- 1Laureate Institute for Brain Research, Tulsa, OK, United States
- 2Department of Community Medicine, University of Tulsa, Tulsa, OK, United States
- 3Women in Recovery, Family and Children’s Services, Tulsa, OK, United States
Introduction: In the US, women are one of the fastest-growing segments of the prison population and more than a quarter of women in state prison are incarcerated for drug offenses. Substance use criminal diversion programs can be effective. It may be beneficial to identify individuals who are most likely to complete the program versus terminate early as this can provide information regarding who may need additional or unique programming to improve the likelihood of successful program completion. Prior research investigating prediction of success in these programs has primarily focused on demographic factors in male samples.
Methods: The current study used machine learning (ML) to examine other non-demographic factors related to the likelihood of completing a substance use criminal diversion program for women. A total of 179 women who were enrolled in a criminal diversion program consented and completed neuropsychological, self-report symptom measures, criminal history and demographic surveys at baseline. Model one entered 145 variables into a machine learning (ML) ensemble model, using repeated, nested cross-validation, predicting subsequent graduation versus termination from the program. An identical ML analysis was conducted for model two, in which 34 variables were entered, including the Women’s Risk/Needs Assessment (WRNA).
Results: ML models were unable to predict graduation at an individual level better than chance (AUC = 0.59 [SE = 0.08] and 0.54 [SE = 0.13]). Post-hoc analyses indicated measures of impulsivity, trauma history, interoceptive awareness, employment/financial risk, housing safety, antisocial friends, anger/hostility, and WRNA total score and risk scores exhibited medium to large effect sizes in predicting treatment completion (p < 0.05; ds = 0.29 to 0.81).
Discussion: Results point towards the complexity involved in attempting to predict treatment completion at the individual level but also provide potential targets to inform future research aiming to reduce recidivism.
1 Introduction
More than 1.2 million people were incarcerated in US state or federal prisons in 2022 (Carson, 2023). The percentage of women in the prison system has grown from 4% of the population in the 1980s to 7% in 2022 (Carson, 2023). In 2022, 64.6% of women in federal prison were serving time for drug offenses compared to only 44.6% of men (Carson, 2023). Further, while nearly half of US prisoners met criteria for a substance use disorder (SUD), only 33 to 46% of prisoners received treatment (Maruschak et al., 2021). Individuals with SUD are more likely to reoffend and have multiple incarcerations, indicating that SUD treatment is critical for reducing recidivism (Baillargeon et al., 2010; Zgoba et al., 2020). Additionally, non-completion of diversion programs has been linked to higher reoffending rates (Ozturk et al., 2022). Conversely, completion of diversion programs has been linked not only with reduced likelihood of returning to prison (Clifasefi et al., 2017; Bernard et al., 2020; Mueller-Smith and Schnepel, 2021; Ozturk et al., 2022), but also reduced likelihood of substance use overdose (Bernard et al., 2020), reduced cost to state and local governments (Anglin et al., 2013), improved likelihood of being employed (Clifasefi et al., 2017; Mueller-Smith and Schnepel, 2021) and access to stable housed (Clifasefi et al., 2017) with effects lasting up to 20 years (Mueller-Smith and Schnepel, 2021).
In response to overall increases in drug-related incarcerations since the “War on Drugs” in the 1980s (Drug Abuse Office and Treatment Act of 1972, 1972; Bureau of Justice Statistics, 1995; Harvey et al., 2007; Carson, 2023), there are calls for improved access to SUD treatment and alternative programs to divert drug offenders from long-term incarceration (National Institute on Drug Abuse, NIOH, U.S. Department of Health and Human, and Services, 2020; Caulkins et al., 2021). SUD diversion programs often offer reduced or commuted sentences for the completion of a specific program or set of requirements (usually involving SUD treatment) set out for the offender. Outcomes for such programs have been the focus of several review papers, with the focus often being on rate of successful program completion (Hartford et al., 2006; Harvey et al., 2007; Loveland and Boyle, 2007; Sirotich, 2009; Brown R. T., 2010; Brorson et al., 2013; Shapiro et al., 2014; Bernard et al., 2020; Lindquist-Grantz et al., 2021). Rates of completion vary between studies, but reviews suggest dropout rates may be slightly higher for diversion programs at 40–50% (Harvey et al., 2007; Brown R., 2010; Shaffer, 2011) compared to an average of 30.4% across SUD treatment programs types (Lappan et al., 2020).
There is a growing literature examining predictors of successful SUD diversion program completion and recidivism. This literature commonly concentrates on demographic factors including gender (Gray and Saum, 2005; Zettler, 2019; Gallagher et al., 2020), race (Hartley and Phillips, 2001; Gray and Saum, 2005; Ho et al., 2018; Zettler, 2019), and age (Ho et al., 2018; Shannon et al., 2018; Zettler, 2019); and socioeconomic factors such as educational attainment (Hartley and Phillips, 2001; Butzin et al., 2002; Dannerbeck et al., 2006; Ho et al., 2018), employment (Hartley and Phillips, 2001; Butzin et al., 2002; Dannerbeck et al., 2006; Gallagher et al., 2015; Gill, 2016; Ho et al., 2018; Shannon et al., 2018; Zettler, 2019; Gallagher et al., 2020), marital status and social relationships (Dannerbeck et al., 2006; DeVall and Lanier, 2012; Ho et al., 2018), and criminal history (DeVall and Lanier, 2012; Ho et al., 2018; Shannon et al., 2018; Gallagher et al., 2020). These studies suggest that a younger age at time of matriculation, greater extent of criminal history, minority status, less than high school education, and unemployment, may relate to lower likelihood of SUD diversion program completion. However, there are numerous inconsistencies across studies, which may be due to variations in treatment programs and populations – but also likely speaks to the difficulty of predicting mental health and substance use related outcomes including treatment completion (Gowin et al., 2019; Symons et al., 2019).
Research focused on demographic factors predicting treatment outcome is valuable in helping to understand which individuals need additional or augmented treatment. However, there have been calls to shift focus to more malleable predictors and to better understand under what conditions dropout occurs, to help inform what to target with treatment modifications or augmentations (Zgoba et al., 2020). The current study extends this call to criminal diversion programs in an attempt to understand additional factors related to dropout and increase retention in these programs.
Research focusing on how comorbid mental health and psychological variables relate to program graduation may be particularly useful for identifying SUD treatment augmentation strategies. For instance, comorbid depression symptoms and/or diagnosis at matriculation has been linked to higher rates of attrition from SUD treatment (Curran et al., 2002; Gray and Saum, 2005; Evans et al., 2009; Hickert et al., 2009). Hickert et al. (2009) found that depression in the 30 days prior to treatment more than doubled the likelihood of treatment termination. Evidence for relationships between other comorbid conditions (e.g., anxiety, posttraumatic stress disorder, psychosis) is less clear, but generally supports increased dropout for individuals with dual diagnoses (Levin et al., 2004; Gray and Saum, 2005; Lejuez et al., 2008; Evans et al., 2009; Szafranski et al., 2017; Zettler, 2018). However, it is important to note that some studies suggest comorbid mental illness is not associated with termination from diversion or treatment programs (Brown R., 2010; Gallagher et al., 2015) or may increase retention (Amaro et al., 2007; Hesse, 2009; López-Goñi et al., 2021), which also may be due to variations in study populations and treatment programs. For full systematic reviews highlighting some of these inconsistencies see Brorson et al. (2013) and Lappan et al. (2020). Dimensional assessments of behavior or personality have also been explored in relation to treatment completion. Specifically, impulsivity, hostility, aggression, and sensation seeking have been identified as potential predictors of treatment dropout in substance use programs (Loree et al., 2015; Hershberger et al., 2017; Choate et al., 2021). However, one limitation of this previous literature is that it has focused on predominantly male samples. Choate et al. (2021) examined whether gender moderated the relationship between personality traits and treatment termination. They found gender moderated this relationship such that some personality traits (e.g., grandiosity and hostility) related to treatment termination only among men, whereas other traits (e.g., submissiveness) predicted outcomes for women. Further highlighting the need for representation of women in SUD literature is the suggestion that women may have specific needs and barriers to treatment, including lack of services for pregnant women and/or affordable childcare, fear of losing custody, greater transportation concerns, economic concerns, higher rates of comorbid psychiatric conditions, increased likelihood of trauma, greater social stigma and discrimination, and less social support (Greenfield et al., 2007; Tuchman, 2010). While there has been prior work examining gender as a potential predictor of outcome for substance use or criminal diversion programs, further work is warranted to examine factors predicting treatment outcomes and treatment completion for women specifically.
The aim of the current study was to use machine learning (ML) techniques to examine predictors of graduation from a criminal diversion program for women facing SUD related prison sentences. ML is well-suited to this aim because it has the capability to describe complex statistical relationships among high-dimensional data. In such cases, ML has the potential to make better predictions than traditional modeling techniques, improving medical prognoses and diagnostic accuracy (Obermeyer and Emanuel, 2016). The use of ML methods is growing in the SUD literature as a way to examine treatment predictors (Barenholtz et al., 2020); however, it has been less commonly used to examine diversion program completion (Delen et al., 2021). The current study included demographic variables as well as a large battery of psychological variables assessing domains of mental health symptoms, sleep, physical activity, personality, and cognitive functioning. Ultimately, understanding characteristics related to graduation for women enrolled in an SUD criminal diversion program has the potential for informing modification of interventions to increase the likelihood of program completion.
