- 1Research Center for Youth Education, National Institution For Youth Education, Tokyo, Japan
- 2Rikkyo University, Tokyo, Japan
Introduction: Environmental sensitivity is defined as the ability to perceive and process internal and external information. Previous studies have suggested that mental health-related factors differ by sensitivity level. This study aimed to investigate whether environmental sensitivity moderates the associations between cognitive emotion regulation strategies (e.g., rumination and blaming others) and mental health.
Materials and methods: In this three-wave longitudinal study, participants (N = 1,233, 585, and 349 at Times 1, 2, and 3, respectively) completed the Cognitive Emotion Regulation Questionnaire-short and Kessler 10 scale at all the measurement points as well as the 10-item version of the Highly Sensitive Person scale and some covariates only at Time 1.
Results: Latent growth model analyses indicated that the blaming others strategy had contrastive effects on changes in mental health by sensitivity level; the increase in refocusing on planning was associated with improved mental health over time only for highly sensitive individuals; and the rumination and catastrophizing strategies were the most important risk factors for mental health problems, although their effects differed slightly by sensitivity level.
Conclusion: The associations between some of the cognitive strategies and mental health differ by environmental sensitivity level. Future investigations based on individual differences in sensitivity could provide innovative insights into practices.
1 Introduction
In daily life, people often experience negative emotions such as anxiety and anger. These emotions must be dealt with appropriately to enhance well-being and/or promote adaptive behaviors (Gross, 1998). The framework of cognitive emotion regulation comprehensively describes the cognitive strategies that individuals use in response to threatening or stressful life events (Garnefski et al., 2001). Garnefski et al. (2001) assumed that emotion regulation through cognition is inextricably associated with human life and reviewed the literature regarding the cognitive aspects of coping. Consequently, nine conceptually different strategies were extracted: (1) positive reappraisal— thinking about attaching a positive meaning to the event in terms of personal growth; (2) putting into perspective—comparing an event other events and downgrading the event’s importance; (3) rumination—thinking about the feelings and thoughts associated with negative events; (4) acceptance—resigning oneself to what has happened; (5) self-blame—blaming oneself after experiencing a stressful event; (6) positive refocusing—thinking about positive experiences instead of thinking about the actual event; (7) blaming others—blaming the environment or another person for a negative event one has experienced; (8) catastrophizing—overemphasizing the terror of what one has experienced; and (9) refocusing on planning—thinking about what steps to take and how to handle negative events (Garnefski et al., 2001; Garnefski and Kraaij, 2006a). These strategies could be useful targets for intervention (Garnefski et al., 2005). For example, Nelis et al. (2011) suggested that a cognitive behavioral approach could enhance the appropriate use of these strategies.
To measure the nine strategies, the Cognitive Emotion Regulation Questionnaire (CERQ) and its short version (CERQ-short) were developed (Garnefski et al., 2001; Garnefski and Kraaij, 2006a). The original assumption was that five strategies—positive reappraisal, putting into perspective, acceptance, positive refocusing, and refocusing on planning—were adaptive, whereas rumination, self-blame, blaming others, and catastrophizing were maladaptive. However, some empirical studies on the relationship between cognitive strategies and mental health indices (e.g., depression and anxiety) have failed to provide evidence consistent with their theoretical background (Garnefski et al., 2001; Garnefski and Kraaij, 2006b; Potthoff et al., 2016). A possible explanation for these inconsistencies between theory and evidence is that personal and contextual factors moderate the relationship between strategies and mental health (Aldao et al., 2010). Although studies have considered specific populations like foot-and-mouth crises (Garnefski et al., 2005), situations like the COVID-19 lockdown (Rodas et al., 2022), and personal factors such as nationality (Potthoff et al., 2016) and age (Garnefski and Kraaij, 2006b), little attention has been paid to psychological characteristics. However, given that different factors predict depressive tendencies based on personality (Yano et al., 2021b), investigating its moderating role in the associations between cognitive emotion regulation strategies and mental health could provide useful findings for more effective interventions.
Recently, psychologists have suggested that the concept of environmental sensitivity provides key information to mental health researchers and practitioners (Greven et al., 2019; Yano et al., 2021b). Environmental sensitivity is an overarching meta-framework of several psychological theories and concepts, including the differential susceptibility hypothesis (Belsky, 1997), biological sensitivity to context (Boyce and Ellis, 2005), and sensory processing sensitivity (SPS; Aron and Aron, 1997), and is defined as the ability to perceive and process internal and external information (Pluess, 2015; Greven et al., 2019).1 The level of sensitivity can be assessed using genetic (e.g., Keers et al., 2016), biological/physiological (e.g., Pluess et al., 2022), and psychological factors (e.g., Slagt et al., 2018). A growing number of studies have focused on SPS, a psychological marker of environmental sensitivity (Greven et al., 2019). SPS is a normally distributed and highly heritable trait (Pluess et al., 2018; Assary et al., 2021), whose core characteristics are the deeper processing of environmental information, showing stronger emotional and physiological reactivity to positive and negative stimuli, having greater awareness of subtle cues, and getting overstimulated more easily (Aron et al., 2012; Homberg et al., 2016). These features could at least partly be captured by two self-reported scales: the Highly Sensitive Person (HSP) scale (Aron and Aron, 1997) and Highly Sensitive Child scale (Pluess et al., 2018). Numerous studies have used these scales and revealed the differences between SPS and other personality constructs (e.g., Lionetti et al., 2019; Iimura et al., 2023).2
In view of susceptibility to environmental influences (Pluess, 2015), people scoring high on SPS benefit more from psychological education programs focusing on emotion regulation (Pluess and Boniwell, 2015; Kibe et al., 2020). These findings suggest that the factors strongly associated with mental health differ by SPS level. A previous systematic review found that dysfunctional thoughts such as rumination and catastrophizing are more important predictors of depression and/or anxiety, particularly for higher-sensitivity individuals (Bratholm Wyller et al., 2017). Lionetti et al.’s (2022) empirical investigation supported this assumption, while emphasizing the moderating role of the parenting environment. Additionally, the results of an open-ended survey conducted to explore the characteristics and effectiveness of coping strategies among university students with high and low sensitivity (Yano et al., 2021a) indicated that although strategies related to emotion regulation were extracted in all the sensitivity groups, their associations with mental health partly differed between the groups. Specifically, while positive thinking (similar to positive reappraisal) may be more effective for individuals with higher sensitivity, it may be important for lower-sensitivity individuals to receive emotional and instrumental support from friends who understand their feelings and offer useful advice. Thus, environmental sensitivity could moderate the association between cognitive emotion regulation strategies and mental health, although its moderating effect has been insufficiently examined. Additionally, there are limitations to adapting the existing evidence to practice because of the data being cross-sectional or qualitative (Yano and Oishi, 2018; Greven et al., 2019).
Given that over 300 million people globally live with mental disorders (GBD 2017 Disease and Injury Incidence and Prevalence Collaborators, 2018), findings regarding the association between cognitive emotion regulation strategies and mental health can provide key information for practice. Although such associations may differ by the level of environmental sensitivity (Aldao et al., 2010; Yano et al., 2021a), few studies have examined their moderating effect. Therefore, further longitudinal studies are required to enhance their adaptability to practice (Yano and Oishi, 2018).
A popular statistical method for longitudinal data is latent growth model analysis (Duncan et al., 2013), as it can describe individuals’ behavior or status, in terms of their initial levels and change rates over time. Additionally, the associations of the (changes in) predictors and their interaction terms (Klein and Moosbrugger, 2000) with the (changes in) outcomes can be evaluated using latent moderated structural equation modeling (LMS).
