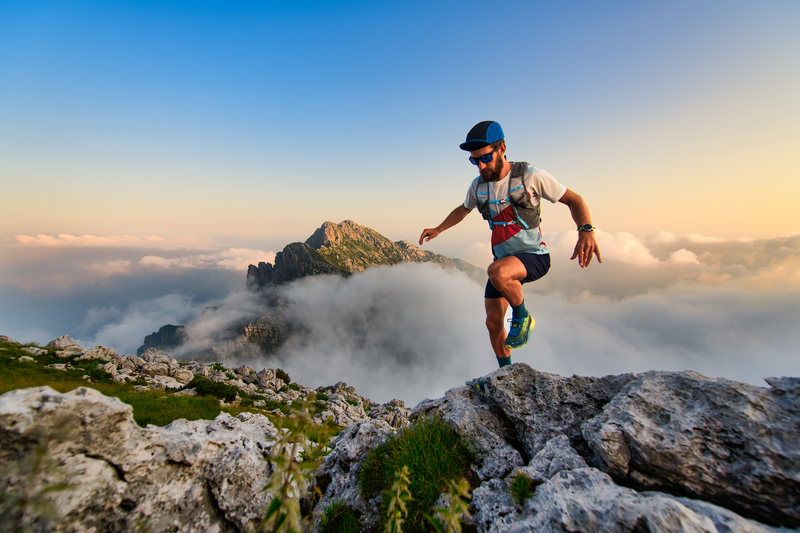
94% of researchers rate our articles as excellent or good
Learn more about the work of our research integrity team to safeguard the quality of each article we publish.
Find out more
EDITORIAL article
Front. Psychol. , 10 October 2024
Sec. Media Psychology
Volume 15 - 2024 | https://doi.org/10.3389/fpsyg.2024.1461881
This article is part of the Research Topic AI as Intelligent Technology and Agent to Understand and Be Understood by Human Minds View all 7 articles
Editorial on the Research Topic
AI as intelligent technology and agent to understand and be understood by human minds
The rapid evolution of artificial intelligence (AI) is profoundly reshaping our lives and work, demonstrating significant potential in areas such as industrial automation, business operations, academic research, and daily applications (Arin, 2023). However, the widespread use of AI has also raised concerns over privacy, algorithmic bias and transparency, and security risks, highlighting the need for ethical, safe, and socially responsible AI systems (Díaz-Rodríguez et al., 2023). Addressing these issues depends on how we further develop AI as a tool to better learn, fit, and align with human psychology and behavior, as well as how humans adapt to and better interact with AI as a social counterpart.
The six empirical and theorizing research papers collected under this Research Topic cover a range of areas or themes, including the acceptance of AI applications in personal health management, consumer behavior, and organizational behavior, the AI-ization of academic research, interpretable AI algorithms, and the comprehensive framework for trustworthy AI. These studies not only align with the objectives set by the Research Topic but also address the issues raised to varying degrees. They are expected to inspire future research to continue deepening and expanding in related fields.
Within the realm of this Research Topic, encompassing both empirical and theoretical inquiries, whether it delves into the interpretability of AI algorithms or explores the acceptance and implementation of AI across various fields, the core issue consistently centers on how to cultivate trustworthy AI.
Zou et al. applied Innovation Resistance Theory (IRT) and the Prototype Willingness Model (PWM) to analyze public resistance to health chatbots, identifying functional and psychological barriers, along with negative prototype perceptions, as key resistance drivers. Their work advances understanding of resistance to emerging healthcare AI technologies.
Yue and Li explored how different human-AI collaboration modes and outcome expectations affect consumer evaluations and intentions through online experiments. They underlined the significance of responsibility attribution and algorithm transparency, noting a preference for AI-dominant products with positive expectations and AI-assisted ones with negative expectations. This research extends consumer behavior studies by including the human-AI interaction perspective, offering insights for better AI integration into products and services.
Fousiani et al.'s empirical study under our Research Topic on the interactive effects of competitive organizational climate and leadership power perception on employee AI acceptance revealed that a competitive environment fosters AI acceptance when leaders view power as a responsibility but hinders it when seen as an opportunity, enriching our understanding of organizational factors in AI acceptance and suggesting ways to enhance AI adoption in organizations.
Three additional theorizing research on trustworthy AI within our Research Topic deliver profound insights too. Liu and Xu outlined two interpretable neural network (INN) methodologies: Model Decomposition and Semantic INNs. The former decomposes traditional mathematical or physical models into learnable modules, making the computational process interpretable by translating it into network parameters. The latter provides semantic explanations for black-box networks through visualization, decision tree regularization, and semantic knowledge graphs. The two approaches, based on pre- or post-network-design respectively, aim to enhance the transparency and interpretability of deep neural networks, thus promoting humans's trust in them.
Yuan et al. focused on the AI-ization of academic research, discussing generative AI's role, like ChatGPT, in environmental psychology. They highlighted its potential to improve research by aiding in question formulation, theory modeling, and tool development, as well as streamlining data analysis and customizing communication. However, they stressed the importance of ethical considerations and the need for professional training and evaluation to ensure research quality and integrity.
