion subscale which is considered the core meaning of Burnout (Lee and Ashforth, 1996). Thus, FEP tends to be more protective against depressive symptoms (Gerber et al., 2018).
Finally, our results indicate that FEP has a great consideration of future consequences (vs. immediate gratification) and a tendency to make decisions that are more future-oriented. Not considering the future consequences of our actions is highly risky for our health (Murphy and Dockray, 2018).
The different results suggest that FEP tends to be associated with better mental and physical health, wellbeing, satisfaction with life as well as decision-making.
This article identifies three distinct AEI profiles (FEP, MEP, PEP) using LPA, which expands upon the previous research by Haag et al. (2024b) which identified only two profiles (FEP and MEP) within a (smaller) sample of managers. While both studies used LPA and the QEPro/QEg model framework, the inclusion of a broader, non-managerial adult population in the present research revealed a more nuanced spectrum of AEI profiles.
The emergence of the additional “Partial Emotional Processing” (PEP) profile suggests a greater heterogeneity in emotional intelligence competencies than previously observed within a managerial context. This highlights the importance of considering diverse populations when investigating the relationship between AEI profiles and real-life outcomes.
This article highlights the direct benefits of FEP on various real-life outcomes. Our results show that being a FEP is beneficial for mental and physical health, wellbeing, and decision-making. Consistent with the existing literature, FEP is significantly and negatively associated with high perceived stress, negative work–home interaction, reduced psychological wellbeing, and emotional exhaustion. Conversely, FEP correlated significantly and positively with gratitude, life satisfaction, and the tendency to make decisions that are more future-oriented.
Our findings are in line with Pascual-Leone and Greenberg (2007) who found that distress is often linked to poor or “unprocessed” emotions. They showed, from a therapeutic approach, that there exist more efficient ways of processing emotions than others. Regarding the advantages of FEP on real-life outcomes, FEP could be considered an efficient way of processing emotions.
While a widely read science magazine and a health-focused television program facilitated the recruitment of a substantial participant pool, this approach may have inadvertently resulted in a sampling bias. Our sampling could bias the generalizability of the findings by disproportionately including individuals who possess a pre-existing interest in science or health-related topics (Franzke et al., 2020). This demographic may limit the generalizability of the study’s conclusions. Future research should consider using more varied recruitment strategies, including outreach to less engaged audiences, to ensure a diverse participant representation that captures a broader spectrum of perspectives on the subject matter (Merriam and Tisdell, 2015).
Not controlling for pre-existing psychiatric and neurological disorders in this study could potentially impact the generalizability and interpretation of the findings. Individuals with such disorders may exhibit unique patterns of emotional processing and regulation that differ substantially from the general population (Aslan et al., 2024; Lezak et al., 2012; Pepping et al., 2024). Failing to account for these differences introduces the risk of confounding variables, obscuring the true relationships between AEI profiles and the measured outcomes. For example, individuals with anxiety disorders may exhibit heightened sensitivity to emotional cues and experience greater emotional reactivity, potentially leading to higher scores on certain QEg dimensions, even in the absence of a “Full Emotional Processing” profile (Barlow, 2002). Conversely, individuals with depressive disorders may show blunted emotional responses, resulting in lower scores regardless of their underlying emotional intelligence competencies (American Psychiatric Association, DSM-5 Task Force, 2013).
This limitation reveals the need for future research incorporating robust screening measures for psychiatric and neurological conditions and potentially implementing stratified analyses to account for their influence on AEI profiles and their associations with real-life outcomes.
Self-report measures as the ones used in this article to evaluate real-life outcomes, while valuable for assessing subjective experiences, are susceptible to biases such as social desirability response bias and acquiescence bias (Paulhus, 1991; Podsakoff et al., 2003). Future research could incorporate methods to mitigate these biases, such as using multiple informants or using techniques such as the Balanced Inventory of Desirable Responding (BIDR; Paulhus, 1991) to assess response styles. Further, exploring the potential for common method variance through techniques such as Harman’s single-factor test would enhance the robustness of the findings.
