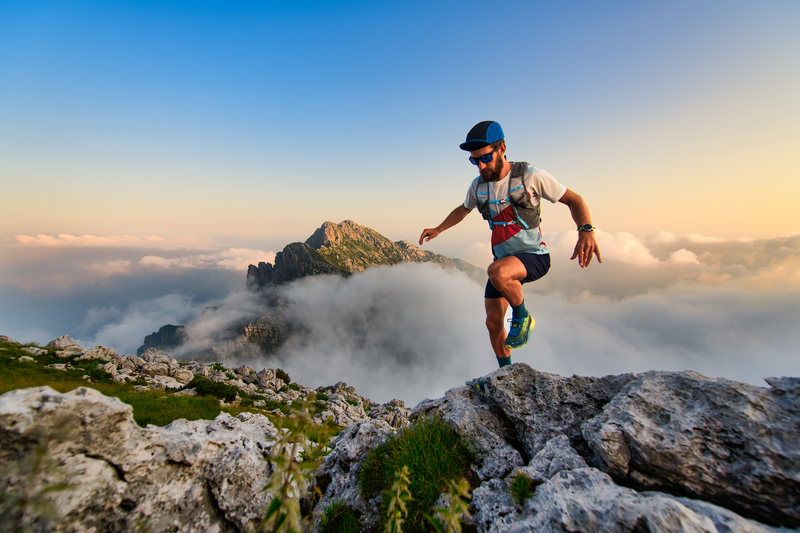
94% of researchers rate our articles as excellent or good
Learn more about the work of our research integrity team to safeguard the quality of each article we publish.
Find out more
ORIGINAL RESEARCH article
Front. Psychol. , 10 January 2025
Sec. Educational Psychology
Volume 15 - 2024 | https://doi.org/10.3389/fpsyg.2024.1476437
Introduction: As we all know, learning engagement is a key indicator for measuring the quality of students’ learning outcome and assessing their learning effectiveness. However, the relationship among personality traits, emotion regulation, and learning engagement has not been thoroughly studied.
Methods: This study aims to investigate the relationship among personality traits, emotion regulation and learning engagement. A questionnaire survey was conducted on some college students in Shandong Province, China.
Results: The results show that: (1) there are no significant differences in Big Five Personality Traits, learning engagement, and emotion regulation by gender, grade level, and subject category to which the major belongs. (2) The personality traits of college students can directly affect learning engagement. (3) Emotion regulation partially mediates the relationship between personality traits and learning engagement.
Discussion: The study reveals the importance of personality traits and emotion regulation on students’ learning. It has a significant meaning in enhancing students’ learning engagement level.
Higher education is an important link in building a strong educational country, hence, it becomes one of the key concerns of researchers at home and abroad. Higher education is committed to cultivating high-level specialists who can master professional knowledge and skills. Learning engagement is an important educational outcome for students in the 21st century (Fullan et al., 2018). The essence of higher education is learning engagement and student development (Hu, 2005). Research has shown that learning engagement is closely related to college students’ academic achievement and growth experience, what’s more, it is a predicting indicator of higher education quality (Axelson and Flick, 2010; Liu, 2015; Wu et al., 2023). Personality traits refer to the psychological structure as well as behavioral responses made by individuals under different kinds of stimuli (Zhao et al., 2019). Compared with intelligence level, personality traits are more predictive of students’ academic performance (Goff and Ackerman, 1992). Understanding the personality traits of college students is an important prerequisite for improving college students’ learning achievement and optimizing their growth experience. Emotions are reactions to situations, actions, or events. During the learning process, emotions inevitably fluctuate (Ahn and Harley, 2020). Emotional fluctuations are responses to the learning process, affecting students’ learning experience and learning outcomes (Fuente et al., 2024; Chen-Bouck et al., 2024). Although there are previous studies that have examined the relationship between personality traits and learning engagement (Mahama et al., 2022; Zhao and Ji, 2024; Morfaki and Skotis, 2022), as well as studies studying on emotion regulation for learning engagement (Pan, 2023; Huang et al., 2023; Zhang et al., 2024) and studies on the role of emotion regulation as a mediator (Zhang et al., 2024; Li et al., 2024; Qiao et al., 2024), there are relatively few studies on the relationships among personality traits, emotion regulation, and learning engagement.
This study aims to design reliable scales to collect data, use authoritative tools to analyze the data, and construct a model of the influencing factors of college students’ personality traits, emotion regulation, and learning engagement through scientific methods in order to deeply explore and reveal the intrinsic correlations among these three items. With the help of this study, the study benefits can help learners learn more easily and happily, and have them actively participate in various learning activities, so as to further promote the overall improvement of the quality of teaching and learning in higher education.
The term learning engagement can be traced as far back as the concept of Time on Task proposed by Ralph Tyler, who argued that success is directly proportional to the time invested (Madaus and Stufflebeam, 1989). According to Liu et al. (2017), learning engagement refers to the positive mindset adopted by an individual during the learning process. According to Astin (1984), learning engagement is the sum of physical and mental energy that students put into their coursework activities. Towards this issue, scholars also have their own views on the dimensional division of learning engagement, hence, expressing their respective viewpoints. Finn and Zimmer (2012) classified learning engagement into four levels, with Level I learning engagement characterized by students simply complying with the basic requirements posed by school, e.g., attending classes. Level II learning engagement is characterized by enthusiasm and initiative input for academic tasks. Level III learning engagement occurs outside the formal classroom and represents student engagement in extracurricular activities. Level IV learning engagement includes goal setting, decision making, and assuming leadership roles. According to Schaufeli et al. (2002), learning engagement is a positive and sustained affective state exhibited by students during the learning process. It is characterized by vigor, dedication, and focus. Kuh et al. (2007) believes that learning engagement has two dimensions. One is the students’ own engagement in activities that promote their academic success. The second is that students engage in activities and contribute to their own improvement through the help of their belonging schools. However, Fredricks and McColskey (2012) argued that learning engagement consists of three dimensions: cognitive engagement, behavioral engagement, and affective engagement. Cognitive engagement mainly includes problem-solving strategies, metacognitive strategies, and so on. Behavioral engagement mainly includes persistence, effort, and attention. Affective engagement mainly includes interest, pride, and so on. After that, Fredricks et al. (2016) gave consideration to the effect of social interaction among students on learning engagement. He proposed that learning engagement includes cognitive engagement, behavioral engagement, affective engagement, and social engagement. Other researchers such as Christenson et al. (2012) pointed out that learning engagement consists of four dimensions: behavioral engagement, cognitive engagement, affective engagement, and initiative engagement. The four aspects with each other and are related to each other. Therefore, based on all these viewpoints, this study argues that learning engagement refers to the active, fulfilling, and sustained state that students exhibit in the learning process. It not only includes the time, energy, and resources that students put into their academics, but also involves students’ investment in multiple dimensions such as affective, cognitive, and behavioral. This study adopts a three-dimensional framework of behavioral engagement, affective engagement, and cognitive engagement to study learning engagement.