To overcome the limitations of past studies, this longitudinal study aimed to investigate whether environmental sensitivity moderates the associations between each strategy of cognitive emotion regulation and mental health. Building on the existing findings (Bratholm Wyller et al., 2017; Yano et al., 2021a; Lionetti et al., 2022), the authors hypothesized the moderating effect of environmental sensitivity on the associations between positive reappraisal, rumination, catastrophizing, and refocusing on planning and mental health: the former three strategies play a vital role for those with higher sensitivity and the other is important for low-sensitivity individuals. Unfortunately, clear hypotheses on other strategies could not be proposed because of a lack of evidence.
2 Materials and methods
2.1 Participants and procedures
This three-wave longitudinal study recruited Japanese university students from all prefectures through Cross Marketing, Inc.3 At Time 1 (November 2020), 1,233 students consented to participate in the study after they received an explanation of the study’s purpose and procedures. Data were subsequently collected from 585 and 349 university students at Time 2 (February 2021) and Time 3 (May 2021), respectively. The intervals between each survey were set so that the overall period was 6 months (Porru et al., 2022; Eisma et al., 2023).
The Directed Questions Scale (Maniaci and Rogge, 2014) was included in each scale to assess the respondents’ attitudes toward the survey. Based on Maniaci and Rogge (2014) recommendation, in each survey, the responses were treated as missing values when a participant did not follow the Directed Questions Scale more than M + 2.7 SD times (i.e., thrice at Time 1, twice at Time 2, and once at Time 3). The number of participants who provided data and were included in the analyses is shown in Table 1. The comparison of the characteristics between the participants who completed all the surveys and those who did not indicated that while more women completed the surveys, men were likely to drop out (ꭓ2(2) = 16.69, p < 0.001, Cramer’s V = 0.12). Moreover, the complete group was older than the incomplete group (t(642.18) = 3.30, p = 0.001, Cohen’s d = 0.21). Among the psychological characteristics, there were insignificant or negligible (though significant) differences (for the details, see Supplementary Table S1).
The study’s procedures were approved by the Ethics Committee of Rikkyo University (Nos. KOMI19001A and KOMI20010A).
2.2 Measurements
2.2.1 Environmental sensitivity
This study considered the concept of SPS as a marker of environmental sensitivity (Slagt et al., 2018; Greven et al., 2019) and assessed it using the 10-item Japanese version of the HSP scale (HSP-J10) (Iimura et al., 2023)—a shorter version of the original HSP scale (Aron and Aron, 1997). The HSP-J10 has a bi-factor structure with a general sensitivity factor, in addition to Ease of Excitation (five items), Low Sensory Threshold (three items), and Aesthetic Sensitivity (two items). Each item was rated on a seven-point Likert-type scale (1 = “Strongly disagree” to 7 = “Strongly agree”), with higher scores indicating higher SPS. As mentioned in the Introduction, SPS is a heritable trait (Assary et al., 2021) and the scores of the HSP scale have high temporal stability (Konrad and Herzberg, 2019; Iimura et al., 2023). Therefore, the authors assessed the HSP-J10 only at Time 1.
2.2.2 Mental health
The Japanese version of the Kessler 10 scale (Kessler et al., 2002; Furukawa et al., 2008) was used to assess mental health at all the measurement points. This scale consists of 10 items, which ask respondents how often they experienced depressive or anxiety symptoms during the last month. Each item was rated on a five-point Likert-type scale (0 = “None of the time” to 4 = “all of the time”), with higher scores indicating poorer mental health.
2.2.3 Cognitive emotion regulation
The Japanese version of the CERQ-short (Garnefski and Kraaij, 2006a; Sakakibara, 2017) was used at all the measurement points to assess how often the participants employed the nine conceptually distinct strategies to regulate their emotions in a general and particular situation, with two items in each strategy. Each item was rated on a five-point Likert-type scale (1 = “[Almost] never” to 5 = “[Almost] always”), with higher scores indicating more frequent use of a specific strategy. This being the first study to investigate the moderating role of sensitivity in the association between cognitive strategies and mental health, the authors decided to focus on a general situation.
2.2.4 Neuroticism
Given that some items of the HSP-J10 involved negative words such as “uncomfortable” and “overwhelmed,” despite the neutrality of environmental sensitivity, it could be negatively biased to correlate with other psychological concepts (Greven et al., 2019). Therefore, in line with previous studies (Yano et al., 2021b), the current study included neuroticism as a control variable. The participants also responded to two items from the Japanese version of the 10-Item Personality Inventory (Gosling et al., 2003; Oshio et al., 2012) only at Time 1. Each item was rated on a seven-point Likert-type scale (1 = “Strongly disagree” to 7 = “Strongly agree”), with higher scores indicating higher neuroticism. As the two-item correlation was weak but significant (r = 0.11, p < 0.001), the authors considered its reliability to be acceptable.
2.3 Statistical analyses
After investigating the correlations of the cognitive strategies with SPS and mental health, the authors performed three statistical analyses. First, second-order univariate latent growth model analyses (Hancock et al., 2001) were conducted to estimate the mean levels (i.e., intercept) and change rates (i.e., slopes) in mental health and each cognitive emotion regulation strategy (Figure 1A).4 To achieve metric equivalence in the latent constructs, unstandardized factor loadings and error variances were constrained equally between the measurement points. Second, the associations between SPS and each strategy with mental health were investigated using a multivariate latent growth model, followed by LMS analyses in which the interaction terms between SPS and the intercept and slope of a cognitive strategy were added into the predictors (Figure 1B). All the models involved three control variables (gender, age, and neuroticism) and were estimated using the robust maximum likelihood estimation method. The Mplus code required for the LMS analysis was taken from Maslowsky et al. (2015). Finally, the models with and without interaction terms were compared through log-likelihood ratio difference tests (Maslowsky et al., 2015), using an appropriate correction for the maximum likelihood estimation method (Satorra and Bentler, 2001). When the former model’s fit to data was better than the latter’s and the interaction terms were significantly associated with the intercept and/or slope of mental health, the authors performed simple slope tests and calculated the region of significance for SPS.5
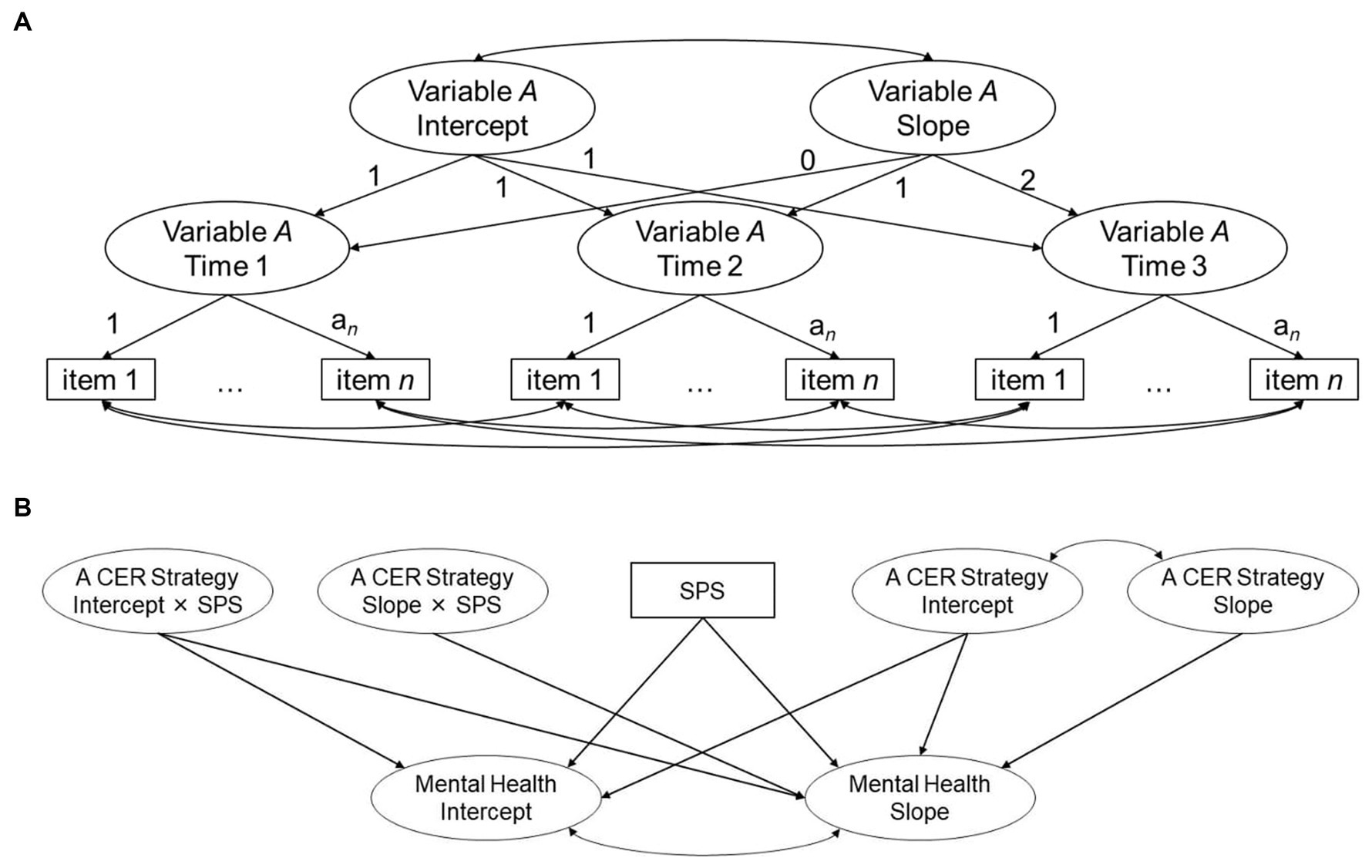