The Need for diverse sampling and methodological approaches. While LPA provides significant insights into patterns and relationships within data, future research will aim to integrate qualitative data and case studies to enhance the ecological validity of our findings. Incorporating qualitative methods can offer a more profound and nuanced understanding of the contexts and experiences underlying the patterns identified through LPA (Creswell and Poth, 2018). This multi-method approach will allow us to triangulate data sources, thus enriching our interpretations and providing a more comprehensive overview of the phenomena under investigation (Flick, 2018). By leveraging both quantitative and qualitative techniques, we aspire to refine our findings and ensure that they are not only statistically robust but also grounded in real-world experiences and perspectives (Yin, 2018).
As LPA shows, emotion regulation considered as meta-competence (Peña-Sarrionandia et al., 2015) is high when the scores on the six dimensions preceding “emotion regulation” are high too. This first insight encourages us in the future to examine if FEP is a “cascading” profile (Joseph and Newman, 2010). Future research could examine the specific cognitive and emotional regulation strategies used by individuals with the FEP profile and explore if a cascading model emerges. This could involve measures of cognitive appraisal, coping mechanisms, and different types of emotion regulation strategies (Gross and Ford, 2024).
Exploring contextual factors. Future research could explore the factors contributing to the emergence of the PEP profile, potentially focusing on contextual factors such as work environment, age, or personality traits. Such a comparative analysis underscores the utility of person-centered approaches for uncovering a more comprehensive understanding of the multifaceted nature of AEI and its impact on various life domains.
The identification of distinct AEI profiles necessitates a shift from generic to personalized AEI training programs. A “one-size-fits-all” approach is demonstrably insufficient given the inherent heterogeneity of emotional processing styles, as evidenced by this study and prior research (Gross and Ford, 2024; Joseph and Newman, 2010).
Individuals exhibiting the full emotional processing (FEP) profile, characterized by robust emotional competence across all dimensions of the QEg model (Haag et al., 2024a), would benefit from advanced training focusing on the refinement and extension of existing strengths. Interventions might emphasize advanced emotion regulation strategies within complex scenarios, cultivating emotional leadership skills, and leveraging their emotional intelligence to enhance decision-making in high-stakes situations.
Conversely, individuals classified as minimal emotional processing (MEP) exhibit substantial deficits across multiple facets of emotional processing. Therefore, training should prioritize foundational skill-building, beginning with the development of emotional literacy. This involves enhancing the ability to accurately identify and label emotions in self and others, fostering comprehension of the causes and consequences of emotional experiences, and introducing fundamental emotion regulation techniques such as antecedent-focused regulation as well as cognitive reappraisal (Gross and Ford, 2024). The observation and understanding of one’s actual emotional functioning will precede the utilization of role-playing exercises and case studies for optimal learning and skill development, acquisition, and application (Greenberg, 2020).
The partial emotional processing (PEP) profile reveals a more nuanced presentation, with varying levels of proficiency across different dimensions of the QEg model. Consequently, interventions must adopt a highly targeted and adaptive approach. A comprehensive assessment of each individual’s specific strengths and weaknesses is crucial in designing personalized training plans that directly address identified areas of deficiency while simultaneously building upon existing competencies. This requires a precise mapping of skills gaps onto the QEg dimensions (Haag et al., 2024a) to inform the development of individualized training strategies.
Regardless of the EI profile, continuous feedback is crucial for optimal progress. This should incorporate multifaceted feedback mechanisms, including self-monitoring tools, peer feedback sessions, and coach-led assessments to provide comprehensive and iterative learning.
The utilization of situational judgment tests (SJTs), reflecting the QEg’s foundation, enables effective training by simulating real-world scenarios in which participants could practice applying emotional processing skills within realistic contexts.
Finally, the adoption of a mixed-methods approach, incorporating both quantitative and qualitative data (interviews, focus groups), is essential for a more nuanced understanding of the training’s effectiveness and for facilitating program refinement and adaptation.
The datasets presented in this study can be found in online repositories. The names of the repository/repositories and accession number(s) can be found at: https://osf.io/phq5y/?view_only=56cb8431148d442894d5a330296ce094.
The studies involving humans were approved by the Emlyon business school ethics committee. The studies were conducted in accordance with the local legislation and institutional requirements. The participants provided their written informed consent to participate in this study.
