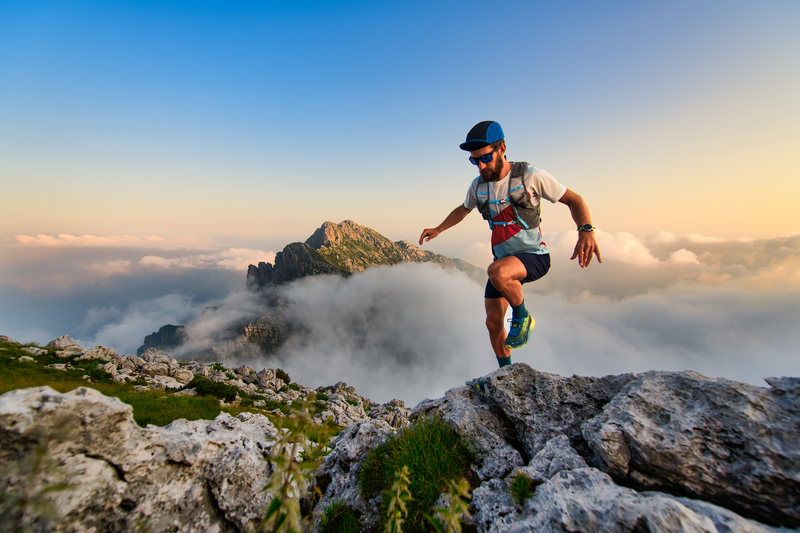
94% of researchers rate our articles as excellent or good
Learn more about the work of our research integrity team to safeguard the quality of each article we publish.
Find out more
ORIGINAL RESEARCH article
Front. Psychol. , 18 December 2024
Sec. Positive Psychology
Volume 15 - 2024 | https://doi.org/10.3389/fpsyg.2024.1474523
Objectives: As a population at high risk for psychological distress, healthcare workers typically experience varying degrees of anxiety, depression, and burnout. Studies have found that depression and anxiety have a negative impact on the mental health domain of burnout in healthcare workers. However, little is known about the symptom-to-symptom interactions between these psychological outcomes. This study aims to elucidate the characteristics of depression, anxiety, and burnout networks among healthcare workers.
Methods: We recruited 846 healthcare workers from March to April 2023 from three hospitals. A total of 826 healthcare workers completed the General Information Scale, the 16-item Quick Inventory of Depressive Symptomatology–Self-Report (QIDS-SR16), the Self-rating Anxiety Scale (SAS), and the Burnout Clinical Subtype Questionnaire (BCSQ)-36. The network models were constructed using network analysis. The expected influence and the bridge expected influence of nodes were calculated. The stability and accuracy of the network was assessed.
Results: The results showed that the core symptoms in the symptom network mainly included QIDS8 (Energy/Fatigability), SAS3 (Easily upset or frightened), SAS11 (Dizzy), SAS8 (Tiredness), SAS10 (Tachycardia) and BCSQ3 (Worn-out), and the key nodes connecting these symptoms were QIDS2 (Sad mood), SAS20 (Have nightmares), BCSQ3 (Worn-out), SAS8 (Tiredness), QIDS8 (Energy/Fatigability), QIDS4 (Concentration/decision-making) and SAS4 (Madness).
Conclusion: Unique pathways of association between burnout, depression, and anxiety were found to exist. Interventions targeting core symptoms can maximize the improvement of depression, anxiety, and burnout, provide a deeper understanding of the relationship between the three conditions, and provide a target and basis for psychological interventions to improve the emotional wellbeing of healthcare workers and enhance their mental health.
Healthcare workers are very valuable resource in the healthcare service industry. In the context of the COVID-19 pandemic, service professionals, including healthcare workers and educators, are often overworked and face significant workloads and psychological pressure (Nucera et al., 2023; Chirico et al., 2022). Once stress becomes chronic, it can translate into anxiety, depression, and burnout (Rehman et al., 2023; Chirico et al., 2023). As a high-risk group for psychological distress, medical workers usually experience varying degrees of anxiety, depression, and burnout (Rizzo et al., 2023; Saragih et al., 2021; Sun et al., 2023). The study (Parmar et al., 2022) predicted that occupational psychological distress, such as depression, anxiety, and burnout, are the determinants that affect turnover intention. Therefore, the mental health of healthcare workers needs urgent attention. Although previous studies (Yıldırım and Ashraf, 2024; Golonka et al., 2019; Ryan et al., 2023) have explored the relationship between burnout, anxiety, and depressive symptoms, previous research still only scratches the surface due to the complexity of the relationship between the three, as well as the shortcomings of traditional methods.
The undertaken research aims to use a network approach to investigate the relationship between burnout, anxiety, and depressive symptoms among Chinese healthcare workers. In addition to assessing the stability and accuracy of the observed model, the study identified central and bridging symptoms in the network. Fundamentally, the main motivation for conducting this study is to improve the understanding of occupational psychological distress among Chinese healthcare workers. Considering the complexity of depression, anxiety, and burnout symptoms among healthcare workers, this study constructed a visual network structure to identify core node symptoms and bridge symptoms. The novelty of this study lies in the adoption of an emerging research methodology, network analysis, and a data-driven approach to studying the interactions between variables, which allows him to understand the degree of closeness of the links between variables, which is rapidly emerging and is widely used in the field of psychology due to its visualization of images and its ability to explore the relationships between variables (Wei et al., 2024).
The significance of this study is that it comprehensively analyses the relationship between burnout, anxiety, and depression symptoms, provides a more systematic and macroscopic understanding of the symptom characteristics of the network, compensates for the traditional method of judging the importance of symptoms by the level of scores, and provides a target point for improving the burnout, anxiety, and depression symptoms of healthcare workers to improve the effectiveness of interventions for related symptoms. This study will help hospital management to develop not only policies to reduce anxiety, depression, and burnout in the hospital setting but also strategies to alleviate occupational psychological distress among healthcare workers to prevent employee accidents, which can be harmful to both the employee and the organization. Finally, the study fills a research gap regarding anxiety, depression, and burnout, and its results provide a basis for future research.
The rest of the paper is structured as follows: the second section covers the literature review. The third section contains research methodology presentation. The fourth section elaborates on the results, while sections five and six include the discussions, limitations and areas for future research, respectively. Finally, we present conclusions.
Burnout is defined as an emotionally depleting response of an individual to prolonged work stress and is a psychological syndrome of depersonalization and low achievement (Tavella et al., 2021). Burnout syndrome includes three syndrome characteristics (frenetic, under-challenged, and worn-out) (Farber, 1990). The frenetic includes a kind of people who are highly engaged and dedicated, and their characteristics are that they devote a lot of time and energy to their work (Montero-Marín et al., 2009). The under-challenged is described as an individual who has no interest in work and superficially performs tasks due to a lack of challenge, motivation, or desire to participate (Montero-Marín et al., 2009). The worn-out includes those whose participation in work has decreased to the point of ignoring their job responsibilities (Montero-Marín et al., 2009). Burnout among healthcare workers has become a global public health problem. One meta-analysis (Aymerich et al., 2022) showed that the prevalence of burnout among healthcare workers exposed to COVID-19 was 37%. In another meta-analysis (Ge et al., 2023) of 30 studies covering more than 94 countries showed a global prevalence of nursing burnout of 0.95%, with an increasing trend over time. Several studies have examined the correlates and potential consequences of burnout. A large sample study (Sun et al., 2023) found that access to psychosocial support, health status, relationship with family, and sleep deprivation were common factors for burnout among healthcare workers. The potential consequences of burnout include decreased health status, work performance, job satisfaction, loss of work enthusiasm, and even lead to uncoordinated doctor-patient relationship, poor colleague relationship, absenteeism, and leaving the job, which directly affects the quality and effectiveness of medical services (Beschoner et al., 2019; Hodkinson et al., 2022; Sohrabi et al., 2022; Zhang et al., 2023).