Personality traits are conceptualized as neurophysiological systems that induce adaptive expressive behaviors in response to stimuli (Allport, 1961). Personality traits are the active behavioral responses that people make to their environment. Scholars do not have a recognized universal standard for defining personality traits. Therefore, they began to revert the directions of their researches from the definition of personality traits to the classification of personality traits. Allport (1961) believed that traits are the root of personality. It is the individual’s own traits and not the environment that determines his behavioral activities. He categorized personality traits into primary, central and secondary traits. Eysenck and Eysenck (2013) utilized the means of trait clusters to categorize personality traits into extraversion, neuroticism, and psychoticism. Cattell (1943) used factor analysis to categorize personality traits into two dimensions: surface traits and root traits. McCrae and Costa (2004) suggested that personality traits consist of five major dimensions, which are extraversion, neuroticism, openness, conscientiousness, and agreeableness. Individuals with high extroversion are good communicators and are prone to more easily acquire pleasure and satisfaction in socialization. Neuroticism reflects emotional stability; individuals high in neuroticism are emotionally highly reactive and exhibit impulsive temper. Openness mainly refers to an individual’s exploration of unfamiliar situations. Individuals with high openness are characterized by high creativity and strong divergent thinking. Conscientiousness refers to the individual’s self-control. Individuals with high conscientiousness are well organized and persistent in their work. Agreeableness refers to an individual’s attitude toward others. Individuals with high agreeableness show empathy and are more willing to help others. Above all, these are referred to as the Big Five Personality Traits. The Big Five Personality Traits are highly representative, therefore, this study uses the Big Five Personality Traits to categorize personality traits. Scholars have also studied the relationship between personality traits and learning engagement. Su (2023) collected questionnaires from senior nursing students and found that senior nursing students’ learning engagement was significantly negatively correlated with neuroticism, and significantly positively correlated with the other four personality traits. Jia (2023) investigated middle school students through the Proactive Personality Scale and Learning Engagement Scale and found that proactive personality directly predicted very good learning engagement and also positively greatly predicted learning engagement through academic resilience. Fan (2021) explored the influencing factors affecting college students’ learning engagement through Nvivo 11 software and found that personality traits are key factors affecting college students’ learning engagement. Jiang et al. (2018) found that perseverance personality positively predicted learning engagement as well as academic achievement. They had distributed questionnaires to primary and secondary school students, discovering that learning engagement fully mediated the role of perseverance personality and academic achievement. Cheng et al. (2022) concluded that the Big Five Personality Traits have a significant impact on students’ motivation to learn, and their attributional styles partially mediate the effect. Kara et al. (2024) used the Student Engagement Scale in Online Learning Environments to survey 437 college students and found that the personality trait was a significant predictor of student engagement in online learning environments. Wu and Yu (2023) collected data from 1,004 university students and used structural equation modelling to explore how students with different personality traits engage in e-learning. Qureshi et al. (2016) explored the predictive role of personality in multidimensional engagement models by surveying students and employees. In addition, the studies of several experts such as Mahama et al. (2022), Ongore (2014), Qureshi et al. (2016), and Bao et al. (2022) have shown that learning engagement is affected by personality traits. Therefore, this study used personality traits as variables, utilized Big Five Personality Traits Scale and Learning Engagement Scale to investigate the relationship between the influence of personality traits and learning engagement among college students.
In our daily lives, we produce a wide range of emotions, and emotions are an individual’s response to the environment (Shi, 2023). Emotion regulation is a kind of mental activity processing engineering, which can influence what emotions an individual produces and the duration of generated emotions (Thompson and Goodman, 2010). Emotions are regulatable and individuals can regulate their emotions through conscious or unconscious behaviors (Li, 2019). Scholars have found that emotion regulation has an impact on learning engagement. Zhang and Wang (2023) found that academic emotions play a mediating role in the relationship between teacher support and foreign language learning engagement by establishing a multiple mediation model, which can significantly and indirectly predict foreign language learning engagement through emotions such as pleasure and anxiety. Liu et al. (2023) found that students’ positive emotions in a blended learning environment had a positive effect on deep cognitive engagement by carrying out through a questionnaire survey. Xu et al. (2023) found that the correlation among self-efficacy, emotional engagement and learning engagement was significant by interviewing 563 college students through a questionnaire survey, which further proved that emotion regulation partially mediated the relationship between self-efficacy and learning engagement. Zhang et al. (2024) conducted an in-depth survey of 1,506 high school English as a Foreign Language (EFL) learners using a questionnaire, and the results of the study showed that emotional inhibition mediated the relationship between perceived peer support and EFL behavioral engagement, i.e., when learners perceive a decrease in support from their peers, they may respond by inhibiting their positive emotions, which in turn affecting their behavioral engagement in EFL. Another quasi-experimental study conducted by Arguedas et al. (2016) further confirmed the positive impact of emotion regulation on learning outcomes. The study targeted a group of high school students and guided them through specific teaching strategies to become aware of their emotional states and learn how to regulate and manage these emotions effectively. The results of the study showed that when students were able to accurately identify and regulate their emotions, their motivation, engagement, and self-regulation were significantly enhanced, thus contributing to improved academic performance. Xiao et al. (2024) found that cognitive reappraisal was positively correlated with learning engagement through the use of a questionnaire targeting 1,200 high school students and that cognitive reappraisal mediated the relationship between Internet addiction and learning engagement. All these studies have emphasized the critical role of emotion regulation strategies in academic engagement. What’s more, some studies have shown that personality traits affect an individual’s emotion regulation (Qu and Wang, 2024). Therefore, this study adopted emotion regulation as a mediating variable to investigate the effect of emotion regulation on learning engagement and the mediating role of emotion regulation in personality traits and learning engagement.
Previous research has demonstrated that personality traits, and emotion regulation all have an impact on learning engagement. However, few researchers have put the three together to study their influence mechanisms. Studying their influence mechanisms has a significant importance for improving students’ emotions and enhancing learning engagement. Therefore, this study takes college students as the research object to investigate the relationship between personality traits and learning engagement, and expound the mediating role of emotion regulation. Based on the results of existing studies, the following hypotheses and conceptual model were proposed in this study (Figure 1).
Figure 1. Hypothetical model of the relationship between personality traits and learning engagement.
Hypothesis 1 (H1): Personality traits will directly affect students’ learning engagement.
Hypothesis 2 (H2): Emotion regulation will mediate the relationship between personality traits and learning engagement.
In this study, some universities in Shandong Province, China, were selected as participating units through convenience sampling method, and college students were treated as the research objects. The wide range of subjects’ specialties makes the collected data more real and reliable. The backend system shows that a total of 251 questionnaires were distributed, while 243 questionnaires were recovered, with a recovery rate of 96.8%, and 235 questionnaires were valid, with an effective rate of 96.7%. The age of the participants ranged from 19 to 23 years old. The average was 21 years old. The oldest was 23 years old. The youngest age was 19 years old. There were 172 female students and 63 male students, accounting for 73.2 and 26.8%, respectively. There were 13 freshmen students, accounting for 5.5%. There were 123 sophomores, accounting for 52.4%. There were 13 junior students, accounting for 5.5%. There were 86 seniors, accounting for 36.6%. There were 4 philosophy majoring students, taking up 1.7%; 4 economics majoring students, taking up 1.7%; 4 law majoring students, taking up 1.7%; 160 education majoring students, taking up 68.1%; 7 literature majoring students, taking up 3.0%; 32 science majoring students, taking up 13.6%; 14 engineering majoring students, taking up 6.0%; 2 medicine majoring student, taking up 0.8%; 5 management majoring students, taking up 2.2%; 2 arts majoring students, taking up 0.8%; 1 cross-disciplinary majoring student, taking up 0.4%.
