- 1Virtual Reality and Nature Lab, Department of Parks, Recreation and Tourism Management, Clemson University, Clemson, SC, United States
- 2Environment and Sustainability Research Initiative, Khulna, Bangladesh
- 3Environmental Science Discipline, Life Science School, Khulna University, Khulna, Bangladesh
- 4Department of Psychology and Neuroscience, Duke University, Durham, NC, United States
Generative Artificial Intelligence (GAI) is an emerging and disruptive technology that has attracted considerable interest from researchers and educators across various disciplines. We discuss the relevance and concerns of ChatGPT and other GAI tools in environmental psychology research. We propose three use categories for GAI tools: integrated and contextualized understanding, practical and flexible implementation, and two-way external communication. These categories are exemplified by topics such as the health benefits of green space, theory building, visual simulation, and identifying practical relevance. However, we also highlight the balance of productivity with ethical issues, as well as the need for ethical guidelines, professional training, and changes in the academic performance evaluation systems. We hope this perspective can foster constructive dialogue and responsible practice of GAI tools.
1 Introduction
Generative Artificial Intelligence (GAI) has sparked enthusiasm and concern in how we conceive knowledge creation (Nature Editorials, 2023; Stokel-Walker and Van Noorden, 2023). Generated content includes language and text but also images, audio, video, and 3D objects (Supplementary Table S1). Their applications in higher education have raised interest and concerns from educators (Chen et al., 2020), including the UNESCO (Sabzalieva and Valentini, 2023). The applications in scientific writing (Lund et al., 2023) and various scientific domains such as healthcare research (Dahmen et al., 2023; Sallam, 2023), environmental research (Agathokleous et al., 2023; Zhu et al., 2023), and environmental planning and design (Fernberg and Chamberlain, 2023; Wang et al., 2023) have also been discussed. As with all powerful tools, GAI offers opportunities and poses risks depending on the user’s knowledge and choices when using the tool (Dwivedi et al., 2023). For example, ChatGPT’s responses can sound plausible for a research paper but may not match the expected precise and accuracy level for scientific discourse. Therefore, it is time to ask what role GAI can play in environmental psychology. This article aims to prepare the field of environmental psychology to take advantage of GAI while, ideally, avoiding the pitfalls.
Environmental psychology investigates the interaction between human and socio-physical environments, with a practical orientation to solving community-environmental problems using psychological research tools and insights (Stokols, 1978). Given this focus, the field has several challenges that GAI tools might assist. First, human environmental experience depends on the person and the physical, social, and situational contexts (Lazarus and Launier, 1978; Altman and Rogoff, 1987; Hartig, 1993; Wapner and Demick, 2002). Due to the field’s preference for generalized, objective knowledge (Seamon, 1982; Franck, 1987), and researchers’ reliance on existing literature instead of learning from real-life experiences and communities, it struggles to cater to diverse human experiences. Second, environmental psychology’s interdisciplinary nature leads to knowledge gaps for researchers trained in specific disciplines. Researchers may miss advancements in related fields or be unaware of older literature, creating barriers to fully informed research and application (Rapoport, 1997). Third, there can be a lack of alignment between empirical research and practice, especially in environmental planning and design. This may be due to communication gaps (Franck, 1987), lack of integrated perspectives (Hillier et al., 1972), and the complex interactions between factors in practice contexts (Alexander, 1964; Altman and Rogoff, 1987). Fourth, researchers face constraints from the techniques and technologies they can access for applying the appropriate method to a research question. Mastering multiple techniques can be overwhelming and cost-prohibitive, and the opportunity to collaborate, practice, and get feedback from others is not always available.
The issues above reflect the limited capacity of human researchers and institutional research structures. We suggest that GAI tools may help fill the various gaps, as they can connect board knowledge to specific contexts, foster interdisciplinary learning through interactive conversations, and assist with various procedures and techniques. To illustrate how GAI can be used to advance environmental psychology as a field, we propose three areas in the research process where GAI tools can be beneficial: (1) integrated and contextualized understanding, (2) effective and flexible implementation, and (3) two-way communication between researchers and external audiences (Figure 1). These three areas cover the various research activities in the research process, such as idea generation, planning and decision support, development of stimuli, data analysis, and communication of findings. To illustrate how these GAI applications could be put into practice, we provide specific examples of our interactions with ChatGPT (see Supplementary material 1–6).
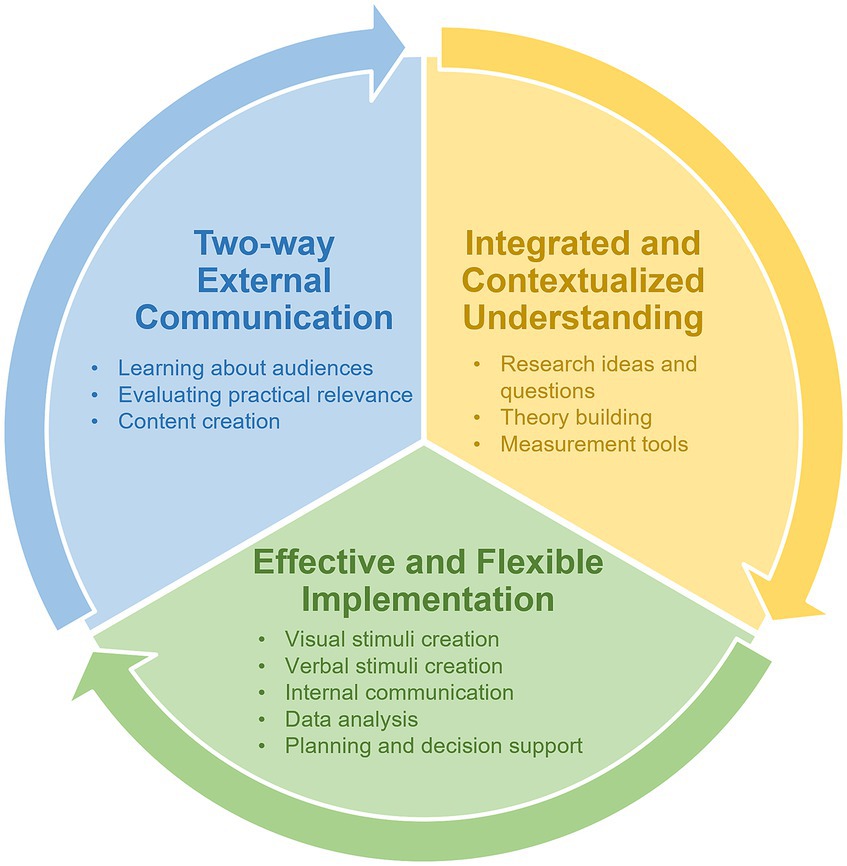
Figure 1. Categories of generative artificial intelligence (GAI) applications for advancing environmental psychology research.