In addition to burnout, anxiety and depression are highly prevalent psychological problems among healthcare workers. A systematic evaluation and meta-analysis (Aymerich et al., 2022) showed that 33% of healthcare workers exposed to COVID-19 experienced depressive symptoms, and 42% experienced anxiety symptoms. Anxiety and depression are also highly prevalent among Chinese healthcare workers. According to a meta-analysis of Chinese healthcare workers (Xiong et al., 2022), the prevalence of moderate to severe anxiety and depression during the pandemic was 17% and 15%, and that of mild to severe anxiety and depression was estimated to be 37 and 39%, respectively. Compared with the general Chinese population, the prevalence of depression and anxiety was high among women and frontline healthcare workers (Bareeqa et al., 2021). A cross-sectional study (Zhang et al., 2021) reported that hardworking and overcommitted public health workers were at higher risk of depression and anxiety. In addition, anxiety and depression have been found to significantly affect the quality of life and productivity of healthcare workers (An et al., 2020; Wong et al., 2024).
Anxiety and depression are highly correlated common psychological disorders and frequently comorbid (Suradom et al., 2020). Anxiety has been shown to be a predictor of depression, preceding depressive episodes (Warner et al., 2008). Combined burnout, anxiety, and depression are also common among healthcare workers. According to two models of burnout, depression is an important determinant of exhaustion, and depression and anxiety also play an important role in explaining burnout syndromes (Golonka et al., 2019). A systematic evaluation and meta-analysis (Koutsimani et al., 2019) showed that symptoms of depression and anxiety were positively associated with high levels of burnout, although these were not overlapping constructs. A mediation effects analysis (Liu et al., 2023) showed that depression acted as a mediator between anxiety and burnout. Another study (Huang et al., 2024) showed that approximately 37.1% of the variance in depression was explained by a component of burnout. A Korean study (Jihn et al., 2021) found that anxiety and depression predicted burnout in hospital health workers.
Network analysis, as a new method for examining the comorbidity of two or more diseases or syndromes, constructs visual symptom network graphs by quantifying complex connections between symptoms. In network analysis, more influential symptoms are placed at the center of the network, and the remaining relevant symptoms are placed around the network, aiming to calculate indicators of the centrality of symptoms in the network (Borsboom and Cramer, 2013). Network analyses are now widely used in the study of psychological conceptualization and psychiatric disorders, and provide a finer granularity of analysis. For example, several studies have used network analysis to elucidate the structure and central symptoms of anxiety and depression (Chen et al., 2024; He et al., 2023; Zainal and Newman, 2024). However, to the best of our knowledge, no studies have examined Chinese healthcare workers with comorbid burnout, depression, and anxiety symptoms via network analysis.
This study used a cross-sectional design with convenience sampling to select physiatrists and nurses with licensed medical practitioners from tertiary-level A medical institutions in Southwest China. We designed an online survey supported by www.wjx.cn, and systematically trained surveyors sent rapid response codes for the survey to physicians and nurses licensed to practice medicine from tertiary-level A medical institutions in Zigong, China. Healthcare professionals who submitted the online questionnaire were considered to have agreed to participate in this study. The Inclusion criteria were: (a) holding a certificate of physician/nurse qualification from the People’s Republic of China; (b) At least six months of clinical or managerial experience (Six months or more of work is required to qualify for clinical work In China.); (c) have basic telephone or computer skills; and (d) Volunteered to participate in this study and sign the informed consent form. Exclusion criteria were: (a) Those who absent from work, vacation or further training; and (b) Those who suffered a major accident or received psychological treatment. The study strictly followed the principles of the Declaration of Helsinki and was approved by the Ethics Committee of the Fourth People’s Hospital of Zigong.
General data collected include demographic information (gender, age, years of working experience, marital status, whether the child is an only child, health status, occupation, education level, and the presence of children).
Burnout symptoms were assessed using the Chinese version of the BCSQ-36 (Chen et al., 2023). The BCSQ-36 is a burnout measurement tool developed by Montero-Marín and García-Campayo (2010) comprising 36 items divided into three subscales: frenetic, under-challenged, and worn-out. Each subscale follows a 7-point Likert scale, with each entry scored on a scale of 1 to 7, where 1 = completely disagree and 7 = completely agree. Higher scores indicate more severe burnout. The BCSQ-36 showed good reliability and validity in the Chinese cultural context, with Cronbach’s α coefficient was 0.90 (Chen et al., 2023). In this study, Cronbach’s α coefficient of the scale was 0.90.
Depressive symptoms were assessed using the Chinese version of QIDS-SR16 (Liu et al., 2013). The QIDS-SR16 is a 16-item self-report scale with 9-dimension that assesses residual symptoms and their severity in respondents (Rush et al., 2003). The questionnaire comprises 16 items, which are categorized into four levels ranging from 0 to 3. The total score of the scale ranges from 0 to 27, with higher scores indicating more severe depressive symptoms. The QIDS-SR16 has good psychometric characteristics in the Chinese population. The Cronbach’s α coefficients was 0.82, with good reliability and validity (Liu et al., 2013; Zhen et al., 2020). In this study, Cronbach’s α coefficient of the scale was 0.78.
Anxiety symptoms were assessed using the Chinese version of SAS (Liu et al., 1997). The SAS is a clinical measurement tool developed by Zung (1971) in 1971 to rate patients’ subjective symptoms of anxiety. The SAS comprises 20 items rated on a 4-point Likert scale based on the respondent’s feelings during the last week. The cumulative score for each item is the total SAS score, with a higher total score indicating a greater degree of anxiety. SAS has been widely used in the Chinese population with Cronbach’s α coefficients of 0.85 (Liu et al., 1997; Sun et al., 2012). Cronbach’α for the present sample was 0.670.
This cross-sectional study used an anonymous web-based questionnaire to collect information. The purpose and methodology of the study, the questionnaire, and the process of information collection were introduced by the researcher to the head of each hospital. After obtaining the consent of the relevant head of the hospital, the relevant department was formally requested to send the electronic questionnaire to the healthcare workers who met the requirements. The participants were introduced to the study’s purpose and methodology so they could fully understand the questionnaire’s content. All the participants of the study filled informed consent form. The data collection period of this study was from March to April 2023. A total of 846 healthcare professionals completed the survey. After excluding questionnaires that were not answered completely, 826 participants were finally included in the study.
We used R software for data statistics and network visualization. A Gaussian graphical model (GGM) was used to fit the data and construct the network (Costantini et al., 2015). In network modeling, each symptom is considered a node, and correlations between individual symptoms are considered edges (Beard et al., 2016). Edges can be positive (depicted by green lines) or negative (depicted by red lines), and the coarseness of the edges reflects the strength of the association between nodes, with thicker edges indicating stronger associations (Fruchterman and Reingold, 1991).