Figure 1. Illustration of the models estimated in this study. CER, Cognitive Emotion Regulation; SPS, Sensory Processing Sensitivity. Panel (A) illustrates a univariate latent growth model in which mental health or a cognitive emotion regulation strategy was modeled. Panel (B) represents a latent moderated structural equation model in which control variables (i.e., gender, age, and neuroticism) predicting the intercept and slope of mental health were omitted for brevity. When the multivariate latent growth model analysis was performed, the two interaction terms were not included in the model.
In the univariate and multivariate latent growth model analyses, the goodness of fit of the model was comprehensively evaluated based on the comparative fit index (CFI) and root mean square error of approximation (RMSEA). Values >0.90 for CFI and < 0.10 for RMSEA were acceptable (Kline, 2005). Given that these analyses estimated a large number of parameters, to control for the inflation of Type I error rates, the false discovery rate procedure was employed (Benjamini and Hochberg, 1995). The authors used the free statistical software HAD ver. 17.20 (Shimizu, 2016) for the correlation analysis; Mplus ver. 8.3 (Muthén and Muthén, 2017) for the latent growth model and LMS analyses; and a free application6 introduced by Roisman et al. (2012) for the simple slope tests and to calculate the regions of significance. The significance levels were set at p < 0.05, and given the large sample size, the effect size was also considered to interpret the correlations (i.e., 0.10, 0.20, and 0.30 as relatively small, typical, and relatively large effect sizes, respectively; Gignac and Szodorai, 2016). Missing values were handled using the full information maximum likelihood estimation method.
3 Results
3.1 Preliminary analyses
For the preliminary analyses, the correlation coefficients between SPS, mental health, and the cognitive emotion regulation strategies were calculated (Table 2). The results indicated that SPS was positively correlated with rumination (relatively large), self-blame (typical), and catastrophizing (typical to relatively large) measured at all the time points as well as with acceptance (relatively small to typical) and refocusing on planning (relatively small) at Time 1 and Time 2 (p < 0.05). Significant correlations were found between mental health and positive reappraisal, self-blame, positive refocusing (relatively small to typical), rumination, and catastrophizing (typical to relatively large), at all the corresponding time points; putting into perspective at Time 2 and Time 3 (relatively small); and refocusing on planning (relatively small) only at Time 2 (p < 0.05). Additionally, the rank-order stabilities for all the nine strategies were greater than “relatively large” (r > 0.40; see Supplementary Table S3).
3.2 Change in mental health and cognitive emotion regulation strategies
To estimate the initial level and change rate in each variable, a series of univariate latent growth model analyses were conducted (Table 3). The covariances were set between some items measured at the same time points based on the modification indices (Slagt et al., 2018). While the fit indices were acceptable for all the models (CFI = 0.90–1.00, RMSEA = 0.00–0.07), for acceptance, only the initial level and its variance were estimated owing to the negative variance of its slope factor. The variances of the slope factors in the other strategies and mental health showed significant values (p < 0.001), indicating that there were significant inter-individual differences in the variables’ change rates. The scores of refocusing on planning decreased over the time points at the mean level (p = 0.009). Furthermore, negative correlations were seen between the intercept and slope in all the variables, except acceptance (p < 0.01).
3.3 Associations between mental health and cognitive emotion regulation strategies
First, nine models were estimated using a multivariate latent growth model, with the intercept and slope of the cognitive strategy, SPS, and control variables (i.e., gender, age, and neuroticism) as predictors and the intercept and slope of mental health as outcomes. The results showed good fit indices for all the models (CFI = 0.91–0.93, RMSEA = 0.04). Next, we estimated nine LMS models in which the interaction terms between SPS and the intercept or slope of the cognitive strategies were added (Figure 1B). A series of log-likelihood ratio difference tests suggested that the models with interactions fitted the data better when putting into perspective, rumination, blaming others, catastrophizing, or refocusing on planning were predictors, whereas those without interactions were supported when positive reappraisal, acceptance, self-blame, or positive refocusing were predictors (Table 4).
The estimated parameters for the final model are presented in Table 5. To save space, the results for the control variables are in the Supplementary materials (see Supplementary Tables S4–S12). In the four models without interactions, the intercepts of positive reappraisal, acceptance, and positive refocusing were negatively associated with the intercept of mental health (p < 0.01), whereas self-blame was not significantly associated. In the five models with interactions, the intercepts of putting into perspective and refocusing on planning were negatively associated with mental health (p < 0.001). Additionally, positive associations were indicated between the intercepts of rumination and catastrophizing and mental health and between the slopes of those strategies and mental health (p < 0.01). Furthermore, (changes in) mental health was significantly associated with the five interaction terms between SPS and the intercepts of rumination and blaming others, and the slopes of blaming others, catastrophizing, and refocusing on planning (p < 0.05). Finally, SPS was positively associated with the intercept of mental health (p < 0.001).
For the significant interaction terms, simple slope tests were conducted and the regions of significance of the moderator (i.e., SPS) were calculated. First, the participants with SPS scores higher than M – 0.46 SD had poorer mental health when they used rumination more frequently (Figure 2). Second, those who frequently used blaming others improved their mental health over time when their SPS scores were higher than M + 0.61 SD, whereas their mental health worsened when their SPS scores were lower than M – 0.49 SD (Figure 3). Third, likewise, the participants who increased their frequency of blaming others over time improved their mental health when their SPS scores were higher than M + 1.79 SD, whereas their mental health worsened with SPS scores lower than M – 0.58 SD (Figure 4). Fourth, the mental health of the participants whose frequent use of catastrophizing increased worsened further only when their SPS scores were lower than M + 0.20 SD (Figure 5). Finally, when the participants’ frequent use of refocusing on planning kept increasing, their mental health further improved only when their SPS scores were higher than M + 0.83 SD (Figure 6).