2 Integrated and contextualized understanding
Environmental psychology researchers benefit from continual learning, building, and expanding integrated and contextualized understanding of human-environment interaction. Large language models can assist with this pursuit throughout the research process, from brainstorming research ideas, evaluating potential research factors, collecting examples, and interpreting research findings.
2.1 Research question formulation
Generative Artificial Intelligence can accelerate the idea-generation process by connecting a loosely described phenomenon or assumption to a list of scientific concepts and theories across different fields. GAI can also help researchers better initiate a study from the perspective of helping to build a knowledge map comprehensively. For example, ChatGPT could assist a researcher interested in human-nature connections by framing questions from the relevant perspectives of psychology, social science, environment, tourism, education, planning, and health science (Ives et al., 2017; Hallsworth et al., 2023). In addition, ChatGPT can provide a matched level of competence to explore unfocused areas (i.e., helping partitioners and researchers to learn other research areas). Therefore, researchers may more likely to identify important but understudied factors and relationships (Supplementary material 1). For example, extreme heat may limit or dissolve how well green space supports healthy behaviors and psychological restoration, though the prevailing modeling has focused on mitigating urban heat islands outside of heat waves (Li et al., 2023).
2.2 Theory building
ChatGPT can help perform three theory-building processes described by Walker and Avant (2014), which are derivation (making an analogy and borrowing a concept), synthesis (combining information into a concept), and analysis (breaking down a concept). These processes are more effective when ChatGPT also generates examples and scenarios as hypothetical empirical materials. Researchers can flexibly use ChatGPT’s capacities, including the three strategies we described here. One is an inductive approach, where researchers use ChatGPT to generate examples with social, physical, situational contexts and/or personal factors and abstract and synthesize those examples. This is particularly useful for an initial phase or when empirical data is unavailable. For example, Stokols (2000) has emphasized the research gap in identifying high-impact social-physical circumstances that enhance or constrain human stress coping and functioning. Researchers can use ChatGPT to generate many examples instantly and identify the key factors or groups (Supplementary material 2). Another deductive strategy can be refining and breaking down an existing concept and using hypothetical examples to test the tentative subconcepts. For example, breaking down the concept of compatibility in attention restoration theory (Kaplan, 1995) may help connect it to various environments and human goals (Supplementary material 2). Third but not least, many theory-building approaches involve purposive sample collection to extend or corroborate a theory (i.e., theoretical sampling; Eisenhardt and Graebner, 2007; Corbin and Strauss, 2015). Environmental psychology is often about and can be challenged by everyday experiences. One can use ChatGPT to deliberately collect hypothetical everyday environmental experiences that may contradict a theory. Such counterexamples can be abstracted or synthesized to identify boundary conditions or new mediators and moderators.
2.3 Development of measurement tools
Generative Artificial Intelligence tools can ease the item pool generation of psychometric scales and environmental audit tools. One can use ChatGPT to generate items or indicators for a concept or phenomenon that is described broadly or precisely. Alternatively, one can first explore the potential construct structure using the inductive and deductive approaches described in theory building, and generate the item pool based on the dimensions. ChatGPT’s large knowledge base and ability to generate many items may help improve content validity. For specific populations or environments (e.g., children, neighborhoods, and urban parks), ChatGPT can also help identify specific factors, assess the suitability of general or related measurement tools, and identify gaps or mismatches. This might improve the relevance of the measurements for the participants and environments. Moreover, ChatGPT’s ability to apply general knowledge and logical reasoning can help explore the potential dimensions of a construct from a list of indicators (Supplementary material 3). By repeating this action, items often categorized into different categories over time may be less valid. Although this cannot replace expert review, it can provide a low-cost check of the quality of ongoing work or existing measurement tools developed using a rational or theoretical approach.
3 Effective and flexible implementation
Implementation plays an important role in environmental psychology, but technical issues are a major barrier for researchers implementing their research ideas. GAI can mitigate such barriers and allow researchers to focus more on the research itself. In addition to the implementation aspects common to the social and behavioral sciences, such as institutional review boards (IRBs), recruitment, surveys, interviews, and statistics, environmental psychology has a strong focus on field observation and the creation of environmental stimuli.
3.1 Visual stimuli generation
Manipulating factors of interest and controlling confounders is crucial for experiment validity. However, learning the required design knowledge and media creation skills can be prohibitive for researchers with non-environmental design backgrounds (Stamps, 2016). Image generators such as DALLE-2, Midjourney, and Stable Diffusion enable rapid generation and modification of imagery to align with the research question (i.e., varying an independent variable in the environment). For example, a Stable Diffusion-based tool, Skybox AI, could generate a VR environment within seconds with a short prompt (Figure 2). Stable Diffusion can also enable style transformation, such as changing the season, time, architectural style and elements while keeping the spatial layout. 3D content generators such as Point E and Shape E can help build virtual 3D Objects. ChatGPT and GitHub Copilot can further help with computer programming tasks in developing virtual scenes in game engines (e.g., Unity 3D or Unreal) for advanced and interactive simulation.
