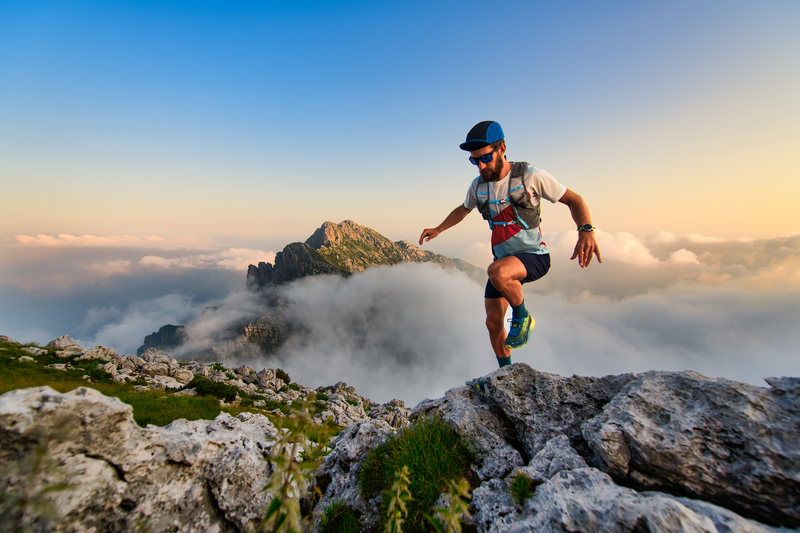
95% of researchers rate our articles as excellent or good
Learn more about the work of our research integrity team to safeguard the quality of each article we publish.
Find out more
ORIGINAL RESEARCH article
Front. Psychol.
Sec. Emotion Science
Volume 15 - 2024 | doi: 10.3389/fpsyg.2024.1459446
The final, formatted version of the article will be published soon.
You have multiple emails registered with Frontiers:
Please enter your email address:
If you already have an account, please login
You don't have a Frontiers account ? You can register here
Emotion recognition plays a crucial role in understanding decision-making processes, as emotional stimuli significantly influence individuals' choices. However, existing emotion recognition systems face challenges in handling complex natural environments, diverse emotional expressions, and limited data availability, hampering their effectiveness and widespread adoption. To address these issues, we propose an Enhanced GhostNet with Transformer Encoder (EGT) model that leverages deep learning techniques for robust emotion recognition through facial expressions. The EGT model integrates GhostNet's efficient feature extraction, the Transformer's ability to capture global context, and a dual attention mechanism to selectively enhance critical features. Experimental results show that the EGT model achieves an accuracy of 89.3% on the RAF-DB dataset and 85.7% on the AffectNet dataset, outperforming current state-of-the-art lightweight models. These results indicate the model's capability to recognize various emotional states with high confidence, even in challenging and noisy environments. Our model's improved accuracy and robustness in emotion recognition can enhance intelligent human-computer interaction systems, personalized recommendation systems, and mental health monitoring tools. This research underscores the potential of advanced deep learning techniques to significantly improve emotion recognition systems, providing better user experiences and more informed decision-making processes.
Keywords: emotion recognition, decision-making, Ghostnet, transformer encoder, Dual attention mechanism, human-computer interaction
Received: 03 Sep 2024; Accepted: 04 Nov 2024.
Copyright: © 2024 Sun, Xu and Yao. This is an open-access article distributed under the terms of the Creative Commons Attribution License (CC BY). The use, distribution or reproduction in other forums is permitted, provided the original author(s) or licensor are credited and that the original publication in this journal is cited, in accordance with accepted academic practice. No use, distribution or reproduction is permitted which does not comply with these terms.
* Correspondence:
Tianwen Xu, Quanzhou Normal University, Quanzhou, 362000, Fujian Province, China
Disclaimer: All claims expressed in this article are solely those of the authors and do not necessarily represent those of their affiliated organizations, or those of the publisher, the editors and the reviewers. Any product that may be evaluated in this article or claim that may be made by its manufacturer is not guaranteed or endorsed by the publisher.
Research integrity at Frontiers
Learn more about the work of our research integrity team to safeguard the quality of each article we publish.