The study was approved by the Ethics Committee of Qufu Normal University. Permission to conduct the study was applied and obtained from the administration of each selected school. Written informed consent was signed from the students of the selected schools prior to the survey. In the offline face-to-face approach, the researcher informed the students about the instructions on how to fill in the questionnaire and the precautions to be taken in filling in the questionnaire. The data would be used for research purposes only and would not affect their grades or studies. Participants were asked to provide truthful answers to each question, with no correct or incorrect answers. The online method used Questionnaire Star to collect the questionnaires, which also had detailed instructions and notes in the preface section of the questionnaire. Through a combination of online and offline methods, questionnaires were randomly distributed to college students of different grades and majors in colleges and universities using Questionnaire Star or paper-based questionnaires. For the convenience of statistical analysis, eventually, the paper version was collected and manually entered into Questionnaire Star. Questionnaire Star has the advantages of simple operation interface, offering various types of question options and robust collection channels, which is very suitable as a data collection tool. The results of the study will help to improve the quality of learners’ learning engagement.
This study was adapted from the NEO-FFI (NEO Five-Factor Inventory) developed by Costa and Mc Crae (1992). The NEO-FFI scale consists of a total of 60 question items categorized into 5 dimensions, namely, Neuroticism, Extraversion, Openness, Agreeableness, and Conscientiousness, with 12 question items per dimension. Question items with critical ratio CR values <3 or p > 0.05 were excluded by the extreme value method. Question items with a product-difference correlation coefficient of <0.4 or p > 0.05 with the total score were excluded by the question-item-to-total-score correlation method. Question items with weak relationships between common factors were removed by the factor analysis commonality method, when the commonality value was <0.2 or the factor loading was <0.4, the question items were removed. The specific process of elimination as well as the final results are shown in Table 1.
In this study, the personality traits scale had good reliability. First, the reliability was good (Ketchen, 2013). The Cronbach’s alpha coefficient of the Big Five personality scale was 0.904. The Cronbach’s alpha coefficients of Neuroticism, Extraversion, Openness, Agreeableness, and Conscientiousness were 0.911, 0.901, 0.905, 0.900, and 0.899, respectively. Second, the structural validity was good. The structural validity indicators were Χ2/df = 2.029, RMSEA = 0.061, GFI = 0.901, NFI = 0.911, IFI = 0.913, and CFI = 0.909. Third, the convergent validity met the requirements. Convergent validity refers to the degree of influence of a factor on other factors. Convergent validity values were determined by combining reliability (CR) and average extracted variance (AVE). The AVE values for Neuroticism, Extraversion, Openness, Agreeableness, and Conscientiousness in the Big Five Personality Scale in this study were 0.590, 0.574, 0.516, 0.521, and 0.510. The values of the combined reliability were 0.742, 0.773, 0.774, 0.743, and 0.805, which indicated that the convergent validity of each item met the desired goal. Fourth, the discriminant validity meets the standard. Discriminant validity means that there should be no strong correlation between different dimensions and that it is possible to distinguish different dimensions between scales. It is mainly measured by three kinds of data: correlation coefficients (r), AVE values, and arithmetic square root of AVE values among the latent variables. The correlation coefficients of the dimensions in this study are less than the arithmetic square root of the corresponding AVE, and the discriminant validity meets the standard. Therefore, the scale is reliable.
In this study, learning engagement was divided into three dimensions: behavioral engagement, affective engagement, and cognitive engagement based on the three-dimensional structure of learning engagement proposed by Fredricks and McColskey (2012). Behavioral engagement is the active participation behavior towards achieving positive academic activities. Affective engagement is students’ sense of belonging to school or positive and negative reactions to teachers and their peers. Cognitive engagement is the adoption of deep learning strategies during the learning process and willingness to work to understand complicated ideas and master difficult skills (Fredricks et al., 2004). The learning engagement survey scale draws on the findings of Ma et al. (2017), Gunuc and Kuzu (2014), Handelsman et al. (2005). The final version of the Learning Engagement Scale was also analyzed through item analysis, exploratory factor analysis, and reliability analysis.
The reliability of the scale is trustworthy. First, the reliability is good (Ketchen, 2013). The indicators were: behavioral engagement: Cronbach’s α = 0.928; emotional engagement: Cronbach’s α = 0.937; cognitive engagement: Cronbach’s α = 0.909; total scale: Cronbach’s α = 0.922. Second, the structural validity was good. The indicators were X2/df = 1.798, RMSEA = 0.071, GFI = 0.913, NFI = 0.919, IFI = 0.924, and CFI = 0.903. Third, the convergent validity met the requirements. The AVE values of behavioral engagement, affective engagement, and cognitive engagement in the learning engagement scale were 0.512, 0.503, and 0.548, and the combined reliability values were 0.777, 0.765, and 0.802. Fourth, discriminant validity met the criteria. The correlation coefficients of each dimension were less than the arithmetic square root of the corresponding AVE.
The Classical Emotion Regulation Scale was used in this study. The Classical Emotion Regulation Scale was developed by Gross (2002) and later revised and translated into a Chinese version by Wang et al. (2007). The scale is divided into two strategies: cognitive reappraisal and expressive inhibition. Cognitive reappraisal is a strategy in which individuals regulate their emotions by re-understanding events. Expressive inhibition is the individual’s ability to control emotions through rationalization. The classical emotion regulation scale uses a seven-point Likert scale. Dawes (2008) found that comparing 5- point or 7-point data with 10-point data can be easily done with simple proportional and arithmetic adjustments. In this study, the scores on the 7-point scale were converted to scores on the 5-point scale for ease of data processing. Based on the formula used by Preston and Colman (2000): present scale score = (score-1)/(original scale-1) × scale to be transformed. In the present study, we needed to convert the 7-point scale to a 5-point scale, and this formula could be used by adapting the formula to the present scoring system score = (rating-1) / (7–1) × 5, thus reformatting all scales to a full score of 5. A feature of this approach is that any score using the lowest scale point on any scale will become a zero. For example, a score of 1 on a 7-point scale would become (1–1)/(7–1) × 5 = 0. Through this formula, all scores on a 7-point scale can be converted to scores on a 5-point scale. In this study, the Cronbach’s alpha coefficient for the emotion regulation scale was 0.896.
SPSS 27 and EXCEL 2016 were used for data processing and analysis in this study. Compared with other software, the above two software are much simpler to use, clearer in displaying results and more authoritative in analyzing data. Therefore, they were used as the data processing and analysis tools in this study. SPSS 27 software was used to analyze the collected data for reliability and validity, descriptive statistical analysis and difference statistical analysis. EXCEL 2016 software was used as an auxiliary tool for organizing all data. The mediating effect was carried out through Model 4 in the SPSS macro program process, and Bootstrap’s method was used to validate the mediating effect of analyzing emotion regulation strategies.