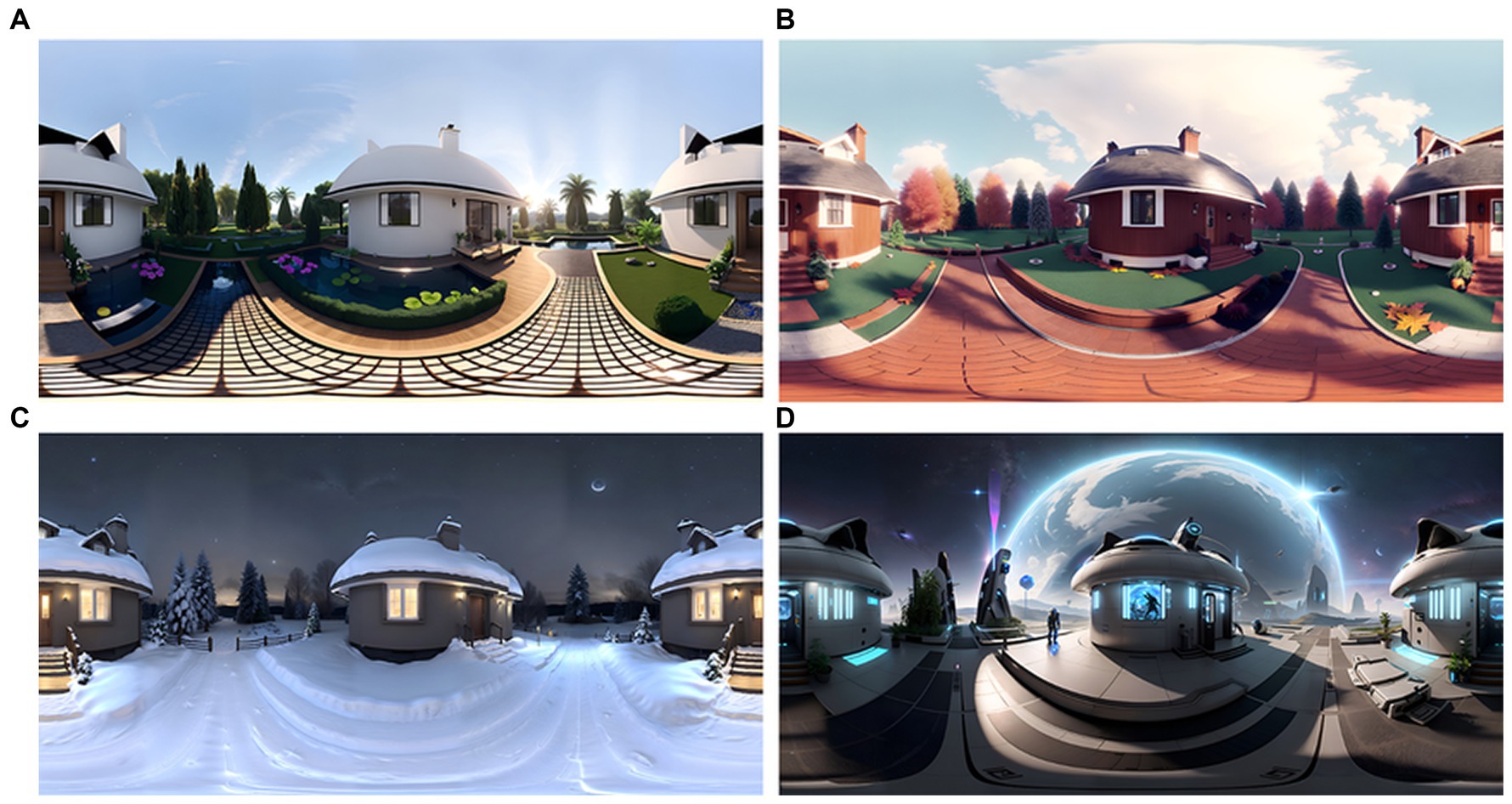
Figure 2. Example of AI generated virtual reality environment form the same structure. Prompts are (A) “a summer scene outside a lovely house, realistic”; (B) “a fall scene outside a lovely house with leave fall on the ground, realistic”; (C) “a winter scene outside a lovely house, with less light and a clear night sky, realistic”; and (D) “a space scene outside a lovely house on the moon, Earth in the distance, Sci-Fi.” All variants used the “Mixed Mode” option, which will generate a new image based on the image of the first prompt. Retrieved from Skybox AI, version beta 0.4.2. on May 31, 2023.
3.2 Verbal stimuli generation
Large language models like ChatGPT can create high-quality verbal stimuli with specific contents or factors, communication purposes, and rhetoric considerations. Several research areas, such as decision-making and information-seeking in travel, health, and pro-environmental behavior, could require more complex stimuli beyond visual perception. In this case, researchers can use text generators to create voiceover scripts in the intervention that require specific tones, inclusive languages, and clear narratives. For example, translating scripts for non-native English speakers can lead to misunderstandings and measurement errors when the local dialect is ignored (Solano-Flores, 2006), which could be assisted by GAI. These tools can also create a series of text-based scenarios (vignettes) for priming, contextualizing, and incorporating different parameters for complex conditions (Aguinis and Bradley, 2014). This technique is frequently employed for forecasted scenarios and recreation behavior, pro-environmental behavior, and acceptance of sustainable design (Jaung, 2022; Hu and Shealy, 2023; Urban et al., 2023). For audio narration, advanced text-to-speech tools such as Vall E can mimic tones and emotions for languages, which can be used to test narration scripts, in intervention materials, or as experiment instructions.
3.3 Internal communication with participants and institutional review boards
Large language models can help edit language in materials presented to participants. For example, ChatGPT can be used to develop engaging and easily understandable recruitment materials and easy-to-understand experimental instructions. Such language models can also reduce issues such as uncommon or obsolete words, non-inclusive language, and leading questions in existing survey batteries. In verbal-based communication, those tools may improve communication with special populations, such as children, elderly individuals, and people with disabilities and mental health conditions, ensuring understanding, inclusiveness, and compliance. IRB-related documents and materials require specific structures, tones, and an understanding of ethical issues. AI tools might also explain ethical concerns and present potential solutions. It can transfer existing materials into new structures and formats, after cross-checking with specific institutional policies. See Supplementary material 4 for using ChatGPT transferring a description of the procedure from a citation into a training manual, an IRB application, and a consent form.