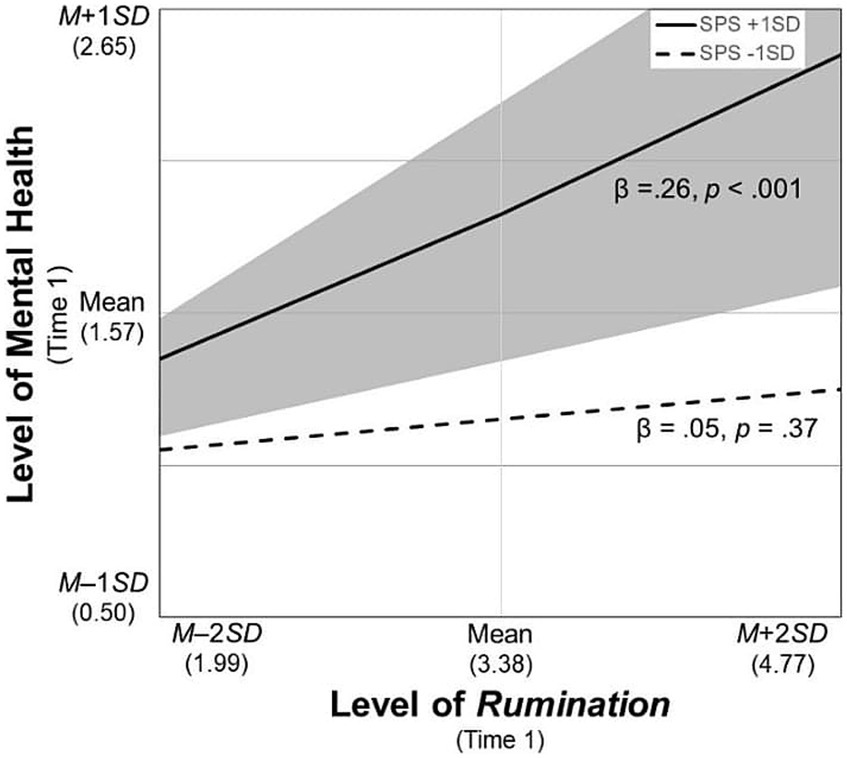
Figure 2. Simple slopes of rumination on mental health at Time 1. SPS, Sensory Processing Sensitivity. The shaded area indicates the SPS values at which the predictor is significantly associated with the level of mental health (i.e., lower than M – 4.38 SD and greater than M – 0.46 SD).
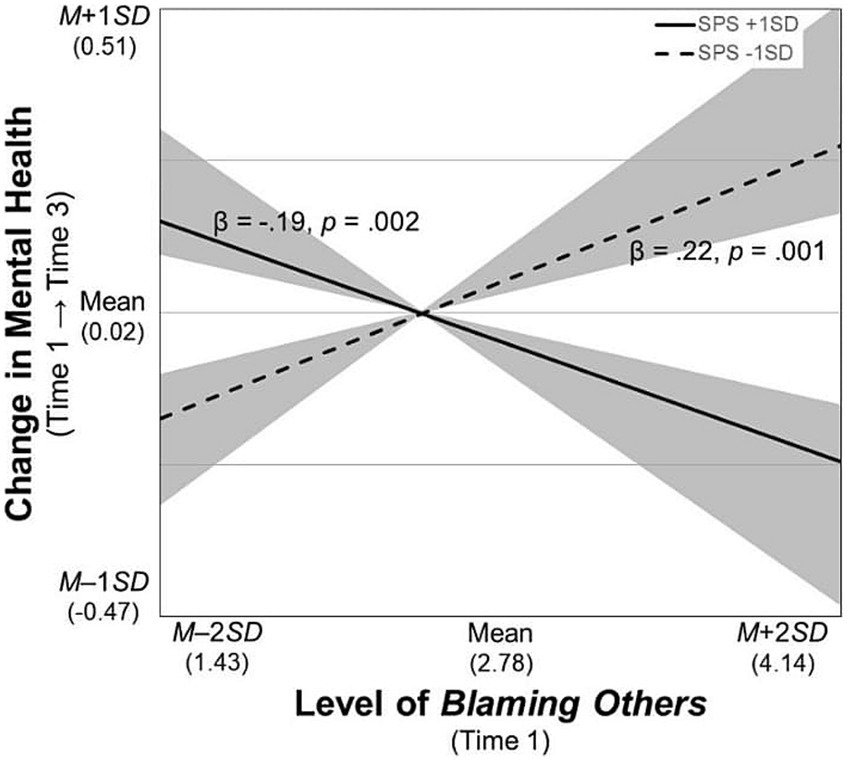
Figure 3. Simple slopes of blaming others at Time 1 on changes in mental health. SPS, Sensory Processing Sensitivity. The shaded area indicates the SPS values at which the predictor is significantly associated with the slopes of mental health (i.e., lower than M – 0.49 SD and greater than M + 0.61 SD).
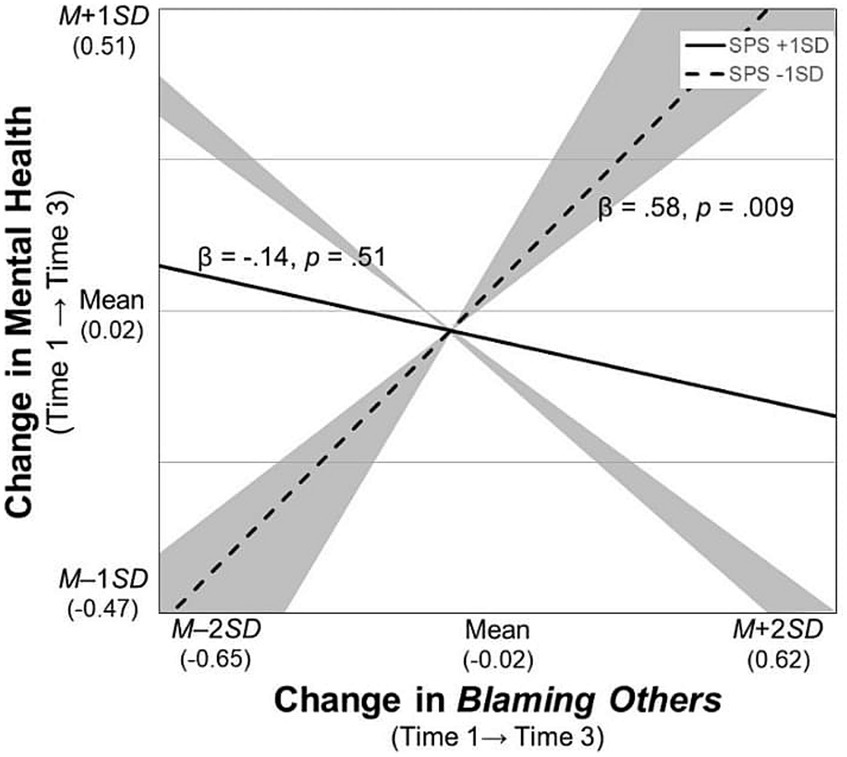
Figure 4. Simple slopes of change in blaming others on changes in mental health. Notes. SPS, Sensory Processing Sensitivity. The shaded area indicates the SPS values at which, the predictor is significantly associated with the slopes of mental health (i.e., lower than M – 0.58 SD and greater than M + 1.79 SD).