CH: Conceptualization, Data curation, Formal analysis, Investigation, Methodology, Project administration, Resources, Software, Supervision, Validation, Visualization, Writing – original draft, Writing – review & editing. LB: Writing – review & editing. CP: Data curation, Formal analysis, Methodology, Supervision, Validation, Writing – original draft, Writing – review & editing.
The author(s) declare that no financial support was received for the research, authorship, and/or publication of this article.
CH and LB were employed by Generation QE, Vannes, France. LB was also employed by Qualia Emotion Institute, Lyon, France. CP was employed by Moodwork, Paris, France.
All claims expressed in this article are solely those of the authors and do not necessarily represent those of their affiliated organizations, or those of the publisher, the editors and the reviewers. Any product that may be evaluated in this article, or claim that may be made by its manufacturer, is not guaranteed or endorsed by the publisher.
American Psychiatric Association, DSM-5 Task Force (2013). Diagnostic and statistical manual of mental disorders: DSM-5™. 5th Edn: American Psychiatric Publishing, Inc.
Aslan, I. H., Dorey, L., Grant, J. E., and Chamberlain, S. R. (2024). Emotion regulation across psychiatric disorders. CNS Spectr. 29, 215–220. doi: 10.1017/S1092852924000270
Bacro, F., Coudronnière, C., Gaudonville, T., Galharret, J.-M., Ferrière, S., Florin, A., et al. (2020). The French adaptation of the satisfaction with life scale (SWLS): factorial structure, age, gender and time-related invariance in children and adolescents. Eur. J. Dev. Psychol. 17, 307–316. doi: 10.1080/17405629.2019.1680359
Barlow, D. H. (2002). Anxiety and its disorders: The nature and treatment of anxiety and panic. 2nd Edn. New York: The Guilford Press.
Bellinghausen, L., Collange, J., Botella, M., Emery, J.-L., and Albert, E. (2009). Factorial validation of the French scale for perceived stress in the workplace. Sante Publique 21, 365–373. doi: 10.3917/spub.094.0365
Brackett, M. A., Rivers, S. E., Shiffman, S., Lerner, N., and Salovey, P. (2006). Relating emotional ability to social functioning: a comparison of self-report and performance measures of emotional intelligence. J. Pers. Soc. Psychol. 91, 780–795. doi: 10.1037/0022-3514.91.4.780
Bryan, V. M., and Mayer, J. D. (2021). Are people-Centered intelligences psychometrically distinct from thing-Centered intelligences? A Meta-Analysis. J. Intellig. 9:48. doi: 10.3390/jintelligence9040048
Camus, G., Berjot, S., and Ernst-Vintila, A. (2014). Validation française de l’échelle des Considérations pour les Conséquences Futures (CCF) de Joireman, et al. 2012 et étude de sa structure. Rev.Int. Psychol. Soc., 27, 35–63.
Cerezo, M. V., Soria-Reyes, L. M., Alarcón, R., and Blanca, M. J. (2022). The satisfaction with life scale in breast cancer patients: psychometric properties. Int. J. Clin. Health Psychol. 22:100274. doi: 10.1016/j.ijchp.2021.100274
Creswell, J. W., and Poth, C. N. (2018). Qualitative inquiry and research design choosing among five approaches. 4th Edn. Thousand Oaks: SAGE Publications, Inc.