In statistics, it is generally required that the data conforms to normal distribution, which is a prerequisite for carrying out data analysis. Common normal distribution test methods mainly include graphical methods, skewness coefficient and kurtosis coefficient checking method and K-S test and S-W test. In this study, skewness coefficient and kurtosis coefficient checking method were chosen to check the normal distribution of each question item of the data. A normal distribution is obeyed when the absolute value of skewness is less than 1.5 and the absolute value of kurtosis is less than 1.5 (Tabachnick and Fidell, 2019). According to Table 2, it can be seen that the skewness of all question items ranges from −1.474 to 1.195 and the kurtosis of all question items ranges from −1.274 to 1.499. Hence, all question items obeyed a normal distribution, which provided a good foundation for the subsequent analysis.
In this study, Harman’s one-factor test was used to examine the collected data for common method bias (Podsakoff et al., 2003). Exploratory factor analysis was conducted on the unrotated factors to assess the results. Common method bias was present when the variance explained by the first common factor was greater than 40%. The results showed that there were 13 factors with eigenroots greater than 1. The explained variance of the first common factor was 16.012%. Consequently, this suggests that the data in this study do not exhibit significant common method bias. In addition, covariance diagnosis was performed to confirm the existence of multicollinearity among variables. The results showed that the variance inflation factor (VIF) values of all the question items were much less than the critical threshold of 3. Therefore, there is no problem of multicollinearity (Chennamaneni et al., 2016).
As shown in Table 3, the standard deviation of Big Five Personality scale is 0.399, the standard deviation of Learning Engagement scale is 0.485, and the standard deviation of Emotion Regulation scale is 0.494. Since 0.494 > 0.485 > 0.399, the data distribution of Big Five Personality scale is the most stable, while the data distribution of Learning Engagement scale is more stable than the data distribution of Emotion Regulation scale. Correlation analysis was conducted to find a significant correlation among Big Five Personality and learning engagement and emotion regulation. Significant correlations were found between emotion regulation and learning engagement as well. A correlation analysis of the dimensions of personality traits and emotion regulation with learning engagement reveals that Extraversion, Openness, Agreeableness, and Conscientiousness have significant positive correlations with learning engagement, while only Neuroticism has a negative correlation with learning engagement. Cognitive reappraisal and expressive inhibition were also significantly positively correlated with learning engagement. These results indicate that a mediated effects analysis is warranted, aligning with the predictions outlined in Hypothesis 1. Thus, Hypothesis 1 is supported.
Firstly, independent samples t-tests were conducted to analyze the difference among college students of different genders. Then one-way ANOVA was used to test for the difference analysis for college students of different grades and major subject disciplines. The results are shown in Table 4. There was no significant difference in the gender factor on the dimensions of neuroticism (t = −0.659, p = 0.511), extraversion (t = 0.809, p = 0.420), openness (t = −1.305, p = 0.193), agreeableness (t = 0.059, p = 0.953), conscientiousness (t = −0.410, p = 0.682), learning engagement (t = −1.150, p = 0.251), and emotion regulation (t = 0.352, p = 0.725). Similarly, the grade factor showed no significant difference in the grade level factor on the dimensions of neuroticism (F = 0.190, p = 0.903), extraversion (F = 0.586, p = 0.625), openness (F = 4.003, p = 0.080), agreeableness (F = 0.691, p = 0.558), conscientiousness (F = 1.195, p = 0.313), learning engagement (F = 1.305, p = 0.273), and emotion regulation (F = 1.056, p = 0.369). There was no significant difference in the academic discipline factor on the dimensions of neuroticism (F = 1.087, p = 0.373), extraversion (F = 0.755, p = 0.658), openness (F = 2.251, p = 0.200), agreeableness (F = 0.296, p = 0.975), conscientiousness (F = 0.855, p = 0.566), learning engagement (F = 0.542, p = 0.843) and emotion regulation (F = 0.979, p = 0.458). The results are shown in Table 4.
To explore the underlying mechanism of the influential role of Big Five Personality Traits on learning engagement, emotion regulation strategy was introduced as a mediating variable to substitute into the model in the study. The test of mediating effect was conducted by using Model 4 in the SPSS macro program process. The Bootstrap method provided by Hayes was used to validate the analysis of the mediating role of emotion regulation strategies between Big Five personality traits and learning engagement. The path coefficients of emotion regulation strategies between the Big Five Personality Traits and learning engagement variables are shown in Figure 2.
Figure 2. Path coefficient plots of Big Five Personality, emotion regulation strategies and learning engagement.
According to Table 5, the Bootstrap 95% confidence interval’s lower and upper limits for the mediating effects of emotion regulation strategies on Big Five personality traits and learning engagement do not contain 0, indicating that Big Five personality traits have a direct effect on learning engagement and also exert a mediating effect through emotion regulation strategies. This direct effect (0.556) and mediating effect (0.028) accounted for 95.21 and 4.79% of the total effect (0.584). This result is consistent with the prediction of hypothesis 2. Thus, hypothesis 2 is confirmed.
This study investigates college students to investigate the effects of personality traits on learning engagement and the mediating role of emotion regulation strategies on this effect.
In terms of exploring the personality traits of college students in depth, this study found through exhaustive data analysis that college students have a relatively high mean score (M = 3.796) on the personality trait of Openness. This is a good indication that they possess an unusually active imagination, not only are they willing to accept all kinds of new ideas and new things, but also show endless curiosity and desire to explore the unknown world. On the dimension of Conscientiousness, the mean score (M = 3.528) of college students is also high. This reflects that they have excellent self-control in daily life, can effectively manage and regulate their impulsive behaviors, are more cautious and considerate in doing things, and show a high sense of responsibility and maturity (Steiner et al., 2023). The mean score (M = 3.345) of college students was also more prominent on the trait of Agreeableness. This means that they show more understanding and generosity in the process of getting along with their classmates and friends, are always helpful and good at building harmonious interpersonal relationships. The mean score of Extraversion (M = 3.142) was found to be at a moderate level in this study. This result reveals a relatively balanced distribution of Extraversion and Introversion personalities among college students. They are able to present themselves positively in social situations as well as maintain inner peace and solitude when needed. In contrast, the mean score for the dimension of Neuroticism (M = 2.899) was the lowest. This further indicates that college students are generally calm. They were able to remain calm and steady in the face of various situations, were not easily overly influenced by emotions, and demonstrated good psychological adjustment ability. In terms of learning engagement, this study also draws encouraging conclusions. College students had higher mean scores on the dimensions of cognitive engagement (M = 3.715), emotional engagement (M = 3.642), and behavioral engagement (M = 3.433). This is a good proof that they are more adept at using cognitive and metacognitive strategies to dig deeper into the deeper meaning of knowledge for meaningful learning constructs. During the learning engagement, they are able to give their full attention to the learning activities, put in unremitting efforts for them, and always maintain a positive emotional response. This state of engagement will undoubtedly help them achieve better academic performance (Lang et al., 2022). In addition, in terms of the use of emotion regulation strategies, this study also found that college students had relatively high mean scores (M = 3.719) on the dimension of cognitive reappraisal, which suggests that they are more inclined to regulate their emotional state by re-cognizing the event, demonstrating a rational and mature way of coping. In contrast, the mean score on the dimension of expression inhibition (M = 2.696) is at a moderate level, which indicates that college students are more inclined to choose to reconstruct things positively when regulating their emotions rather than simply inhibit their emotional expression, a tendency that undoubtedly reflects their more positive and healthy psychological state (Zhao et al., 2016).