Last but not least, as a summary of our Research Topic and its related research, Li et al. encapsulate the essence of building trustworthy AI systems by examining critical factors from a tripartite framework of trustor, trustee, and their interactive context. They highlight the importance of demographic characteristics, familiarity with AI, self-efficacy, and emotional experiences for human trustors, alongside the technical attributes of AI systems such as reliability and ethical alignment. Additionally, the influence of cultural and organizational contexts on trust dynamics is emphasized. This synthesis underscores the need for a holistic approach that integrates technical excellence with human-centric ethics to enhance AI acceptance.
The excellent papers above delve into developing trustworthy AI systems from various angles, including user acceptance, organizational environment, algorithm interpretability, the AI-ization of academic research and so on, offering rich perspectives and insights. Key factors affecting AI acceptance identified include functionality, psychological aspects, accountability, and transparency of algorithms. For example, Zou et al. highlights how overcoming functional and psychological barriers to health chatbots and improving their prototype perceptions can boost public acceptance. Additionally, organizational factors like leadership style significantly influence AI adoption; Fousiani et al. found that employees are more receptive to AI when leaders view power as a responsibility rather than an opportunity, underscoring the need for adaptive organizational cultures and management styles. Moreover, advancements in interpretable AI algorithms summarized by Liu and Xu, such as model decomposition and semantic neural networks, are crucial for making AI's decision-making processes more understandable and transparent, thus fostering trust among users.
Despite progress in the field, critical challenges remain. Firstly, deepening our understanding of human psychological expectations and trust mechanisms toward AI is essential. Current research often focuses on single dimensions, lacking a holistic view of the human-AI interaction process. Future studies should adopt an integrated theoretical framework to explore the interplay between trustors, trustees, and interaction contexts. Secondly, the question of designing more humane and ethically aligned AI systems is significant. Existing technological solutions struggle to fully address biases and safety concerns in AI. Researchers should refine interpretable AI algorithms and enhance assessments of AI systems' ethics and social impacts to align with human values. Lastly, improving organizational and societal-level AI governance is challenging, with most research focusing on the individual level and lacking systematic policy and institutional recommendations. Efforts should aim at establishing relevant regulations and standards, promoting stakeholder participation and collaboration, to foster healthy AI development.
In summary, building truly trustworthy AI requires collaborative efforts across technical, ethical, and governance dimensions from scholars, businesses, and governments. Only through such comprehensive efforts can AI become an intelligent technology and agent that is both understanding and understandable by humanity.
HC: Writing – original draft. XR: Writing – review & editing. LH: Writing – original draft. JH: Writing – review & editing.
The author(s) declare financial support was received for the research, authorship, and/or publication of this article. This study was funded by Nankai University Liberal Arts Development Fund (Grant No. ZB22BZ0218) and the Fundamental Research Funds for the Central Universities (Grant No. 63233147).
The authors declare that the research was conducted in the absence of any commercial or financial relationships that could be construed as a potential conflict of interest.
The author(s) declared that they were an editorial board member of Frontiers, at the time of submission. This had no impact on the peer review process and the final decision.
All claims expressed in this article are solely those of the authors and do not necessarily represent those of their affiliated organizations, or those of the publisher, the editors and the reviewers. Any product that may be evaluated in this article, or claim that may be made by its manufacturer, is not guaranteed or endorsed by the publisher.
Arin, H. (2023). AI and the Human Experience: Embracing the Age of Intelligent Machines. San Diego: Holly Arin.
Díaz-Rodríguez, N., Del Ser, J., Coeckelbergh, M., de Prado, M. L., Herrera-Viedma, E., and Herrera, F. (2023). Connecting the dots in trustworthy Artificial Intelligence: from AI principles, ethics, and key requirements to responsible AI systems and regulation. Inform. Fus. 99:101896. doi: 10.1016/j.inffus.2023.101896
Keywords: health chatbots, human-AI collaboration, AI acceptance in organizations, environmental psychology, AI-ization of academic research, interpretable neural networks, ChatGPT, trustworthy AI
Citation: Chen H, Ren X, He L and Huang J (2024) Editorial: AI as intelligent technology and agent to understand and be understood by human minds. Front. Psychol. 15:1461881. doi: 10.3389/fpsyg.2024.1461881
Received: 09 July 2024; Accepted: 05 August 2024;
Published: 10 October 2024.
Edited and reviewed by: Erin A. Vogel, University of Oklahoma Health Sciences Center, United States
Copyright © 2024 Chen, Ren, He and Huang. This is an open-access article distributed under the terms of the Creative Commons Attribution License (CC BY). The use, distribution or reproduction in other forums is permitted, provided the original author(s) and the copyright owner(s) are credited and that the original publication in this journal is cited, in accordance with accepted academic practice. No use, distribution or reproduction is permitted which does not comply with these terms.
*Correspondence: Lingnan He, aGVsbjNAbWFpbC5zeXN1LmVkdS5jbg==
Disclaimer: All claims expressed in this article are solely those of the authors and do not necessarily represent those of their affiliated organizations, or those of the publisher, the editors and the reviewers. Any product that may be evaluated in this article or claim that may be made by its manufacturer is not guaranteed or endorsed by the publisher.
Research integrity at Frontiers
Learn more about the work of our research integrity team to safeguard the quality of each article we publish.