Cunha, L. F., Pellanda, L. C., and Reppold, C. T. (2019). Positive psychology and gratitude interventions: a randomized clinical trial. Front. Psychol. 10:584. doi: 10.3389/fpsyg.2019.00584
Di Fabio, A., and Kenny, M. E. (2016). Promoting well-being: The contribution of emotional intelligence. Front. Psychol. 7, 1–13. doi: 10.3389/fpsyg.2016.01182
Diener, E., Oishi, S., and Tay, L. (2018). Advances in subjective well-being research. Nat. Hum. Behav. 2, 253–260. doi: 10.1038/s41562-018-0307-6
Diniz, G., Korkes, L., Tristão, L. S., Pelegrini, R., Bellodi, P. L., and Bernardo, W. M. (2023). The effects of gratitude interventions: a systematic review and meta-analysis. Einstein 21:eRW0371. doi: 10.31744/einstein_journal/2023RW0371
Franzke, A. S., Bechmann, A., Ess, C. M., and Zimmer, M. (2020). Internet research: ethical guidelines 3.0. AoIR (the International Association of Internet Researchers). Available at: https://aoir.org/reports/ethics3.pdf
Gerber, M., Colledge, F., Mücke, M., Schilling, R., Brand, S., and Ludyga, S. (2018). Psychometric properties of the Shirom-Melamed burnout measure (SMBM) among adolescents: results from three cross-sectional studies. BMC Psychiatry 18:266. doi: 10.1186/s12888-018-1841-5
Geurts, S. A. E., Kompier, M. A. J., Roxburgh, S., and Houtman, I. L. D. (2003). Does work-home interference mediate the relationship between workload and well-being? J. Vocat. Behav. 63, 532–559. doi: 10.1016/S0001-8791(02)00025-8
Gillet, N., Morin, A. J., Choisay, F., and Fouquereau, E. (2019). A person-centered representation of basic need satisfaction balance at work. J. Pers. Psychol. 18, 113–128. doi: 10.1027/1866-5888/a000228
Goleman, D. P. (1995). Emotional intelligence: Why it can matter more than IQ for character, health and lifelong achievement. New York: Bantam Books.
Gross, J. J., and Ford, B. Q. (2024). Handbook of emotion regulation. 3rd Edn. New York, NY: Guilford Press.
Haag, C., Bellinghausen, L., and Jilinskaya-Pandey, M. (2023). QEPro: an ability measure of emotional intelligence for managers in a French cultural environment. Curr. Psychol. 42, 4080–4102. doi: 10.1007/s12144-021-01715-6
Haag, C., Bellinghausen, L., and Poirier, C. (2024a). The QEg: a generalized version of QEPro ability measure of emotional intelligence. Psychology 15, 393–406. doi: 10.4236/psych.2024.153024
Haag, C., Poirier, C., and Bellinghausen, L. (2024b). Emotional intelligence and the dark triad: a latent profile analysis to investigate the Jekyll and Hyde of the emotionally intelligent manager. Front. Psychol. 15:1459997. doi: 10.3389/fpsyg.2024.1459997
Joseph, D. L., and Newman, D. A. (2010). Emotional intelligence: an integrative meta-analysis and cascading model. J. Appl. Psychol. 95, 54–78. doi: 10.1037/a0017286
Kruger, J., and Dunning, D. (1999). Unskilled and unaware of it: how difficulties in recognizing one's own incompetence lead to inflated self-assessments. J. Pers. Soc. Psychol. 77, 1121–1134. doi: 10.1037/0022-3514.77.6.1121
Lee, R., and Ashforth, B. E. (1996). A meta-analytic examination of the correlates of the three dimensions of job burnout. J. Appl. Psychol. 81, 123–133. doi: 10.1037/0021-9010.81.2.123
Lezak, M. D., Howieson, D. B., Bigler, E. D., and Tranel, D. (2012). Neuropsychological assessment. 5th Edn. New York: Oxford university press.
Lievens, F., Peeters, H., and Schollaert, E. (2008). Situational judgment tests: a review of recent research. Pers. Rev. 37, 426–441. doi: 10.1108/00483480810877598
Lopes, P. N., Brackett, M. A., Nezlek, J. B., Schütz, A., Sellin, I., and Salovey, P. (2004). Emotional intelligence and social interaction. Pers. Soc. Psychol. Bull. 30, 1018–1034. doi: 10.1177/0146167204264762
Lourel, M., Gana, K., and Wawrzyniak, S. (2005). L’interface « vie privée–vie au travail »: adaptation et validation française de l’échelle SWING (survey work–home interaction-Nijmegen). Psychologie du travail et des organisations 11, 227–239. doi: 10.1016/j.pto.2005.10.003
Lu, X., Juon, H.-S., He, X., Dallal, C. M., Wang, M. Q., and Lee, S. (2019). The association between perceived stress and hypertension among Asian Americans: does social support and social network make a difference? J. Community Health 44, 451–462. doi: 10.1007/s10900-018-00612-7
Martínez-Monteagudo, M. C., Inglés, C. J., Suriá, R., Lagos, N., Delgado, B., and García-Fernández, J. M. (2021). Emotional intelligence profiles and self-concept in Chilean adolescents. Curr. Psychol. 40, 3860–3867. doi: 10.1007/s12144-019-00350-6
Matthews, G., Zeidner, M., and Roberts, R. D. (2004). Emotional intelligence: Science and myth. Cambridge, Massachusetts: MIT press.