In order to determine the importance of each node in the network and to explore the bridge nodes in the network that act as a bridge between depression, anxiety, and burnout, this study calculated expected influence (EI) and bridge expected influence (BEI) using the bridge function in the qgraph package and the R package network tools (version 1.3.0), respectively (McNally, 2016). The EI was weighted by the absolute magnitude of related edges, and the higher the EI of a node, the more central it is (Blanchard et al., 2021). The BEI value is used to assess the EI of a node from one community on nodes of another community, and nodes with higher bridge expected impacts were thought to potentially have a higher risk of passing on from this symptom cluster to other symptom clusters (McNally, 2016). Bridging nodes are typically the top 20–30% of nodes in the study for bridging strength centrality or expected influence (Jones et al., 2021). Due to the large number of entries in the scale of this study, the points with the top 20% of EI values were used as central nodes, and the nodes with the top 30% of BEI in the network were set as bridging nodes.
Additionally, the stability of the edge weights and the expected impact of the nodes were also assessed using a non-parametric self-help method for calculating the 95% confidence intervals (CIs) and a sample descent self-help method for calculating the correlation stability (CS) coefficients, respectively, which should not be lower than 0.25 and preferably higher than 0.50 (Epskamp et al., 2018). Bootstrap difference tests were used to assess whether the difference between two edge weights or two node expected impacts was statistically significant (α = 0.05).
A total of 826 healthcare workers qualified for this study, including 684 females (82.8%) and 142 males (17.2%). Their mean age was 31.65 ± 7.91 years, and they worked a mean 9.96 ± 8.36 years. Their marital status was 543 married (65.7%), 256 unmarried (31.0%), and 27 divorced (3.3%). 548 were only children (66.3%), and 278 were not (33.7%). 577 were in good health (69.6%), 243 were in fair health (29.4%), and 6 were in poor health (0.7%). 694 participants were nurses (84.0%) and 132 doctors (16.0%). 14 attended secondary school (1.7%), 285 tertiary school (34.5%), 507 bachelor’s degree (61.4%), and 20 master’s degree (2.4%). 508 participants had children (61.5%), and 318 had no children (38.5%). Table 1 presents abbreviations, items, mean scores, EI, and BEI for each node.
Figure 1 presents the network visualization of depression, anxiety, and work burnout. The network has 32 nodes, with 237 non-zero edges out of 496 possible edges, and showed excellent stability (0.67 for EI and BEI) and accuracy (Supplementary Figures 1, 2). The most strongly connected edges appeared within their respective communities rather than across communities. Within the depression community, the most significant edge was QIDS7 (General interest)–QIDS8 (Energy/Fatigability) (weight = 0.231). Within the anxiety community, the strongest edge was SAS2 (Scared for no reason)–SAS3 (Easily upset or frightened) (weight = 0.373), followed by SAS9 (Akathisia)–SAS17 (Excessive sweating) (weight = 0.276). Within the burnout community, BCSQ2 (Underchallenged)–BCSQ3 (Worn-out) (weight = 0.613) was the strongest edge. The most robust transdiagnostic edge throughout the community was SAS20 (Have nightmares)–QIDS1 (Sleep problems) (weight = 0.178), followed by SAS8 (Tiredness)–QIDS8 (Energy/Fatigability) (weight = 0.149), SAS20 (Have nightmares)–QIDS2 (Sad mood) (weight = 0.144), and SAS8 (Tiredness)–BCSQ3 (Worn-out) (weight = 0.113). All edge weights are listed in Supplementary Table 1.
The EI of the nodes is shown in Figure 2A, where the horizontal axis indicates the magnitude of the expected impacts, and the closer to the right side, the larger the expected impacts. As shown in Figure 2A, the nodes with the highest EI are QIDS8 (Energy/Fatigability), SAS3 (Easily upset or frightened), SAS11 (Dizzy), SAS8 (Tiredness), SAS10 (Tachycardia), and BCSQ3 (Worn-out), which suggests that they are strongly and closely linked to other variables in the network. The BEI of the nodes is shown in Figure 2B, where the horizontal axis indicates the magnitude of the expected bridge impact, and the closer to the right side, the larger the expected bridge impact. As shown in Figure 2B, the nodes with the highest BEI are QIDS2 (Sad mood), SAS20 (Have nightmares), BCSQ3 (Worn-out), SAS8 (Tiredness), QIDS8 (Energy/Fatigability), QIDS4 (Concentration/decision-making), and SAS4 (Madness), which suggests that they serve as important bridges within the network. Figure 3 shows the network structure of the burnout, depression, and anxiety cluster with bridging connections, where blue nodes represent bridge nodes, and yellow nodes represent items in the SAS scale. Orange nodes represent items in the BCSQ scale, and green nodes represent items in the QIDS scale. In addition, bootstrapped difference tests between expected influence and bootstrapped difference tests between bridge expected influence are shown in Supplementary Figures 3, 4.
Figure 2. The plot of centrality indices of all symptoms within the network (N = 826). The (A) is the plot of expected influence, and (B) is the plot of bridge expected influence.
Figure 3. Network structure of burnout, depression, and anxiety showing bridge connections (N = 826).
This study explored the complex interactions between the microscopic symptoms of depression, anxiety, and burnout in Chinese healthcare workers using psychological network analysis. We identified several central symptoms [i.e., QIDS8 (Energy/Fatigability), SAS3 (Easily upset or frightened), SAS11 (Dizzy), SAS8 (Tiredness), SAS10 (Tachycardia) and BCSQ3 (Worn-out)] and bridge symptoms [i.e., QIDS2 (Sad mood), SAS20 (Have nightmares), BCSQ3 (Worn-out), SAS8 (Tiredness), QIDS8 (Energy/Fatigability), QIDS4 (Concentration/decision-making) and SAS4 (Madness)]. QIDS8 (Energy/Fatigability), SAS8 (Tiredness), and BCSQ3 (Worn-out) were both central and bridge symptoms.
Our findings suggest that there may be comorbidities of burnout, depression, and anxiety, which is consistent with previous studies involving medical students (Ernst et al., 2021; Peng et al., 2023). However, burnout is conceptually distinct from anxiety or depression, and burnout has been categorized previously as a separate work-related phenomenon (de Amorim Macedo et al., 2023). The core criteria of anxiety and depression may not apply to burnout, as burnout may focus only on anxiety and worry associated with occupational stress. Depression is more closely related to burnout than anxiety, possibly because burnout and depression are similarly related to work stressors (Verkuilen et al., 2021). Burnout is a state of physical and mental fatigue and exhaustion that occurs when an individual is under the weight of work, and depression can also be caused by job stress. The complex and unique relationship between burnout, depression, and anxiety provides further evidence that symptoms of burnout in healthcare workers should not be viewed as a single, unified syndrome, but rather as a multidimensional syndrome, consistent with previous research (Bianchi et al., 2015). Moreover, our results suggest that burnout, anxiety, and depression are closely related on one hand, but, on the other, each has its unique characteristics. This finding provides a plausible explanation for why the debate of whether burnout overlaps with depression or anxiety continues to this day (Ahola et al., 2005; Bianchi et al., 2015).
The present study shows that depression and anxiety symptoms seem to present a closer link in the symptom network. The co-morbid relationship between the two seems to exist not only in the specific group of healthcare workers. With regard to the co-morbidity between the two, the US National Comorbidity Survey stated that approximately 50% of people with major depressive disorder have one or more anxiety disorders. Anxiety symptoms and depressive symptoms presented by different populations have been shown to be highly correlated (Beard et al., 2016; Kessler et al., 2003; Park and Kim, 2020; Rouquette et al., 2018). The interdependence between depression and anxiety has long been proposed and described, with early conceptualizations of depression emphasizing the central role of anxiety in depression (Bui and Fava, 2017). The American Psychological Association stated that the depressive response is considered an attenuated form of anxiety (Mendelson, 1995). There are multiple possible mechanisms for anxiety-depression co-morbidity and symptom correlation, one of which is that the two share a common pathogenic mechanism. Current hypotheses to explain this common pathogenic mechanism include dysfunction in the serotonergic system (Bui and Fava, 2017), and altered activation and connectivity of the ventral cingulate gyrus and amygdala (Niu et al., 2017).