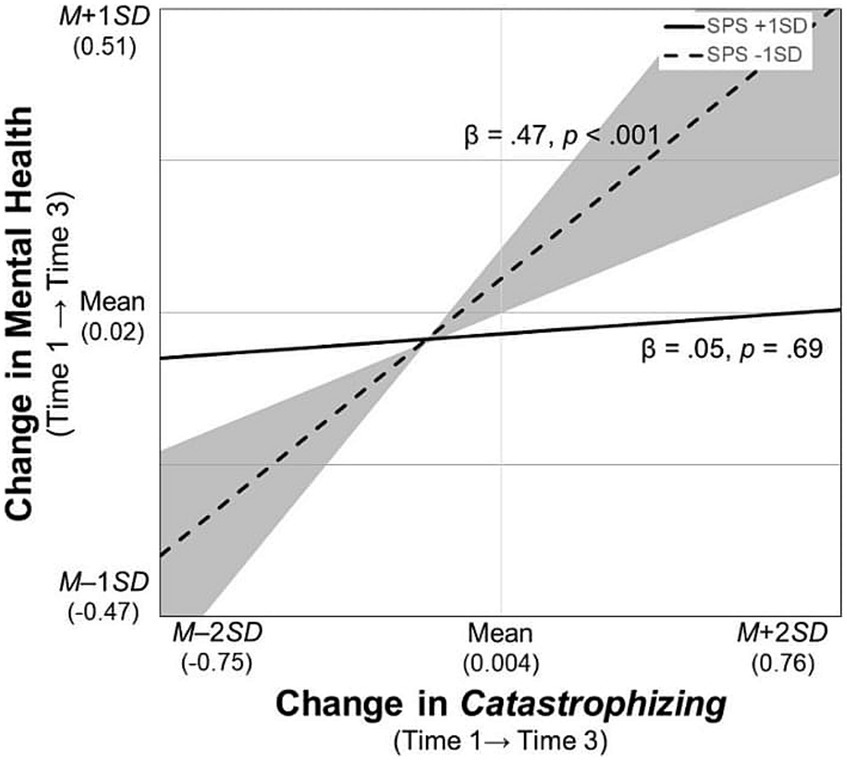
Figure 5. Simple slopes of change in catastrophizing on changes in mental health. SPS, Sensory Processing Sensitivity. The shaded area indicates the SPS values, at which, the predictor is significantly associated with the slopes of mental health (i.e., lower than M + 0.20 SD and greater than M + 2.51 SD).
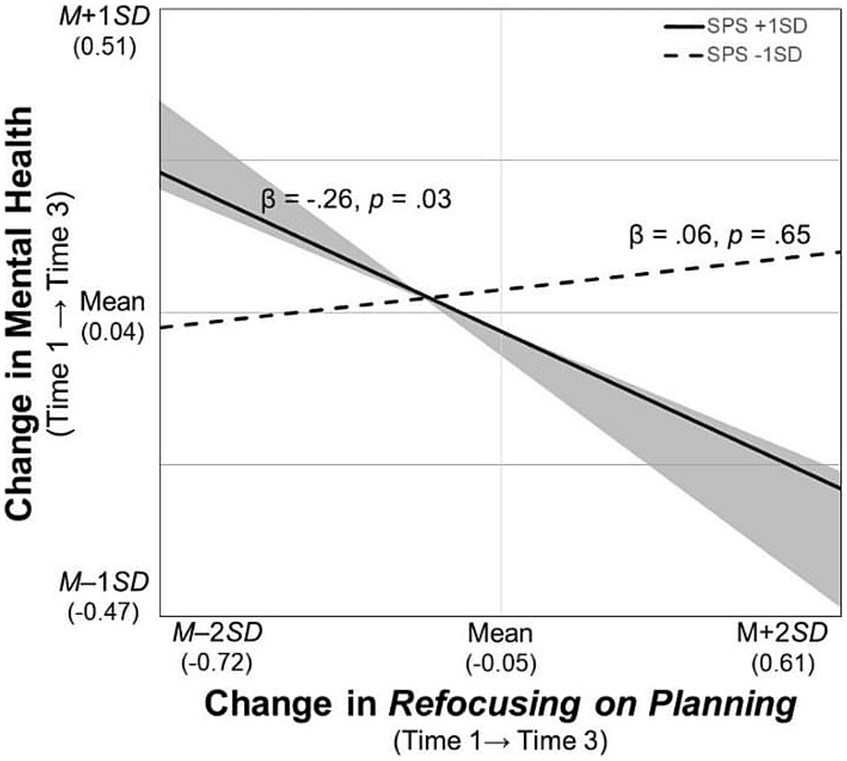
Figure 6. Simple slopes of change in refocusing on planning on changes in mental health. SPS, Sensory Processing Sensitivity. The shaded area indicates the SPS values, at which, the predictor is significantly associated with the slopes of mental health (i.e., lower than M – 2.47 SD and greater than M + 0.83 SD).
4 Discussion
4.1 Associations of the cognitive emotion regulation strategies with mental health
This study conducted a three-wave longitudinal survey and investigated the associations between (the changes in) the nine cognitive emotion regulation strategies and (the changes in) mental health based on individual differences in environmental sensitivity. The results indicated that the interactions between sensitivity and (the changes in) four of the strategies significantly predicted mental health at the initial level or its rate of change over time (Table 5).
First, more frequent use of rumination was associated with poorer mental health at Time 1 only for those whose sensitivity scores were higher than M – 0.46 SD (Figure 2). This result was consistent with our hypothesis and could elaborate the existing finding that highly sensitive individuals tend to engage in ruminative thinking in less supportive environments (Lionetti et al., 2022). Compared with individuals with low sensitivity, the effect of rumination on mental health may be stronger for highly sensitive individuals owing to their characteristics related to in-depth processing of internal information such as feelings and thoughts (Aron et al., 2012; Greven et al., 2019). Further, given the positive association between the slopes of these variables, regardless of their sensitivity level, an increase in rumination could be a risk factor even when individuals have low sensitivity.
While the significantly positive association between catastrophizing and mental health at Time 1 was consistent with previous findings (Garnefski and Kraaij, 2006a; Potthoff et al., 2016; Urano et al., 2022), their association in terms of the longitudinal data was inconsistent with the hypothesis. Rather than in high-sensitivity individuals, the change in catastrophizing may be an important risk factor for worsened mental health in lower-sensitivity individuals (i.e., scores lower than M + 0.20 SD) (Figure 5). The positive and relatively large correlation between sensitivity and catastrophizing at Time 1 (see Table 2) is a possible explanation for the insignificant association between the slopes of this strategy and mental health in highly sensitive individuals; that is, they might have little room for the strategy to increase over time.
In terms of the cross-sectional data, those participants who frequently used refocusing on planning were likely to have better mental health, which is consistent with previous findings (Potthoff et al., 2016; Urano et al., 2022). Contrary to the hypothesis, only when sensitivity was higher than M + 0.83 SD did those with an increase in using refocusing on planning tend to improve their mental health over time (Figure 6). This finding could provide novel insights into future research and practice because previous studies have suggested that problem-focused coping has negative consequences for highly sensitive individuals in some cases (Yano et al., 2021a) and that they could benefit more from an intervention program focusing on emotional coping skills (Pluess and Boniwell, 2015; Kibe et al., 2020). However, a significant association was not found between the slopes of refocusing on planning and mental health in low-sensitivity individuals, indicating that just increasing the frequency of thinking about how to resolve problems does not improve mental health. Given that decision-making skills such as summarizing information and planning play a vital role in alleviating depressive symptoms in individuals with low sensitivity (Yano et al., 2021b), future studies should consider the process through which this strategy could be linked to their behaviors.
