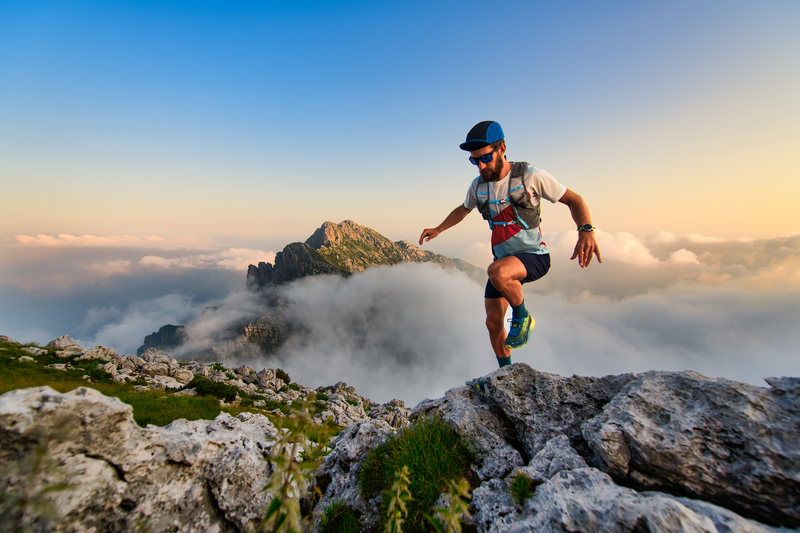
95% of researchers rate our articles as excellent or good
Learn more about the work of our research integrity team to safeguard the quality of each article we publish.
Find out more
PERSPECTIVE article
Front. Psychol. , 30 August 2024
Sec. Quantitative Psychology and Measurement
Volume 15 - 2024 | https://doi.org/10.3389/fpsyg.2024.1404060
This article is part of the Research Topic Critical Debates on Quantitative Psychology and Measurement: Revived and Novel Perspectives on Fundamental Problems View all 13 articles
Over the past few years, more attention has been paid to jingle and jangle fallacies in psychological science. Jingle fallacies arise when two or more distinct psychological phenomena are erroneously labeled with the same term, while jangle fallacies occur when different terms are used to describe the same phenomenon. Jingle and jangle fallacies emerge due to the vague linkage between psychological theories and their practical implementation in empirical studies, compounded by variations in study designs, methodologies, and applying different statistical procedures’ algorithms. Despite progress in organizing scientific findings via systematic reviews and meta-analyses, effective strategies to prevent these fallacies are still lacking. This paper explores the integration of several approaches with the potential to identify and mitigate jingle and jangle fallacies within psychological science. Essentially, organizing studies according to their specifications, which include theoretical background, methods, study designs, and results, alongside a combinatorial algorithm and flexible inclusion criteria, may indeed represent a feasible approach. A jingle-fallacy detector arises when identical specifications lead to disparate outcomes, whereas jangle-fallacy indicators could operate on the premise that varying specifications consistently yield overrandomly similar results. We discuss the role of advanced computational technologies, such as Natural Language Processing (NLP), in identifying these fallacies. In conclusion, addressing jingle and jangle fallacies requires a comprehensive approach that considers all levels and phases of psychological science.
In recent years, there has been increased attention on jingle and jangle fallacies in psychological science (Altgassen et al., 2024; Ayache et al., 2024; Beisly, 2023; Fischer et al., 2023; Hook et al., 2015; Marsh et al., 2019; Porter, 2023). Jingle fallacies occur when two or more distinct psychological phenomena are labeled with the same name, as Thorndike (1904, p. 14) defined over 120 years ago. Kelley (1927, p. 64) later defined jangle fallacies as labeling the same phenomenon with different terms, exemplified by his use of ‘intelligence’ and ‘achievement’. Gonzalez et al. (2021) highlighted that jingle and jangle fallacies pose a significant threat to the validity of the research. These fallacies are not always explicitly labeled as such; they may also be characterized as a déjà-variable phenomenon (Hagger, 2014; Hanfstingl, 2019; Skinner, 1996).
Why do jingle and jangle fallacies emerge? In essence, Thorndike (1904) and Kelley (1927) attributed their occurrence to a vague connection between psychological theory and its operationalization in empirical studies. Recent studies have emphasized the caution needed regarding jingle-jangle fallacies due to differences in algorithms used in statistical procedures (Grieder and Steiner, 2022). Another reason that exacerbates this problem is the substantial increase in scientific research since the Second World War, which has led to an increase in the overall number of studies carried out. However, as scientific knowledge continues to expand, there is an increasing need for its systematic organization and categorization. Without adequate systematization, the risk of poorly aligned parallel fields and trends operating independently increases, resulting in a disjointed theoretical landscape lacking overarching theories or paradigms. Finally, efficient progress is hindered by undetected inconsistencies in empirical evidence. Despite the long-standing knowledge of jingle and jangle fallacies, effective strategies to prevent psychological science from encountering these issues have not yet been developed.
