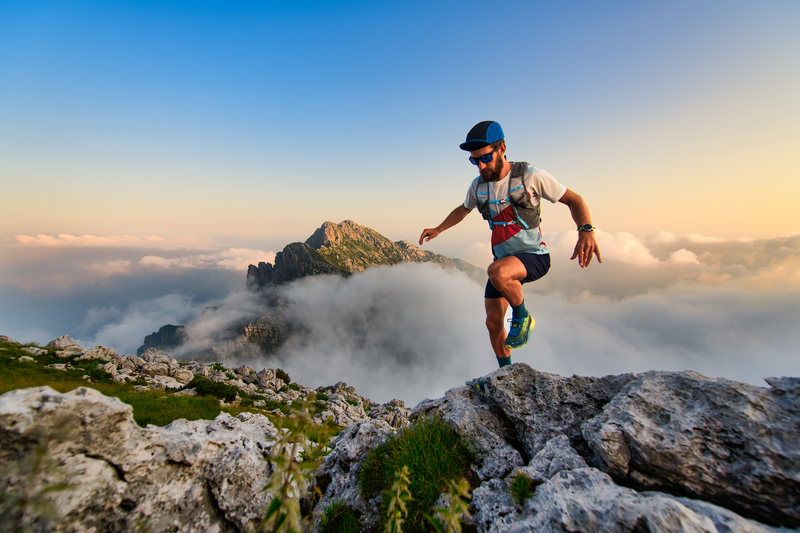
95% of researchers rate our articles as excellent or good
Learn more about the work of our research integrity team to safeguard the quality of each article we publish.
Find out more
ORIGINAL RESEARCH article
Front. Psychol. , 08 January 2025
Sec. Addictive Behaviors
Volume 15 - 2024 | https://doi.org/10.3389/fpsyg.2024.1395957
This article is part of the Research Topic Executive Functions, Reward Systems and Addiction in Adolescents and Young Adults View all 5 articles
Objective: Problematic media use (PMU) is addiction-like media use. No study has examined if genetic factors for addiction relate to PMU during childhood. This study tested the association between genetic risk factors for addiction and PMU among 9-to-12-year-olds.
Method: Data were from a cohort of 9-to-12-year-olds recruited from Northern New England, 2018–2022, for a study examining obesity risk among children. Two polymorphisms related to dopaminergic (ANNK1 rs1800497 [the Taq1A polymorphism] and COMT rs4680) and one related to nicotinic (CHRNA4 rs1044396) pathways that were previously associated with internet addiction or internet video game addiction in adolescents and young adults were genotyped. Parent-reported PMU for children was measured with a validated nine-item scale (range for final scores: 1 to 5); higher scores indicate more severe PMU.
Results: Among children (n = 180; 43.9% female; 90.0% white, non-Hispanic; 82.2% of parents were college graduates), the median PMU score was 2.22 (IQR: 1.78, 2.78). In a linear regression model adjusted for child age, sex, European ancestry, and parent education, there was an additive association between the number of Taq1A1 alleles and PMU among children. Specifically, geometric mean PMU scores were 8.6% greater for each additional copy of the Taq1A1 allele (p = 0.030; R2 = 5.2%). No other polymorphisms were statistically associated with PMU at the p < 0.05 level.
Conclusion: These preliminary findings suggest that a genetic predisposition to reduced dopamine sensitivity as indicated by the Taq1A polymorphism may relate to PMU in early adolescence. Findings need confirmation in larger samples.
Problematic media use (PMU) reflects addiction-like media use that can negatively affect wellbeing. PMU includes preoccupation with media, use of media for mood regulation, increasing tolerance and withdrawal symptoms around media use, and media use interfering with family relationships (Domoff et al., 2019; Domoff et al., 2021; Kuss et al., 2014). Personal ownership of smartphones rapidly increases during the preadolescent years (The Common Sense Census: Media Use by Tweens and Teens, 2021), and PMU specific to smartphones has been documented in several studies of adolescents and young adults, with prevalence estimates ranging from 14 to 31% (Sohn et al., 2019).
The media content frequently accessed by preadolescents, including online video platforms and social media (The Common Sense Census: Media Use by Tweens and Teens, 2021), are highly engineered and persuasively designed to prolong engagement, with features such as intermittent reinforcement schedules for presenting highly enjoyable content and indicators of social approval (e.g., “likes”) (Franqueira et al., 2022; Griffiths, 2018; Kuss and Griffiths, 2017; Bringing Dark Patterns to Light: An FTC Workshop, 2022; Lindström et al., 2021). Importantly, a user’s interaction with online media is tailored using extremely sophisticated algorithms based on user-specific data to maximize the likelihood that the content presented is enticing (Bringing Dark Patterns to Light: An FTC Workshop, 2022). The design of online video platforms and social media have, in fact, been compared to slot machines (The Perfect Slot Machine, 2022), where rewards are anticipated but intermittent to prolong engagement (Lindström et al., 2021). Gaming apps, even those marketed as educational (Meyer et al., 2021), are also often persuasively designed with rewards that are “unlocked” with continued play. Online games popular with preadolescents also include virtual social networks that may increase a child’s affinity for mobile media use (Kuss and Griffiths, 2017). As presented in the Interaction of Person-Affect-Cognition-Execution (I-PACE) model for the development of internet use disorder (Brand et al., 2016), engaging with such rewarding content may train reactive and reward-seeking behavior that reinforces media use and may start a cycle of addiction-like behavior around media use. Preadolescents may be especially vulnerable to the persistent and highly accessible reward-based training that mobile media devices provide because neural networks that manage behavior and self-regulation do not fully mature until late adolescence (Strang et al., 2013; Klaczynski, 2004; Stevens, 2016). Importantly, such media use behaviors may be harmful to mental health. A meta-analysis of 18 studies that enrolled adolescents and young adults documented positive associations between problematic social media use and depression, anxiety, and stress (Shannon et al., 2022).