2 Method
2.1 Participants
Participants were enrolled between November 2015 and October 2018. Rate of study enrollment was influenced by the rate of WIR enrollment combined with the number of women who met inclusion/exclusion criteria for the study. Approximately 315 women enrolled into WIR during the 3 years of study recruitment (approximately 105 per year) of these 304 were screened for the current study and 125 were excluded. The study protocol was designed to be very similar to the Tulsa 1,000 study (Victor et al., 2018) as such inclusion and exclusion criteria were the same with the exception that these participants had to be enrolled in WIR. For full inclusion and exclusion criteria (see Victor et al., 2018). Participants were primarily excluded for psychosis, OCD, or bipolar disorders (n = 47). Followed by untreated or complicating medical conditions (e.g., seizure disorders, untreated cancers, history of significant traumatic brain injury, and factors that would impede neuroimaging including high body mass factors that would impede neuroimaging including high body mass; n = 43). Additional participants were excluded for pregnancy (n = 6), low inclusion scores (n = 12), and age (n = 1). Reason for exclusion was not listed for 16 participants. Included participants were 179 women (ages 20–55; m = 32.8, SD = 7.21) enrolled in Women in Recovery (WIR), a court-ordered mental health diversion program for drug-related offenses. Participants were relatively diverse with a slight majority (51.7%) identifying as Non-Hispanic, White (n = 91), followed by 29.5% Native American, 8% Black, and 7.4% Hispanic. The most identified drugs of choice were stimulants (55.7%, n = 98) and opiates (22.7%, n = 40). A total of 130 women (74%) graduated from the program (n = 46, 26% terminated). Participants who complete the program receive deferred or dismissed sentences, whereas participants who do not complete are sentenced by the Oklahoma Department of Corrections (DOC). Average time to graduation was approximately 17 months (current sample mdays = 526.39, SD = 172.44). See Table 1 for full demographic information.
The women were recruited for the current study during their first 3 months of beginning the WIR program. Baseline data collected from WIR participants has been combined with the Tulsa 1,000 study for prior cross-sectional analysis studies relating to substance use (Aupperle et al., 2020; Smith et al., 2020a,b, 2021; Stewart et al., 2020). For recruitment research staff from Laureate Institute for Brain Research (LIBR) went to the WIR site on a weekly basis. Any women who were interested in participating could come and complete screening with study staff during these times. With the exception of the Women’s Risk/Needs Assessment (WRNA; Van Voorhis et al., 2010), all measures described were completed by LIBR research staff either on-site at WIR or at LIBR, based on what was most convenient for the participant at the time of assessment. Information provided during study assessments was kept confidential within LIBR study staff, including not being shared with WIR staff or court officials. The WRNA was completed by WIR clinical staff; participants provided consent for this information to be shared with LIBR study staff for the purposes of the research study.
The study was approved by the Western Institutional Review Board. All participants provided written informed consent, in accordance with the Declaration of Helsinki. Additional precautions were taken to decrease potential coercion including individual discussions with each participant by research staff placing an emphasis on the voluntary nature of the study and that their choice to participate or not in the study would have no impact on their treatment at WIR or other correctional facilities. Further, no identifiable data was shared with WIR or the court. Women were compensated for their participation at the rate of $20 per hour via ClinCard1, a prepaid debit card used commonly used in clinical trials research.
Controlling charges were determined by the level of security the Oklahoma DOC mandates for an individual based on a conviction for the charge. If an individual had multiple charges the charge with the highest level of security was designated as the controlling charge. The most frequent controlling charge category was Property charges (38.7%, n = 67; e.g., prohibited carry of a firearm, carrying a weapon/drug/alcohol into jail). Women fell into four controlling charge categories: Property, Violent, Drug, and Driving Under the Influence (DUI). Table 2 lists the frequency of all criminal charges, wherein the most frequently occurring charges were Unlawful Possession of Paraphernalia (40.3%, n = 71), Possession of Controlled Substance (39.2%, n = 69), and Larceny of Merchandise from Retailer (23.9%, n = 42).
During their enrollment in WIR, women engaged in empirically supported treatment for SUD such as Twelve Step Programs (Kaskutas, 2009) and Seeking Safety (Najavits et al., 1998). As well as for mental health (e.g., individual therapy) including as needed access to trauma/PTSD treatment (e.g., Cognitive Processing Therapy) and pharmacological therapy. Additionally WIR takes a holistic approach to treatment and individuals typically were also enrolled in parenting classes, General Educational Development classes, and occupational assistance as indicated.
2.2 Measures
The outcome variable for both models was a binary variable indicating whether or not the participant had completed the WIR program. Within the primary analysis, predictors included 145 clinically relevant measures: demographics, Patient-Reported Outcomes Measurement Information System (PROMIS; Cella et al., 2010), neuropsychological, clinical symptomatology (e.g., positive and negative affect, mental health symptoms), interoception, and personality measures, as well as information collected about criminal charges. For full list of variables see Table 3. The psychological measures were selected to focus on positive and negative valence, cognition, and interoception domains hypothesized to be important transdiagnostic factors important for understanding SUD and other mental health related outcomes. Two indices of criminal behavior/history were included in the analyses: prior probation and 85% crime. Prior probation was entered as a binary variable indicating whether or not the individual had served probation time for their current offense prior to entering the WIR program. Oklahoma Statue has a specific designation for certain criminal offenses that due to the nature of the offense a person shall not serve less than 85% of their imposed sentence. This was coded as a binary variable (Table 2).
A secondary analysis was conducted to examine variables from the Women’s Risk/Needs Assessment (WRNA; Van Voorhis et al., 2010). The WRNA is the only validated assessment aimed to determine gender specific risk and needs of women in the criminal justice system (see Table 4 for subscales). The WRNA is completed by WIR staff as part of the clinical intake assessment and the women enrolled in the current study consented to have this intake information shared as part of the research study.
2.3 Statistical analyses
For the primary analysis, a ML model was used to explore the contribution of 145 unique variables in prediction of graduation status. The R package caretStack (Forthman and Yeh, 2021) was used to train a ML ensemble method which combines the predictions of several ML models for a ‘wisdom of the crowds’ approach (Marbach et al., 2012). We applied three prediction models in this method in order to capture diverse linear and non-linear relationships using methods that are robust to multicollinearity and overfitting: support vector machines (Suykens and Vandewalle, 1999), elastic net (Friedman et al., 2021), and randomForest (Bergstra and Bengio, 2012). Repeated, nested cross-validation (rNCV) has been shown to produce unbiased performance estimates regardless of sample size, making it appropriate for use in the current sample (particularly given that sample size exceeded the number of variables in the model; Vabalas et al., 2019). Optimal hyper-parameter values were chosen using random search (Bergstra and Bengio, 2012), and the one standard error rule (Hastie et al., 2009) using area under the curve (AUC) as the model performance metric. Performance was summarized as the AUC mean and 95% confidence interval. Each prediction model had a different measure provided for variable importance (VI): a “filter” approach (Kuhn, 2021) was used for Support Vector Regression (SVR), absolute values of regression coefficients for elastic net, and an “out-of-bag” mean square error obtained by permutation for randomForest. The VI measures were then scaled between 0 and 100 and overall importance was calculated as the average VI weighted by the performance metric associated with the prediction model. Post-hoc exploratory logistic regressions were conducted between each predictor and graduation status using the glm function in R. Confidence intervals were calculated as two standard errors on the link scale and then the inverse of the link function was applied to map the values back to the response scale. A false discovery rate (FDR) correction was used to correct for multiple comparisons.
For secondary analysis, identical ML and exploratory logistic regressions were conducted but with 34 unique variables (30 subscales from the WRNA, as well as age, race, and substance use variables). The decision was made to not include the WRNA in the primary analysis as the WRNA was only available for a subset of participants (n = 125). This was due to the administration of the WRNA. A subsect of the WRNA assessments conducted via paper-and-pencil interviews. Electronic data entry was given priority to WIR graduates which led to a higher graduation rate for this sample.
3 Results
3.1 Analysis sample
Of the 179 participants recruited for the study, three were excluded for missing the outcome variable (n = 2) or having greater than 30% missing data (n = 1) for a final analysis sample of n = 176 included in the main analysis. Of the 125 participants with data available on the WRNA, one was excluded from the analysis due to missing the outcome variable, leaving a final sample for the WRNA analysis of n = 124. The WRNA analysis sample had a higher graduation rate than for women who WRNA data was not available, but there were no other identified demographic differences for those with and without WRNA data available (see Table 5).
All variables of interest had missingness less than 8%. With the exception of age of primary use, which was missing for 31.8% of participants in the full sample.
3.2 Machine learning
The stacked model for the primary analysis was conducted examining multidimensional measures of neuropsychological, clinical symptom, interoception, and personality measures (AUC = 0.49; SE = 0.08). This was not significantly different from predicting the base rate of completion (see Supplementary Figure S1). Of the variables with the highest variable importance, four were related to trauma (i.e., Childhood Trauma Questionnaire Denial, Emotional Abuse, Emotional Neglect, and Total Score), two were related to impulsivity (UPPS-P Impulsive Behavior Scale Lack of Premeditation and Positive Urgency), and two were related to interoception (Multidimensional Assessment of Interoceptive Awareness Self-Regulation and Not Distracting; see Figure 1). Trauma, self-regulation, and age were positively related to graduation, whereas impulsivity and denial of trauma were negatively related to graduation.