3.4 Basic data analysis
Generative Artificial Intelligence tools can facilitate basic quantitative methods such as the use of R in data cleaning, regression, and factor analysis. ChatGPT can interpret statistical software outputs or model results and suggest visualization options. These tools can assist researchers with qualitative or environmental backgrounds in conducting quantitative or mixed-methods research. Qualitative analysis may benefit less from GAI tools because most qualitative approaches need researchers’ knowledge and experience to interpret data meaning and significance (Creswell and Poth, 2017). However, GAI tools may perform minor roles such as suggesting codes to merge, checking code abstraction levels, and interpreting ambiguous sentences. Also, qualitative data analysis software such as MAXQDA provides a GAI tool1 for defining codes based on related quotations. QDA software may also offer an auto-coding function based on regular expression, which is suitable for the exploration of data or more descriptive research. ChatGPT can be used to generate regular expressions to identify objects or features in different texts.
3.5 Computer programming
In addition, computational thinking and programming are trending skills for researchers to effectively and flexibly implement data-driven methods and analysis (Chen and Wojcik, 2016). Environmental psychology researchers may increasingly feel they need to learn and apply “advanced” data analysis skill sets, including image, spatial and spatial–temporal data, network data, natural language, or physiological data, in their research. GAI tools excel in assisting the process of program learning and analysis with natural and instant interaction. For example, ChatGPT and GitHub Copilot could help explain code, generate queries, identify relevant coding libraries and functions, explain errors, assisting debug, or write prototype coding for future studies in almost every coding language, such as R, Python, Matlab, etc. (Biswas, 2023; Feng et al., 2023; Rahman and Watanobe, 2023). These capabilities help researchers with both limited and massive coding experience to speed up the research data cleaning, analysis, and visualization process.
3.6 Planning and decision support
Successful study implementation requires careful planning, effective problem-solving, and informed decision-making. While support from colleagues, committee members, and advisors is invaluable, such mentors have limited availability. GAI tools’ knowledge base and planning capacities (Wu et al., 2023) can help researchers evaluate methodological choices and trade-offs between them. For example, an environmental psychologist could prompt ChatGPT to generate approaches with higher vs. lower levels of experimental control and ecological validity in a healthcare facility wayfinding study using virtual reality (Joseph et al., 2020). When used in dealing with planning and problem-solving, ChatGPT can facilitate describing an entire procedure and adding details, analyzing the cause of problems, and planning for worst-case scenarios. This can be applied to a large variety of data collection procedures, such as experimental sessions, equipment use, and focus groups. Another example is field observation in urban public spaces (Sussman, 2016). We found that ChatGPT could describe potential iterations in refining observational protocol (Supplementary material 5). It also identified potential issues such as public space being unexpectedly closed, equipment malfunctions or being stolen, failure to adequately familiarize with the site, and notes or data being damaged in transportation. Then one can use ChatGPT to draft a comprehensive logistical checklist and preparation action list.
4 Two-way communication between researchers and external audiences
Communicating science with practitioners, policymakers, and the general public requires unique skill sets. This is especially true in environmental psychology, where from its beginnings, this field of research has had an applied emphasis that would benefit from collaboration with practitioners (Wohlwill, 1970; Stokols, 1978, 1995; Devlin, 2018). Communication requires advanced knowledge of external audiences and is not a one-directional stream of information (Ham, 1992). Multi-perspective thinking helps ensure messages are received as intended, and that feedback is considered (Blythe, 2010). GAI tools can assist with this through their large knowledge base that encompasses scientific, professional, and everyday content in addition to the ability to explain audience characteristics, identify relevant scientific concepts, and demonstrate practical relevance.
4.1 Learning about audiences and stakeholders
Generative Artificial Intelligence tools can suggest possible expectations and needs of diverse external audiences. Environmental psychology can have implications for designers, planners, healthcare professionals, business owners, residents, land managers, and many other fields. Researchers may be familiar with select professions and communities but are unlikely to consider and know all relevant stakeholders. For example, scholars familiar with speaking with conservation biologists may use an entirely different lexicon about urban greening than scholars trained in public and environmental policy (Vogt, 2018). ChatGPT can be used to identify new stakeholders for research studies, recommend how to communicate with these audiences, learn about their needs and concerns, and provide nuanced “pitches” to capture and maintain stakeholders’ interest (Supplementary material 6).
4.2 Evaluating practical relevance
The broad base of procedure knowledge in GAI tools can facilitate identifying relevant practical actions that emerge from research findings. These actions can be useful in suggesting practical implications in scientific publications and practical guidelines. The broad knowledge base can also help assess the feasibility and requirements for a proposed action/approach, provide alternatives, and discuss alignment with practitioner needs, resources, and constraints. These tools can also be used to generate ideas to discuss directly with practitioners. For example, natural sounds may have clear applicability to environmental psychologists interested in restorative landscapes but also have widescale applicability—though perhaps less known to these environmental psychologists—for measuring biodiversity and urban ecology (Fleming et al., 2023).
4.3 Communication with the media
In addition to the uses of GAI tools to assist with scientific writing (Buriak et al., 2023; Dwivedi et al., 2023; Lund et al., 2023), these tools can translate scientific writing to social media posts, blogs, storyboards for short videos, technical reports, conference abstracts, and many other content types (Supplementary material 7). The complex concepts and technical language in the original text can be simplified; GAI tools create examples, analogies, and real-world case studies to explain concepts and engage audiences. These tools can also articulate the procedures and steps to implement practical guidance and takeaways from research findings. The text from ChatGPT can emphasize the important implications and limitations for practitioners, policymakers, and other stakeholders and can translate the language into the appropriate tone for a specific audience. For a practical guide on ChatGPT and social media content creation, see Stone (2023).