Few studies have investigated a potential genetic predisposition to addiction-like media use and all have been completed among adolescents or young adults with a focus on internet gaming disorders (Han et al., 2007; Lee et al., 2008; Montag et al., 2012; Jeong et al., 2017; Tereshchenko, 2023). Specifically, gene variants previously associated with internet gaming disorder or addiction include SNPs rs180097 (ANKK1) (Han et al., 2007), rs4680 (COMT) (Han et al., 2007), and rs1044396 (CHRNA4) (Montag et al., 2012; Jeong et al., 2017) and short/long variants of 5HTTLPR (Lee et al., 2008). In particular, each of these SNPs relates to addictive behaviors. rs1800497 (i.e., the Taq1A polymorphism) is in an exon of the ANKK1 gene (previously believed to be within the DRD2 gene) that impacts the dopamine receptor DRD2 (ANKK1, n.d.). The alternate allele (Taq1A1; a G > A variant) is associated with alcohol dependence (Smith et al., 2008) and other disorders of self-regulation including cocaine use (Blum et al., 1996) and overeating (Sun et al., 2017). COMT rs4680 results in a variant of catechol-O-methyltransferase (COMT), an enzyme that degrades catecholamines including dopamine (COMT, n.d.). The alternate allele (a G > A variant) results in greater instability and less enzymatic activity of COMT (COMTL) (Chen et al., 2004) and has been associated with the risk of major depressive disorder in some (Bækken et al., 2008; Wang et al., 2016) but not a majority (Antypa et al., 2013) of studies. rs4680 may also relate to nicotine dependence (Choi and Shin, 2015) including among adolescents (O’Loughlin et al., 2014), although findings are not conclusive (Choi and Shin, 2015). CHRNA4 encodes the cholinergic receptor nicotinic alpha 4 subunit, a nicotinic acetylcholine receptor (CHRNA4, n.d.), and the alternate allele in rs1044396 (a G > A variant) has been related to less nicotine dependence (Feng et al., 2004). 5HTTLPR is a repeat polymorphism in the promotor region of SLC6A4, a gene that encodes the serotonin transporter (SLC6A4, n.d.). 5HTTLPR has two variants, a short and long version that differ in the number of 44-bp repeats in the promotor region (Heils et al., 2002), although extra-long variants have been reported (Goldman et al., 2010). The short-form variant relates to reduced serotonin transporter expression (SLC6A4, n.d.) and has been associated with an increased susceptibility to major depression and alcohol dependence (Oo et al., 2016), although findings are not conclusive (Goldman et al., 2010; Fratelli et al., 2020).
No studies have assessed if preadolescents with these genetic risk factors may be more susceptible to the rewarding effects of digital media and, in turn, be more prone to developing addiction-like media use behaviors. Therefore, the goal of this study was to leverage existing genetic data to examine the associations between previously documented genetic risk factors for internet addiction and problematic media use among 9-to-12-year-old children. It was hypothesized that children with SNPs reflective of a greater risk for addiction (e.g., the presence of the Taq1A1 allele in rs180097) or depression (e.g., variants in rs4680) would demonstrate greater PMU.
Data are from a cohort of 9-to-12-year-old children enrolled in a prospective study to analyze genetic risk factors related to pediatric obesity. Children and one parent each residing in Northern New England were recruited from the community. Data for this secondary analysis were collected from study activities that took place between April 2018 and April 2023 when saliva was collected for genetic analyses at the baseline visit. Children were eligible for the primary study if they were fluent in English. Children who had any relevant food allergies or dietary restrictions; were taking appetite- or attention-altering medications; had an attention, psychiatric, or neurological disorder; or who had a contradiction for an MRI scan (completed in the parent study) were excluded. This current analysis used data from the baseline visit that included the collection of a saliva sample from children for genotyping and an online survey completed by parents. Child–parent dyads were compensated up to $75 for completing that baseline study visit.
Saliva samples were collected from 189 children at the baseline visit with the Oragene Kit (DNA Genotek, Kanata, Canada), and per the original study protocol, isolated DNA was genotyped for more than 600,000 single nucleotide polymorphisms (SNPs) with the Illumina Global Screening Array 24 (v1.0 or v3.0); GenomeStudio software was used to generate clusters and genotype calls using manufacturer-recommended quality control thresholds (Illumina, 2014). As previously described (Renier et al., 2023), R (R Core Team, 2021) and PLINK (Chang et al., 2015; Meyer, 2023) were used for quality control. One participant was excluded for genotyping rate < 5%, five for outlying heterozygosity, and one for sex discordant from self-report. No sibling pairs existed in the sample (identity-by-descent pi-hat >0.2). Genotype data were available on 182 participants after quality control. SNPs deviating from Hardy–Weinberg equilibrium (Fisher’s exact test , with more than 5% missingness, or with minor allele frequency < 1% were removed. Genetic European ancestry was determined as previously described (Renier et al., 2023; Meyer, 2023; Auton et al., 2015). To expand the scope of SNP data, haplotype-based imputation was performed using the Michigan Imputation Server (das et al., 2016) as previously described (Renier et al., 2023; McCarthy et al., 2016).