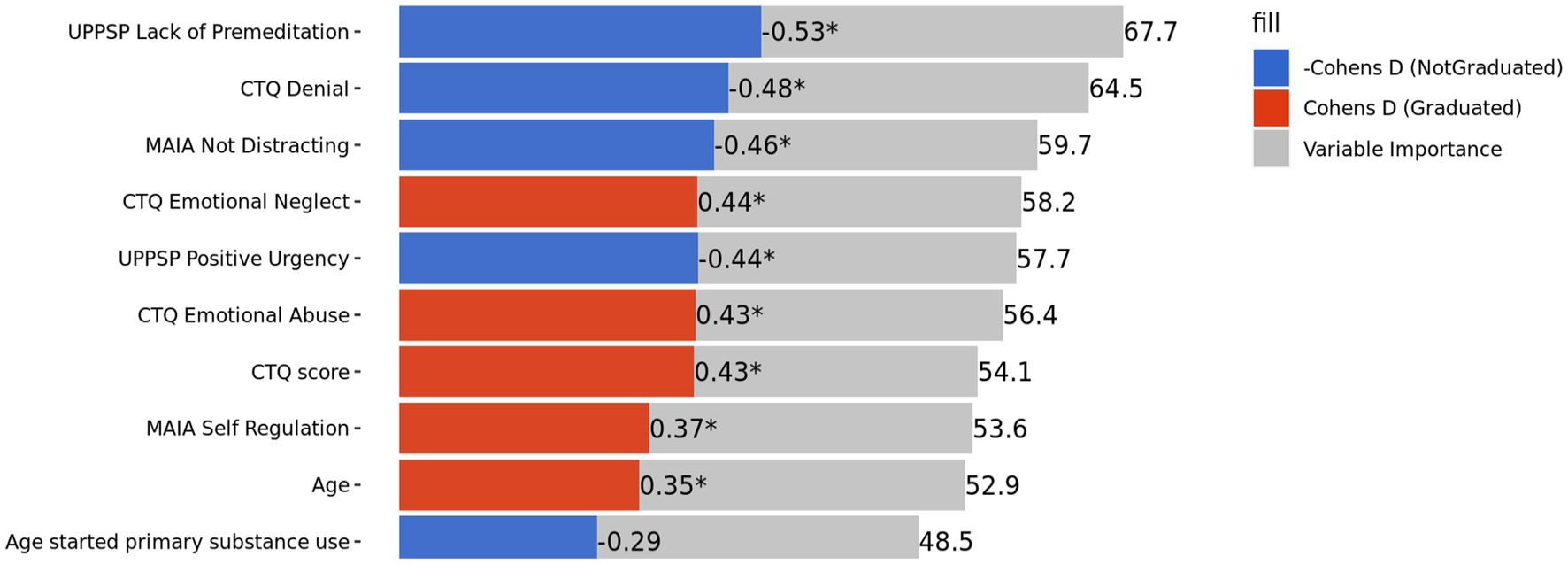
Figure 1. Top 10 variable importance from machine learning model one. “*” Indicates p-value <0.05. UPPSP, urgency, premeditation, perseverance, sensation seeking, positive urgency, impulsive behavior scale; CTQ, childhood trauma questionnaire; MAIA, multidimensional assessment of interoceptive awareness.
When conducting the secondary analysis examining graduation prediction using variables from the WRNA, the model once again was not an improvement on predicting the base rate of completion (see Supplementary Figure S2). The AUC of the stacked model was 0.54 (SE = 0.13). Variable Importance was highest for the Employment/Financial score, Housing Safety score, Antisocial Friends score, age of first use of primary substance, Total Risk Needs score, Total score on the WRNA, Anger/Hostility score, Child Abuse score, Self-Efficacy score, and age (see Figure 2). Overall, the top 7 predictors were negatively related to graduation; however, Child Abuse score and Self-Efficacy were positively related to graduation.
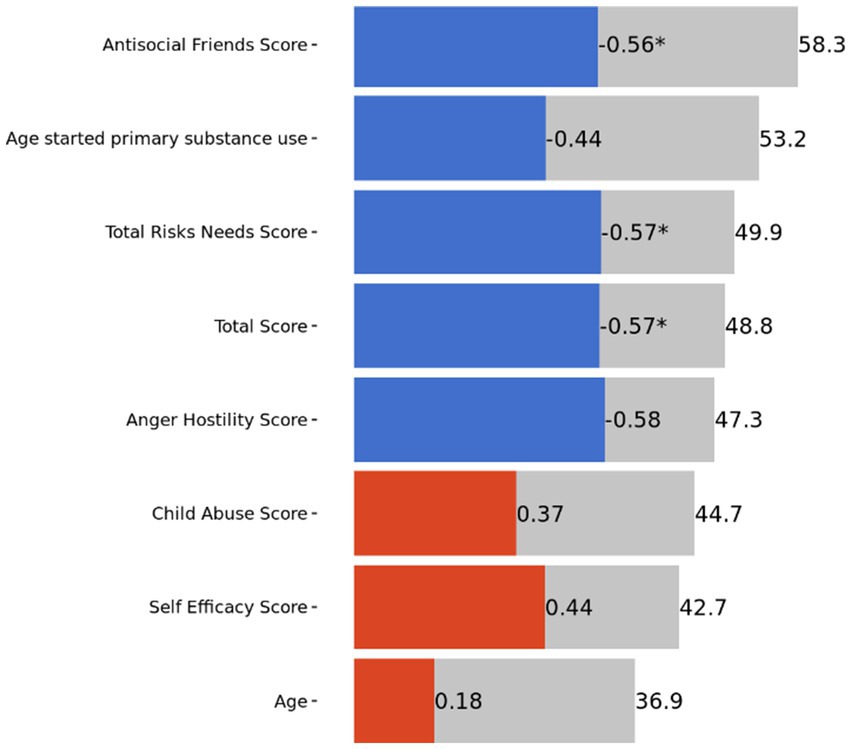
Figure 2. Top 10 variable importance from machine learning model two. “*” Indicates p-value <0.05. “***” Indicates significance after Bonferroni correction. Scores were derived from the Women’s Risk/Needs Assessment (WRNA).
3.3 Post-hoc analyses
Since ML models were unable to predict graduation better than chance, exploratory logistic regressions were conducted. UPPS-P Lack of Premeditation (d = −0.53), CTQ Denial (d = −0.48) and MAIA Not Distracting (d = 0.46) predicted graduation with medium effect sizes (p < 0.05; not significant after FDR correction). See Figure 3.
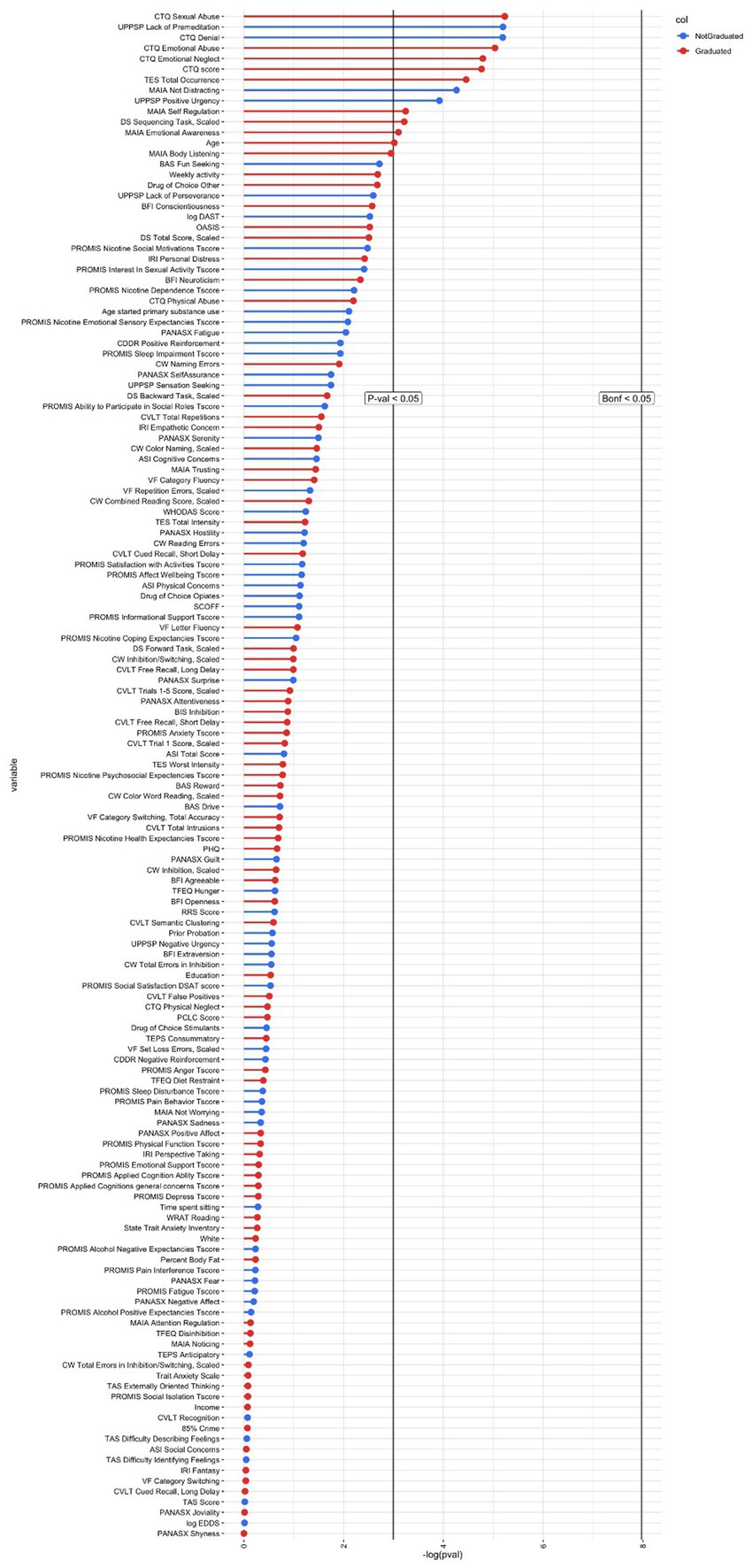
Figure 3. Logistic regressions for variables included in model one. Bonf <0.05 is the threshold for p-value significance after Bonferroni correction; red color = negatively related to graduation; blue color = positively related to graduation.