Previous studies have found that, in networks composed of different communities, the strongest edges generally exist within communities rather than cross-community edges (Li et al., 2023). The results of the network analysis in this study reaffirmed this finding. Specifically, the strongest edges were present in the burnout community [i.e., BCSQ2 (Underchallenged)–BCSQ3 (Worn-out)], anxiety community [i.e., SAS2 (Scared for no reason)–SAS3 (Easily upset or frightened) and SAS9 (Akathisia)–SAS17 (Excessive sweating)], and depression community [i.e., QIDS7 (General interest)–QIDS8 (Energy/Fatigability)]. The close relationship between BCSQ2 (Underchallenged)–BCSQ3 (Worn-out) has been supported by previous studies. The under-challenged subtype describes that individuals respond to work superficially through indifference and detachment. Although they have not been ignored, they have not participated much. The worn-out subtype describes that individuals cope with work-related difficulties by neglecting their responsibilities, that is, they lack participation in their work so that they will give up when facing any difficulties (Montero-Marín et al., 2009). In this study, the relationship between them is the closest, which may be because theoretically, the three subtypes of job burnout can be divided into a continuum according to their devotion to work-related tasks, from frenetic subtype (high devotion) to under-challenged subtype (moderate devotion) to worn-out subtype (low devotion), which correspond to the values of participation, indifference and neglect. Of these, BCSQ2 (Underchallenged) and BCSQ3 (Worn-out) reflect the deterioration of burnout symptoms from moderate to severe (Montero-Marín and García-Campayo, 2010). In addition, SAS2 (Scared for no reason)–SAS3 (Easily upset or frightened) and SAS9 (Akathisia)–SAS17 (Excessive sweating) are the edges connecting the second and third strongest in the network, respectively. “Scared for no reason” refers to a lesser degree of fear, which is often an internal experience, while “Easily upset or frightened” refers to a heavier degree of intense and uncontrollable fear, which is often accompanied by physical reaction, that is, the long-term accumulation of fear leads to the expression of strong panic (Craske et al., 2017). Previous studies (Zyss et al., 2009) have emphasized the close relationship between akathisia and hyperhidrosis. Akathisia is a kind of restless movement, which is manifested by uncontrollable agitation, body restlessness, and the uncomfortable feeling that he can’t sit still because he wants to walk back and forth or stand still. Subjectively, patients have the impulse to be restless and forced to move, and objectively, they often perform repetitive movements, especially in the lower limbs, such as constantly swinging or crossing their legs, constantly exchanging the relative positions of their legs, constantly pacing and fidgeting, resulting in Excessive sweating. The possible explanation for the strong connection between QIDS7 (General interest) and QIDS8 (Energy/Fatigability) is that the huge workload and pressure make medical workers devote most of their energy to their work, unable to balance the time between work and pursuing interests and hobbies, and the interest level decreases over time.
Furthermore, strong cross-community edges are key edges that lead to activation and contagion between different communities, which is essential to the emergence and persistence of symbiotic psychological issues. Specifically, this study identified four strong cross-community edges: SAS20 (Have nightmares)–QIDS1 (Sleep problems), SAS8 (Tiredness)–QIDS8 (Energy/Fatigability), SAS20 (Have nightmares)–QIDS2 (Sad mood) and SAS8 (Tiredness)–BCSQ3 (Worn-out). These findings hint the critical pathways of SAS20 (Have nightmares)–QIDS1 (Sleep problems), SAS8 (Tiredness)–QIDS8 (Energy/Fatigability), SAS20 (Have nightmares)–QIDS2 (Sad mood) may be the underlying mechanisms for the strong association between anxiety and depression, often leading to their co-occurrence. Sleep disorder mainly refers to abnormal sleep volume; Abnormal behaviors including night terrors and nightmares (Akman et al., 2015) often occur during sleep and are often accompanied by anxiety, depression, and other negative emotions (Yennurajalingam et al., 2016). With regard to the connection between SAS20 (Have nightmares) and QIDS1 (Sleep problems), the possible explanation is that nightmares can lead to insomnia, and people cannot sleep because they are afraid of nightmares (Stefani and Högl, 2021). As for the connection between SAS8 (Tiredness) and QIDS8 (Energy/Fatigability), the possible reason is that medical workers can’t stay energetic due to physical and psychological fatigue, and then feel weak. About the connection between SAS20 (Have nightmares) and QIDS2 (Sad mood), it may be that frequent nightmares cause sadness. Additionally, the connective pathway between SAS8 (Tiredness) and BCSQ3 (Worn-out) may be the key connection that activates the development of anxiety and burnout. One of the characteristics of the worn-out subtype is “lack of control,” describing feelings of powerlessness due to dealing with uncontrollable situations (Montero-Marín and García-Campayo, 2010), which may go some way to explaining the relationship between fatigue and exhaustion in healthcare workers. Previous research has tended to support the conclusion that depression, anxiety, and burnout co-exist and that the four strong trans-community margins described above may be a key underlying mechanism that leads to the co-existence of all three, which needs to be explored in further research.
Centrality results indicated that QIDS8 (Energy/Fatigability) had the highest EI, followed by SAS3 (Easily upset or frightened), SAS11 (Dizzy), SAS8 (Tiredness), SAS10 (Tachycardia), and BCSQ3 (Worn-out). In other words, QIDS8 (Energy/Fatigability) was the most central symptom in the anxiety-depression-burnout network of healthcare workers and should be considered the driver and trigger of all psychological stress symptoms. A previous study on COPD patients (Yohannes et al., 2022) also found that “Energy” had a high centrality weight and was one of the central symptoms activating a network of depression and anxiety symptoms. Another study on nasopharyngeal cancer patients (Zhan et al., 2024) also confirmed the strong centrality of “Energy.” According to the DSM-5, low energy is a major symptom of depression, while panic, dizziness, fatigue, and palpitations are possible physical signs of anxiety (American Psychiatric Association, DSMTF and Association, American Psychiatric, 2013). We speculate that the lack of energy in healthcare workers makes it difficult for them to remain enthusiastic and focused on their work, which in turn trigger depression, anxiety, and burnout. Additionally, the EI for SAS3 (Easily upset or frightened) was second only to that for QIDS8 (Energy/Fatigability), suggesting that “Easily upset or frightened” may be another important trigger in the network with a high potential to activate other neighboring symptoms and further lead to serious mental problems. The centrality of “Easily upset or frightened” is confirmed by the above results on the edges, that is, SAS2 (Scared for no reason)–SAS3 (Easily upset or frightened) has the second strongest connection in the entire network. Fright is a particularly strong fear conditioning that can lead to excessive psychophysiological responses to stimuli (Olivera-Pasilio and Dabrowska, 2023) and may therefore feature prominently in the depression-anxiety-burnout network. This study highlights the urgency of prioritizing screening and intervention for QIDS8 (Energy/Fatigability), SAS3 (Easily upset or frightened), SAS11 (Dizzy), SAS8 (Tiredness), SAS10 (Tachycardia), and BCSQ3 (Worn-out) symptoms in healthcare workers to minimize the impact on mental health.