The current analysis initially considered four genetic variants previously related to internet video game addiction or internet addiction among adolescents or young adults: (Han et al., 2007; Lee et al., 2008; Montag et al., 2012; Jeong et al., 2017) the single nucleotide polymorphisms (SNPs) rs1800497, rs4680, and rs1044396 and the repeat polymorphism 5HTTLPR. SNPs rs1800497 (TaqA1 in ANKK1) and rs4680 (COMT) were directly genotyped and had complete information for all participants; rs1044396 (CHRNA4) was also directly genotyped but was treated as missing for three participants where genotype calling failed and imputation resulted in a posterior genotype probability below 0.9. The genotype frequencies for rs1800497, rs4680, and rs1044396 were each in Hardy–Weinberg equilibrium (exact test p = 0.847, 0.135, and 0.764, respectively). Short/long alleles of 5HTTLPR were assessed with two SNP haplotype proxies, including rs4251417 and rs2020934 (Wray et al., 2009), though the resulting genotypes deviated from Hardy–Weinberg equilibrium (exact test p = 0.013) and were excluded from downstream analyses.
Parents completed the 9-item, Problematic Media Use Measure, short form (Domoff et al., 2019) for their child. This scale was developed to measure PMU among children 4-to-11-year-olds and was based on the nine DSM-V criteria for internet gaming disorder. Scale items address preoccupation with and unsuccessful control of media use, loss of interest in other activities, psychosocial and family-related problems because of media use, use of media to relieve mood, withdrawal, tolerance, and deception related to use. The internal reliability of the scale in the original validation study was high (α = 0.93) (Domoff et al., 2019). Parents rated how much each item reflects their child; all items are scored on a 5-point Likert scale anchored at never (1) and always (5). Final scores are the mean across all nine items with higher scores reflecting more severe PMU. There is no established threshold for this scale to create a binary classification of PMU, and scores were treated as continuous in all analyses. Two of the 182 participants with genetic data were missing the PMU measure, and thus, the final sample size was 180.
Parents reported their child’s date of birth to compute age and their child’s biological sex, race, and ethnicity; parents reported their relationship to the child, their own educational level, annual household income, and marital status. Parents also reported which media devices their child used in the past month (TV set, desktop or laptop computer, touchscreen tablet, smartphone, video game console, etc.) and their child’s typical screen media use per week (hours) across all devices. Specifically, parents were asked to report the total time their child spent across all of those media devices for a weekday and separately, a weekend day. Parents were asked to include non-school leisure use only. Total leisure screen media use per week was a weighted sum of weekday (weight = 5/7) and weekend (weight = 2/7) use.
The distribution of PMU was right skewed and thus natural log transformed for regression analyses. Specifically, linear regression was used to model PMU (log-transformed) on each genetic risk factor, separately, adjusted for child age, biological sex, European ancestry, and parent education. Covariates were selected a priori and initially included household income; however, household income was not statistically associated with PMU and its inclusion did not improve the model fit as measured by the coefficient of determination (R2) and was thus not retained in the model. Regression analyses for each variant considered additive, dominant, and recessive models. The alternate allele of each SNP was modeled as the risk allele for each analysis. Residual plots were inspected and there were no apparent violations of model assumptions (normality of residuals, homoscedasticity, and no high leverage values per Cook’s distance). p < 0.05 was the threshold for statistical significance for all main effects. There were no adjustments for multiple comparisons. All analyses were conducted with the R Language and Environment for Statistical Computing, version 3.15.
Written informed consent was collected from the parent, and children provided verbal and written study assent. All study activities were approved of by the Committee for the Protection of Human Subjects at Dartmouth College (Protocol 30723; approved 12/15/2017).
The mean age of children in the analytic sample (n = 180) was 10.9 (SD 1.2) years, 43.9% of children were female, and most (90.0%) were identified by the parent as white, non-Hispanic. Families were largely from a higher socioeconomic status (Table 1). Children averaged 18.1 (SD 12.1) h per week of non-school-related screen media use, and nearly all (92.2%) of children used a touchscreen tablet or smartphone in the past month. The median PMU was 2.22 (IQR: 1.78, 2.78) which reflects a median response of rarely (2) to sometimes (3) across the nine scale items. Children’s leisure screen time per week was moderately correlated to PMU (Pearson’s r for log-transformed PMU and total screen time = 0.27; p < 0.001). The distribution of genotypes is presented in Table 2. Mean leisure screen media use per week was unrelated to rs1800497, rs4680, or rs1044396 genotype (all ANOVA p-values >0.117). Median PMU differed by rs1800497 genotype, which was of borderline statistical significance (Kruskal–Wallis p = 0.054; Figure 1) but did not differ by rs4680 (p = 0.917) or rs1044396 genotype (p = 0.944).
Table 2. Genotype frequencies of the final three candidate genetic risk factors for problematic media use.
Figure 1. Distribution of problematic media use (PMU) scores by rs1800497 (Taq1A) genotype (n = 180).