The secondary logistic regression models examined the contribution of WRNA variables in predicting graduation status. Employment/ Financial Risk was the only variable that was significant after FDR correction and was negatively related to program completion with a large effect size (Cohen’s d = −0.81). Five additional variables had medium to large effect sizes (and were significant at p < 0.05) including Housing Safety score (Cohen’s d = −0.68), Antisocial Friends score (Cohen’s d = −0.56), Anger/Hostility score (Cohen’s d = −0.58), Total Risks Needs score (Cohen’s d = −0.57), and Total WRNA score (Cohen’s d = −0.57). See Figure 4.
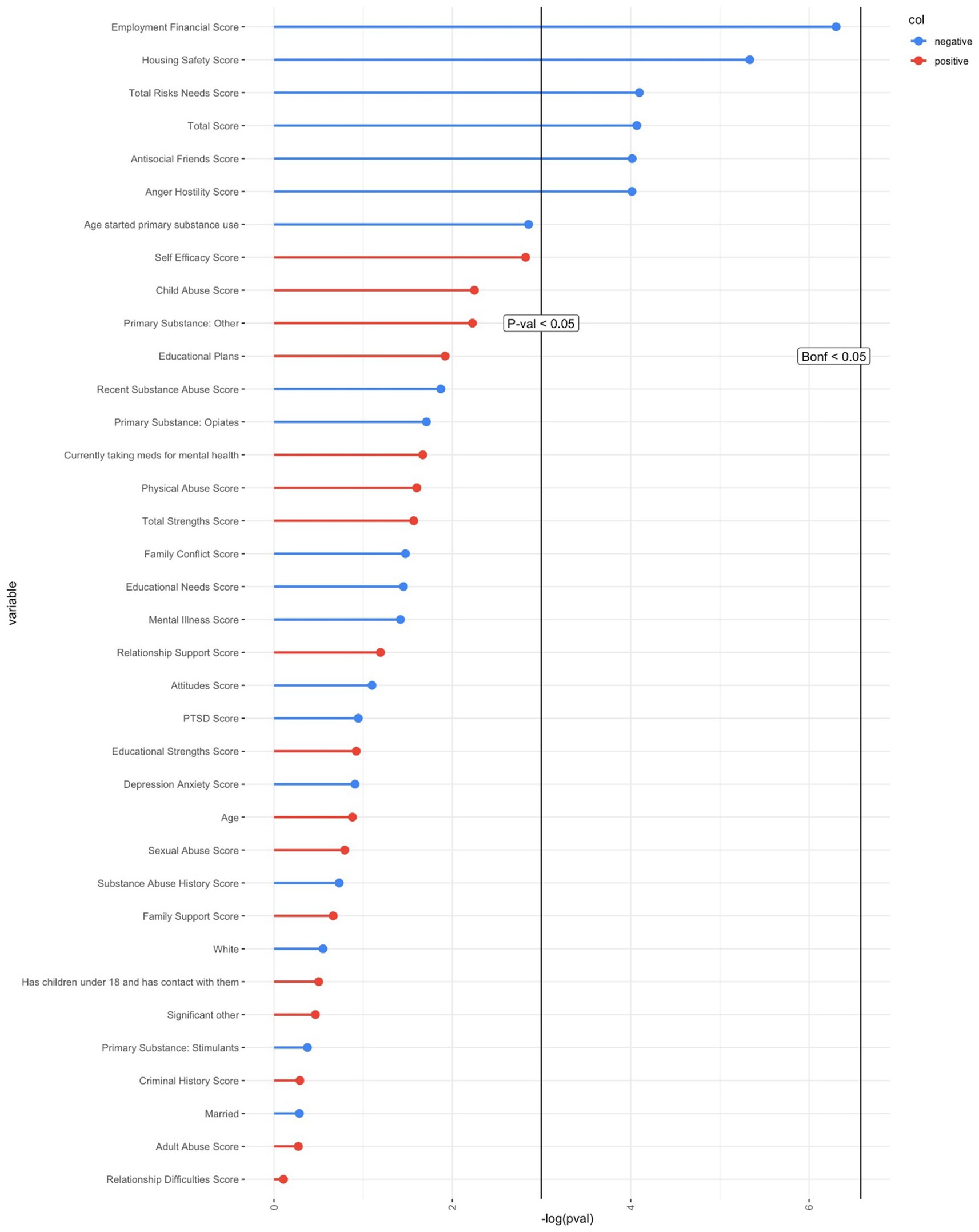
Figure 4. Logistic regressions for variables included in model two. Bonf <0.05 = p value corrected using Bonferroni; red color = positively related to graduation; blue color = negatively related to graduation.
4 Discussion
The goal of the current investigation was to use data driven techniques to examine predictors of graduation for women participating in an SUD diversion program. Previous work dedicated to identifying predictors of treatment completion often emphasizes the relation between demographic and socioeconomic variables and graduation. This study extends this literature through the inclusion of these variables as well as a large battery of psychological variables assessing domains of mental health symptoms sleep, physical activity, personality, and cognitive functioning. Further, the current study explored graduation in women, a population historically underrepresented in SUD literature. The larger goal was to understand characteristics associated with program completion, with the aspiration to identify strategies for possible intervention or modification of such programs. Unfortunately, even with 147 variables, the ML models were unable to provide individual-level prediction of graduation better than the base rate. However, several variables were identified as being related to graduation with medium to large effect sizes including employment/financial risk, housing safety, anger/hostility, antisocial friends, impulsivity, trauma history, and interoceptive awareness. Many of these identified variables could be potentially targeted in SUD treatment and diversion programs to help improve completion rates.
At the forefront of this discussion, it is important to recognize that the use of ML or other predictive modeling has the potential to perpetuate bias against minoritized populations by relying on factors such as socioeconomic background to make decisions about sentencing (e.g., those sentenced to prison versus criminal diversion programs; Klingele, 2015; DiBenedetto, 2019). We suggest that the identification of such predictors is most beneficial if used to identify potential avenues to enhance treatment retention or success for all individuals enrolled in diversion programs, rather than to identify which individuals to enroll in these programs. ML has been heralded as an approach that may be able to predict at the individual-level mental and substance use related outcomes including treatment completion (Acion et al., 2017; Mak et al., 2019); however, this study and others demonstrate the difficulty of predicting outcomes, even when there is a breadth of information available to include in the models (Gowin et al., 2019; Symons et al., 2019). This may suggest more fine-grained temporal data, such as ecological momentary assessment (Shiffman, 2009), is needed to better predict program completion at the individual level. While our current ML models failed to predict graduation better than chance, the variable importance and logistic regression analyses identified several variables that could potentially be targeted to increase completion rates and may be important to explore in future research. Thus, while the goal of being able to indicate the probability of success of program graduation for any one individual entering treatment remains elusive, the identification of cognitive or behavioral factors relating to treatment completion remain important for informing potential targets for treatment augmentation at the group level (Czajkowski et al., 2015).
In prior work, insight and recognition of one’s problems have been related to increased motivation for treatment, treatment attendance, and abstinence from substances (Raftery et al., 2020). Similarly, in the current study, the CTQ Denial subscale was related negatively to graduation, which may suggest that women who have more insight into their prior experiences of trauma or who are more willing to report trauma, may engage more meaningfully in treatment. Additionally, trauma-informed substance use programs or programs that treat SUD and trauma concurrently have a lower rate of treatment dropout compared to treatment as usual (Amaro et al., 2007; López-Goñi et al., 2021). The current study found trauma to be positively related to graduation, which is seemingly in contradiction to previous studies indicating trauma or trauma-related symptoms relate to worse treatment outcomes (Hyman et al., 2008; Szafranski et al., 2017). This may be related to fact that those who are high in denial are also going to report less trauma experiences and thus, reduced trauma history may instead reflect reduced awareness or willingness to report. However, this finding may also be due to the uniqueness of the current sample. First, the current study examined only women and previous studies recruited majority male samples. Secondly, the women in the current study were recruited from a trauma-informed diversion program. This may have impacted treatment seeking behaviors such that those with significant trauma history may have been more likely to seek out PTSD treatment during WIR. Taken together, findings suggest that insight into one’s mental health concerns and trauma-related care may be important to consider in reducing attrition for women-focused substance use and criminal diversion treatment programs.
The current study also found that UPPS-P Lack of Premeditation was negatively associated with program completion. The UPPS-P Lack of Premeditation subscale assesses one’s tendency to act impulsively without consideration of consequences. A recent meta-analysis found pre-treatment Lack of Premeditation to be a robust predictor of poor therapy outcomes in SUD (Hershberger et al., 2017). Further, this meta-analysis examined pre to post treatment changes in impulsivity and reported a lack of significant change for Lack of Premeditation, Positive Urgency, and Lack of Perseverance and only small effect sizes for the changes in Negative Urgency and Sensation Seeking (Hershberger et al., 2017). Together, these findings suggest that impulsivity is related to treatment completion and that standard substance use treatments may not effectively target impulsivity, indicating potential benefit in identifying strategies that may do so. Various novel treatment augmentation approaches, such as cognitive remediation (Anderson et al., 2021; Nardo et al., 2021) and episodic future thinking (Aonso-Diego et al., 2021; Forster et al., 2021) have shown promise in reducing impulsivity in substance use or other populations. Future research and funding are warranted to identify whether impulsivity interventions in SUD or diversion programs may show promise for better retention and treatment outcomes.