The results of bridge centrality suggested that QIDS2 (Sad mood), SAS20 (Have nightmares), BCSQ3 (Worn-out), SAS8 (Tiredness), QIDS8 (Energy/Fatigability), QIDS4 (Concentration/decision-making) and SAS4 (Madness) had the highest BEI and should be considered key bridge symptoms leading to the comorbidity of depression, anxiety and burnout. The top three were QIDS2 (Sad mood), SAS20 (Have nightmares), and BCSQ3 (Worn-out). QIDS2 (Sad mood) was the most critical bridge symptom in the network, a finding supported by a large-sample systematic evaluation (Cai et al., 2024). This systematic evaluation identified sadness as the most common bridge-centered symptom in the depression-anxiety network. BCSQ3 (Worn-out) was also an important bridge symptom, which is consistent with the results of a study (Peng et al., 2023) of the network of burnout, depression, anxiety, and dropout intention in undergraduate medical students. In addition, the above marginal results suggest that the bridging of these nodes may be realized through specific pathways, e.g., the bridging of SAS8 (Tiredness) may be realized through the SAS8 (Tiredness)–QIDS8 (Energy/Fatigability) energy level, and SAS8 (Tiredness)–BCSQ3 (Worn-out) specific pathways. This finding provides insights for hospital administrators looking to target interventions that are less labor intensive and more effective, i.e., interventions that target “Tiredness” may achieve a more significant double or even triple-stacking effect. Specifically, when healthcare workers are severely anxious, interventions targeting “SAS8 (Tiredness)” not only help to alleviate anxiety but also reduce the psychiatric crises of depression and burnout through an intrinsic link to QIDS8 (Energy/Fatigability) and BCSQ3 (Worn-out). It is also worth noting that SAS8 (Tiredness), BCSQ3 (Worn-out), and QIDS8 (Energy/Fatigability) are important central as well as bridging symptoms in the current network model. These findings suggest that interventions aimed at reducing SAS8 (Tiredness), BCSQ3 (Worn-out), and QIDS8 (Energy/Fatigability) in Southwest China, may not only reduce anxiety-depressive symptoms but also reduce the incidence of burnout.
This study has several implications for clinical practice and management. In order to detect and reduce depression, anxiety and burnout among healthcare workers early, hospital administrators and policymakers must conduct timely screening and targeted interventions for specific symptoms. Specifically, to address the psychological symptoms of “Energy/Fatigability,” managers can take necessary interventions, including mindfulness-based interventions, cognitive behavioral therapy, stress inoculation therapy, psychoeducation and stress management (Moore et al., 2024). For the “Easily upset or frightened” symptoms, Managers can organize startle-relieving workshops and invite healthcare workers to share measures to mitigate the startle response to help healthcare workers develop optimal coping strategies (Luger and Koziol, 2024). For the “Have nightmares,” moderate-intensity aerobic exercise, which includes walking at a moderate pace, water aerobics, dancing, recreational swimming, gardening, table tennis, and climbing stairs at a moderate pace, is a cost-effective health-promoting strategy that improves the quality and duration of sleep (Okechukwu et al., 2022). Nursing managers must be vigilant about “Sad mood” in the depressed population and should implement potential prevention strategies as soon as possible. Healthcare organizations can conduct online surveys for early screening of depressed nurses and provide timely professional psychotherapy (Moore et al., 2024). For the “Worn-out” symptoms in the burnout group, managers can organize appropriate relaxation training for healthcare workers before their shifts, such as positive thinking meditation, breathing relaxation or playing some soothing music (Jones, 2019). This can improve their mental health as well as their professional performance.
Our study has several limitations. Firstly, due to the cross-sectional nature of the study design, we were unable to determine causal relationships or long-term dynamic changes between symptoms, although the primary findings provide a basis for future highly focused causal hypotheses. Future studies should conduct longitudinal cross-lagged network analyses to determine the direction between psychiatric symptoms and provide more accurate information for interventions. Second, the use of convenience sampling may affect the representativeness of our sample. The majority of subjects were female, which may affect the generalizability of the results. Future studies should include more male healthcare workers or verify the applicability of our results in other populations. Thirdly, the assessments used in our study were based on self-reports and therefore may be subject to recall bias and/or social desirability bias. Fourth, symptoms of depression, anxiety, and burnout were highly correlated with factors such as social support, workload, and economic conditions, which we did not collect in this study. Future research needs to further examine how these factors affect the depression-anxiety-burnout network in the healthcare worker population, thus providing valuable insights into the prevention and treatment of depression, anxiety and burnout in healthcare workers. Finally, as this study only recruited healthcare workers in Southwest China, caution should be exercised in generalizing the findings to populations in other countries. There is a need to extend the study to healthcare workers in other countries around the world to further assess the external validity of the findings.
In summary, our study assessed the network structure of depression, anxiety, and symptoms among healthcare workers in Southwest China. We found that QIDS8 (Energy/Fatigability) was the strongest central symptom in the network, whereas QIDS2 (Sad mood), SAS20 (Have nightmares), BCSQ3 (Worn-out), SAS8 (Tiredness), QIDS8 (Energy/Fatigability), QIDS4 (Concentration/decision-making) and SAS4 (Madness) were the bridging symptoms in the network. These findings provide new insights into the symptom-symptom relationships of depression, anxiety, and burnout, and are valuable for the prevention and treatment of these three common psychiatric disorders among healthcare workers.
The raw data supporting the conclusions of this article will be made available by the authors, without undue reservation.
The studies involving human participants were reviewed and approved by the Ethics Committee of Zigong Fourth People’s Hospital. The participants provided their written informed consent to participate in this study.
CY: Data curation, Investigation, Software, Validation, Writing – original draft, Writing – review and editing. YC: Data curation, Writing – review and editing. XW: Writing – original draft. PX: Writing – original draft. JS: Writing – original draft. LY: Writing – original draft. YF: Writing – review and editing.
The authors declare that financial support was received for the research, authorship, and/or publication of this article. This work was supported by the Sichuan Medical Research Project Program (grant no. Q21074).
We thank all the participants in this study.
The authors declare that the research was conducted in the absence of any commercial or financial relationships that could be construed as a potential conflict of interest.
All claims expressed in this article are solely those of the authors and do not necessarily represent those of their affiliated organizations, or those of the publisher, the editors and the reviewers. Any product that may be evaluated in this article, or claim that may be made by its manufacturer, is not guaranteed or endorsed by the publisher.
The Supplementary Material for this article can be found online at: https://www.frontiersin.org/articles/10.3389/fpsyg.2024.1474523/full#supplementary-material
Supplementary Figure 1 | Centrality stability for network.
Supplementary Figure 2 | Edge-weight accuracy for network.
Supplementary Figure 3 | Bootstrapped difference tests between expected influence.
Supplementary Figure 4 | Bootstrapped difference tests between bridge expected influence.
Supplementary Table 1 | Edge weights between all nodes of network.
BCSQ, Burnout Clinical Subtype Questionnaire; BEI, bridge expected influence; CI, confidence intervals; CS, correlation stability; EI, expected influence; GGM, Gaussian graphical model; QIDS, Quick Inventory of Depressive Symptomatology; SAS, Self-rating Anxiety Scale.