The other hypothesis was also not supported. The strategy of positive reappraisal, as well as putting into perspective, acceptance, and positive refocusing, was associated with better mental health at Time 1, regardless of the sensitivity level, which was consistent with their theoretical backgrounds (Garnefski et al., 2001). However, in some cases, acceptance was correlated with more serious symptoms of depression and anxiety owing to this strategy’s scope being measured by the CERQ (−short), namely, a passive form of acceptance similar to resignation to negative experiences (Garnefski and Kraaij, 2006b; Urano et al., 2022). This study failed to replicate the association of self-blame with mental health, despite plenty of evidence for its contribution to psychological symptoms (Garnefski and Kraaij, 2006a, 2006b). This inconsistency could be explained by that the use of this strategy in the present study’s sample at Time 1 was more frequent than that in Garnefski and Kraaij (2006a) study where the sample responded to the CERQ-short (Cohen’s d = 1.39). However, the reason for our results remains questionable.
Finally, the more frequent use of blaming others at Time 1, or its increase over time, may worsen mental health when individuals have a sensitivity score lower than M – 0.49 SD or M – 0.58 SD (Figures 3, 4). These results advance the findings of the positive correlations between this strategy and depression or anxiety reported in cross-sectional research (Garnefski et al., 2001; Garnefski and Kraaij, 2006b; Potthoff et al., 2016; Urano et al., 2022). By contrast, when individuals are highly sensitive (scores higher than M + 0.61 SD or M + 1.79 SD), the initial level or the increase in this strategy could contribute to improving mental health over time. These findings are inconsistent with the existing evidence, and they suggest that this strategy is not always associated with poorer mental health, although it has been considered maladaptive a priori (Garnefski et al., 2001). The moderating effect of sensitivity on these associations could be explained by individual differences in behavior after attributing negative events to another person. For example, in-depth processing of a variety of information and pausing to check before taking actions—the core characteristics of highly sensitive individuals (Aron et al., 2012)—could prevent them from blaming others at a behavioral level. Conversely, given that individuals with low sensitivity often make decisions without using enough caution (Yano et al., 2021b), they may blame others at the behavioral level, resulting in loss of social support (Tennen and Affleck, 1990). However, it should be noted, that the aforementioned explanation cannot fully capture the mechanism through which blaming others contributes to an adaptive outcome, as this study did not measure the level of social support that the participants received.
4.2 Strengths, limitations, and future directions
This study’s main findings are as follows: (1) the strategy of blaming others has contrastive effects on changes in mental health by the level of environmental sensitivity; (2) the increase in refocusing on planning is associated with improved mental health over time only for highly sensitive individuals; (3) the strategies of rumination and catastrophizing were the most important risk factors for mental health problems, but had different effects by sensitivity level; and (4) positive reappraisal, putting into perspective, acceptance, and positive refocusing were associated with better mental health only at the beginning of the study. In short, the strengths of our results are that they reveal the individual differences in the effects of the cognitive strategies and provide evidence for the role of sensitivity in mental health research. The current findings may also provide useful information for practice. Combined with Assary et al.’s (2023) suggestion, assessment before practice enables support providers to design and implement intervention programs based on individual differences in sensitivity. For example, while the program for promoting refocusing on planning and preventing rumination could improve mental health in highly sensitive people, emphasizing the reduction in blaming others could be effective for low-sensitivity people.
Despite the aforementioned strengths, some limitations should also be acknowledged. The first is the extent to which our findings can be generalized. As previous studies have suggested that age (Garnefski and Kraaij, 2006b) and cultural factors (Potthoff et al., 2016) moderate the association between these strategies and depression or anxiety, future studies should adopt a sample that excludes Japanese university students. Furthermore, contextual factors also moderate such associations, as mentioned above (e.g., Garnefski et al., 2005; Aldao et al., 2010). While this study focused on the use of each strategy in general, it is unclear which situations our findings could be generalized to, such as final semester exams and job hunting. Second, the measurement of the cognitive emotion regulation strategies should be improved. Given the respondents’ burden, this three-wave longitudinal study used the CERQ-short (Garnefski and Kraaij, 2006a; Sakakibara, 2017) that has acceptable but lower reliability than the original version (Garnefski and Kraaij, 2006a). Considering that a recent study revised the Japanese version of the CERQ and improved its psychometric properties (Urano et al., 2022), replicating this study using the revised scale could be useful for examining the robustness of this study’s findings. Finally, as this study focused on the cognitive aspects of emotion regulation strategies, it could not investigate the mechanisms through which each cognitive strategy was linked to what behavioral strategies and (mal) adaptive outcomes. Therefore, it is necessary to clarify this mechanism, as it is expected to provide key information for designing effective intervention programs. Further investigations should reveal the adaptive process of emotion regulation based on individual differences in sensitivity considering the framework of behavioral emotion regulation, which was recently proposed by Kraaij and Garnefski (2019).
Data availability statement
The data analyzed in this study and Mplus code can be found in the Open Science Framework [https://osf.io/jymqk/?view_only=899e7768fc21410eb959fbfaa6ca7c17].
Ethics statement
The studies involving humans were approved by College of Community and Human Services, Rikkyo University. The studies were conducted in accordance with the local legislation and institutional requirements. The participants provided their written informed consent to participate in this study.
Author contributions
KY: Conceptualization, Formal analysis, Investigation, Writing – original draft, Writing – review & editing. KO: Investigation, Supervision, Writing – review & editing.
Funding
The author(s) declare that financial support was received for the research, authorship, and/or publication of this article. This study was funded by JSPS KAKENHI (Grant numbers 18 K03079, 19 J20902, and 22 K20329).
Acknowledgments
The authors thank Professor Yukitaka Ikeda (Wayo Women’s University) for his valuable advice. English language editing services were provided by Editage (www.editage.jp). This study was not registered with any repositories.
Conflict of interest
The authors declare that the research was conducted in the absence of any commercial or financial relationships that could be construed as a potential conflict of interest.
Publisher’s note
All claims expressed in this article are solely those of the authors and do not necessarily represent those of their affiliated organizations, or those of the publisher, the editors and the reviewers. Any product that may be evaluated in this article, or claim that may be made by its manufacturer, is not guaranteed or endorsed by the publisher.
Supplementary material
The Supplementary material for this article can be found online at: https://www.frontiersin.org/articles/10.3389/fpsyg.2024.1322544/full#supplementary-material
Footnotes
1. ^Pluess (2015) found that all the theories and concepts shared the perspective that people differ in sensitivity to both positive and negative influences; therefore, these were integrated into an umbrella framework.
2. ^It should be noted that an empirical study failed to replicate the existing findings (Hellwig and Roth, 2021).
3. ^Cross Marketing Inc. is one of the largest academic survey companies in Japan. At the time of the first survey (November 2020), they had about 5.4 million web survey monitors all over Japan. Many researchers have collaborated with the company to collect data (Yano et al., 2021a), suggesting the high quality and reliability of their data. The respondents were given points exchangeable for cash as rewards if they completed a questionnaire online.
4. ^Some variables (e.g., putting into perspective) did not have sufficient reliability in this study. Thus, the authors decided to employ this method to control measurement errors as far as possible. Additionally, as a preliminary analysis, the authors examined the change pattern in each variable, namely, the nonlinear model vs. the linear model (Slagt et al., 2018). The log-likelihood ratio difference tests indicated that the fit of the nonlinear model, in which the Time 2 loading from the slope factor was freely estimated, did not significantly increase from that in the linear model, in which the loading was constrained to 1, for most of the variables (see Supplementary Table S2). Therefore, the authors decided to analyze the variables assuming their linear changes.