In adjusted regression models (Table 3), there was an additive association between the number of Taq1A1 alleles and greater PMU. Specifically, adjusted geometric mean PMU scores were 8.6% (95% CI: 0.8, 16.3%) greater for each additional copy of the Taq1A1 allele (p = 0.030; R2 = 5.2%) and were 2.13 for homozygous low-risk (n = 99), 2.31 for heterozygous (n = 70), and 2.58 for homozygous high-risk (n = 11) children. A dominant (p = 0.041; R2 = 4.9%) model was also supported by the data, although the additive model had a slightly better fit (Table 3). A recessive model was not statistically related to PMU (p = 0.209; R2 = 3.5%).
Table 3. Adjusted associations between the four candidate genetic risk factors and problematic media use, log-transformed.
No other allele variants were statistically associated with PMU at the p < 0.05 level. Findings were consistent when limiting the analysis to children of European descent, although the additive model for rs1800497 was not statistically significant (p = 0.097) and the dominant model for rs1800497 was borderline significant (p = 0.055) in this subset (Supplementary Table 1). In particular, post-hoc tests for an interaction between rs1800497 genotype and European ancestry were not statistically significant in the full dataset for the additive or dominant models (p-for-interaction = 0.273 and 0.858, respectively).
In this preliminary study of 180 9-to-12-year-olds, rs1800497 (i.e., the Taq1A polymorphism) was positively associated with more severe PMU as measured with a validated scale appropriate for youth. This finding aligns with that of Han et al. (2007), where the rs1800497 alternate allele was more frequent among excessive internet video game players (n = 79) than age-matched controls (n = 75) in a study of male adolescents. This is the second study to suggest that rs1800497 relates to more addictive-like media use behaviors and the first to examine this association among preadolescents. Findings are important to understand the neurological mechanisms underlying the development of PMU and if some children may be more susceptible to PMU because of a genetic risk factor.
The alternate allele (A1) of rs1800497 results in a decreased density and binding potential of DRD2 in the striatum in humans and this association follows an additive model (Ritchie and Noble, 2003; Jönsson et al., 1999; Pohjalainen et al., 1998; Gluskin and Mickey, 2016). As stated by Blum et al. (1996) and Blum et al. (2000), a dampened responsivity to dopamine likely contributes to a reward deficiency syndrome that increases the risk of addictive, impulsive, and compulsive behaviors. The media contemporary preadolescents engage with is highly engineered to be persuasive and to prolong user engagement (Franqueira et al., 2022; Griffiths, 2018; Kuss and Griffiths, 2017; Bringing Dark Patterns to Light: An FTC Workshop, 2022; Lindström et al., 2021), which can result in compulsive use of mobile devices (Soror et al., 2015). Social media itself can be uniquely rewarding. Sherman et al. found heightened activity in reward networks of the brain when adolescents viewed photographs with many “likes” (Sherman et al., 2016) or when “liking” photographs from others (Sherman et al., 2018) using a simulated Instagram platform. Thus, it is feasible that those with reward deficiency syndrome may be most prone to develop PMU because of the rewarding nature of contemporary screen media. Unfortunately, our study did not measure children’s media use on the different device types or platforms, and we cannot test whether rs1800497 is related to the use of more (e.g., social media) vs. less (e.g., watching TV shows) persuasively engineered media.
This study did not find an association between PMU and rs4680 or rs1044396. rs4680 affects the stability of catechol-O-methyltransferase and the degradation of dopamine, suggesting that once introduced, dopamine is available in the synapses longer for those with COMT of low enzymatic activity (COMTL) (Chen et al., 2004). A previous case–control study of Asian male adolescents found a greater frequency of the allele encoding COMTL among excessive internet game players (0.329) versus the control group (0.233) (Han et al., 2007). Regarding rs1044396, differing findings were reported in two previous studies examining rs1044396 and internet addiction, where the alternate (Montag et al., 2012) or reference (Jeong et al., 2017) allele related to greater internet addiction in two separate studies. This current study did not define cases with PMU and the overall mean value of PMU was low. It is possible that rs4680 or rs1044396 affects the severity or persistence of a media-based disorder once established but is not involved in the early development of that disorder. Conversely, the previous findings could have been confounded by comorbid mental health conditions (Lee et al., 2008; Ha et al., 2006).
Children’s total leisure screen media use per week was positively correlated with PMU in this current study. Screen time did not consider time spent on mobile devices specifically, and a direct test of persuasive media use and PMU is not possible in our study. However, 92.2% of preadolescents in the study used a mobile device in the past month, suggesting mobile device use was common. Screen time was unrelated to rs1044396 genotype, however, suggesting that the association with PMU is not fully dependent on total screen use quantity. Indeed, PMU captures many elements of problematic use including screen use to regulate negative mood. Longitudinal studies are needed to understand how a genetic risk for addiction relates to PMU considering the trajectories of screen use quantity and quality over time. That information is needed to best inform preventive interventions and guidance for parents and youth.