This study found that there was no significant difference in Big Five personality, learning engagement, and emotion regulation across gender, grade level, and subject discipline. This is inconsistent with the findings of previous studies. For example, Xu et al. (2023) discovered that there was a significant difference in learning engagement between grades. Yang (2020) found that male students used expressive inhibition strategies more frequently than female students. In her study, Zhang (2022) found that the level of learning engagement differed between genders. This inconsistency in findings may be a result of differences in research participants and research settings. Meanwhile, college students have matured mentally, have passed the impulsive period of adolescence, are emotionally desensitized, and are able to make a full commitment to learning engagement. Regardless of grade level or major, they are able to perceive the implementation of learning and to regulate their emotions during the learning process.
Research indicates that both Extraversion and Agreeableness have a significant positive correlation between behavioral engagement and emotional engagement. This means that students who are extroverted and lively, sociable as well as helpful and empathetic tend to show more positive behavioral engagement and rich emotional engagement in the learning process (Mahama et al., 2022; Zhao and Ji, 2024). Meanwhile, both traits of Openness and Conscientiousness showed significant positive correlations with behavioral engagement, emotional engagement, and cognitive engagement. This shows that students with open-mindedness, openness to new things as well as rigor and conscientiousness, and dutifulness are able to engage in learning engagement in all aspects, not only showing a high degree of concentration in action and emotion, but also thinking deeply and exploring actively at the cognitive level (Sahinidis et al., 2020). It is worth noting that there is a negative correlation between only one trait, Neuroticism, and learning engagement. Such results have been obtained by other researchers (Laidra et al., 2007; Barrick et al., 1998). Consequently, students who excel at teamwork, and face challenges with optimism and positivity, while approaching knowledge with modesty and caution, tend to be more effective learners. They are able to think well and willing to make unremitting efforts to find solutions when facing difficulties and challenges in learning, and show strong stress resistance. They have higher learning engagement in the learning process (Morfaki and Skotis, 2022; Quigley et al., 2022; Zhang et al., 2020).
Research indicates that there is a significant positive correlation between each emotion regulation strategy in terms of students’ learning engagement, affective engagement, and cognitive engagement (Santos et al., 2021). Effective utilization of these emotion regulation strategies can greatly enhance students’ overall learning engagement in the learning process. When individuals inevitably encounter various problems and challenges in their studies, work, and life, they are often prone to negative emotions such as anxiety, restlessness, and irritability (Pan, 2023). If left unregulated, these negative emotions can significantly impede students’ learning engagement. Notably, there are substantial differences in emotion regulation abilities among individuals (Shafiee Rad and Jafarpour, 2022). Individuals with stronger emotion regulation abilities are usually more adept at flexibly utilizing a variety of effective regulation strategies to quickly adjust their state of mind to a normal state, and then are able to engage in learning engagement with more enthusiasm and higher efficiency (Yu et al., 2022); in contrast, individuals with weaker emotion regulation abilities tend to have difficulties in adjusting from negative emotions, which can adversely affect their learning outcomes (Bielak and Mystkowska-Wiertelak, 2020). Therefore, individuals with better emotion regulation tend to be better able to regulate their emotions and thus effectively improve their learning engagement.
Research indicates that emotion regulation plays a significant partial mediating role between personality traits and learning engagement in college students. This further reveals the complex mechanism of the influence of personality traits on learning engagement. This finding is consistent with previous studies (Huang et al., 2023; Zhang et al., 2024). Specifically, personality traits not only directly affect learning engagement, but also influence it indirectly via emotion regulation. Individuals with high Openness, Conscientiousness, and Agreeableness are able to show a spirit of courage to face challenges and defy difficulties when facing heavy learning tasks or sudden learning difficulties. They are less likely to fall into pessimistic and negative moods. These individuals usually have strong self-confidence and can experience a sense of achievement and satisfaction in the learning process, thus gaining more positive emotions. These positive emotions, in turn, motivate them to focus more on their studies, forming a virtuous cycle.
In stark contrast, individuals low in Openness, Conscientiousness, and Agreeableness are more susceptible to negative emotions such as frustration and anxiety when confronted with academic tasks. These emotions bind them, making it difficult for them to enter a normal learning state, and may even lead to a serious setback in learning motivation (Brady et al., 2018). It is worth noting that even under negative emotions, if individuals can effectively master and flexibly use emotion regulation strategies, they still have the opportunity to adjust their own emotional state, gradually get rid of the plague of negative emotions, and then enhance learning engagement, injecting new vitality and hope into the learning process (Gong, 2023). This process not only reflects the importance of emotion regulation, but also emphasizes the far-reaching significance of cultivating good personality traits and enhancing emotion regulation for improving learning engagement.
This study has important implications for the enhancing of college students’ learning engagement. This study shows that there is a strong relationship between college students’ personality traits, emotion regulation strategies and learning engagement. The personality traits of college students directly influence learning engagement. Emotion regulation partially mediates the relationship between personality traits and learning engagement. Students who excel at teamwork, optimistic and positive, modest and prudent, and responsible and self-disciplined are more engaged in the learning process, and are better at adopting emotion regulation strategies to regulate their emotions in the face of learning challenges, which is more conducive to learning activities. Therefore, in the process of education, teachers not only need to teach students according to their different personality traits, but also cultivate and shape students’ positive personality traits, and actively guide students to use emotion regulation strategies to eliminate negative emotions, so that students can be happily engaged in learning activities. School counselors can also use psychological counseling skills to provide personalized psychological counseling services for college students, guiding college students to meet difficulties and challenges with an optimistic attitude.
This study has certain limitations regarding the research tools employed. This is manifested in the fact that it only relies on a single research tool, the questionnaire, to collect and analyze data, and does not make full use of a variety of other research methods, such as the interview method and the experimental method, as auxiliary means to enrich the perspective and depth of the study. This single way of using research tools may limit the comprehensiveness and accuracy of the research results to a certain extent. In order to compensate for this deficiency, in our future research, we will be committed to comprehensively utilizing a variety of investigative tools and methods, including, but not limited to, in-depth interviews, laboratory experiments, case studies, etc., with a view to exploring in depth the relationship between personality traits, learning engagement, and emotion regulation from a variety of dimensions and levels so as to further enhance the reliability and validity of the research results. Additionally, there are limitations related to the sample selection. The sample of college students we selected was mainly concentrated in colleges and universities in Shandong Province, which makes the geographic distribution of the data relatively narrow and may not fully reflect the real situation and differences in personality traits, learning engagement and emotion regulation among college students nationwide. In order to overcome this limitation, future research should further expand the coverage of the sample data and extend the study object to students in colleges and universities in other regions or even nationwide, so as to more comprehensively analyze and explore the relationships among personality traits, learning engagement and emotion regulation among college students in different regions, as well as the differences and commonalities of these relationships in the context of different geographic and cultural backgrounds. Conducting cross-regional and cross-cultural comparative studies could yield more comprehensive, in-depth, and generalized conclusions, providing a stronger scientific basis and reference for educational practice and policy development in related fields.