Mayer, J. D., and Salovey, P. (1997). “What is emotional intelligence?” in Emotional development and emotional intelligence: Educational implications. eds. P. Salovey and D. J. Sluyter (New York: Basic Books), 3–34.
Mayer, J. D., Salovey, P., and Caruso, D. R. (2004). Emotional intelligence: Theory, findings, and implications. Psychol. Inq. 15, 197–215. doi: 10.1207/s15327965pli1503_02
Mayer, J. D., Caruso, D. R., and Salovey, P. (2016). The ability model of emotional intelligence: Principles and updates. Emot. Rev. 8, 290–300. doi: 10.1177/1754073916639667
Merriam, S. B., and Tisdell, E. J. (2015). Qualitative research: A guide to design and implementation. San Francisco, CA: Wiley.
Meyer, J. P., and Morin, A. J. S. (2016). A person-centered approach to commitment research: theory, research, and methodology. J. Organ. Behav. 37, 584–612. doi: 10.1002/job.2085
Morin, A. J. S. (2016). Person-centered research strategies in commitment research. The handbook of employee commitment. Cheltenham: Edward Elgar. 490–508.
Murphy, L., and Dockray, S. (2018). The consideration of future consequences and health behaviour: a meta-analysis. Health Psychol. Rev. 12, 357–381. doi: 10.1080/17437199.2018.1489298
O’Boyle, E. H. Jr., Forsyth, D. R., Banks, G. C., and McDaniel, M. A. (2012). A meta-analysis of the dark triad and work behavior: A social exchange perspective. J. Appl. Psychol. 97, 557–579. doi: 10.1037/a0025679
Pascual-Leone, A., and Greenberg, L. S. (2007). Emotional processing in experiential therapy: why" the only way out is through.". J. Consult. Clin. Psychol. 75, 875–887. doi: 10.1037/0022-006X.75.6.875
Paulhus, D. L. (1991). “Measurement and control of response bias” in Measures of personality and social psychological attitudes. eds. J. P. Robinson, P. R. Shaver, and L. S. Wrightsman (San Diego: Academic Press), 17–59.
Paulhus, D. L., and Reid, D. (1991). Enhancement and denial in social desirability: the role of self-deceptive styles. J. Pers. Soc. Psychol. 60, 307–317. doi: 10.1037/0022-3514.60.2.307
Peña-Sarrionandia, A., Mikolajczak, M., and Gross, J. J. (2015). Integrating emotion regulation and emotional intelligence traditions: a meta-analysis. Front. Psychol. 6:160. doi: 10.3389/fpsyg.2015.00160
Pepping, N., Weinborn, M., Pestell, C. F., Preece, D. A., Malkani, M., Moore, S., et al. (2024). Improving emotion regulation ability after brain injury: a systematic review of targeted interventions. Neuropsychol. Rehabil. 4, 1–41. doi: 10.1080/09602011.2024.2398029
Petrides, K. V., and Furnham, A. (2000). On the Dimensional Structure of Emotional Intelligence. Pers. Individ. Differ. 29, 313–320. doi: 10.1016/S0191-8869(99)00195-6
Petrides, K. V., and Furnham, A. (2003). Trait emotional intelligence: Behavioural validation in two studies of emotion recognition and reactivity to mood induction. Eur. J. Pers. 17, 39–57. doi: 10.1002/per.466
Pirsoul, T., Parmentier, M., and Nils, F. (2022). Emotional intelligence profiles and job search correlates in the context of the school-to-work transition. J. Career Dev. 50, 1038–1057. doi: 10.1177/08948453221141445
Podsakoff, P. M., MacKenzie, S. B., Lee, J.-Y., and Podsakoff, N. P. (2003). Common method biases in behavioral research: a critical review of the literature and recommended remedies. J. Appl. Psychol. 88, 879–903. doi: 10.1037/0021-9010.88.5.879
Robinson, M. D. (2024). Ability-related emotional intelligence: an introduction. J. Intelligence 12:51. doi: 10.3390/jintelligence12050051