PMU was measured with a validated scale based on the nine DSM-V criteria for internet gaming disorder (Domoff et al., 2019). Importantly, this PMU measure is not specific to device type, which is an important consideration as preadolescent children frequently access online media via multiple devices including smartphones and touchscreen tablets (The Common Sense Census: Media Use by Tweens and Teens, 2021). However, measuring PMU in preadolescents is relatively new and thresholds to define clinically relevant PMU do not yet exist. Problematic smartphone use, defined as answering yes to at least six items of the 9-item Social Media Disorder Scale, was consistently related to lower mental, school, and social wellbeing in a study among 154,981 adolescents across 29 countries (Boer et al., 2020). That scale was also designed based on clinical criteria of addiction and scale items are similar to PMU items, suggesting that increased PMU may similarly relate to wellbeing among adolescents. The mean PMU was below 3 in this current study or a mean response of “sometimes” to each scale item. Additional studies are needed to understand how rs1800497 may affect PMU at more extreme levels, and if that genetic risk increases with age.
Strengths of this study include the collection of genetic data along with PMU among a sample of preadolescents. As a limitation, PMU and child media use were parent-reported and may be biased. This sample included children with lower levels of media use than national norms (The Common Sense Census: Media Use by Tweens and Teens, 2021), and the sample excluded children taking medications for attention disorders. Thus, included children were at a relatively low risk of developing more severe PMU. The sample was mostly white, non-Hispanic of higher socioeconomic status, limiting the generalizability of study findings. There were a limited number of preadolescents with two copies of the Taq1A1 allele (n = 11), which limits inference. The borderline findings among the subset of children with European ancestry may particularly be affected by a small sample size. We further did not measure comorbid mental health conditions that may confound reported associations. The goal of this study was not to conduct a genome-wide association study, but instead to test specific candidate SNPs as informed by the literature that suggests screen time may impact dopaminergic neural pathways.
In summary, this study found a positive, additive association between rs1800497, the Taq1A polymorphism, and more severe PMU among a sample of preadolescents. The effect size was small, and results need to be replicated in more diverse samples. Future studies should additionally include confirmation of PMU based on clinical criteria versus solely relying on parent reports Regardless, findings support that a neurological mechanism related to a reward deficiency may make some children more prone to developing addiction-like media use behaviors.
The datasets presented in this study can be found in online repositories. The names of the repository/repositories and accession number(s) can be found at: https://www.ncbi.nlm.nih.gov/gap/, phs003550.v1.
The studies involving humans were approved by the Committee for the Protection of Human Subjects at Dartmouth College. The studies were conducted in accordance with the local legislation and institutional requirements. Written informed consent for participation in this study was provided by the participants’ legal guardians/next of kin.
JE: Conceptualization, Formal analysis, Writing – original draft, Writing – review & editing. TR: Formal analysis, Writing – review & editing. DY: Formal analysis, Writing – review & editing. DC: Project administration, Writing – review & editing, Methodology. GB: Project administration, Writing – review & editing, Methodology. DG-D: Conceptualization, Data curation, Resources, Writing – review & editing.
The author(s) declare financial support was received for the research, authorship, and/or publication of this article. This study was supported by the National Institutes for Health (R01HD092604 and K01DK117971).
The authors declare that the research was conducted in the absence of any commercial or financial relationships that could be construed as a potential conflict of interest.
All claims expressed in this article are solely those of the authors and do not necessarily represent those of their affiliated organizations, or those of the publisher, the editors and the reviewers. Any product that may be evaluated in this article, or claim that may be made by its manufacturer, is not guaranteed or endorsed by the publisher.
The Supplementary material for this article can be found online at: https://www.frontiersin.org/articles/10.3389/fpsyg.2024.1395957/full#supplementary-material
ANKK1. (n.d.). ANKK1 ankyrin repeat and kinase domain containing 1 [Homo sapiens (human)] – gene – NCBI. Available at: https://www.ncbi.nlm.nih.gov/gene/255239 (Accessed December 27, 2024).
Antypa, N., Drago, A., and Serretti, A. (2013). The role of COMT gene variants in depression: bridging neuropsychological, behavioral and clinical phenotypes. Neurosci. Biobehav. Rev. 37, 1597–1610. doi: 10.1016/j.neubiorev.2013.06.006
Auton, A., Brooks, L. D., Durbin, R. M., Garrison, E. P., Kang, H. M., and Korbel, J. O. (2015). A global reference for human genetic variation. Nature 526, 68–74. doi: 10.1038/nature15393
Bækken, P. M., Skorpen, F., Stordal, E., Zwart, J. A., and Hagen, K. (2008). Depression and anxiety in relation to catechol-O-methyltransferase Val158Met genotype in the general population: the Nord-Trøndelag health study (HUNT). BMC Psychiatry 8:48. doi: 10.1186/1471-244X-8-48
Blum, K., Braverman, E. R., Holder, J. M., Lubar, J. F., Monastra, V. J., Miller, D., et al. (2000). The reward deficiency syndrome: a biogenetic model for the diagnosis and treatment of impulsive, addictive and compulsive behaviors. J. Psychoactive Drugs 32, 1–112. doi: 10.1080/02791072.2000.10736099
Blum, K., Sheridan, P. J., Wood, R. C., Braverman, E. R., Chen, T. J. H., phd, J. G. C., et al. (1996). The D2 dopamine receptor gene as a determinant of reward deficiency syndrome. J. R. Soc. Med. 89, 396–400. doi: 10.1177/014107689608900711
Boer, M., van den Eijnden, R. J. J. M., Boniel-Nissim, M., Wong, S. L., Inchley, J. C., Badura, P., et al. (2020). Adolescents’ intense and problematic social media use and their well-being in 29 countries. J. Adolesc. Health 66, S89–S99. doi: 10.1016/j.jadohealth.2020.02.014
Brand, M., Young, K. S., Laier, C., Wölfling, K., and Potenza, M. N. (2016). Integrating psychological and neurobiological considerations regarding the development and maintenance of specific internet-use disorders: an interaction of person-affect-cognition-execution (I-PACE) model. Neurosci. Biobehav. Rev. 71, 252–266. doi: 10.1016/j.neubiorev.2016.08.033
Bringing Dark Patterns to Light: An FTC Workshop. (2022). Federal Trade Commission. Available at: https://www.ftc.gov/news-events/events/2021/04/bringing-dark-patterns-light-ftc-workshop (Accessed July 29, 2022).