5 Responsible use of GAI in environmental psychology research
Generative Artificial Intelligence tools can have many benefits, but some uses can reduce these benefits or even threaten the value of their research applications. Relying on ChatGPT and other language models for research ideas or a conceptual understanding may compromise originality and critical thinking, or even lead to false information and plagiarism (Beerbaum, 2023; Polonsky and Rotman, 2023; Rahimi and Talebi Bezmin Abadi, 2023; Xames and Shefa, 2023). ChatGPT’s responses can be influenced by how prompts are formulated, such as language ambiguity, complexity level, and clarity of user’s intentions. It is also susceptible to leading questions and can generate misinformation with questions beyond its knowledge base, for example, questions about underrepresented countries and regions. Such complexities of this tool operation can limit the tool’s value. Also, ChatGPT is trained on publicly available datasets. It may copy phrases from the trained documents without giving credit, thus leading to plagiarism. Approximately half of the citations provided by ChatGPT are fabricated, depending on the model version and the type of reference (Bhattacharyya et al., 2023; Walters and Wilder, 2023). Therefore, researchers should always evaluate and fact-check the output to avoid misinformation or plagiarism (Kitamura, 2023). In addition, reliance on GAI in literature searches and idea generation without other sources may constrain creativity (Dwivedi et al., 2023) or impede scholars from developing a foundational knowledge base of a research area.
Reliance on GAI tools to enhance productivity in research can also compromise research validity and the value of research findings. When motivated to publish and seek grants, researchers may use GAI to accelerate their productivity at the expense of originality and professional growth (Dwivedi et al., 2023). While using GAI undeniably boosts productivity, it can bias topic and methodology selection toward those it can assist. These could, in turn, decrease the validity and reliability of research findings, and undercut the benefit of effective and flexible implementation from GAI tools. Furthermore, increased use of GAI for productivity may entrench competition between researchers or institutions regarding publication output, increasing the share of “fast food” publications (van Dalen and Henkens, 2012) with less contribution and significance for understanding human-environment problems.
Therefore, we support the many calls to action from scholars and publishers to create ethical guidelines for GAI use (Dwivedi et al., 2023; Graf and Bernardi, 2023; Liebrenz et al., 2023; Lund et al., 2023; Rahimi and Talebi Bezmin Abadi, 2023). To maintain research originality and significance, it is important to incorporate diverse sources and find a balance between GAI automation and researchers’ thinking. We can refer to the conceptual model of levels and stages of automation from human factor psychology (Parasuraman et al., 2000; Parasuraman and Wickens, 2008), which includes a continuum of automation levels and four automation stages from information-related (information acquisition and information analysis) to action-related (decision selection and action implementation). We recommend using GAI tools for limited stages and at a lower level of automation for the information-related stages. For example, one may select an existing perspective or technique and have ChatGPT facilitate application to new topics (information analysis) or have ChatGPT suggest programming codes and manually test the codes (decision selection). By contrast, using ChatGPT to write a paragraph with references compromises originality (high automation at multiple stages).
Institutions should integrate GAI into professional training. For example, the book by An (2023) provides beginner-friendly guidance on ChatGPT for environmental and health behavior research. Training may also cover advanced skills of GAI, such as prompt engineering, retrieval from files, and fine-tuning. Prompt engineering has been recommended as the initial attempt to obtain a more accurate output (OpenAI, 2024c). It involves improving prompts by providing context, defining expected results, and using intermediate steps for complex tasks (Nori et al., 2023; Wei et al., 2023; White et al., 2023; OpenAI, 2024d). Supplementary material 1, for example, explored the impacts of heat events on greenspace health benefits with an interest in comprehensive, design-oriented answers. Therefore, it set the context of “public health and environmental design” and the expected result structure of “physical, social, and personal contexts.” In addition, uploading files allows the model to retrieve relevant contextual information from a data source outside its training dataset (Retrieval Augmented Generation; OpenAI, 2024a,b,e), which is supported by GPT-4 and the OpenAI API. For example, reference measurement tools may be uploaded in the measurement tool creation example (Supplementary material 3), and a related research proposal can be uploaded for creating field observation protocols (Supplementary material 5). Fine-tuning adapts a model for focused and specialized tasks such as rating, sentiment analysis, FAQs, or generating structured results (Google, 2024; OpenAI, 2024c). This process involves a simplified training process (“tuning”) with specific input and output examples, rather than just supplying instructions (prompt engineering) or unstructured data (retrieval). Thus, it offers the largest level of customization but requires the researcher’s technical capabilities.
We also argue that institutions need to update their policies to promote ethical GAI practices and maintaining academic integrity. Without highlighting the ethical GAI use, scholars and committees involved with tenure and promotion might associate GAI with neutral or negative connotations (i.e., hallucinating false information and biases in training data) instead of their tremendous values to promote scholarship and produce knowledge. Additionally, it is important to recognize topics and methods that are less likely to be promoted by GAI, such as qualitative approaches seeking to deeply understand the lived experiences of individuals and groups. Correspondingly, we see an urgency to modify performance evaluation systems related to publications, funding, and career advancement to protect researchers who use GAI tools in pursuit of increasing the quality and transparency of their research, not only the quantity.
Data availability statement
The original contributions presented in the study are included in the article/Supplementary material, further inquiries can be directed to the corresponding author.
Author contributions
SY: Conceptualization, Investigation, Project administration, Visualization, Writing – original draft, Writing – review & editing. FL: Conceptualization, Investigation, Visualization, Writing – original draft, Writing – review & editing. MBr: Conceptualization, Investigation, Project administration, Writing – original draft, Writing – review & editing. MBa: Conceptualization, Investigation, Writing – review & editing. KZ: Conceptualization, Writing – review & editing. OM: Writing – review & editing. MP: Writing – review & editing. AR: Writing – review & editing.