This study sheds light on the impact of college students’ personality traits on learning engagement and highlights the mediating role of emotion regulation. The results of the study showed a significant correlation between college students’ personality traits and learning engagement. Students who are cooperative, optimistic and positive, modest and prudent, and responsible and self-disciplined are more likely to be engaged in learning activities. Emotion regulation mediates the relationship between personality traits and learning engagement. Appropriate use of emotion regulation strategies can improve mindfulness and help learning engagement. Thus, the results of this study hold considerable significance for enhancing college students’ learning engagement.
The original contributions presented in the study are included in the article/supplementary material, further inquiries can be directed to the corresponding author/s.
The studies involving humans were approved by The Ethics Committee of Qufu Normal University. The studies were conducted in accordance with the local legislation and institutional requirements. The participants provided their written informed consent to participate in this study.
TD: Writing – original draft. WD: Writing – original draft. MN: Writing – review & editing. ZX: Writing – review & editing.
The author(s) declare that financial support was received for the research, authorship, and/or publication of this article. This research was supported by the National Natural Science Foundation of China (No. 62007020) and Shandong Provincial Natural Science Foundation (ZR2024QF058).
We are grateful for the comments provided by the reviewers.
The authors declare that the research was conducted in the absence of any commercial or financial relationships that could be construed as a potential conflict of interest.
All claims expressed in this article are solely those of the authors and do not necessarily represent those of their affiliated organizations, or those of the publisher, the editors and the reviewers. Any product that may be evaluated in this article, or claim that may be made by its manufacturer, is not guaranteed or endorsed by the publisher.
Ahn, B., and Harley, J. M. (2020). Facial expressions when learning with a queer history app: application of the control value theory of achievement emotions. Br. J. Educ. Technol. 51, 1563–1576. doi: 10.1111/bjet.12989
Arguedas, M., Daradoumis, A., and Xhafa, F. (2016). Analyzing how emotion awareness influences students' motivation, engagement, self-regulation and learning outcome. Educ. Technol. Soc. 19, 87–103.
Astin, A. W. (1984). Student involvement: a developmental theory for higher education. J. Coll. Stud. Dev. 25, 297–308.
Axelson, R. D., and Flick, A. (2010). Defining student engagement. Change 43, 38–43. doi: 10.1080/00091383.2011.533096
Bao, X., Huang, J., Li, N., Li, J., and Li, Y. (2022). The influence of proactive personality on learning engagement: the chain mediating effect of perceived social support and positive emotions. Stud. Psychol. Behav. 20, 508–514. doi: 10.12139/j.1672-0628.2022.04.011
Barrick, M. R., Stewart, G. L., Neubert, M. J., and Mount, M. K. (1998). Relating member ability and personality to work-team processes and team effectiveness. J. Appl. Psychol. 83, 377–391. doi: 10.1037/0021-9010.83.3.377
Bielak, J., and Mystkowska-Wiertelak, A. (2020). Investigating language learners’ emotion-regulation strategies with the help of the vignette methodology. System 90:102208. doi: 10.1016/j.system.2020.102208
Brady, S. T., Hard, B. M., and Gross, J. J. (2018). Reappraising test anxiety increases academic performance of first-year college students. J. Educ. Psychol. 110, 395–406. doi: 10.1037/edu0000219
Cattell, R. B. (1943). The description of personality: basic traits resolved into clusters. J. Abnorm. Soc. Psychol. 38, 476–506. doi: 10.1037/h0054116
Chen-Bouck, L., Patterson, M. M., and Peng, A. (2024). Relations of coping strategies and cognitive emotion regulation to Chinese Children’s academic achievement goals and academic performance. Front. Psychol. 15:1454886. doi: 10.3389/fpsyg.2024.1454886
Cheng, F. M., Sheng, N., Zhang, C. M., Zhou, P., and Huang, W. J. (2022). The relationship between the big five personality traits and learning motivation of nursing students in vocational colleges: the mediating effect of attribution methods. Health Vocat. Educ. 40, 131–134.
Chennamaneni, P. R., Echambadi, R., Hess, J. D., and Syam, N. (2016). Diagnosing harmful collinearity in moderated regressions: a roadmap. Int. J. Res. Mark. 33, 172–182. doi: 10.1016/j.ijresmar.2015.08.004
Christenson, S. L., Reschly, A. L., and Wylie, C. (2012). Handbook of research on student engagement. Boston, MA: Springer US.
Costa, P.T., and Mc Crae, R.R. (1992). Revised NEO personality inventory (NEO-PI-R) and NEO five-factor inventory (NEO-FFI) professional manual. Odessa, FL: Psychological Assessment Resources.
Dawes, J. (2008). Do data characteristics change according to the number of scale points used? An experiment using 5-point, 7-point and 10-point scales. Int. J. Mark. Res. 50, 61–104. doi: 10.1177/147078530805000106
Eysenck, H. J., and Eysenck, S. B. G. (2013). Personality structure and measurement (psychology revivals). London Routledge.
Fan, X. (2021). Study on influencing factors of college students’ learning input: a qualitative analysis based on Nvivo11. Res. Teach. 44, 12–19. doi: 10.3969/j.issn.1005-4634.2021.03.004
Finn, J. D., and Zimmer, K. S. (2012). Student engagement: what is it? Why does it matter? Handbook Res. Student Engage., 97–131. doi: 10.1007/978-1-4614-2018-7_5
Fredricks, J. A., Blumenfeld, P. C., and Paris, A. H. (2004). School engagement: potential of the concept, state of the evidence. Rev. Educ. Res. 74, 59–109. doi: 10.3102/00346543074001059
Fredricks, J. A., and McColskey, W. (2012). The measurement of student engagement: a comparative analysis of various methods and student self-report instruments. Handbook Res. Student Engage., 763–782. doi: 10.1007/978-1-4614-2018-7_37
Fredricks, J. A., Wang, M.-T., Schall Linn, J., Hofkens, T. L., Sung, H., Parr, A., et al. (2016). Using qualitative methods to develop a survey measure of math and science engagement. Learn. Instr. 43, 5–15. doi: 10.1016/j.learninstruc.2016.01.009
Fuente, J. D. L., Sander, P., Garzón Umerenkova, A., Urien, B., Pachón-Basallo, M., Luis, O., et al. (2024). The big five factors as differential predictors of self-regulation, achievement emotions, co** and health behavior in undergraduate students. BMC psychology 12:267. doi: 10.1186/s40359-024-01768-9
Fullan, M., Quinn, J., and Mceachen, J. (2018). Deep learning: Engage the world, change the world. Thousand Oaks, CA: Corwin, a Sage Publishing Company.