In the 1970s, several solutions emerged to address the lack of systematization in scientific findings, with the development of review and meta-analytical approaches, albeit without explicit reference to jingle or jangle fallacies. According to Shadish and Lecy (2015), meta-analysis is considered “one of the most significant methodological advancements in science over the past century” (p. 246). Notably, Gene V. Glass focused on psychotherapy effects, Frank L. Schmidt emphasized psychological test validity, and Robert Rosenthal aimed to synthesize findings on interpersonal expectancy effects, all of whom contributed significantly to the development of meta-analysis (Shadish and Lecy, 2015).
While the practice of summarizing single studies in reviews and meta-analytical procedures has become common and well-accepted, several problems have become apparent: Systematic reviews and meta-analyses, while valuable, are not immune to bias and fail to detect jingle or jangle fallacies. Despite several initiatives like the PRISMA statement (Page et al., 2021) or meta-analysis reporting standards (MARS; Lakens et al., 2017), they still lack quality criteria (Glass, 2015; Pigott and Polanin, 2020) or ignore the influence of methodologies on the result (Elson, 2019). Some biases are extremely difficult to control, as, for example, those caused by scientists themselves (Hanfstingl, 2019; Wicherts et al., 2016) or by operationalization variances (Simonsohn et al., 2020; Steegen et al., 2016; Voracek et al., 2019). Furthermore, as with single studies, without transparency and free access to each point of the research process, reproducibility is not given (Maassen et al., 2020; Polanin et al., 2020). In sum, current review and meta-analytical approaches fail to uncover jingle or jangle fallacies.
Essentially, we need not only programs to systematize empirical evidence and knowledge but also strategies to detect and prevent jingle and jangle fallacies, ideally combining single-study analyses at the meta-level. To address these challenges, we explore several potentially beneficial approaches. One such approach involves the systematization not only of results but also of theoretical backgrounds, methodological approaches, study designs, and outcomes. This provides, for example, specification curve analysis developed by Simonsohn et al. (2020). The procedure delineates all reasonable and debatable choices and specifications for addressing a research inquiry at the single-study level. These specifications must (1) logically examine the research question, (2) be expected to maintain statistical validity, and (3) avoid redundancy with other specifications in the array. Steegen et al. (2016) introduced the multiverse analysis concept, offering additional plotting alternatives as a similar approach. Voracek et al. (2019) combined these approaches at a meta-analytical level, revealing the range of formally valid specifications, including theoretical frameworks, methodological approaches, and researchers’ degrees of freedom. They distinguish between internal (“which,” e.g., the selection of data for meta-analysis) and external (“how,” e.g., the methodology of data meta-analysis) factors. Identifying reasonable and formally valid specifications is considered a crucial first step in gaining an overview of which aspects and perspectives of a psychological phenomenon have already been empirically investigated.
Detecting and preventing jingle and jangle fallacies requires considering as many studies as possible to obtain a comprehensive overview. However, addressing the relatively strict and sometimes poorly justified inclusion and exclusion criteria in systematic reviews and meta-analyses presents a further challenge (Uttley et al., 2023). The current practice of setting rigid criteria in meta-analyses may be overly stringent, leading to the exclusion of valuable but non-quantifiable studies. Several approaches have less strict inclusion criteria, such as the harvest plot (Ogilvie et al., 2008). The harvest plot considers studies by graphical displays that otherwise would be excluded due to missing quantifiable data or effect estimates for meta-analyses, plotting the quality, the study design variances, differences of included variables, and outcome information of the studies. Foulds et al. (2022) described harvest plots as an exploratory method that allows for grouping outcomes, including non-parametric statistical tests, studies without effect sizes, and depiction of biases within studies. Comparing the results of a meta-analysis and a harvest plot analysis derived from the same study corpus reveals that the harvest plot approach allows for the inclusion of a significantly higher number of studies in the analysis (Foulds et al., 2022, Table 3). Accordingly, techniques like harvest plots play a vital role in expanding the scope of analyzed findings, which is crucial for achieving a comprehensive understanding of studies on a specific phenomenon.