Ahola, K., Honkonen, T., Isometsä, E., Kalimo, R., Nykyri, E., Aromaa, A., et al. (2005). The relationship between job-related burnout and depressive disorders–results from the Finnish Health 2000 Study. J. Affect. Disord. 88, 55–62. doi: 10.1016/j.jad.2005.06.004
Akman, T., Yavuzsen, T., Sevgen, Z., Ellidokuz, H., and Yilmaz, A. U. (2015). Evaluation of sleep disorders in cancer patients based on Pittsburgh Sleep Quality Index. Eur. J. Cancer Care 24, 553–559. doi: 10.1111/ecc.12296
American Psychiatric Association, DSMTF and Association, American Psychiatric (2013). Diagnostic and statistical manual of mental disorders: DSM-5. Washington, DC: American Psychiatric Association.
An, Y., Yang, Y., Wang, A., Li, Y., Zhang, Q., Cheung, T., et al. (2020). Prevalence of depression and its impact on quality of life among frontline nurses in emergency departments during the COVID-19 outbreak. J. Affect. Disord. 276, 312–315. doi: 10.1016/j.jad.2020.06.047
Aymerich, C., Pedruzo, B., Pérez, J. L., Laborda, M., Herrero, J., Blanco, J., et al. (2022). COVID-19 pandemic effects on health worker’s mental health: systematic review and meta-analysis. Eur. Psychiatry 65:e10. doi: 10.1192/j.eurpsy.2022.1
Bareeqa, S. B., Ahmed, S. I., Samar, S. S., Yasin, W., Zehra, S., Monese, G. M., et al. (2021). Prevalence of depression, anxiety and stress in china during COVID-19 pandemic: a systematic review with meta-analysis. Int. J. Psychiatry Med. 56, 210–227. doi: 10.1177/0091217420978005
Beard, C., Millner, A. J., Forgeard, M. J., Fried, E. I., Hsu, K. J., Treadway, M. T., et al. (2016). Network analysis of depression and anxiety symptom relationships in a psychiatric sample. Psychol. Med. 46, 3359–3369. doi: 10.1017/S0033291716002300
Beschoner, P., Limbrecht-Ecklundt, K., and Jerg-Bretzke, L. (2019). Mental health among physicians: burnout, depression, anxiety and substance abuse in the occupational context. Der Nervenarzt 90, 961–974. doi: 10.1007/s00115-019-0739-x
Bianchi, R., Schonfeld, I. S., and Laurent, E. (2015). Burnout-depression overlap: a review. Clin. Psychol. Rev. 36, 28–41. doi: 10.1016/j.cpr.2015.01.004
Blanchard, M. A., Roskam, I., Mikolajczak, M., and Heeren, A. (2021). A network approach to parental burnout. Child Abuse Neglect 111:104826. doi: 10.1016/j.chiabu.2020.104826
Borsboom, D., and Cramer, A. O. (2013). Network analysis: an integrative approach to the structure of psychopathology. Annu. Rev. Clin. Psychol. 9, 91–121. doi: 10.1146/annurev-clinpsy-050212-185608
Bui, E., and Fava, M. (2017). From depression to anxiety, and back. Acta Psychiatr. Scand. 136, 341–342. doi: 10.1111/acps.12801
Cai, H., Chen, M. Y., Li, X. H., Zhang, L., Su, Z., Cheung, T., et al. (2024). A network model of depressive and anxiety symptoms: a statistical evaluation. Mol. Psychiatry 29, 767–781. doi: 10.1038/s41380-023-02369-5
Chen, S. Z., Zainal, N. H., and Newman, M. G. (2024). Elevated depression and anxiety predict future patterns of individualistic and collectivistic cultural values: a cross-lagged longitudinal network analysis. J. Affect. Disord. 349, 310–320. doi: 10.1016/j.jad.2023.12.083
Chen, Y., Nie, C., Bu, W., You, W., and Xu, P. (2023). Reliability and validity of the Chinese version of the burnout clinical subtypes questionnaire. Contempor. Nurse 30, 11–17.
Chirico, F., Batra, K., Batra, R., Ferrari, G., Crescenzo, P., Nucera, G., et al. (2023). Spiritual well-being and burnout syndrome in healthcare: a systematic review. J. Health Soc. Sci. 8:13.
Chirico, F., Crescenzo, P., Nowrouzi-Kia, B., Tarchi, L., Batra, K., Ferrari, G., et al. (2022). Prevalence and predictors of burnout syndrome among schoolteachers during the COVID-19 pandemic in Italy: a cross-sectional survey. J. Health Soc. Sci. 7, 195–211. doi: 10.19204/2022/PRVL6
Costantini, G., Epskamp, S., Borsboom, D., Perugini, M., Mõttus, R., Waldorp, L. J., et al. (2015). State of the aRt personality research: a tutorial on network analysis of personality data in R. J. Res. Pers. 54, 13–29.
Craske, M. G., Stein, M. B., Eley, T. C., Milad, M. R., Holmes, A., Rapee, R. M., et al. (2017). Anxiety disorders. Nat. Rev. Dis. Prim. 3:17024. doi: 10.1038/nrdp.2017.24
de Amorim Macedo, M. J., de Freitas, C. P. P., Bermudez, M. B., Souza Vazquez, A. C., Salum, G. A., and Dreher, C. B. (2023). The shared and dissociable aspects of burnout, depression, anxiety, and irritability in health professionals during COVID-19 pandemic: a latent and network analysis. J. Psychiatr. Res. 166, 40–48. doi: 10.1016/j.jpsychires.2023.09.005
Epskamp, S., Borsboom, D., and Fried, E. I. (2018). Estimating psychological networks and their accuracy: a tutorial paper. Behav. Res. Methods 50, 195–212. doi: 10.3758/s13428-017-0862-1
Ernst, J., Jordan, K. D., Weilenmann, S., Sazpinar, O., Gehrke, S., Paolercio, F., et al. (2021). Burnout, depression and anxiety among Swiss medical students–A network analysis. J. Psychiatr. Res. 143, 196–201. doi: 10.1016/j.jpsychires.2021.09.017
Farber, B. A. (1990). Burnout in psychotherapists: incidence, types, and trends. Psychother. Private Pract. 8, 35–44.
Fruchterman, T. M., and Reingold, E. M. (1991). Graph drawing by force-directed placement. Softw. Pract. Exp. 21, 1129–1164.