Chang, C. C., Chow, C. C., Tellier, L. C. A. M., Vattikuti, S., Purcell, S. M., and Lee, J. J. (2015). Second-generation PLINK: rising to the challenge of larger and richer datasets. Gigascience 4:7. doi: 10.1186/s13742-015-0047-8
Chen, J., Lipska, B. K., Halim, N., Ma, Q. D., Matsumoto, M., Melhem, S., et al. (2004). Functional analysis of genetic variation in catechol-O-methyltransferase (COMT): effects on mrna, protein, and enzyme activity in postmortem human brain. Am. J. Hum. Genet. 75, 807–821. doi: 10.1086/425589
Choi, H. D., and Shin, W. G. (2015). Association between catechol-O-methyltransferase (COMT) Val/met genotype and smoking cessation treatment with nicotine: a meta-analysis. Pharmacogenomics 16, 1879–1885. doi: 10.2217/pgs.15.127
CHRNA4 (n.d.). CHRNA4 cholinergic receptor nicotinic alpha 4 subunit [Homo sapiens (human)] – gene – NCBI. Available at: https://www.ncbi.nlm.nih.gov/gene/1137 (Accessed December 10, 2024).
COMT (n.d.). COMT catechol-O-methyltransferase [Homo sapiens (human)] – Gene – NCBI. Available at: https://www.ncbi.nlm.nih.gov/gene/1312 (Accessed December 10, 2024).
das, S., Forer, L., Schönherr, S., Sidore, C., Locke, A., Kwong, A., et al. (2016). Next-generation genotype imputation service and methods. Nat. Genet. 48, 1284–1287. doi: 10.1038/ng.3656
Domoff, S. E., Borgen, A. L., Kim, S. J., and Emond, J. A. (2021). Prevalence and predictors of children’s persistent screen time requests: a national sample of parents. Hum. Behav. Emerg. Technol. 3, 700–709. doi: 10.1002/hbe2.322
Domoff, S. E., Harrison, K., Gearhardt, A. N., Gentile, D. A., Lumeng, J. C., and Miller, A. L. (2019). Development and validation of the problematic media use measure: a parent report measure of screen media “addiction” in children. Psychol. Pop. Media Cult. 8, 2–11. doi: 10.1037/ppm0000163
Feng, Y., Niu, T., Xing, H., Xu, X., Chen, C., Peng, S., et al. (2004). A common haplotype of the nicotine acetylcholine receptor α4 subunit gene is associated with vulnerability to nicotine addiction in men. Am. J. Hum. Genet. 75, 112–121. doi: 10.1086/422194
Franqueira, VNL, Annor, JA, and Kafali, O. (2022). Age appropriate design: assessment of TikTok, twitch, and YouTube kids. Available at: http://arxiv.org/abs/2208.02638 (Accessed September 10, 2022).
Fratelli, C., Siqueira, J., Silva, C., Ferreira, E., and Silva, I. (2020). 5httlpr genetic variant and major depressive disorder: a review. Genes 11, 1–21. doi: 10.3390/genes11111260
Gluskin, B. S., and Mickey, B. J. (2016). Genetic variation and dopamine D2 receptor availability: a systematic review and meta-analysis of human in vivo molecular imaging studies. Transl. Psychiatry 6:e747. doi: 10.1038/tp.2016.22
Goldman, N., Glei, D. A., Lin, Y. H., and Weinstein, M. (2010). The serotonin transporter polymorphism (5-HTTLPR): allelic variation and links with depressive symptoms. Depress. Anxiety 27, 260–269. doi: 10.1002/da.20660
Griffiths, M. D. (2018). Adolescent social networking: how do social media operators facilitate habitual use? Educ. Health 36, 66–69.
Ha, J. H., Yoo, H. J., Cho, I. H., Chin, B., Shin, D., and Kim, J. H. (2006). Psychiatric comorbidity assessed in Korean children and adolescents who screen positive for internet addiction. J. Clin. Psychiatry 67, 821–826. doi: 10.4088/JCP.v67n0517
Han, D. H., Lee, Y. S., Yang, K. C., Kim, E. Y., Lyoo, I. K., and Renshaw, P. F. (2007). Dopamine genes and reward dependence in adolescents with excessive internet video game play. J. Addict. Med. 1, 133–138. doi: 10.1097/ADM.0b013e31811f465f
Heils, A., Teufel, A., Petri, S., Stöber, G., Riederer, P., Bengel, D., et al. (2002). Allelic variation of human serotonin transporter gene expression. J. Neurochem. 66, 2621–2624. doi: 10.1046/j.1471-4159.1996.66062621.x
Illumina. (2014). Infinium genotyping data analysis. Available at: https://www.illumina.com/Documents/products/technotes/technote_infinium_genotyping_data_analysis.pdf (Accessed January 26, 2023).