As described, various useful approaches effectively structure and organize studies on a psychological phenomenon. But how can we detect potential jingle and jangle fallacies? Harvest plots summarize the findings of studies based on their suitability of study design, quality of execution, variance-explaining dimensions (such as gender and race), and outcomes quality (e.g., behavioral, self-reports). The plots offer descriptive representations and provide an overview of previously investigated results. Empty lines indicate missing data for known combinations of variables or specifications (see, e.g., Ogilvie et al., 2008, Figure 1). However, they still lack a combinatorial approach, as suggested by Simonsohn et al., 2020 or Voracek et al. (2019). After implementing the permutational aspect on specifications, a jingle fallacy detector could be based on the idea that, in the presence of a jingle, the same specifications would lead to different results. Conversely, jangle fallacy detectors would operate vice-versa and indicate jangle if different specifications yield overrandomly similar results. Figure 1 illustrates how combining different theoretical approaches, methodologies, data availabilities, and outcomes can help identify potential jingle and jangle fallacies.
Figure 1. Exemplary specifications derived from theory, methodology, data availability, and results.
Thus far, two promising approaches have concentrated on detecting jingle and jangle fallacies at a taxonomic level. Larsen and Bong (2016) presented six different so-called construct identity detectors for literature reviews and meta-analyses, applying different natural language processing algorithms. Wulff and Mata (2023) provided a solution in a preprint, utilizing GPT at the level of personality taxonomies to analyze the items and their scale assignments in the international personality item pool (IPIP; Goldberg et al., 2006). Since GPT is based on Natural Language Processing, it is well-suited to detect jingle and jangle fallacies within taxonomic approaches. However, reliance on taxonomies alone is insufficient for detecting jingle and jangle fallacies in psychological science. We understand psychological phenomena through theories, operationalized with concepts, constructs, and methodologies, and measured through physiological and behavioral data, self-reports, and external reports. Empirical data hinges on these interconnected elements alongside methodologies and study designs (Uher, 2023). Therefore, to detect jingle and jangle fallacies, we must consider all these levels and phases of psychological science.
The growing attention to jingle and jangle fallacies in recent years underscores their significance in psychological science, posing a threat to validity and often going unrecognized. These fallacies, originally defined by Thorndike (1904) and Kelley (1927), emerge due to vague connections between theoretical concepts and empirical operationalizations but also have pure computational roots (Grieder and Steiner, 2022). Developments like meta-analyses and systematization through reviews help to systematize knowledge, but these practices are not immune to biases and limitations (Uttley et al., 2023) and do not detect jingle and jangle fallacies – such detectors are not yet developed. These detectors need to consider all levels and phases of psychological science, from theoretical frameworks to methodological approaches and study designs, called study specifications (Simonsohn et al., 2020). Additionally, flexible inclusion criteria for considered studies and new computational approaches, as conducted by Larsen and Bong (2016) or Wulff and Mata (2023) are needed. Ultimately, addressing jingle and jangle fallacies requires a concerted effort across the scientific community, incorporating diverse theories, perspectives, and methodologies. Simply defining the problem – finding one term for multiple phenomena (jingle) or different terms for the same phenomenon (jangle) – is insufficient. A systematic revision of jingle and jangle fallacies, achieved through discussion and analysis of detected instances is essential, as outlined in Figure 2.
The original contributions presented in the study are included in the article, further inquiries can be directed to the corresponding author.
BH: Conceptualization, Methodology, Writing – original draft, Writing – review & editing. SO: Writing – review & editing. JP: Writing – review & editing. UT: Writing – review & editing, Conceptualization. MV: Conceptualization, Writing – review & editing.
The author(s) declare that no financial support was received for the research, authorship, and/or publication of this article.
The authors declare that the research was conducted in the absence of any commercial or financial relationships that could be construed as a potential conflict of interest.
The author(s) declared that they were an editorial board member of Frontiers, at the time of submission. This had no impact on the peer review process and the final decision.
All claims expressed in this article are solely those of the authors and do not necessarily represent those of their affiliated organizations, or those of the publisher, the editors and the reviewers. Any product that may be evaluated in this article, or claim that may be made by its manufacturer, is not guaranteed or endorsed by the publisher.