Ge, M. W., Hu, F. H., Jia, Y. J., Tang, W., Zhang, W. Q., and Chen, H. L. (2023). Global prevalence of nursing burnout syndrome and temporal trends for the last 10 years: a meta-analysis of 94 studies covering over 30 countries. J. Clin. Nurs. 32, 5836–5854. doi: 10.1111/jocn.16708
Golonka, K., Mojsa-Kaja, J., Blukacz, M., Gawłowska, M., and Marek, T. (2019). Occupational burnout and its overlapping effect with depression and anxiety. Int. J. Occup. Med. Environ. Health 32, 229–244. doi: 10.13075/ijomeh.1896.01323
He, Y., Wu, C., LeMoult, J., Huang, J., Zhao, Y., Liang, K., et al. (2023). Exploring symptom-level associations between anxiety and depression across developmental stages of adolescence: a network analysis approach. BMC Psychiatry 23:941. doi: 10.1186/s12888-023-05449-6
Hodkinson, A., Zhou, A., Johnson, J., Geraghty, K., Riley, R., Zhou, A., et al. (2022). Associations of physician burnout with career engagement and quality of patient care: systematic review and meta-analysis. BMJ 378:e070442. doi: 10.1136/bmj-2022-070442
Huang, C. P., Zou, J. M., Ma, H., and Zhong, Y. (2024). Role stress, occupational burnout and depression among emergency nurses: a cross-sectional study. Int. Emerg. Nurs. 72:101387. doi: 10.1016/j.ienj.2023.101387
Jihn, C. H., Kim, B., and Kim, K. S. (2021). Predictors of burnout in hospital health workers during the COVID-19 outbreak in South Korea. Int. J. Environ. Res. Public Health 18:11720. doi: 10.3390/ijerph182111720
Jones, B. (2019). Fifteen minutes may decrease nursing burnout: a discussion paper. Int. J. Nurs. Sci. 7, 121–123. doi: 10.1016/j.ijnss.2019.11.004
Jones, P. J., Ma, R., and McNally, R. J. (2021). Bridge centrality: a network approach to understanding comorbidity. Multivar. Behav. Res. 56, 353–367. doi: 10.1080/00273171.2019.1614898
Kessler, R. C., Berglund, P., Demler, O., Jin, R., Koretz, D., Merikangas, K. R., et al. (2003). The epidemiology of major depressive disorder: results from the National Comorbidity Survey Replication (NCS-R). JAMA 289, 3095–3105. doi: 10.1001/jama.289.23.3095
Koutsimani, P., Montgomery, A., and Georganta, K. (2019). The relationship between burnout, depression, and anxiety: a systematic review and meta-analysis. Front. Psychol. 10:284. doi: 10.3389/fpsyg.2019.00284
Li, Y., Guo, Z., Tian, W., Wang, X., Dou, W., Chen, Y., et al. (2023). An investigation of the relationships between suicidal ideation, psychache, and meaning in life using network analysis. BMC Psychiatry 23:257. doi: 10.1186/s12888-023-04700-4
Liu, F., Zhao, Y., Chen, Y., and Tu, Z. (2023). The mediation effect analysis of nurse’s mental health status and burnout under COVID-19 epidemic. Front. Public Health 11:1221501. doi: 10.3389/fpubh.2023.1221501
Liu, J., Xiang, Y. T., Wang, G., Zhu, X. Z., Ungvari, G. S., Kilbourne, A. M., et al. (2013). Psychometric properties of the Chinese versions of the Quick Inventory of Depressive Symptomatology - Clinician Rating (C-QIDS-C) and Self-Report (C-QIDS-SR). J. Affect. Disord. 147, 421–424. doi: 10.1016/j.jad.2012.08.035
Liu, X. C., Oda, S., Peng, X., and Asai, K. (1997). Life events and anxiety in Chinese medical students. Soc. Psychiatry Psychiatr. Epidemiol. 32, 63–67. doi: 10.1007/BF00788922
Luger, S., and Koziol, D. (2024). Authentic nursing leadership theory and nurse leaders’ stories: storytelling workshop impact on nurse leader burnout. Nurs. Manag. 55, 22–27. doi: 10.1097/nmg.0000000000000129
McNally, R. J. (2016). Can network analysis transform psychopathology? Behav. Res. Ther. 86, 95–104. doi: 10.1016/j.brat.2016.06.006
Mendelson, G. (1995). Diagnostic and Statistical Manual of Mental Disorders, (DSM-IV). Washington, DC: American Psychiatric Association.
Montero-Marín, J., and García-Campayo, J. (2010). A newer and broader definition of burnout: validation of the “Burnout Clinical Subtype Questionnaire (BCSQ-36)”. BMC Public Health 10:302. doi: 10.1186/1471-2458-10-302
Montero-Marín, J., García-Campayo, J., Mosquera Mera, D., and López del Hoyo, Y. (2009). A new definition of burnout syndrome based on Farber’s proposal. J. Occup. Med. Toxicol. (London, England) 4:31. doi: 10.1186/1745-6673-4-31
Moore, C., Kelly, S., and Melnyk, B. M. (2024). The use of mHealth apps to improve hospital nurses’ mental health and well-being: a systematic review. Worldviews Evid. Based Nurs. 21, 110–119. doi: 10.1111/wvn.12716
Niu, M., Wang, Y., Jia, Y., Wang, J., Zhong, S., Lin, J., et al. (2017). Common and specific abnormalities in cortical thickness in patients with major depressive and bipolar disorders. Ebiomedicine 16, 162–171. doi: 10.1016/j.ebiom.2017.01.010
Nucera, G., Chirico, F., Yildirim, M., Szarpak, L., and Magnavita, N. (2023). Addressing burnout and PTSD among paramedics and emergency staff after the COVID-19 pandemic: the role of occupational health services and workplace health promotion programs. Disaster Emerg. Med. J. 8, 131–133.
Okechukwu, C. E., Masala, D., D’Ettorre, G., and La Torre, G. (2022). Moderate-intensity aerobic exercise as an adjunct intervention to improve sleep quality among rotating shift nurses. Clin. Ter. 173, 184–186. doi: 10.7417/CT.2022.2414
Olivera-Pasilio, V., and Dabrowska, J. (2023). Fear-conditioning to unpredictable threats reveals sex and strain differences in rat Fear-Potentiated Startle (FPS). Neuroscience 530, 108–132. doi: 10.1016/j.neuroscience.2023.08.030
Park, S. C., and Kim, D. (2020). The centrality of depression and anxiety symptoms in major depressive disorder determined using a network analysis. J. Affect. Disord. 271, 19–26. doi: 10.1016/j.jad.2020.03.078
Parmar, V., Channar, Z. A., Ahmed, R. R., Streimikiene, D., Pahi, M. H., and Streimikis, J. (2022). Assessing the organizational commitment, subjective vitality and burnout effects on turnover intention in private universities. Oeconomia Copernicana 13, 251–286.