5. ^The results of the simple slope analyses were interpreted when the region of significance on SPS was from −2 SD to +2 SD in which most of the respondents existed.
References
Aldao, A., Nolen-Hoeksema, S., and Schweizer, S. (2010). Emotion-regulation strategies across psychopathology: a meta-analytic review. Clin. Psychol. Rev. 30, 217–237. doi: 10.1016/j.cpr.2009.11.004
Aron, E. N., and Aron, A. (1997). Sensory-processing sensitivity and its relation to introversion and emotionality. J. Pers. Soc. Psychol. 73, 345–368. doi: 10.1037/0022-3514.73.2.345
Aron, E. N., Aron, A., and Jagiellowicz, J. (2012). Sensory processing sensitivity: a review in the light of the evolution of biological responsivity. Personal. Soc. Psychol. Rev. 16, 262–282. doi: 10.1177/1088868311434213
Assary, E., Krebs, G., and Eley, T. C. (2023). Practitioner review: differential susceptibility theory: might it help in understanding and treating mental health problems in youth? J. Child Psychol. Psyc. 64, 1104–1114. doi: 10.1111/jcpp.13801
Assary, E., Zavos, H. M. S., Krapohl, E., Keers, R., and Pluess, M. (2021). Genetic architecture of environmental sensitivity reflects multiple heritable components: a twin study with adolescents. Mol. Psychiatry 26, 4896–4904. doi: 10.1038/s41380-020-0783-8
Belsky, J. (1997). Variation in susceptibility to environmental influence: an evolutionary argument. Psychol. Inq. 8, 182–186. doi: 10.1207/s15327965pli0803_3
Benjamini, Y., and Hochberg, Y. (1995). Controlling the false discovery rate: a practical and powerful approach to multiple testing. J. R. Stat. Soc. B. 57, 289–300. doi: 10.1111/j.2517-6161.1995.tb02031.x
Boyce, W. T., and Ellis, B. J. (2005). Biological sensitivity to context: an evolutionary-developmental theory of the origins and functions of stress reactivity. Dev. Psychopathol. 17, 271–301. doi: 10.1017/s0954579405050145
Bratholm Wyller, H., Bratholm Wyller, V. B., Crane, C., and Gjelsvik, B. (2017). The relationship between sensory processing sensitivity and psychological distress: A model of underpinning mechanisms and an analysis of therapeutic possibilities. Scandinavian Psychologist. 4, E15.
Duncan, T. E., Duncan, S. C., and Strycker, L. A. (2013). An introduction to latent variable growth curve modeling: concepts, issues, and application. New York: Routledge.
Eisma, M. C., Janshen, A., Huber, L. F. T., and Schroevers, M. J. (2023). Cognitive reappraisal, emotional expression and mindfulness in adaptation to bereavement: a longitudinal study. Anxiety Stress Coping 36, 577–589. doi: 10.1080/10615806.2023.2165647
Furukawa, T. A., Kawakami, N., Saitoh, M., Ono, Y., Nakane, Y., Nakamura, Y., et al. (2008). The performance of the Japanese version of the K6 and K10 in the world mental health survey Japan. Int. J. Methods Psychiatr. Res. 17, 152–158. doi: 10.1002/mpr.257
Garnefski, N., Baan, N., and Kraaij, V. (2005). Psychological distress and cognitive emotion regulation strategies among farmers who fell victim to the foot-and-mouth crisis. Pers. Individ. Differ. 38, 1317–1327. doi: 10.1016/j.paid.2004.08.014
Garnefski, N., and Kraaij, V. (2006a). Cognitive emotion regulation questionnaire—development of a short 18-item version (CERQ-short). Pers. Individ. Differ. 41, 1045–1053. doi: 10.1016/j.paid.2006.04.010
Garnefski, N., and Kraaij, V. (2006b). Relationships between cognitive emotion regulation strategies and depressive symptoms: a comparative study of five specific samples. Pers. Individ. Differ. 40, 1659–1669. doi: 10.1016/j.paid.2005.12.009
Garnefski, N., Kraaij, V., and Spinhoven, P. (2001). Negative life events, cognitive emotion regulation and emotional problems. Pers. Individ. Differ. 30, 1311–1327. doi: 10.1016/S0191-8869(00)00113-6
GBD 2017 Disease and Injury Incidence and Prevalence Collaborators (2018). Disease and injury incidence and prevalence collaborators (2018). Global, regional, and national incidence, prevalence, and years lived with disability for 354 diseases and injuries for 195 countries and territories, 1990–2017: a systematic analysis for the global burden of disease study 2017. Lancet 392, 1789–1858. doi: 10.1016/S0140-6736(18)32279-7
Gignac, G. E., and Szodorai, E. T. (2016). Effect size guidelines for individual differences researchers. Pers. Individ. Differ. 102, 74–78. doi: 10.1016/j.paid.2016.06.069
Gosling, S. D., Rentfrow, P. J., and Swann, W. B. (2003). A very brief measure of the big-five personality domains. J. Res. Pers. 37, 504–528. doi: 10.1016/S0092-6566(03)00046-1
Greven, C. U., Lionetti, F., Booth, C., Aron, E. N., Fox, E., Schendan, H. E., et al. (2019). Sensory processing sensitivity in the context of environmental sensitivity: a critical review and development of research agenda. Neurosci. Biobehav. Rev. 98, 287–305. doi: 10.1016/j.neubiorev.2019.01.009
Gross, J. J. (1998). Antecedent- and response-focused emotion regulation: divergent consequences for experience, expression, and physiology. J. Pers. Soc. Psychol. 74, 224–237. doi: 10.1037/0022-3514.74.1.224
Hancock, G. R., Kuo, W.-L., and Lawrence, F. R. (2001). An illustration of second-order latent growth models. Struct. Equ. Modeling 8, 470–489. doi: 10.1207/S15328007SEM0803_7
Hellwig, S., and Roth, M. (2021). Conceptual ambiguities and measurement issues in sensory processing sensitivity. J. Res. Pers. 93:104130. doi: 10.1016/j.jrp.2021.104130
Homberg, J. R., Schubert, D., Asan, E., and Aron, E. N. (2016). Sensory processing sensitivity and serotonin gene variance: insights into mechanisms shaping environmental sensitivity. Neurosci. Biobehav. Rev. 71, 472–483. doi: 10.1016/j.neubiorev.2016.09.029
Iimura, S., Yano, K., and Ishii, Y. (2023). Environmental sensitivity in adults: psychometric properties of the Japanese version of the highly sensitive person scale 10-item version. J. Pers. Assess. 105, 87–99. doi: 10.1080/00223891.2022.2047988
Keers, R., Coleman, J. R. I., Lester, K. J., Roberts, S., Breen, G., Thastum, M., et al. (2016). A genome-wide test of the differential susceptibility hypothesis reveals a genetic predictor of differential response to psychological treatments for child anxiety disorders. Psychother. Psychosom. 85, 146–158. doi: 10.1159/000444023
Kessler, R. C., Andrews, G., Colpe, L. J., Hiripi, E., Mroczek, D. K., Normand, S. L. T., et al. (2002). Short screening scales to monitor population prevalences and trends in non-specific psychological distress. Psychol. Med. 32, 959–976. doi: 10.1017/S0033291702006074
Kibe, C., Suzuki, M., Hirano, M., and Boniwell, I. (2020). Sensory processing sensitivity and culturally modified resilience education: differential susceptibility in Japanese adolescents. PLoS One 15:e0239002. doi: 10.1371/journal.pone.0239002
Klein, A., and Moosbrugger, H. (2000). Maximum likelihood estimation of latent interaction effects with the LMS method. Psychometrika 65, 457–474. doi: 10.1007/BF02296338
Kline, R. B. (2005). Principles and practice of structural equation modeling. New York: Guilford Press.