Funding
The author(s) declare that no financial support was received for the research, authorship, and/or publication of this article.
Conflict of interest
The authors declare that the research was conducted in the absence of any commercial or financial relationships that could be construed as a potential conflict of interest.
The author(s) declared that they were an editorial board member of Frontiers, at the time of submission. This had no impact on the peer review process and the final decision.
Publisher’s note
All claims expressed in this article are solely those of the authors and do not necessarily represent those of their affiliated organizations, or those of the publisher, the editors and the reviewers. Any product that may be evaluated in this article, or claim that may be made by its manufacturer, is not guaranteed or endorsed by the publisher.
Supplementary material
The Supplementary material for this article can be found online at: https://www.frontiersin.org/articles/10.3389/fpsyg.2024.1295275/full#supplementary-material
Footnotes
References
Agathokleous, E., Saitanis, C. J., Fang, C., and Yu, Z. (2023). Use of ChatGPT: What does it mean for biology and environmental science? Sci. Total Environ. 888:164154. doi: 10.1016/j.scitotenv.2023.164154
Aguinis, H., and Bradley, K. J. (2014). Best practice recommendations for designing and implementing experimental vignette methodology studies. Organ. Res. Methods 17, 351–371. doi: 10.1177/1094428114547952
Altman, I., and Rogoff, B. (1987). “World views in psychology: trait, interactional, organismic, and transactional perspectives” in Handbook of Environmental Psychology. eds. D. Stokols and I. Altman (New York, NY: Willey)
An, R. (2023). Supercharge Your Research Productivity with ChatGPT: A Practical Guide. Independently published.
Beerbaum, D. O. (2023). Generative Artificial Intelligence (GAI) Software—Assessment on Biased Behavior. SSRN [Preprint]. doi: 10.2139/ssrn.4386395
Bhattacharyya, M., Miller, V. M., Bhattacharyya, D., Miller, L. E., Bhattacharyya, M., Miller, V., et al. (2023). High rates of fabricated and inaccurate references in ChatGPT-generated medical content. Cureus 15:e39238. doi: 10.7759/cureus.39238
Biswas, S. (2023). Role of ChatGPT in computer programming.: ChatGPT in computer programming. Mesopotam. J. Comput. Sci. 2023, 8–16. doi: 10.58496/MJCSC/2023/002
Blythe, J. (2010). Trade fairs as communication: a new model. J. Bus. Ind. Mark. 25, 57–62. doi: 10.1108/08858621011009155
Buriak, J. M., Akinwande, D., Artzi, N., Brinker, C. J., Burrows, C., Chan, W. C. W., et al. (2023). Best practices for using AI when writing scientific manuscripts. ACS Nano 17, 4091–4093. doi: 10.1021/acsnano.3c01544
Chen, E. E., and Wojcik, S. P. (2016). A practical guide to big data research in psychology. Psychol. Methods 21, 458–474. doi: 10.1037/met0000111
Chen, X., Xie, H., Zou, D., and Hwang, G.-J. (2020). Application and theory gaps during the rise of artificial intelligence in education. Comput. Educ. Artific. Intellig. 1:100002. doi: 10.1016/j.caeai.2020.100002
Corbin, J. M., and Strauss, A. L. (2015). Basics of Qualitative Research: Techniques and Procedures for Developing Grounded Theory. 4th Edn. Los Angeles, CA: Sage.
Creswell, J. W., and Poth, C. N. (2017). Qualitative Inquiry & Research Design: Choosing Among Five Approaches. 4th Edn. Los Angeles, CA: Sage.
Dahmen, J., Kayaalp, M. E., Ollivier, M., Pareek, A., Hirschmann, M. T., Karlsson, J., et al. (2023). Artificial intelligence bot ChatGPT in medical research: the potential game changer as a double-edged sword. Knee Surg. Sports Traumatol. Arthrosc. 31, 1187–1189. doi: 10.1007/s00167-023-07355-6
Devlin, A. S. (2018). “1—Concepts, theories, and research approaches” in Environmental Psychology and Human Well-Being. ed. A. S. Devlin (Academic Press), 1–28.
Dwivedi, Y. K., Kshetri, N., Hughes, L., Slade, E. L., Jeyaraj, A., Kar, A. K., et al. (2023). "So what if ChatGPT wrote it?" Multidisciplinary perspectives on opportunities, challenges and implications of generative conversational AI for research, practice and policy. Int. J. Inf. Manag. 71:102642. doi: 10.1016/j.ijinfomgt.2023.102642
Eisenhardt, K. M., and Graebner, M. E. (2007). Theory building from cases: opportunities and challenges. Acad. Manag. J. 50, 25–32. doi: 10.5465/amj.2007.24160888
Feng, Y., Vanam, S., Cherukupally, M., Zheng, W., Qiu, M., and Chen, H. (2023). “Investigating Code Generation Performance of Chat-GPT with Crowdsourcing Social Data” in Proceedings of the 47th IEEE Computer Software and Applications Conference, 1–10.
Fernberg, P., and Chamberlain, B. (2023). Artificial intelligence in landscape architecture: a literature review. Landscape J. Design Plan. Manag. Land 42, 13–35. doi: 10.3368/lj.42.1.13
Fleming, G. M., ElQadi, M. M., Taruc, R. R., Tela, A., Duffy, G. A., Ramsay, E. E., et al. (2023). Classification and ecological relevance of soundscapes in urban informal settlements. People Nat. 5, 742–757. doi: 10.1002/pan3.10454
Franck, K. (1987). “Phenomenology, positivism, and empiricism as research strategies in environment—behavior research and in design” in Advances in Environment, Behavior, and Design. eds. E. H. Zube and G. T. Moore (New York, NY: Springer), vol. 1, 59–67.