Altgassen, E., Geiger, M., and Wilhelm, O. (2024). Do you mind a closer look? A jingle-jangle fallacy perspective on mindfulness. Eur. J. Personal. 38, 365–387. doi: 10.1177/08902070231174575
Ayache, J., Dumas, G., Sumich, A., Kuss, D. J., Rhodes, D., and Heym, N. (2024). The «jingle-jangle fallacy» of empathy: delineating affective, cognitive and motor components of empathy from behavioral synchrony using a virtual agent. Personal. Individ. Differ. 219:112478. doi: 10.1016/j.paid.2023.112478
Beisly, A. H. (2023). The jingle-jangle of approaches to learning in prekindergarten: A construct with too many names. Educ. Psychol. Rev. 35:79. doi: 10.1007/s10648-023-09796-4
Elson, M. (2019). Examining psychological science through systematic meta-method analysis: a call for research. Adv. Methods Pract. Psychol. Sci. 2, 350–363. doi: 10.1177/2515245919863296
Fischer, A., Voracek, M., and Tran, U. S. (2023). Semantic and sentiment similarities contribute to construct overlaps between mindfulness, big five, emotion regulation, and mental health. Personal. Individ. Differ. 210:112241. doi: 10.1016/j.paid.2023.112241
Foulds, J., Knight, J., Young, J. T., Keen, C., and Newton-Howes, G. (2022). A novel graphical method for data presentation in alcohol systematic reviews: the interactive harvest plot. Alcohol Alcohol. 57, 16–25. doi: 10.1093/alcalc/agaa145
Glass, G. V. (2015). Meta-analysis at middle age: a personal history. Res. Synth. Methods 6, 221–231. doi: 10.1002/jrsm.1133
Goldberg, L. R., Johnson, J. A., Eber, H. W., Hogan, R., Ashton, M. C., Cloninger, C. R., et al. (2006). The international personality item pool and the future of public-domain personality measures. J. Res. Pers. 40, 84–96. doi: 10.1016/j.jrp.2005.08.007
Gonzalez, O., MacKinnon, D. P., and Muniz, F. B. (2021). Extrinsic convergent validity evidence to prevent jingle and jangle fallacies. Multivar. Behav. Res. 56, 3–19. doi: 10.1080/00273171.2019.1707061
Grieder, S., and Steiner, M. D. (2022). Algorithmic jingle jungle: a comparison of implementations of principal axis factoring and promax rotation in R and SPSS. Behav. Res. Methods 54, 54–74. doi: 10.3758/s13428-021-01581-x
Hagger, M. S. (2014). Avoiding the “déjà-variable” phenomenon: social psychology needs more guides to constructs. Front. Psychol. 5:52. doi: 10.3389/fpsyg.2014.00052
Hanfstingl, B. (2019). Should we say goodbye to latent constructs to overcome replication crisis or should we take into account epistemological considerations? Front. Psychol. 10:1949. doi: 10.3389/fpsyg.2019.01949
Hook, J. N., Davis, D. E., van Tongeren, D. R., Hill, P. C., Worthington, E. L., Farrell, J. E., et al. (2015). Intellectual humility and forgiveness of religious leaders. J. Posit. Psychol. 10, 499–506. doi: 10.1080/17439760.2015.1004554
Kelley, T. L. (1927). Interpretation of educational measurements. New York and Chicago: World Book Company.
Lakens, D., Page-Gould, E., van Assen, M. A. L. M., Spellman, B., Schönbrodt, F. D., Hasselman, F., et al. (2017). Examining the reproducibility of meta-analyses in psychology: A preliminary report. Available at: https://doi.org/10.31222/osf.io/xfbjf
Larsen, K. R., and Bong, C. H. (2016). A tool for addressing construct identity in literature reviews and meta-analyses. MIS Q. 40, 529–551. doi: 10.25300/MISQ/2016/40.3.01
Maassen, E., van Assen, M. A. L. M., Nuijten, M. B., Olsson-Collentine, A., and Wicherts, J. M. (2020). Reproducibility of individual effect sizes in meta-analyses in psychology. PLoS One 15:e0233107. doi: 10.1371/journal.pone.0233107
Marsh, H. W., Pekrun, R., Parker, P. D., Murayama, K., Guo, J., Dicke, T., et al. (2019). The murky distinction between self-concept and self-efficacy: beware of lurking jingle-jangle fallacies. J. Educ. Psychol. 111, 331–353. doi: 10.1037/edu0000281
Ogilvie, D., Fayter, D., Petticrew, M., Sowden, A., Thomas, S., Whitehead, M., et al. (2008). The harvest plot: a method for synthesising evidence about the differential effects of interventions. BMC Med. Res. Methodol. 8:8. doi: 10.1186/1471-2288-8-8
Page, M. J., Moher, D., Bossuyt, P. M., Boutron, I., Hoffmann, T. C., Mulrow, C. D., et al. (2021). PRISMA 2020 explanation and elaboration: updated guidance and exemplars for reporting systematic reviews. BMJ 372:n160. doi: 10.1136/bmj.n160