Sánchez-Álvarez, N., Extremera, N., and Fernández-Berrocal, P. (2016). The relation between emotional intelligence and subjective well-being: A meta-analytic investigation. J. Posit. Psychol. 11, 276–285. doi: 10.1080/17439760.2015.1058968
Sassi, N., and Neveu, J.-P. (2010). Translation and validation of a new measurement of professional exhaustion: the Shirom-Melamed burnout measure. Can. J. Behav. Sci. 42, 177–184. doi: 10.1037/a0017700
Schlegel, K., and Mortillaro, M. (2019). The Geneva emotional competence test (GECo): an ability measure of workplace emotional intelligence. J. Appl. Psychol. 104, 559–580. doi: 10.1037/apl0000365
Scrucca, L., Fop, M., Murphy, T. B., and Raftery, A. E. (2016). Mclust 5: clustering, classification and density estimation using Gaussian finite mixture models. R J. 8:289. doi: 10.32614/RJ-2016-021
Shahin, M. A. (2020). Emotional intelligence and perceived stress among students in Saudi health colleges: a cross-sectional correlational study. J. Taibah Univ. Med. Sci. 15, 463–470. doi: 10.1016/j.jtumed.2020.09.001
Shankland, R., and Martin-Krumm, C. (2012). Évaluer le fonctionnement optimal: Échelles de psychologie positive validées en langue française. Prat. Psychol. 18, 171–187. doi: 10.1016/j.prps.2012.02.004
Toyama, H., and Mauno, S. (2016). A latent profile analysis of trait emotional intelligence to identify beneficial and risk profiles in well-being and job performance: a study among Japanese eldercare nurses. Int. J. Work Organisation Emotion 7, 336–353. doi: 10.1504/IJWOE.2016.081841
Van Hooff, M., Geurts, S. A. E., Taris, T. W., Kompier, M. A. J., Dikkers, J. S. E., Houtman, I. L. D., et al. (2005). Disentangling the relationships between work-home interference and employee health: a longitudinal study among Dutch police officers. Scand. J. Work Environ. Health 31, 15–29. doi: 10.5271/sjweh.844
Yin, R. K. (2018). Case study research and applications: Design and methods. 6th Edn. Thousand Oaks, CA: Sage.
Keywords: ability emotional intelligence (AEI), LPA, life outcomes, well - being, decision-making, health, gratitude, emotional intelligence assessment
Citation: Haag C, Bellinghausen L and Poirier C (2025) Ability emotional intelligence profiles and real-life outcomes: a latent profile analysis of a large adult sample. Front. Psychol. 16:1465774. doi: 10.3389/fpsyg.2025.1465774
Received: 16 July 2024; Accepted: 15 January 2025;
Published: 07 February 2025.
Edited by:
Kesong Hu, University of Arkansas at Little Rock, United StatesReviewed by:
África Martos Martínez, University of Almeria, SpainCopyright © 2025 Haag, Bellinghausen and Poirier. This is an open-access article distributed under the terms of the Creative Commons Attribution License (CC BY). The use, distribution or reproduction in other forums is permitted, provided the original author(s) and the copyright owner(s) are credited and that the original publication in this journal is cited, in accordance with accepted academic practice. No use, distribution or reproduction is permitted which does not comply with these terms.
*Correspondence: Christophe Haag, aGFhZ0BlbS1seW9uLmNvbQ==
Disclaimer: All claims expressed in this article are solely those of the authors and do not necessarily represent those of their affiliated organizations, or those of the publisher, the editors and the reviewers. Any product that may be evaluated in this article or claim that may be made by its manufacturer is not guaranteed or endorsed by the publisher.
Research integrity at Frontiers
Learn more about the work of our research integrity team to safeguard the quality of each article we publish.