Google (2024). Tune language foundation models—Generative AI on Vertex AI. Google Cloud. Available at: https://cloud.google.com/vertex-ai/generative-ai/docs/models/tune-models
Graf, A., and Bernardi, R. E. (2023). ChatGPT in research: balancing ethics, transparency and advancement. Neuroscience 515, 71–73. doi: 10.1016/j.neuroscience.2023.02.008
Hallsworth, J. E., Udaondo, Z., Pedrós-Alió, C., Höfer, J., Benison, K. C., Lloyd, K. G., et al. (2023). Scientific novelty beyond the experiment. Microb. Biotechnol. 16, 1131–1173. doi: 10.1111/1751-7915.14222
Ham, S. (1992). Environmental interpretation: a practical guide for people with big ideas and small budgets. Golden, CO: North American Press.
Hartig, T. (1993). Nature experience in transactional perspective. Landsc. Urban Plan. 25, 17–36. doi: 10.1016/0169-2046(93)90120-3
Hillier, B., Musgrove, J., and O'Sullivan, P. (1972). “Knowledge and design” in Environmental Design: Research and Practice: Proceedings. ed. W. J. Mitchell.
Hu, M., and Shealy, T. (2023). Priming the public to construct preferences for sustainable design: A discrete choice model for green infrastructure. J. Environ. Psychol. 88:102005. doi: 10.1016/j.jenvp.2023.102005
Ives, C. D., Giusti, M., Fischer, J., Abson, D. J., Klaniecki, K., Dorninger, C., et al. (2017). Human–nature connection: a multidisciplinary review. Curr. Opin. Environ. Sustain. 26–27, 106–113. doi: 10.1016/j.cosust.2017.05.005
Jaung, W. (2022). Digital forest recreation in the metaverse: opportunities and challenges. Technol. Forecast. Soc. Chang. 185:122090. doi: 10.1016/j.techfore.2022.122090
Joseph, A., Browning, M. H., and Jiang, S. (2020). Using immersive virtual environments (IVEs) to conduct environmental design research: A primer and decision framework. Health Environ. Res. Design J. 13, 11–25. doi: 10.1177/1937586720924787
Kaplan, S. (1995). The restorative benefits of nature: Toward an integrative framework. J. Environ. Psychol. 15, 169–182. doi: 10.1016/0272-4944(95)90001-2
Kitamura, F. C. (2023). ChatGPT is shaping the future of medical writing but still requires human judgment. Radiology 307:e230171. doi: 10.1148/radiol.230171
Lazarus, R. S., and Launier, R. (1978). “Stress-related transactions between person and environment” in Perspectives in Interactional Psychology. eds. L. A. Pervin and M. Lewis (US: Springer), 287–327.
Li, H., Browning, M. H. E. M., Dzhambov, A. M., Mainuddin Patwary, M., and Zhang, G. (2023). Potential pathways of association from green space to smartphone addiction. Environ. Pollut. 331:121852. doi: 10.1016/j.envpol.2023.121852
Liebrenz, M., Schleifer, R., Buadze, A., Bhugra, D., and Smith, A. (2023). Generating scholarly content with ChatGPT: Ethical challenges for medical publishing. Lancet Digit. Health 5, e105–e106. doi: 10.1016/S2589-7500(23)00019-5
Lund, B. D., Wang, T., Mannuru, N. R., Nie, B., Shimray, S., and Wang, Z. (2023). ChatGPT and a new academic reality: Artificial Intelligence-written research papers and the ethics of the large language models in scholarly publishing. J. Assoc. Inf. Sci. Technol. 74, 570–581. doi: 10.1002/asi.24750
Nature Editorials (2023). Tools such as ChatGPT threaten transparent science; here are our ground rules for their use. Nature 613:612. doi: 10.1038/d41586-023-00191-1
Nori, H., King, N., McKinney, S. M., Carignan, D., and Horvitz, E. (2023). Capabilities of GPT-4 on medical challenge problems. arXiv [Preprint]. doi: 10.48550/arXiv.2303.13375
OpenAI. (2024a). Embeddings—OpenAI Documentation. OpenAI Platform. Available at: https://platform.openai.com/docs/guides/embeddings
OpenAI (2024b). File uploads FAQ. OpenAI Help Center. Available at: https://help.openai.com/en/articles/8555545-file-uploads-faq
OpenAI (2024c). Fine-tuning. OpenAI Platform. Available at: https://platform.openai.com/docs/guides/fine-tuning
OpenAI. (2024d). Prompt engineering—OpenAI Documentation. OpenAI Platform. Available at: https://platform.openai.com/docs/guides/prompt-engineering
OpenAI (2024e). Retrieval Augmented Generation (RAG) and Semantic Search for GPTs. OpenAI Help Center. Available at: https://help.openai.com/en/articles/8868588-retrieval-augmented-generation-rag-and-semantic-search-for-gpts
Parasuraman, R., Sheridan, T. B., and Wickens, C. D. (2000). A model for types and levels of human interaction with automation. IEEE Trans. Syst. Man Cybern. Syst. Hum. 30, 286–297. doi: 10.1109/3468.844354
Parasuraman, R., and Wickens, C. D. (2008). Humans: still vital after all these years of automation. Hum. Fact. 50, 511–520. doi: 10.1518/001872008X312198
Polonsky, M. J., and Rotman, J. D. (2023). Should artificial intelligent agents be your co-author? arguments in favour, informed by ChatGPT. Australas. Mark. J. 31, 91–96. doi: 10.1177/14413582231167882
Rahimi, F., and Talebi Bezmin Abadi, A. (2023). ChatGPT and publication ethics. Arch. Med. Res. 54, 272–274. doi: 10.1016/j.arcmed.2023.03.004
Rahman, M. M., and Watanobe, Y. (2023). ChatGPT for education and research: opportunities, threats, and strategies. Appl. Sci. 13:5783. doi: 10.3390/app13095783
Rapoport, A. (1997). “Theory in environment behavior studies” in Handbook of Japan-United States Environment-Behavior Research: Toward a Transactional Approach. eds. S. Wapner, J. Demick, T. Yamamoto, and T. Takahashi (US: Springer), 399–421.