Pigott, T. D., and Polanin, J. R. (2020). Methodological guidance paper: high-quality meta-analysis in a systematic review. Rev. Educ. Res. 90, 24–46. doi: 10.3102/0034654319877153
Polanin, J. R., Hennessy, E. A., and Tsuji, S. (2020). Transparency and reproducibility of meta-analyses in psychology: a meta-review. Perspect. Psychol. Sci. 15, 1026–1041. doi: 10.1177/1745691620906416
Porter, T. (2023). Jingle-jangle fallacies in intellectual humility research. J. Posit. Psychol. 18, 221–223. doi: 10.1080/17439760.2022.2154698
Shadish, W. R., and Lecy, J. D. (2015). The meta-analytic big bang. Res. Synth. Methods 6, 246–264. doi: 10.1002/jrsm.1132
Simonsohn, U., Simmons, J. P., and Nelson, L. D. (2020). Specification curve analysis. Nat. Hum. Behav. 4, 1208–1214. doi: 10.1038/s41562-020-0912-z
Skinner, E. A. (1996). A guide to constructs of control. J. Pers. Soc. Psychol. 71, 549–570. doi: 10.1037/0022-3514.71.3.549
Steegen, S., Tuerlinckx, F., Gelman, A., and Vanpaemel, W. (2016). Increasing transparency through a multiverse analysis. Perspect. Psychol. Sci. 11, 702–712. doi: 10.1177/1745691616658637
Thorndike, E. L. (1904). An introduction to the theory of mental and social measurements. New York: Teachers College, Columbia University.
Uher, J. (2023). What are constructs? Ontological nature, epistemological challenges, theoretical foundations and key sources of misunderstandings and confusions. Psychol. Inq. 34, 280–290. doi: 10.1080/1047840X.2023.2274384
Uttley, L., Quintana, D. S., Montgomery, P., Carroll, C., Page, M. J., Falzon, L., et al. (2023). The problems with systematic reviews: a living systematic review. J. Clin. Epidemiol. 156, 30–41. doi: 10.1016/j.jclinepi.2023.01.011
Voracek, M., Kossmeier, M., and Tran, U. S. (2019). Which data to meta-analyze, and how? A specification-curve and multiverse-analysis approach to meta-analysis. Z. Psychol. 227, 64–82. doi: 10.1027/2151-2604/a000357
Wicherts, J. M., Veldkamp, C. L. S., Augusteijn, H. E. M., Bakker, M., van Aert, R. C. M., and van Assen, M. A. L. M. (2016). Degrees of freedom in planning, running, analyzing, and reporting psychological studies: a checklist to avoid p-hacking. Front. Psychol. 7:1832. doi: 10.3389/fpsyg.2016.01832
Keywords: jingle fallacies, jangle fallacies, validity, meta-analysis, systematic review, specification analysis, harvest plot
Citation: Hanfstingl B, Oberleiter S, Pietschnig J, Tran US and Voracek M (2024) Detecting jingle and jangle fallacies by identifying consistencies and variabilities in study specifications – a call for research. Front. Psychol. 15:1404060. doi: 10.3389/fpsyg.2024.1404060
Received: 20 March 2024; Accepted: 14 August 2024;
Published: 30 August 2024.
Edited by:
Mengcheng Wang, Guangzhou University, ChinaReviewed by:
Longxi Li, University of Washington, United StatesCopyright © 2024 Hanfstingl, Oberleiter, Pietschnig, Tran and Voracek. This is an open-access article distributed under the terms of the Creative Commons Attribution License (CC BY). The use, distribution or reproduction in other forums is permitted, provided the original author(s) and the copyright owner(s) are credited and that the original publication in this journal is cited, in accordance with accepted academic practice. No use, distribution or reproduction is permitted which does not comply with these terms.
*Correspondence: Barbara Hanfstingl, YmFyYmFyYS5oYW5mc3RpbmdsQGFhdS5hdA==
†ORCID: Barbara Hanfstingl, https://orcid.org/0000-0002-2458-7585
Sandra Oberleiter, https://orcid.org/0000-0003-1291-6609
Jakob Pietschnig, https://orcid.org/0000-0003-0222-9557
Ulrich S. Tran, https://orcid.org/0000-0002-6589-3167
Martin Voracek, https://orcid.org/0000-0001-6109-6155
Disclaimer: All claims expressed in this article are solely those of the authors and do not necessarily represent those of their affiliated organizations, or those of the publisher, the editors and the reviewers. Any product that may be evaluated in this article or claim that may be made by its manufacturer is not guaranteed or endorsed by the publisher.
Research integrity at Frontiers
Learn more about the work of our research integrity team to safeguard the quality of each article we publish.