Jeong, J., Rhee, J., Kim, T., Kwak, S., Bang, S., Cho, H., et al. (2017). The association between the nicotinic acetylcholine receptor α4 subunit gene (CHRNA4) rs1044396 and internet gaming disorder in Korean male adults. PLoS One 12:e0188358. doi: 10.1371/journal.pone.0188358
Jönsson, E. G., Nöthen, M. M., Grünhage, F., Farde, L., Nakashima, Y., Propping, P., et al. (1999). Polymorphisms in the dopamine D2 receptor gene and their relationships to striatal dopamine receptor density of healthy volunteers. Mol. Psychiatry 4, 290–296. doi: 10.1038/sj.mp.4000532
Klaczynski, P. A. (2004). A dual-process model of adolescent development: implications for decision making, reasoning, and identity. Adv. Child Dev. Behav. 32, 73–123. doi: 10.1016/S0065-2407(04)80005-3
Kuss, D. J., and Griffiths, M. D. (2017). Social networking sites and addiction: ten lessons learned. Int. J. Environ. Res. Public Health 14, 351–366. doi: 10.3390/ijerph14030311
Kuss, D. J., Shorter, G. W., van Rooij, A. J., Griffiths, M. D., and Schoenmakers, T. M. (2014). Assessing internet addiction using the parsimonious internet addiction components model-a preliminary study. Int. J. Ment. Health Addict. 12, 351–366. doi: 10.1007/s11469-013-9459-9
Lee, Y. S., Han, D. H., Yang, K. C., Daniels, M. A., Na, C., Kee, B. S., et al. (2008). Depression like characteristics of 5HTTLPR polymorphism and temperament in excessive internet users. J. Affect. Disord. 109, 165–169. doi: 10.1016/j.jad.2007.10.020
Lindström, B., Bellander, M., Schultner, D. T., Chang, A., Tobler, P. N., and Amodio, D. M. (2021). A computational reward learning account of social media engagement. Nat. Commun. 12:1311. doi: 10.1038/s41467-020-19607-x
McCarthy, S., das, S., Kretzschmar, W., Delaneau, O., Wood, A. R., Teumer, A., et al. (2016). A reference panel of 64,976 haplotypes for genotype imputation. Nat. Genet. 48, 1279–1283. doi: 10.1038/ng.3643
Meyer, HV. (2023). plinkQC: genotype quality control in genetic association studies. Available at: https://meyer-lab-cshl.github.io/plinkQC/index.html (Accessed January 26, 2023).
Meyer, M., Zosh, J. M., McLaren, C., Robb, M., McCaffery, H., Golinkoff, R. M., et al. (2021). How educational are “educational” apps for young children? App store content analysis using the four pillars of learning framework. J. Child. Media 15, 526–548. doi: 10.1080/17482798.2021.1882516
Montag, C., Kirsch, P., Sauer, C., Markett, S., and Reuter, M. (2012). The role of the CHRNA4 gene in internet addiction. J. Addict. Med. 6, 191–195. doi: 10.1097/ADM.0b013e31825ba7e7
O’Loughlin, J., Sylvestre, M. P., Labbe, A., Low, N. C., Roy-Gagnon, M., Dugas, E. N., et al. (2014). Genetic variants and early cigarette smoking and nicotine dependence phenotypes in adolescents. PLoS One 9:e0115716. doi: 10.1371/journal.pone.0115716
Oo, K. Z., Aung, Y. K., Jenkins, M. A., and Win, A. K. (2016). Associations of 5HTTLPR polymorphism with major depressive disorder and alcohol dependence: a systematic review and meta-analysis. Aust. N. Z. J. Psychiatry 50, 842–857. doi: 10.1177/0004867416637920
Pohjalainen, T., Rinne, J. O., Någren, K., Lehikoinen, P., Anttila, K., Syvälahti, E. K. G., et al. (1998). The A1 allele of the human D2 dopamine receptor gene predicts low D2 receptor availability in healthy volunteers. Mol. Psychiatry 3, 256–260. doi: 10.1038/sj.mp.4000350
R Core Team. (2021). R: a language and environment for statistical computing. Available at: https://www.R-project.org/.
Renier, T. J., Yeum, D., Emond, J. A., Lansigan, R. K., Ballarino, G. A., Carlson, D. D., et al. (2023). Elucidating pathways to pediatric obesity: a study evaluating obesity polygenic risk scores related to appetitive traits in children. Int. J. Obes. 48, 71–77. doi: 10.1038/S41366-023-01385-3
Ritchie, T., and Noble, E. P. (2003). Association of seven polymorphisms of the D2 dopamine receptor gene with brain receptor-binding characteristics. Neurochem. Res. 28, 73–82. doi: 10.1023/A:1021648128758
Shannon, H., Bush, K., Villeneuve, P. J., Hellemans, K. G. C., and Guimond, S. (2022). Problematic social media use in adolescents and young adults: systematic review and meta-analysis. JMIR Ment. Health 9:e33450. doi: 10.2196/33450
Sherman, L. E., Hernandez, L. M., Greenfield, P. M., and Dapretto, M. (2018). What the brain “likes”: neural correlates of providing feedback on social media. Soc. Cogn. Affect. Neurosci. 13, 699–707. doi: 10.1093/scan/nsy051
Sherman, L. E., Payton, A. A., Hernandez, L. M., Greenfield, P. M., and Dapretto, M. (2016). The power of the like in adolescence: effects of peer influence on neural and behavioral responses to social media. Psychol. Sci. 27, 1027–1035. doi: 10.1177/0956797616645673
SLC6A4 (n.d.). SLC6A4 solute carrier family 6 member 4 [Homo sapiens (human)] – gene – NCBI. Available at: https://www.ncbi.nlm.nih.gov/gene/6532 (Accessed December 10, 2024).