Interoceptive awareness was another psychological construct identified as related to WIR program completion. Increasingly, research has shown a connection between diminished interoceptive awareness and substance use disorder (Smith et al., 2020a; Stewart et al., 2020). The current study suggested that not distracting oneself from bodily sensations was related negatively to graduation with a medium effect size, specifically that more distracting increases likelihood of graduating, and interoceptive self-regulation also had a small positive effect size with respect to graduation (Cohen’s d = 0.37). Thus, greater awareness of and attempts to regulate interoceptive sensations (via distraction or self-regulation) may be positive indicators for SUD or criminal diversion program completion. Distraction can be both adaptive and maladaptive depending on the context (Wolgast and Lundh, 2017; Waugh et al., 2020), specifically distraction for avoidance is often considered maladaptive; however, distraction for emotion regulation can be adaptive and is considered a useful distress tolerance technique to regulate intense emotions (e.g., as used in dialectical behavioral therapy; Linehan, 2014). It may be that for individuals recovering from SUD, distraction from internal cues, such as cravings, is an adaptive strategy. Treatment strategies that may hold promise for targeting interoceptive awareness and regulation include general distress tolerance and emotion regulation strategies (Linehan, 2014) as well as mind–body interventions, such as mindfulness (Black and Amaro, 2019), yoga (Petker et al., 2021), or Mindful Awareness in Body-Oriented Therapy (Price and Hooven, 2018; Price et al., 2019a,b).
Additional factors which may be useful to address in treatment are employment/finance and housing safety risk factors, which were negatively associated with program completion. While this is the first study to examine the WRNA as it relates to a diversion program for substance-related offences, previous studies have found WRNA scales to be significantly, positively related to subsequent arrests, convictions, and failure to complete probation (Van Voorhis et al., 2010). Additionally, previous studies have found employment to be significantly related to diversion program completion (Dannerbeck et al., 2006; Ho et al., 2018; Shannon et al., 2018). These findings highlight the need to address residential and occupational concerns early in treatment, as doing so may lead to higher rates of program completion.
4.1 Limitations
The WIR program is unique in regards to the length of the program and the focus on comprehensive treatment services targeting not only SUD and criminal behavior, but also mental and physical health, and occupational and educational needs. As such, the completion rate of the WIR program (~75%) exceeds that of most SUD or diversion programs in the community and in prior research (Harvey et al., 2007). Thus, generalizability of current findings to other treatment programs is uncertain. It is possible that the high completion rate is due to factors related to selection for the WIR program. Admittance into the WIR program is an extensive process which includes an admissions panel of the WIR clinical directors and must be agreed upon by the district attorney. This admissions panel considers things such as the charges and safety concerns to both the public and treatment milieu. Additionally, participants must be female presenting, have not engaged in this program previously, no psychotic spectrum diagnosis, and at the time of recruitment have charges in Tulsa County. This screening process likely impacted results and may have reduced generalizability. Further, there was a differential rate of graduation in the sample of participants for which the WRNA was available due to preferential data entry for those who graduated. This likely skewed the analyses and could explain some of the null results. Therefore, the results should be interpreted accordingly and replicated in future work. In addition, there may have been factors influencing which women were willing to enroll and met criteria for the research study, which may also limit generalizability. It would be ideal if future studies are conducted using combined data from populations across a variety of diversion programs and numerous sites across the United States. One strength of the current study is the use of longitudinal measurement to predict program completion. Future studies may benefit from assessing changes in some of the domains identified as related to treatment outcome (e.g., impulsivity) to ascertain whether treatment is effectively impacting functioning in these areas. Finally, the study enrollment period was over a 3 year period. While the core content and primary treatments involved in WIR remained similar across the enrollment period, the specific treatment content could have differed across time and participant/provider, given the individualized nature of the WIR program content. These differences could have influenced which measures were identified as predictors of treatment completion.
5 Conclusion
This study used a data-driven framework to identify variables contributing to drop out from a women’s substance use criminal diversion program. While the ML model failed to predict graduation rates at the individual level a rate higher than chance, examination of VI and results from logistic regression analyses identified several factors relating to treatment completion with medium to large effect sizes – including trauma, interoceptive awareness, and impulsivity. Future studies are warranted to examine whether interventions aimed at processing trauma or improving interoceptive awareness and impulsivity are related to increased retention in substance use treatment settings.
Data availability statement
The raw data supporting the conclusions of this article will be made available by the authors, without undue reservation.
Ethics statement
The studies involving humans were approved by Western Institutional Review Board – Copernicus Group an independent review board. The studies were conducted in accordance with the local legislation and institutional requirements. The participants provided their written informed consent to participate in this study.
Author contributions
EC: Conceptualization, Visualization, Writing – original draft, Writing – review & editing, Validation. KF: Conceptualization, Data curation, Formal analysis, Visualization, Writing – review & editing. NK: Conceptualization, Writing – review & editing. JS: Conceptualization, Writing – review & editing. MC: Conceptualization, Writing – review & editing. EA: Conceptualization, Writing – review & editing. NM: Conceptualization, Writing – review & editing. MM: Conceptualization, Writing – review & editing. MT: Conceptualization, Writing – review & editing. RK: Formal analysis, Writing – review & editing, Conceptualization. MP: Funding acquisition, Methodology, Project administration, Writing – review & editing, Conceptualization. RA: Methodology, Project administration, Supervision, Writing – review & editing, Conceptualization.
Funding
The author(s) declare that financial support was received for the research, authorship, and/or publication of this article. Funding was provided by the Laureate Institute for Brain Research. The funders had no role in the study design; data collection, analysis or interpretation of the data; in the writing of this report; or in the decision to submit the article for publication. All researchers are independent from the funders and had access to all of the data.
Conflict of interest
NM, MT, and MM are employed by Family and Children’s Services, the parent organization for the Women in Recovery Program.
The remaining authors declare that the research was conducted in the absence of any commercial or financial relationships that could be construed as a potential conflict of interest.
Publisher’s note
All claims expressed in this article are solely those of the authors and do not necessarily represent those of their affiliated organizations, or those of the publisher, the editors and the reviewers. Any product that may be evaluated in this article, or claim that may be made by its manufacturer, is not guaranteed or endorsed by the publisher.
Supplementary material
The Supplementary material for this article can be found online at: https://www.frontiersin.org/articles/10.3389/fpsyg.2024.1390199/full#supplementary-material
Footnotes
References
Acion, L., Kelmansky, D., van der Laan, M., Sahker, E., Jones, D., and Arndt, S. (2017). Use of a machine learning framework to predict substance use disorder treatment success. PLoS One 12:e0175383. doi: 10.1371/journal.pone.0175383
Amaro, H., Chernoff, M., Brown, V., Arévalo, S., and Gatz, M. (2007). Does integrated trauma-informed substance abuse treatment increase treatment retention? J. Community Psychol. 35, 845–862. doi: 10.1002/jcop.20185
Anderson, A. C., Youssef, G. J., Robinson, A. H., Lubman, D. I., and Verdejo-Garcia, A. (2021). Cognitive boosting interventions for impulsivity in addiction: a systematic review and meta-analysis of cognitive training, remediation and pharmacological enhancement. Addiction 116, 3304–3319. doi: 10.1111/add.15469
Anglin, M. D., Nosyk, B., Jaffe, A., Urada, D., and Evans, E. (2013). Offender diversion into substance use disorder treatment: the economic impact of California’s proposition 36. Am. J. Public Health 103, 1096–1102. doi: 10.2105/AJPH.2012.301168
Aonso-Diego, G., González-Roz, A., Martínez-Loredo, V., Krotter, A., and Secades-Villa, R. (2021). Episodic future thinking for smoking cessation in individuals with substance use disorder: treatment feasibility and acceptability. J. Subst. Abus. Treat. 123:108259. doi: 10.1016/j.jsat.2020.108259
Aupperle, R. L., Paulus, M. P., Kuplicki, R., Touthang, J., Victor, T., Yeh, H.-W., et al. (2020). Web-based graphic representation of the life course of mental health: cross-sectional study across the Spectrum of mood, anxiety, eating, and substance use disorders. JMIR Mental Health 7, –e16919. doi: 10.2196/16919
Badu, E., Mitchell, R., O’Brien, A. P., Osei, A., and Rubin, M. (2021). Measuring disability in consumers of mental health services – psychometric properties of the World Health Organization disability assessment schedule 2.0 (WHODAS 2.0) in Ghana. Int. J. Ment. Health Nurs. 30, 1274–1288. doi: 10.1111/inm.12911
Baillargeon, J., Penn, J. V., Knight, K., Harzke, A. J., Baillargeon, G., and Becker, E. A. (2010). Risk of reincarceration among prisoners with co-occurring severe mental illness and substance use disorders. Adm. Policy Ment. Health Ment. Health Serv. Res. 37, 367–374. doi: 10.1007/s10488-009-0252-9
Barenholtz, E., Fitzgerald, N. D., and Hahn, W. E. (2020). Machine-learning approaches to substance-abuse research: emerging trends and their implications. Curr. Opin. Psychiatry 33, 334–342. doi: 10.1097/YCO.0000000000000611
Bergstra, J., and Bengio, Y. (2012). Random search for hyper-parameter optimization. J. Mach. Learn. Res. 13.