Goff, M., and Ackerman, P. L. (1992). Personality-intelligence relations: assessment of typical intellectual engagement. J. Educ. Psychol. 84, 537–552. doi: 10.1037/0022-0663.84.4.537
Gong, X. (2023). Relationship between the doctrine of the mean and learning burnout of college students: the mediation of negative cognitive emotion regulation. Chin. J. Health Psychol. 31, 1735–1739. doi: 10.13342/j.cnki.cjhp.2023.11.025
Gross, J. J. (2002). Emotion regulation: affective, cognitive, and social consequences. Psychophysiology 39, 281–291. doi: 10.1017/s0048577201393198
Gunuc, S., and Kuzu, A. (2014). Student engagement scale: development, reliability and validity. Assess. Eval. High. Educ. 40, 587–610. doi: 10.1080/02602938.2014.938019
Handelsman, M. M., Briggs, W. L., Sullivan, N., and Towler, A. (2005). A measure of college student course engagement. J. Educ. Res. 98, 184–192. doi: 10.3200/joer.98.3.184-192
Hu, B. (2005). Development of students:basic category of economics of individual education. Educ. Res. 26, 15–50.
Huang, C., Zhang, L., He, T., Wu, X., Pan, Y., Han, Z., et al. (2023). The role of emotion regulation in predicting emotional engagement mediated by meta-emotion in online learning environments: a two-stage SEM-ANN approach. Educ. Psychol. 43, 736–755. doi: 10.1080/01443410.2023.2254524
Jia, Y. M. (2023). The relationship between proactive personality and learning engagement in middle school students: The mediating role of academic resilience and future time insight. Jinan: University of Jinan.
Jiang, W., Jiang, J., Du, X. P., Gu, D., and Sun, Y. (2018). Study on the effect of teacher support on online learners’ learning engagement. Chin. J. Spec. Educ. 4, 91–96.doi: 10.3969/j.issn.1007-3728.2018.04.015
Kara, A., Ergulec, F., and Eren, E. (2024). The mediating role of self-regulated online learning behaviors: exploring the impact of personality traits on student engagement. Educ. Inf. Technol. 29, 23517–23546. doi: 10.1007/s10639-024-12755-3
Ketchen, D. J. (2013). A primer on partial least squares structural equation Modeling. Long Range Plan. 46, 184–185. doi: 10.1016/j.lrp.2013.01.002
Kuh, G. D., Chen, D., and Laird, T. F. N. (2007). Why teacher-scholars matter: some insights from FSSE and NSSE. Lib. Educ. 4, 40–45.
Laidra, K., Pullmann, H., and Allik, J. (2007). Personality and intelligence as predictors of academic achievement: a cross-sectional study from elementary to secondary school. Personal. Individ. Differ. 42, 441–451. doi: 10.1016/j.paid.2006.08.001
Lang, Y., Gong, S., Cao, Y., and Wu, Y. (2022). The relationship between teacher-student interaction and college students’ learning engagement in online learning: mediation of autonomous motivation and academic emotions. Psychol. Dev. Educ. 38, 530–537. doi: 10.16187/j.cnki.issn1001-4918.2022.04.09
Li, M. J. (2019). The relationship between emotion regulation, interpersonal relationship satisfaction and subjective well-being of secondary vocational students and its intervention. Qufu: Qufu Normal University.
Li, Y., Ni, X., Zhang, W., Wang, J., Yu, C., and Zou, H. (2024). The relationship between work–family conflict and job burnout among primary and secondary school teachers: the role of depression and cognitive reappraisal. Front. Psychol. 15:1438933. doi: 10.3389/fpsyg.2024.1438933
Liu, Z. (2015). On the developments and characteristics of secondary school students’ engagement in learning. Chinese J. Spec. Educ 6, 71–77. doi: 10.3969/j.issn.1007-3728.2015.06.012
Liu, D., Guo, Y. Y., and Zhang, X. M. (2023). Research on the influencing factors of academic emotion on cognitive engagement in mixed learning environment. Digital Education 9, 49–56. doi: 10.3969/j.issn.2096-0069.2023.03.008
Liu, B., Zhan, W., and Liu, J. (2017). Study on the effect of teacher support on online learners’ learning engagement. e-Educ. Res. 38:63–68+80. doi: 10.13811/j.cnki.eer.2017.11.010
Ma, J., Cheng, J., and Han, X. (2017). Initial development process of a student engagement scale in blended learning environment. IEEE Computer Society. doi: 10.1109/eitt.2017.63
Madaus, G. F., and Stufflebeam, D. L. (1989). An interview with Ralph Tyler. Dordrecht: Springer eBooks, 243–272.
Mahama, I., Dramanu, B. Y., Eshun, P., Nandzo, A., Baidoo-Anu, D., and Amponsah, M. A. (2022). Personality traits as predictors of self-regulated learning and academic engagement among college students in Ghana: a dimensional multivariate approach. Educ. Res. Int. 2022, 1–12. doi: 10.1155/2022/2255533
McCrae, R. R., and Costa, P. T. (2004). A contemplated revision of the NEO five-factor inventory. Personal. Individ. Differ. 36, 587–596. doi: 10.1016/s0191-8869(03)00118-1
Morfaki, C., and Skotis, A. (2022). Academic online learning experience during COVID-19 – a systematic literature review based on personality traits. Higher Educ. Skills Work-Based Learn. 13, 697–719. doi: 10.1108/heswbl-03-2022-0062
Ongore, O. (2014). A study of relationship between personality traits and job engagement. Procedia. Soc. Behav. Sci. 141, 1315–1319. doi: 10.1016/j.sbspro.2014.05.226
Pan, P. (2023). A study of the correlation between emotion regulation strategies use and English learning engagement among Chinese junior high school students: Taking students in Suzhou S middle school as an example. Suzhou: Soochow University.
Podsakoff, P. M., MacKenzie, S. B., Lee, J.-Y., and Podsakoff, N. P. (2003). Common method biases in Behavioral research: a critical review of the literature and recommended remedies. J. Appl. Psychol. 88, 879–903. doi: 10.1037/0021-9010.88.5.879
Preston, C. C., and Colman, A. M. (2000). Optimal number of response categories in rating scales: reliability, validity, discriminating power, and respondent preferences. Acta Psychol. 104, 1–15. doi: 10.1016/s0001-6918(99)00050-5
Qiao, T., Sun, Y., Ye, P., Yan, J., Wang, X., and Song, Z. (2024). The association between family functioning and problem Behaviors among Chinese preschool left-behind children: the chain mediating effect of emotion regulation and psychological resilience. Front. Psychol. 15:1343908. doi: 10.3389/fpsyg.2024.1343908
Qu, J., and Wang, Y. (2024). Modelling the interaction between EFL teachers’ personality traits, emotion regulation strategies, and teachers’ depression: a structural equation approach. Eur. J. Educ. 59. doi: 10.1111/ejed.12727
Quigley, M., Bradley, A., Playfoot, D., and Harrad, R. (2022). Personality traits and stress perception as predictors of students’ online engagement during the COVID-19 pandemic. Personal. Individ. Differ. 194:111645. doi: 10.1016/j.paid.2022.111645
Qureshi, A., Wall, H., Humphries, J., and Bahrami Balani, A. (2016). Can personality traits modulate student engagement with learning and their attitude to employability? Learn. Individ. Differ. 51, 349–358. doi: 10.1016/j.lindif.2016.08.026
Sahinidis, A. G., Tsaknis, P. A., Gkika, E., and Stavroulakis, D. (2020). “The influence of the big five personality traits and risk aversion on entrepreneurial intention” in Strategic innovative marketing and tourism: 8th ICSIMAT, northern Aegean, Greece, 2019. (Cham: Springer International Publishing), 215–224.