Sabzalieva, E., and Valentini, A. (2023). ChatGPT and Artificial Intelligence in Higher Education: Quick Start Guide. Paris: UNESCO.
Sallam, M. (2023). ChatGPT utility in healthcare education, research, and practice: systematic review on the promising perspectives and valid concerns. Health 11:887. doi: 10.3390/healthcare11060887
Seamon, D. (1982). The phenomenological contribution to environmental psychology. J. Environ. Psychol. 2, 119–140. doi: 10.1016/S0272-4944(82)80044-3
Solano-Flores, G. (2006). Language, dialect, and register: sociolinguistics and the estimation of measurement error in the testing of English language learners. Teach. Coll. Rec. 108, 2354–2379. doi: 10.1111/j.1467-9620.2006.00785.x
Stamps, A. E. III (2016). “Simulating designed environments” in Research Methods for Environmental Psychology. ed. R. Gifford (Chichester, UK: John Wiley & Sons, Ltd.), 197–220.
Stokel-Walker, C., and Van Noorden, R. (2023). What ChatGPT and generative AI mean for science. Nature 614, 214–216. doi: 10.1038/d41586-023-00340-6
Stokols, D. (1978). Environmental psychology. Annu. Rev. Psychol. 29, 253–295. doi: 10.1146/annurev.ps.29.020178.001345
Stokols, D. (1995). The paradox of environmental psychology. Am. Psychol. 50, 821–837. doi: 10.1037/0003-066X.50.10.821
Stokols, D. (2000). “Theory Development in Environmental Psychology” in Theoretical Perspectives in Environment-Behavior Research: Underlying Assumptions, Research Problems, and Methodologies. eds. S. Wapner, J. Demick, T. Yamamoto, and H. Minami (US: Springer), 269–276.
Stone, K. (2023). ChatGPT for social media marketing: the supreme guide to thriving on social media with the power of artificial intelligence. Independently published.
Sussman, R. (2016). “Observational methods” in Research Methods for Environmental Psychology. ed. R. Gifford (Chichester, UK: John Wiley & Sons, Ltd.), 9–27.
Urban, J., Bahník, Š., and Braun Kohlová, M. (2023). Pro-environmental behavior triggers moral inference, not licensing by observers I. Environ. Behav. 55, 74–98. doi: 10.1177/00139165231163547
van Dalen, H. P., and Henkens, K. (2012). Intended and unintended consequences of a publish-or-perish culture: A worldwide survey. J. Am. Soc. Inf. Sci. Technol. 63, 1282–1293. doi: 10.1002/asi.22636
Vogt, J. (2018). "Ships that pass in the night": Does scholarship on the social benefits of urban greening have a disciplinary crosstalk problem? Urban For. Urban Green. 32, 195–199. doi: 10.1016/j.ufug.2018.03.010
Walker, L. O., and Avant, K. C. (2014). Strategies for Theory Construction in Nursing. 5th Edn. Harlow, UK: Pearson.
Walters, W. H., and Wilder, E. I. (2023). Fabrication and errors in the bibliographic citations generated by ChatGPT. Sci. Rep. 13:14045. doi: 10.1038/s41598-023-41032-5
Wang, D., Lu, C.-T., and Fu, Y. (2023). Towards automated urban planning: when generative and ChatGPT-like AI meets urban planning. arXiv [Preprint]. doi: 10.48550/arXiv.2304.03892
Wapner, S., and Demick, J. (2002). “The increasing contexts of context in the study of environment behavior relations” in Handbook of Environmental Psychology. eds. R. B. Bechtel and A. Churchman (New York, NY: John Wiley & Sons, Inc.), 3–14.
Wei, J., Wang, X., Schuurmans, D., Bosma, M., Ichter, B., Xia, F., et al. (2023). Chain-of-thought prompting elicits reasoning in large language models. arXiv [Preprint]. doi: 10.48550/arXiv.2201.11903
White, J., Fu, Q., Hays, S., Sandborn, M., Olea, C., Gilbert, H., et al. (2023). A prompt pattern catalog to enhance prompt engineering with ChatGPT. arXiv [Preprint]. doi: 10.48550/arXiv.2302.11382
Wohlwill, J. F. (1970). The emerging discipline of environmental psychology. Am. Psychol. 25, 303–312. doi: 10.1037/h0029448
Wu, T., He, S., Liu, J., Sun, S., Liu, K., Han, Q.-L., et al. (2023). A brief overview of ChatGPT: the history, status quo and potential future development. IEEE/CAA J. Automat. Sin. 10, 1122–1136. doi: 10.1109/JAS.2023.123618
Xames, M. D., and Shefa, J. (2023). ChatGPT for research and publication: opportunities and challenges. J. Appl. Learn. Teach. 6. doi: 10.2139/ssrn.4381803
Keywords: environmental psychology, generative artificial intelligence, ChatGPT, research methods, research ethics
Citation: Yuan S, Li F, Browning MHEM, Bardhan M, Zhang K, McAnirlin O, Patwary MM and Reuben A (2024) Leveraging and exercising caution with ChatGPT and other generative artificial intelligence tools in environmental psychology research. Front. Psychol. 15:1295275. doi: 10.3389/fpsyg.2024.1295275
Edited by:
Paola Passafaro, Sapienza University of Rome, ItalyReviewed by:
Kathryn E. Schertz, University of Michigan, United StatesMassimiliano Caretti, National Research Council (CNR), Italy
Copyright © 2024 Yuan, Li, Browning, Bardhan, Zhang, McAnirlin, Patwary and Reuben. This is an open-access article distributed under the terms of the Creative Commons Attribution License (CC BY). The use, distribution or reproduction in other forums is permitted, provided the original author(s) and the copyright owner(s) are credited and that the original publication in this journal is cited, in accordance with accepted academic practice. No use, distribution or reproduction is permitted which does not comply with these terms.
*Correspondence: Shuai Yuan, syuan2@g.clemson.edu