Bernard, C. L., Rao, I. J., Robison, K. K., and Brandeau, M. L. (2020). Health outcomes and cost-effectiveness of diversion programs for low-level drug offenders: a model-based analysis. PLoS Med. 17:e1003239. doi: 10.1371/journal.pmed.1003239
Bernstein, D. P., Stein, J. A., Newcomb, M. D., Walker, E., Pogge, D., Ahluvalia, T., et al. (2003). Development and validation of a brief screening version of the childhood trauma questionnaire. Child Abuse Negl. 27, 169–190. doi: 10.1016/S0145-2134(02)00541-0
Black, D. S., and Amaro, H. (2019). Moment-by-moment in Women's recovery (MMWR): mindfulness-based intervention effects on residential substance use disorder treatment retention in a randomized controlled trial. Behav. Res. Ther. 120:103437. doi: 10.1016/j.brat.2019.103437
Brorson, H. H., Ajo Arnevik, E., Rand-Hendriksen, K., and Duckert, F. (2013). Drop-out from addiction treatment: a systematic review of risk factors. Clin. Psychol. Rev. 33, 1010–1024. doi: 10.1016/j.cpr.2013.07.007
Brown, R. (2010). Associations with substance abuse treatment completion among drug court participants. Subst. Use Misuse 45, 1874–1891. doi: 10.3109/10826081003682099
Brown, R. T. (2010). Systematic review of the impact of adult drug-treatment courts. Transl. Res. 155, 263–274. doi: 10.1016/j.trsl.2010.03.001
Brown, S. A., Myers, M. G., Lippke, L., Tapert, S. F., Stewart, D. G., and Vik, P. W. (1998). Psychometric evaluation of the customary drinking and drug use record (CDDR): a measure of adolescent alcohol and drug involvement. J. Stud. Alcohol 59, 427–438. doi: 10.15288/jsa.1998.59.427
Bureau of Justice Statistics (1995). Correctional populations in the United States : Bureau of Justice Statistics. Available at: https://bjs.ojp.gov/library/publications/correctional-populations-united-states-1995.
Butzin, C. A., Saum, C. A., and Scarpitti, F. R. (2002). Factors associated with completion of a drug treatment court diversion program. Subst. Use Misuse 37, 1615–1633. doi: 10.1081/JA-120014424
Carson, E. A. (2023). Prisoners in 2022 - statistical tables : Bureau of Justice Statistics. Available at: https://bjs.ojp.gov/library/publications/prisoners-2022-statistical-tables.
Carver, C., and White, T. (1994). Behavioral inhibition, Behavioral activation, and affective responses to impending reward and punishment: the BIS/BAS scales. J. Pers. Soc. Psychol. 67, 319–333. doi: 10.1037/0022-3514.67.2.319
Caulkins, J. P., Gould, A., Pardo, B., Reuter, P., and Stein, B. D. (2021). Opioids and the criminal justice system: new challenges posed by the modern opioid epidemic. Ann. Rev. Criminol. 4, 353–375. doi: 10.1146/annurev-criminol-061020-125715
Cella, D., Riley, W., Stone, A., Rothrock, N., Reeve, B., Yount, S., et al. (2010). The patient-reported outcomes measurement information system (PROMIS) developed and tested its first wave of adult self-reported health outcome item banks: 2005–2008. J. Clin. Epidemiol. 63, 1179–1194. doi: 10.1016/j.jclinepi.2010.04.011
Choate, A. M., Gorey, C., Rappaport, L. M., Wiernik, B. M., and Bornovalova, M. A. (2021). Alternative model of personality disorders traits predict residential addictions treatment completion. Drug Alcohol Depend. 228:109011. doi: 10.1016/j.drugalcdep.2021.109011
Clifasefi, S. L., Lonczak, H. S., and Collins, S. E. (2017). Seattle’s law enforcement assisted diversion (LEAD) program: within-subjects changes on housing, employment, and income/benefits outcomes and associations with recidivism. Crime Delinq. 63, 429–445. doi: 10.1177/0011128716687550
Conybeare, D., Behar, E., Solomon, A., Newman, M. G., and Borkovec, T. D. (2012). The PTSD checklist-civilian version: reliability, validity, and factor structure in a nonclinical sample. J. Clin. Psychol. 68, 699–713. doi: 10.1002/jclp.21845
Craig, C. L., Marshall, A. L., Sjöström, M., Bauman, A. E., Booth, M. L., Ainsworth, B. E., et al. (2003). International physical activity questionnaire: 12-country reliability and validity. Med. Sci. Sports Exerc. 35, 1381–1395. doi: 10.1249/01.MSS.0000078924.61453.FB
Curran, G. M., Kirchner, J. E., Worley, M., Rookey, C., and Booth, B. M. (2002). Depressive symptomatology and early attrition from intensive outpatient substance use treatment. J. Behav. Health Serv. Res. 29, 138–143. doi: 10.1007/BF02287700
Czajkowski, S. M., Powell, L. H., Adler, N., Naar-King, S., Reynolds, K. D., Hunter, C. M., et al. (2015). From ideas to efficacy: the ORBIT model for developing behavioral treatments for chronic diseases. Health Psychol. 34, 971–982. doi: 10.1037/hea0000161
Dannerbeck, A., Harris, G., Sundet, P., and Lloyd, K. (2006). Understanding and responding to racial differences in drug court outcomes. J. Ethn. Subst. Abus. 5, 1–22. doi: 10.1300/J233v05n02_01
Davis, M. (1980). A multidimensional approach to individual differences in empathy. JSAS Catalog of Selected Documents in Psychology, 10, 85–103.
Delen, D., Zolbanin, H. M., Crosby, D., and Wright, D. (2021). To imprison or not to imprison: an analytics model for drug courts. Ann. Oper. Res. 303, 101–124. doi: 10.1007/s10479-021-03984-7
Delis, D. C., Kaplan, E., and Kramer, J. H. (2001). Delis-Kaplan executive function system. Assessment.
Delis, D. C., Kramer, J. H., Kaplan, E., and Ober, B. A. (2000). California verbal learning test. 2nd Edn. San Antonio, TX: Psychological Corporation.
DeVall, K. E., and Lanier, C. L. (2012). Successful completion: an examination of factors influencing drug court completion for White and non-White male participants. Subst. Use Misuse 47, 1106–1116. doi: 10.3109/10826084.2012.680171
DiBenedetto, R. (2019). Reducing recidivism or misclassifying offenders? How implementing risk and needs assessment in the Federal Prison System will perpetuate racial bias. J. Law Policy 27:414.
Evans, E., Li, L., and Hser, Y.-I. (2009). Client and program factors associated with dropout from court mandated drug treatment. Eval. Program Plann. 32, 204–212. doi: 10.1016/j.evalprogplan.2008.12.003
Forster, S. E., Steinhauer, S. R., Ortiz, A., and Forman, S. D. (2021). Evaluating effects of episodic future thinking on valuation of delayed reward in cocaine use disorder: a pilot study. Am. J. Drug Alcohol Abuse 47, 199–208. doi: 10.1080/00952990.2020.1865997
Forthman, K.L., and Yeh, H.W. (2021). caretStack. Available at: https://github.com/kforthman/caretStack.
Friedman, J., Hastie, T., Tibshirani, R., Narasimhan, B., Tay, K., Simon, N., et al. (2021). Package ‘glmnet’. J. Stat. Softw. 33.
Gallagher, J. R., Nordberg, A., Deranek, M. S., Ivory, E., Carlton, J., and Miller, J. W. (2015). Predicting termination from drug court and comparing recidivism patterns: treating substance use disorders in criminal justice settings. Alcohol. Treat. Q. 33, 28–43. doi: 10.1080/07347324.2015.982451
Gallagher, J. R., Wahler, E. A., and Nordberg, A. (2020). Predictors of graduation and criminal recidivism: findings from a drug court that primarily serves African Americans. J. Ethn. Cult. Divers. Soc. Work, 31, 40–50. doi: 10.1080/15313204.2020.1799472
Gard, D., Germans Gard, M., Kring, A., and John, O. (2006). Anticipatory and consummatory components of the experience of pleasure: a scale development study. J res personal. J. Res. Pers. 40, 1086–1102. doi: 10.1016/j.jrp.2005.11.001
Gill, M. E. (2016). Predictors of drug court client graduation. J. Offender Rehabil. 55, 564–588. doi: 10.1080/10509674.2016.1229710
Gowin, J. L., Ernst, M., Ball, T., May, A. C., Sloan, M. E., Tapert, S. F., et al. (2019). Using neuroimaging to predict relapse in stimulant dependence: a comparison of linear and machine learning models. NeuroImage: Clinical 21:101676. doi: 10.1016/j.nicl.2019.101676
Gray, A. R., and Saum, C. A. (2005). Mental health, gender, and drug court completion. Am. J. Crim. Justice 30, 55–69. doi: 10.1007/BF02885881
Greenfield, S. F., Brooks, A. J., Gordon, S. M., Green, C. A., Kropp, F., McHugh, R. K., et al. (2007). Substance abuse treatment entry, retention, and outcome in women: a review of the literature. Drug Alcohol Depend. 86, 1–21. doi: 10.1016/j.drugalcdep.2006.05.012
Hartford, K., Carey, R., and Mendonca, J. (2006). Pre-arrest diversion of people with mental illness: literature review and international survey. Behav. Sci. Law 24, 845–856. doi: 10.1002/bsl.738
Hartley, R. E., and Phillips, R. C. (2001). Who graduates from drug courts? Correlates of client success. Am. J. Crim. Justice 26, 107–119. doi: 10.1007/BF02886860
Harvey, E., Shakeshaft, A., Hetherington, K., Sannibale, C., and Mattick, R. P. (2007). The efficacy of diversion and aftercare strategies for adult drug-involved offenders: a summary and methodological review of the outcome literature. Drug Alcohol Rev. 26, 379–387. doi: 10.1080/09595230701373917
Hastie, T., Tibshirani, R., and Friedman, J. (2009). The elements of statistical learning. New York: Springer.