Santos, A. C., Simões, C., Cefai, C., Freitas, E., and Arriaga, P. (2021). Emotion regulation and student engagement: age and gender differences during adolescence. Int. J. Educ. Res. 109:101830. doi: 10.1016/j.ijer.2021.101830
Schaufeli, W. B., Martínez, I. M., Pinto, A. M., Salanova, M., and Bakker, A. B. (2002). Burnout and engagement in university students. J. Cross-Cult. Psychol. 33, 464–481. doi: 10.1177/0022022102033005003
Shafiee Rad, H., and Jafarpour, A. (2022). Effects of well-being, grit, emotion regulation, and resilience interventions on L2 learners’ writing skills. Read. Writ. Q. 39, 228–247. doi: 10.1080/10573569.2022.2096517
Shi, H. M. (2023). Research on the cultivation of emotional regulation in primary school students based on educational games. Qingdao: Qingdao University.
Steiner, C., Jeszenszky, P., Stebler, V., and Leemann, A. (2023). Extraverted innovators and conscientious laggards? Investigating effects of personality traits on language change. Lang. Var. Chang. 35, 1–28. doi: 10.1017/s0954394523000091
Su, W. C. (2023). Related study between adversity quotient and personality traits and learning engagement of higher vocational nursing students. Health Vocat. Educ. 41, 140–144. doi: 10.20037/j.issn.1671-1246.2023.18.44
Tabachnick, B. G., and Fidell, L. S. (2019). Using multivariate statistics. 7th Edn. Boston: Boston Pearson.
Thompson, R.A., and Goodman, M. (2010). Development of emotion regulation: more than meets the eye, In A.M. Kring, D.M. Sloan, A.M Kring, and D.M Sloan, Emotion regulation and psychopathology: A transdiagnostic approach to Etiology and Treatmen t. Guilford Press, New York, NY, US, pp. 38–58.
Wang, L., Liu, H. C., Li, Z. Q., and Du, W. (2007). Reliability and validity of the emotion regulation scale. Chin. J. Behav. Med. Brain Sci. 16, 846–848. doi: 10.3760/cma.j.issn.1674-6554.2007.09.030
Wu, J., Fu, H., and Zhang, Y. (2023). A meta-analysis of the relationship between perceived social support and student academic achievement: the mediating role of student engagement. Adv. Psychol. Sci. 31:552. doi: 10.3724/sp.j.1042.2023.00552
Wu, R., and Yu, Z. (2023). Relationship between university students’ personalities and e-learning engagement mediated by achievement emotions and adaptability. Educ. Inf. Technol. 29, 10821–10850. doi: 10.1007/s10639-023-12222-5
Xiao, X., Cai, J., and Yang, Q. (2024). The relationship between internet addiction and adolescent learning engagement: the role of future orientation and cognitive reappraisal. Curr. Psychol. 43, 23103–23114. doi: 10.1007/s12144-024-05982-x
Xu, Z., Wang, X., Zhao, Z., and Zhan, N. (2023). The Infiuence of college students’ self-efficacy on learning engagement: the mediating role of emotion regulation. Psychol. Magaz. 18:99–101+105. doi: 10.19738/j.cnki.psy.2023.11.026
Yang, L. (2020). The relationship between emotion regulation strategy and mental health of high school students and its group Counseling intervention. Wuhan: Central China Normal University.
Yu, X., Wang, Y., and Liu, F. (2022). Language learning motivation and burnout among English as a foreign language undergraduates: the moderating role of maladaptive emotion regulation strategies. Front. Psychol. 13:808118. doi: 10.3389/fpsyg.2022.808118
Zhang, J. (2022). A study of the status quo and influencing factors of student engagement in senior high school English class. Changchun: Jilin University.
Zhang, X., Chen, G., and Xu, B. (2020). The influence of group big-five personality composition on student engagement in online discussion. Int. J. Inform. Educ. Technol. 10, 744–750. doi: 10.18178/ijiet.2020.10.10.1452
Zhang, Y., Hu, Y., and Yu, M. (2024). Exploring emotional support and engagement in adolescent EFL learning: the mediating role of emotion regulation strategies. Lang. Teach. Res. doi: 10.1177/13621688241266184
Zhang, Q., and Wang, J. H. (2023). The effects of teacher support on students’ engagement in foreign language classrooms: multiple mediating role of academic emotions. Foreign Lang. China 20, 69–77. doi: 10.13564/j.cnki.issn.1672-9382.2023.05.011
Zhao, K., and Ji, W. (2024). The influence of gratitude and personality traits on career resilience and career success among college students. Front. Psychol. 15:1340200. doi: 10.3389/fpsyg.2024.1340200
Zhao, X., Li, Y., Jin, J., and Jin, G. (2016). Boring tendency and learning burnout:the mediating effect of emotion regulation strategies. Chin. J. Clin. Psych. 24:64–68+80. doi: 10.16128/j.cnki.1005-3611.2016.01.015
Keywords: personality traits, Big Five Personality Traits, learning engagement, emotion regulation, mediating role
Citation: Dang T, Du W, Niu M and Xu Z (2025) The effects of personality traits on learning engagement among college students: the mediating role of emotion regulation. Front. Psychol. 15:1476437. doi: 10.3389/fpsyg.2024.1476437
Received: 05 August 2024; Accepted: 27 December 2024;
Published: 10 January 2025.
Edited by:
Nelly Lagos San Martín, University of the Bío-Bío, ChileReviewed by:
Carolina Bringas Molleda, University of Extremadura, SpainCopyright © 2025 Dang, Du, Niu and Xu. This is an open-access article distributed under the terms of the Creative Commons Attribution License (CC BY). The use, distribution or reproduction in other forums is permitted, provided the original author(s) and the copyright owner(s) are credited and that the original publication in this journal is cited, in accordance with accepted academic practice. No use, distribution or reproduction is permitted which does not comply with these terms.
*Correspondence: Zhenguo Xu, cWZudXh6Z0BxZm51LmVkdS5jbg==
Disclaimer: All claims expressed in this article are solely those of the authors and do not necessarily represent those of their affiliated organizations, or those of the publisher, the editors and the reviewers. Any product that may be evaluated in this article or claim that may be made by its manufacturer is not guaranteed or endorsed by the publisher.
Research integrity at Frontiers
Learn more about the work of our research integrity team to safeguard the quality of each article we publish.