Konrad, S., and Herzberg, P. Y. (2019). Psychometric properties and validation of a German high sensitive person scale (HSPS-G). Eur. J. Psychol. Assess. 35, 364–378. doi: 10.1027/1015-5759/a000411
Kraaij, V., and Garnefski, N. (2019). The behavioral emotion regulation questionnaire: development, psychometric properties and relationships with emotional problems and the cognitive emotion regulation questionnaire. Pers. Individ. Differ. 137, 56–61. doi: 10.1016/j.paid.2018.07.036
Lionetti, F., Klein, D. N., Pastore, M., Aron, E. N., Aron, A., and Pluess, M. (2022). The role of environmental sensitivity in the development of rumination and depressive symptoms in childhood: a longitudinal study. Eur. Child Adolesc. Psychiatry 31, 1815–1825. doi: 10.1007/s00787-021-01830-6
Lionetti, F., Pastore, M., Moscardino, U., Nocentini, A., Pluess, K., and Pluess, M. (2019). Sensory processing sensitivity and its association with personality traits and affect: a meta-analysis. J. Res. Pers. 81, 138–152. doi: 10.1016/j.jrp.2019.05.013
Maniaci, M. R., and Rogge, R. D. (2014). Caring about carelessness: participant inattention and its effects on research. J. Res. Pers. 48, 61–83. doi: 10.1016/j.jrp.2013.09.008
Maslowsky, J., Jager, J., and Hemken, D. (2015). Estimating and interpreting latent variable interactions: a tutorial for applying the latent moderated structural equations method. Int. J. Behav. Dev. 39, 87–96. doi: 10.1177/0165025414552301
Muthén, L. K., and Muthén, B. O. (2017) Mplus: statistical analysis with latent variables: user’s guide Los Angeles: Muthén & Muthén.
Nelis, D., Kotsou, I., Quoidbach, J., Hansenne, M., Weytens, F., Dupuis, P., et al. (2011). Increasing emotional competence improves psychological and physical well-being, social relationships, and employability. Emotion 11, 354–366. doi: 10.1037/a0021554
Oshio, A., Abe, S., and Cutrone, P. (2012). Development, reliability, and validity of the Japanese version of ten item personality inventory (TIPI-J). Jpn. J. Pers. 21, 40–52. doi: 10.2132/personality.21.40
Pluess, M. (2015). Individual differences in environmental sensitivity. Child Dev. Perspect. 9, 138–143. doi: 10.1111/cdep.12120
Pluess, M., Assary, E., Lionetti, F., Lester, K. J., Krapohl, E., Aron, E. N., et al. (2018). Environmental sensitivity in children: development of the highly sensitive child scale and identification of sensitivity groups. Dev. Psychol. 54, 51–70. doi: 10.1037/dev0000406
Pluess, M., and Boniwell, I. (2015). Sensory-processing sensitivity predicts treatment response to a school-based depression prevention program: evidence of vantage sensitivity. Pers. Individ. Differ. 82, 40–45. doi: 10.1016/j.paid.2015.03.011
Pluess, M., De Brito, S. A., Bartoli, A. J., McCrory, E., and Viding, E. (2022). Individual differences in sensitivity to the early environment as a function of amygdala and hippocampus volumes: an exploratory analysis in 12-year-old boys. Dev. Psychopathol. 34, 901–910. doi: 10.1017/S0954579420001698
Porru, F., Schuring, M., Bültmann, U., Portoghese, I., Burdorf, A., and Robroek, S. J. W. (2022). Associations of university student life challenges with mental health and self-rated health: a longitudinal study with 6 months follow-up. J. Affect. Disord. 296, 250–257. doi: 10.1016/j.jad.2021.09.057
Potthoff, S., Garnefski, N., Miklósi, M., Ubbiali, A., Domínguez-Sánchez, F. J., Martins, E. C., et al. (2016). Cognitive emotion regulation and psychopathology across cultures: a comparison between six European countries. Pers. Individ. Differ. 98, 218–224. doi: 10.1016/j.paid.2016.04.022
Rodas, J. A., Jara-Rizzo, M. F., Greene, C. M., Moreta-Herrera, R., and Oleas, D. (2022). Cognitive emotion regulation strategies and psychological distress during lockdown due to COVID-19. Int. J. Psychol. 57, 315–324. doi: 10.1002/ijop.12818
Roisman, G. I., Newman, D. A., Fraley, R. C., Haltigan, J. D., Groh, A. M., and Haydon, K. C. (2012). Distinguishing differential susceptibility from diathesis–stress: recommendations for evaluating interaction effects. Dev. Psychopathol. 24, 389–409. doi: 10.1017/S0954579412000065
Sakakibara, R. (2017). How does cognitive appraisal moderate the relationship between cognitive emotion regulation strategies and psychological health? Jpn. J. Soc. Psychol. 32, 163–173. doi: 10.14966/jssp.0949
Satorra, A., and Bentler, P. M. (2001). A scaled difference chi-square test statistic for moment structure analysis. Psychometrika 66, 507–514. doi: 10.1007/BF02296192
Shimizu, H. (2016). An introduction to the statistical free software HAD: suggestions to improve teaching, learning and practice data analysis. J. Media Inform. Commun. 1, 59–73.
Slagt, M., Dubas, J. S., Van Aken, M. A. G., Ellis, B. J., and Deković, M. (2018). Sensory processing sensitivity as a marker of differential susceptibility to parenting. Dev. Psychol. 54, 543–558. doi: 10.1037/dev0000431
Tennen, H., and Affleck, G. (1990). Blaming others for threatening events. Psychol. Bull. 108, 209–232. doi: 10.1037/0033-2909.108.2.209
Urano, Y., Kobayashi, R., and Sakakibara, R. (2022). Revision and validation of the Japanese-version cognitive emotion regulation questionnaire: psychometric properties and measurement invariance across gender. Cogent Psychol. 9:2064790. doi: 10.1080/23311908.2022.2064790
Yano, K., Endo, S., Kimura, S., and Oishi, K. (2021a). Effective coping strategies employed by university students in three sensitivity groups: a quantitative text analysis. Cogent Psychol. 8:1988193. doi: 10.1080/23311908.2021.1988193
Yano, K., Kase, T., and Oishi, K. (2021b). Sensory processing sensitivity moderates the relationships between life skills and depressive tendencies in university students. Jpn. Psychol. Res. 63, 152–163. doi: 10.1111/jpr.12289
Keywords: environmental sensitivity, sensory processing sensitivity, mental health, emotion regulation, longitudinal study
Citation: Yano K and Oishi K (2024) Individual differences in environmental sensitivity: associations between cognitive emotion regulation and mental health. Front. Psychol. 15:1322544. doi: 10.3389/fpsyg.2024.1322544
Edited by:
Karl Schweizer, Goethe University Frankfurt, GermanyReviewed by:
Paweł Larionow, Kazimierz Wielki University, PolandFrancesca Lionetti, University of Studies G. d'Annunzio Chieti and Pescara, Italy
Copyright © 2024 Yano and Oishi. This is an open-access article distributed under the terms of the Creative Commons Attribution License (CC BY). The use, distribution or reproduction in other forums is permitted, provided the original author(s) and the copyright owner(s) are credited and that the original publication in this journal is cited, in accordance with accepted academic practice. No use, distribution or reproduction is permitted which does not comply with these terms.
*Correspondence: Kosuke Yano, a29zdWtlLnlhbm8xMDEyQGdtYWlsLmNvbQ==