Hershberger, A. R., Um, M., and Cyders, M. A. (2017). The relationship between the UPPS-P impulsive personality traits and substance use psychotherapy outcomes: a meta-analysis. Drug Alcohol Depend. 178, 408–416. doi: 10.1016/j.drugalcdep.2017.05.032
Hesse, M. (2009). Integrated psychological treatment for substance use and co-morbid anxiety or depression vs. treatment for substance use alone. A systematic review of the published literature. BMC Psychiatry 9:6. doi: 10.1186/1471-244X-9-6
Hickert, A. O., Boyle, S. W., and Tollefson, D. R. (2009). Factors that predict drug court completion and drop out: findings from an evaluation of salt Lake County's adult felony drug court. J. Soc. Serv. Res. 35, 149–162. doi: 10.1080/01488370802678926
Ho, T., Carey, S. M., and Malsch, A. M. (2018). Racial and gender disparities in treatment courts: do they exist and is there anything we can do to change them. J. Advanc. Just. 1, 5–34.
Hyman, S. M., Paliwal, P., Chaplin, T. M., Mazure, C. M., Rounsaville, B. J., and Sinha, R. (2008). Severity of childhood trauma is predictive of cocaine relapse outcomes in women but not men. Drug Alcohol Depend. 92, 208–216. doi: 10.1016/j.drugalcdep.2007.08.006
Kaskutas, L. A. (2009). Alcoholics anonymous effectiveness: faith meets science. J. Addict. Dis. 28, 145–157. doi: 10.1080/10550880902772464
Klingele, C. (2015). The promises and perils of evidence-based corrections. Notre Dame Law Review 91:537.
Kroenke, K., Spitzer, R. L., and Williams, J. B. (2001). The PHQ-9: validity of a brief depression severity measure. J. Gen. Intern. Med. 16, 606–613. doi: 10.1046/j.1525-1497.2001.016009606.x
Lappan, S. N., Brown, A. W., and Hendricks, P. S. (2020). Dropout rates of in-person psychosocial substance use disorder treatments: a systematic review and meta-analysis. Addiction 115, 201–217. doi: 10.1111/add.14793
Lejuez, C. W., Zvolensky, M. J., Daughters, S. B., Bornovalova, M. A., Paulson, A., Tull, M. T., et al. (2008). Anxiety sensitivity: a unique predictor of dropout among inner-city heroin and crack/cocaine users in residential substance use treatment. Behav. Res. Ther. 46, 811–818. doi: 10.1016/j.brat.2008.03.010
Levin, F. R., Evans, S. M., Vosburg, S. K., Horton, T., Brooks, D., and Ng, J. (2004). Impact of attention-deficit hyperactivity disorder and other psychopathology on treatment retention among cocaine abusers in a therapeutic community. Addict. Behav. 29, 1875–1882. doi: 10.1016/j.addbeh.2004.03.041
Lindquist-Grantz, R., Mallow, P., Dean, L., Lydenberg, M., and Chubinski, J. (2021). Diversion programs for individuals who use substances: a review of the literature. J. Drug Issues 51, 483–503. doi: 10.1177/00220426211000330
López-Goñi, J. J., Haro, B., Fernández-Montalvo, J., and Arteaga, A. (2021). Impact of a trauma intervention on reducing dropout from substance use disorder treatment. Psychol. Trauma Theory Res. Pract. Policy 13, 847–855. doi: 10.1037/tra0001127
Loree, A. M., Lundahl, L. H., and Ledgerwood, D. M. (2015). Impulsivity as a predictor of treatment outcome in substance use disorders: review and synthesis. Drug Alcohol Rev. 34, 119–134. doi: 10.1111/dar.12132
Loveland, D., and Boyle, M. (2007). Intensive case management as a jail diversion program for people with a serious mental illness: a review of the literature. Int. J. Offender Ther. Comp. Criminol. 51, 130–150. doi: 10.1177/0306624X06287645
Mak, K. K., Lee, K., and Park, C. (2019). Applications of machine learning in addiction studies: a systematic review. Psychiatry Res. 275, 53–60. doi: 10.1016/j.psychres.2019.03.001
Marbach, D., Costello, J. C., Küffner, R., Vega, N. M., Prill, R. J., Camacho, D. M., et al. (2012). Wisdom of crowds for robust gene network inference. Nat. Methods 9, 796–804. doi: 10.1038/nmeth.2016
Maruschak, L.M., Bronson, J., and Alper, M. (2021). Survey of Prision inmates, 2016 alcohol and drug use and treatment reported by prisoners. Available at: https://bjs.ojp.gov/library/publications/alcohol-and-drug-use-and-treatment-reported-prisoners-survey-prison-inmates.
Mehling, W. E., Price, C., Daubenmier, J. J., Acree, M., Bartmess, E., and Stewart, A. (2012). The multidimensional assessment of interoceptive awareness (MAIA). PLoS One 7:e48230. doi: 10.1371/journal.pone.0048230
Mueller-Smith, M., and Schnepel, K. T. (2021). Diversion in the criminal justice system. Rev. Econ. Stud. 88, 883–936. doi: 10.1093/restud/rdaa030
Najavits, L. M., Weiss, R. D., Shaw, S. R., and Muenz, L. R. (1998). “Seeking safety”: outcome of a new cognitive-behavioral psychotherapy for women with posttraumatic stress disorder and substance dependence. J. Traumatic Stress 11, 437–456. doi: 10.1023/A:1024496427434
Nardo, T., Batchelor, J., Berry, J., Francis, H., Jafar, D., and Borchard, T. (2021). Cognitive remediation as an adjunct treatment for substance use disorders: a systematic review. Neuropsychol. Rev. doi: 10.1007/s11065-021-09506-3
National Institute on Drug Abuse, NIOH, U.S. Department of Health and Human, and Services. (2020). Opioid overdose crisis. Available at: https://www.drugabuse.gov/drugs-abuse/opioids/opioid-overdose-crisis (Accessed August 15, 2024).
Norman, S. B., Cissell, S. H., Means-Christensen, A. J., and Stein, M. B. (2006). Development and validation of an overall anxiety severity and impairment scale (OASIS). Depress. Anxiety 23, 245–249. doi: 10.1002/da.20182
Obermeyer, Z., and Emanuel, E. J. (2016). Predicting the future - big data, machine learning, and clinical medicine. N. Engl. J. Med. 375, 1216–1219. doi: 10.1056/NEJMp1606181
Ozturk, B., Bell, A., McLeod, D., and Gentzler, R. (2022). Criminal justice diversion and the importance of program retention. Journal of Forensic Social Work 6, 43–56. doi: 10.15763/issn.1936-9298.2022.6.1.43-56
Perry, L., Morgan, J., Reid, F., Brunton, J., O'Brien, A., Luck, A., et al. (2002). Screening for symptoms of eating disorders: reliability of the SCOFF screening tool with written compared to oral delivery. Int. J. Eat. Disord. 32, 466–472. doi: 10.1002/eat.10093
Petker, T., Yanke, C., Rahman, L., Whalen, L., Demaline, K., Whitelaw, K., et al. (2021). Naturalistic evaluation of an adjunctive yoga program for women with substance use disorders in inpatient treatment: within-treatment effects on cravings, self-efficacy, psychiatric symptoms, impulsivity, and mindfulness. Substance Abuse: Research and Treatment 15:11782218211026651. doi: 10.1177/11782218211026651
Price, C. J., and Hooven, C. (2018). Interoceptive awareness skills for emotion regulation: theory and approach of mindful awareness in body-oriented therapy (MABT). Front. Psychol. 9:798. doi: 10.3389/fpsyg.2018.00798
Price, C. J., Thompson, E. A., Crowell, S., and Pike, K. (2019a). Longitudinal effects of interoceptive awareness training through mindful awareness in body-oriented therapy (MABT) as an adjunct to women’s substance use disorder treatment: a randomized controlled trial. Drug Alcohol Depend. 198, 140–149. doi: 10.1016/j.drugalcdep.2019.02.012
Price, C. J., Thompson, E. A., Crowell, S. E., Pike, K., Cheng, S. C., Parent, S., et al. (2019b). Immediate effects of interoceptive awareness training through mindful awareness in body-oriented therapy (MABT) for women in substance use disorder treatment. Subst. Abus. 40, 102–115. doi: 10.1080/08897077.2018.1488335
Raftery, D., Kelly, P. J., Deane, F. P., Baker, A. L., Ingram, I., Goh, M. C. W., et al. (2020). Insight in substance use disorder: a systematic review of the literature. Addict. Behav. 111:106549. doi: 10.1016/j.addbeh.2020.106549
Shaffer, D. K. (2011). Looking inside the black box of drug courts: a meta-analytic review. Justice Q. 28, 493–521. doi: 10.1080/07418825.2010.525222
Shannon, L. M., Jones, A. J., Newell, J., and Payne, C. (2018). Examining individual characteristics and program performance to understand two-year recidivism rates among drug court participants: comparing graduates and terminators. Int. J. Offender Ther. Comp. Criminol. 62, 4196–4220. doi: 10.1177/0306624X18769602
Shapiro, G. K., Cusi, A., Kirst, M., O’Campo, P., Nakhost, A., and Stergiopoulos, V. (2014). Co-responding police-mental health programs: a review. Adm. Policy Ment. Health Ment. Health Serv. Res. 42, 606–620. doi: 10.1007/s10488-014-0594-9
Shiffman, S. (2009). Ecological momentary assessment (EMA) in studies of substance use. Psychol. Assess. 21, 486–497. doi: 10.1037/a0017074
Sirotich, F. (2009). The criminal justice outcomes of jail diversion programs for persons with mental illness: a review of the evidence. J. Am. Acad. Psychiatry Law 37, 461–472
Skinner, H. A. (1982). The drug abuse screening test. Addict. Behav. 7, 363–371. doi: 10.1016/0306-4603(82)90005-3
Smith, R., Feinstein, J. S., Kuplicki, R., Forthman, K. L., Stewart, J. L., Paulus, M. P., et al. (2021). Perceptual insensitivity to the modulation of interoceptive signals in depression, anxiety, and substance use disorders. Sci. Rep. 11:2108. doi: 10.1038/s41598-021-81307-3
Smith, R., Kuplicki, R., Feinstein, J., Forthman, K. L., Stewart, J. L., Paulus, M. P., et al. (2020a). A Bayesian computational model reveals a failure to adapt interoceptive precision estimates across depression, anxiety, eating, and substance use disorders. PLoS Comput. Biol. 16:e1008484. doi: 10.1371/journal.pcbi.1008484
Smith, R., Schwartenbeck, P., Stewart, J. L., Kuplicki, R., Ekhtiari, H., and Paulus, M. P. (2020b). Imprecise action selection in substance use disorder: evidence for active learning impairments when solving the explore-exploit dilemma. Drug Alcohol Depend. 215:108208. doi: 10.1016/j.drugalcdep.2020.108208
Spielberger, C. D., Gorsuch, R. L., and Lushene, R. E. (1970).Manual for the State-Trait Anxiety Inventory. Polo Alto, CA: Consulting Psychologists Press.