Peng, P., Chen, S., Hao, Y., He, L., Wang, Q., Zhou, Y., et al. (2023). Network of burnout, depression, anxiety, and dropout intention in medical undergraduates. Int. J. Soc. Psychiatry 69, 1520–1531. doi: 10.1177/00207640231166629
Rehman, U., Yıldırım, M., and Shahnawaz, M. G. (2023). A longitudinal study of depression, anxiety, and stress among Indians during COVID-19 pandemic. Psychol. Health Med. 28, 60–68. doi: 10.1080/13548506.2021.2023751
Rizzo, A., Yıldırım, M., Öztekin, G. G., Carlo, A. D., Nucera, G., Szarpak, Ł, et al. (2023). Nurse burnout before and during the COVID-19 pandemic: a systematic comparative review. Front. Public Health 11:1225431. doi: 10.3389/fpubh.2023.1225431
Rouquette, A., Pingault, J. B., Fried, E. I, Orri, M., Falissard, B., Kossakowski, J. J., et al. (2018). Emotional and behavioral symptom network structure in elementary school girls and association with anxiety disorders and depression in adolescence and early adulthood: a network analysis. JAMA Psychiatry 75, 1173–1181. doi: 10.1001/jamapsychiatry.2018.2119
Rush, A. J., Trivedi, M. H., Ibrahim, H. M., Carmody, T. J., Arnow, B., Klein, D. N., et al. (2003). The 16-item Quick Inventory of Depressive Symptomatology (QIDS), clinician rating (QIDS-C), and self-report (QIDS-SR): a psychometric evaluation in patients with chronic major depression. Biol. Psychiatry 54, 573–583. doi: 10.1016/s0006-3223(02)01866-8
Ryan, E., Hore, K., Power, J., and Jackson, T. (2023). The relationship between physician burnout and depression, anxiety, suicidality and substance abuse: a mixed methods systematic review. Front. Public Health 11:1133484. doi: 10.3389/fpubh.2023.1133484
Saragih, I. D., Tonapa, S. I., Saragih, I. S., Advani, S., Batubara, S. O., Suarilah, I., et al. (2021). Global prevalence of mental health problems among healthcare workers during the COVID-19 pandemic: a systematic review and meta-analysis. Int. J. Nurs. Stud. 121:104002. doi: 10.1016/j.ijnurstu.2021.104002
Sohrabi, Y., Yarmohammadi, H., Pouya, A. B., Arefi, M. F., Hassanipour, S., and Poursadeqiyan, M. (2022). Prevalence of job burnout in Iranian nurses: a systematic review and meta-analysis. Work 73, 937–943. doi: 10.3233/WOR-210283
Stefani, A., and Högl, B. (2021). Nightmare disorder and isolated sleep paralysis. Neurotherapeutics 18, 100–106. doi: 10.1007/s13311-020-00966-8
Sun, H., Zhang, T., Wang, X., Wang, C., Zhang, M., and Song, H. (2023). The occupational burnout among medical staff with high workloads after the COVID-19 and its association with anxiety and depression. Front. Public Health 11:1270634. doi: 10.3389/fpubh.2023.1270634
Sun, W., Fu, J., Chang, Y., and Wang, L. (2012). Epidemiological study on risk factors for anxiety disorder among Chinese doctors. J. Occupat. Health 54, 1–8. doi: 10.1539/joh.11-0169-oa
Suradom, C., Wongpakaran, N., Wongpakaran, T., Lerttrakarnnon, P., Jiraniramai, S., Taemeeyapradit, U., et al. (2020). Mediation model of comorbid anxiety disorders in late-life depression. Ann. Gen. Psychiatry 19:63. doi: 10.1186/s12991-020-00313-3
Tavella, G., Hadzi-Pavlovic, D., and Parker, G. (2021). Burnout: redefining its key symptoms. Psychiatry Res. 302:114023. doi: 10.1016/j.psychres.2021.114023
Verkuilen, J., Bianchi, R., Schonfeld, I. S., and Laurent, E. (2021). Burnout-depression overlap: exploratory structural equation modeling bifactor analysis and network analysis. Assessment 28, 1583–1600. doi: 10.1177/1073191120911095
Warner, V., Wickramaratne, P., and Weissman, M. M. (2008). The role of fear and anxiety in the familial risk for major depression: a three-generation study. Psychol. Med. 38, 1543–1556. doi: 10.1017/S0033291708002894
Wei, H., Liu, M., Wang, Z., Qu, W., Zhang, S., Zhang, B., et al. (2024). Anxiety, depression, and post-traumatic stress disorder in nurses exposed to horizontal violence: a network analysis. BMC Nurs. 23:750. doi: 10.1186/s12912-024-02408-8
Wong, K. W., Wu, X., and Dong, Y. (2024). Interventions to reduce burnout and improve the mental health of nurses during the COVID-19 pandemic: a systematic review of randomised controlled trials with meta-analysis. Int. J. Ment. Health Nurs. 33, 324–343. doi: 10.1111/inm.13251
Xiong, N., Fritzsche, K., Pan, Y., Löhlein, J., and Leonhart, R. (2022). The psychological impact of COVID-19 on Chinese healthcare workers: a systematic review and meta-analysis. Soc. Psychiatry Psychiatr. Epidemiol. 57, 1515–1529. doi: 10.1007/s00127-022-02264-4
Yennurajalingam, S., Tayjasanant, S., Balachandran, D., Padhye, N. S., Williams, J. L., Liu, D. D., et al. (2016). Association between daytime activity, fatigue, sleep, anxiety, depression, and symptom burden in advanced cancer patients: a preliminary report. J. Palliat. Med. 19, 849–856. doi: 10.1089/jpm.2015.0276
Yıldırım, M., and Ashraf, F. (2024). Fear of COVID-19, coronavirus anxiety, COVID-19 burnout, and resilience: examining psychometric properties of COVID-19 burnout scale in Urdu. J. Asian Afric. Stud. 59, 2303–2315.
Yohannes, A. M., Murri, M. B., Hanania, N. A., Regan, E. A., Iyer, A., Bhatt, S. P., et al. (2022). Depressive and anxiety symptoms in patients with COPD: a network analysis. Respir. Med. 198:106865.
Zainal, N. H., and Newman, M. G. (2024). A cross-lagged prospective network analysis of depression and anxiety and cognitive functioning components in midlife community adult women - CORRIGENDUM. Psychol. Med. 54:434. doi: 10.1017/S0033291723003574
Zhan, Z. J., Huang, H. Y., Xiao, Y. H., Zhao, Y. P., Cao, X., Cai, Z. C., et al. (2024). Anxiety and depression in nasopharyngeal carcinoma patients and network analysis to identify central symptoms: a cross-sectional study from a high-incidence area. Radiother. Oncol. 197:110324. doi: 10.1016/j.radonc.2024.110324
Zhang, F., Lin, C., Li, X., Li, M., Jia, R., Guo, X., et al. (2023). The relationships between burnout, general wellbeing, and psychological detachment with turnover intention in Chinese nurses: a cross-sectional study. Front. Public Health 11:1216810. doi: 10.3389/fpubh.2023.1216810
Zhang, J., Wang, Y., Xu, J., You, H., Li, Y., Liang, Y., et al. (2021). Prevalence of mental health problems and associated factors among front-line public health workers during the COVID-19 pandemic in China: an effort-reward imbalance model-informed study. BMC Psychol. 9:55. doi: 10.1186/s40359-021-00563-0
Zhen, L., Wang, G., Xu, G., Xiao, L., Feng, L., Chen, X., et al. (2020). Evaluation of the paper and smartphone versions of the Quick Inventory of Depressive Symptomatology-Self-Report (QIDS-SR16) and the Patient Health Questionnaire-9 (PHQ-9) in depressed patients in China. Neuropsychiatr. Dis. Treatm. 16, 993–1001. doi: 10.2147/NDT.S241766
Zung, W. W. (1971). A rating instrument for anxiety disorders. Psychosomatics 12, 371–379. doi: 10.1016/S0033-3182(71)71479-0
Keywords: job burnout, anxiety, depression, medical worker, network analysis
Citation: Yang C, Chen Y, Wang X, Xu P, Song J, Yang L and Fu Y (2024) A network approach to understanding occupational psychological distress: linking depression, anxiety, and burnout among Chinese healthcare professionals. Front. Psychol. 15:1474523. doi: 10.3389/fpsyg.2024.1474523
Received: 01 August 2024; Accepted: 06 December 2024;
Published: 18 December 2024.
Edited by:
Murat Yildirim, Ağrı Ýbrahim Çeçen University, TürkiyeReviewed by:
Luis Felipe Dias Lopes, Federal University of Santa Maria, BrazilCopyright © 2024 Yang, Chen, Wang, Xu, Song, Yang and Fu. This is an open-access article distributed under the terms of the Creative Commons Attribution License (CC BY). The use, distribution or reproduction in other forums is permitted, provided the original author(s) and the copyright owner(s) are credited and that the original publication in this journal is cited, in accordance with accepted academic practice. No use, distribution or reproduction is permitted which does not comply with these terms.
*Correspondence: Yao Chen, MTA3MDE0MzgyNUBxcS5jb20=
†ORCID: Cui Yang, orcid.org/0000-0002-6489-2090
Disclaimer: All claims expressed in this article are solely those of the authors and do not necessarily represent those of their affiliated organizations, or those of the publisher, the editors and the reviewers. Any product that may be evaluated in this article or claim that may be made by its manufacturer is not guaranteed or endorsed by the publisher.
Research integrity at Frontiers
Learn more about the work of our research integrity team to safeguard the quality of each article we publish.