Smith, L., Watson, M., Gates, S., Ball, D., and Foxcroft, D. (2008). Meta-analysis of the association of the Taq1A polymorphism with the risk of alcohol dependency: a HuGE gene-disease association review. Am. J. Epidemiol. 167, 125–138. doi: 10.1093/aje/kwm281
Sohn, S., Rees, P., Wildridge, B., Kalk, N. J., and Carter, B. (2019). Prevalence of problematic smartphone usage and associated mental health outcomes amongst children and young people: a systematic review, meta-analysis and GRADE of the evidence. BMC Psychiatry 19:356. doi: 10.1186/s12888-019-2350-x
Soror, A. A., Hammer, B. I., Steelman, Z. R., Davis, F. D., and Limayem, M. M. (2015). Good habits gone bad: explaining negative consequences associated with the use of mobile phones from a dual-systems perspective. Inf. Syst. J. 25, 403–427. doi: 10.1111/isj.12065
Stevens, M. C. (2016). The contributions of resting state and task-based functional connectivity studies to our understanding of adolescent brain network maturation. Neurosci. Biobehav. Rev. 70, 13–32. doi: 10.1016/j.neubiorev.2016.07.027
Strang, N. M., Chein, J. M., and Steinberg, L. (2013). The value of the dual systems model of adolescent risk-taking. Front. Hum. Neurosci. 7:223. doi: 10.3389/fnhum.2013.00223
Sun, X., Luquet, S., and Small, D. M. (2017). DRD2: bridging the genome and ingestive behavior. Trends Cogn. Sci. 21, 372–384. doi: 10.1016/j.tics.2017.03.004
Tereshchenko, S. Y. (2023). Neurobiological risk factors for problematic social media use as a specific form of Internet addiction: a narrative review. World J. Psychiatry 13, 160–173. doi: 10.5498/WJP.V13.I5.160
The Common Sense Census: Media Use by Tweens and Teens. (2021). Common sense media. Available at: https://www.commonsensemedia.org/research/the-common-sense-census-media-use-by-tweens-and-teens-2021 (Accessed June 6, 2022).
The Perfect Slot Machine. (2022). The perfect slot machine’: TikTok’s most addictive design features are being cloned across the internet — the information. Available at: https://www.theinformation.com/articles/the-perfect-slot-machine-tiktoks-most-addictive-design-features-are-being-cloned-across-the-internet (Accessed September 9, 2022).
Wang, M., Ma, Y., Yuan, W., Su, K., and Li, M. D. (2016). Meta-analysis of the COMT Val158Met polymorphism in major depressive disorder: effect of ethnicity. J. Neuroimmune Pharmacol. 11, 434–445. doi: 10.1007/s11481-016-9651-3
Wray, N. R., James, M. R., Gordon, S. D., Dumenil, T., Ryan, L., Coventry, W. L., et al. (2009). Accurate, large-scale genotyping of 5HTTLPR and flanking single nucleotide polymorphisms in an association study of depression, anxiety, and personality measures. Biol. Psychiatry 66, 468–476. doi: 10.1016/j.biopsych.2009.04.030
Keywords: problematic media use, Taq1A, screen time, children, addiction
Citation: Emond JA, Renier TJ, Yeum D, Carlson DD, Ballarino GA and Gilbert-Diamond D (2025) Association between the Taq1A polymorphism and problematic media use in preadolescent children. Front. Psychol. 15:1395957. doi: 10.3389/fpsyg.2024.1395957
Received: 30 July 2024; Accepted: 24 December 2024;
Published: 08 January 2025.
Edited by:
Alessandra Maria Passarotti, University of Illinois Chicago, United StatesReviewed by:
MoonSoo Lee, Korea University, Republic of KoreaCopyright © 2025 Emond, Renier, Yeum, Carlson, Ballarino and Gilbert-Diamond. This is an open-access article distributed under the terms of the Creative Commons Attribution License (CC BY). The use, distribution or reproduction in other forums is permitted, provided the original author(s) and the copyright owner(s) are credited and that the original publication in this journal is cited, in accordance with accepted academic practice. No use, distribution or reproduction is permitted which does not comply with these terms.
*Correspondence: Jennifer A. Emond, SmVubmlmZXIuQS5FbW9uZEBEYXJ0bW91dGguZWR1
Disclaimer: All claims expressed in this article are solely those of the authors and do not necessarily represent those of their affiliated organizations, or those of the publisher, the editors and the reviewers. Any product that may be evaluated in this article or claim that may be made by its manufacturer is not guaranteed or endorsed by the publisher.
Research integrity at Frontiers
Learn more about the work of our research integrity team to safeguard the quality of each article we publish.