Stewart, J. L., Khalsa, S. S., Kuplicki, R., Puhl, M., Investigators, T., and Paulus, M. P. (2020). Interoceptive attention in opioid and stimulant use disorder. Addict. Biol. 25:e12831. doi: 10.1111/adb.12831
Stice, E., Telch, C. F., and Rizvi, S. L. (2000). Development and validation of the eating disorder diagnostic scale: a brief self-report measure of anorexia, bulimia, and binge-eating disorder. Psychol. Assess. 12, 123–131. doi: 10.1037/1040-3590.12.2.123
Stunkard, A. J., and Messick, S. (1985). The three-factor eating questionnaire to measure dietary restraint, disinhibition and hunger. J. Psychosom. Res. 29, 71–83. doi: 10.1016/0022-3999(85)90010-8
Suykens, J. A. K., and Vandewalle, J. (1999). Least squares support vector machine classifiers. Neural. Process. Lett. 9, 293–300. doi: 10.1023/A:1018628609742
Symons, M., Feeney, G. F. X., Gallagher, M. R., Young, R. M., and Connor, J. P. (2019). Machine learning vs addiction therapists: a pilot study predicting alcohol dependence treatment outcome from patient data in behavior therapy with adjunctive medication. J. Subst. Abus. Treat. 99, 156–162. doi: 10.1016/j.jsat.2019.01.020
Szafranski, D. D., Snead, A., Allan, N. P., Gros, D. F., Killeen, T., Flanagan, J., et al. (2017). Integrated, exposure-based treatment for PTSD and comorbid substance use disorders: predictors of treatment dropout. Addict. Behav. 73, 30–35. doi: 10.1016/j.addbeh.2017.04.005
Taylor, G. J., Bagby, R. M., and Parker, J. D. A. (2003). The 20-item Toronto alexithymia scale: IV. Reliability and factorial validity in different languages and cultures. J. Psychosom. Res. 55, 277–283. doi: 10.1016/S0022-3999(02)00601-3
Taylor, S., Zvolensky, M. J., Cox, B. J., Deacon, B., Heimberg, R. G., Ledley, D. R., et al. (2007). Robust dimensions of anxiety sensitivity: development and initial validation of the anxiety sensitivity Index-3. Psychol. Assess. 19, 176–188. doi: 10.1037/1040-3590.19.2.176
Treynor, W., Gonzalez, R., and Nolen-Hoeksema, S. (2003). Rumination reconsidered: a psychometric analysis. Cogn. Ther. Res. 27, 247–259. doi: 10.1023/A:1023910315561
Tuchman, E. (2010). Women and addiction: the importance of gender issues in substance abuse research. J. Addict. Dis. 29, 127–138. doi: 10.1080/10550881003684582
Vabalas, A., Gowen, E., Poliakoff, E., and Casson, A. J. (2019). Machine learning algorithm validation with a limited sample size. PLoS One 14:e0224365. doi: 10.1371/journal.pone.0224365
Van Voorhis, P., Wright, E. M., Salisbury, E., and Bauman, A. (2010). Women’s risk factors and their contributions to existing risk/needs assessment: the current status of a gender-responsive supplement. Crim. Justice Behav. 37, 261–288. doi: 10.1177/0093854809357442
Victor, T. A., Khalsa, S. S., Simmons, W. K., Feinstein, J. S., Savitz, J., Aupperle, R. L., et al. (2018). Tulsa 1000: a naturalistic study protocol for multilevel assessment and outcome prediction in a large psychiatric sample. BMJ Open 8:e016620. doi: 10.1136/bmjopen-2017-016620
Vrana, S., and Lauterbach, D. (1994). Prevalence of traumatic events and post-traumatic psychological symptoms in a nonclinical sample of college students. J. Trauma. Stress. 7, 289–302. doi: 10.1007/BF02102949
Watson, D.B., and Clark, L.A. (1994). The PANAS-X: manual for the positive and negative affect schedule - expanded form. doi: 10.17077/48vt-m4t2
Waugh, C. E., Shing, E. Z., and Furr, R. M. (2020). Not all disengagement coping strategies are created equal: positive distraction, but not avoidance, can be an adaptive coping strategy for chronic life stressors. Anxiety Stress Coping 33, 511–529. doi: 10.1080/10615806.2020.1755820
Wechsler, D. (2008). Manual for the Wechsler Adult Intelligence Scale. 4th Edn. San Antonio, TX: Pearson.
Whiteside, S. P., and Lynam, D. R. (2001). The five factor model and impulsivity: using a structural model of personality to understand impulsivity. Personal. Individ. Differ. 30, 669–689. doi: 10.1016/S0191-8869(00)00064-7
Whiteside, S. P., Lynam, D. R., Miller, J. D., and Reynolds, S. K. (2005). Validation of the UPPS impulsive behaviour scale: a four-factor model of impulsivity. Eur. J. Personal. 19, 559–574. doi: 10.1002/per.556
Wilkinson, G. S. R. G. J. P. A. R. I. (2006). WRAT 4: wide range achievement test; professional manual. Lutz, FL: Psychological Assessment Resources, Inc.
Wolgast, M., and Lundh, L.-G. (2017). Is distraction an adaptive or maladaptive strategy for emotion regulation? A person-oriented approach. J. Psychopathol. Behav. Assess. 39, 117–127. doi: 10.1007/s10862-016-9570-x
Zettler, H. R. (2018). Exploring the relationship between dual diagnosis and recidivism in drug court participants. Crime Delinq. 64, 363–397. doi: 10.1177/0011128717697960
Zettler, H. R. (2019). The impact of dual diagnosis on drug court failure. Int. J. Offender Ther. Comp. Criminol. 63, 357–382. doi: 10.1177/0306624X18803832
Keywords: substance use treatment completion, substance abuse treatment, prison diversion program completion, women’s substance use, machine learning
Citation: Choquette EM, Forthman KL, Kirlic N, Stewart JL, Cannon MJ, Akeman E, McMillan N, Mesker M, Tarrasch M, Kuplicki R, Paulus MP and Aupperle RL (2024) Impulsivity, trauma history, and interoceptive awareness contribute to completion of a criminal diversion substance use treatment program for women. Front. Psychol. 15:1390199. doi: 10.3389/fpsyg.2024.1390199
Edited by:
Jesús-Nicasio García-Sánchez, University of León, SpainReviewed by:
Lina Harvold Ellingsen-Dalskau, Norwegian Institute of Public Health (NIPH), NorwayAlicia Ito Ford, Oklahoma State University Center for Health Sciences, United States
Richard Hammersley, University of Hull, United Kingdom
Copyright © 2024 Choquette, Forthman, Kirlic, Stewart, Cannon, Akeman, McMillan, Mesker, Tarrasch, Kuplicki, Paulus and Aupperle. This is an open-access article distributed under the terms of the Creative Commons Attribution License (CC BY). The use, distribution or reproduction in other forums is permitted, provided the original author(s) and the copyright owner(s) are credited and that the original publication in this journal is cited, in accordance with accepted academic practice. No use, distribution or reproduction is permitted which does not comply with these terms.
*Correspondence: Emily M. Choquette, echoquette@laureateinstitute.org