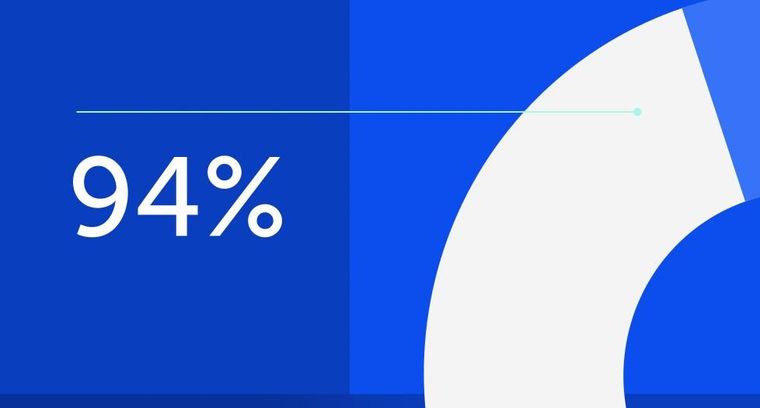
94% of researchers rate our articles as excellent or good
Learn more about the work of our research integrity team to safeguard the quality of each article we publish.
Find out more
ORIGINAL RESEARCH article
Front. Psychol., 14 February 2024
Sec. Quantitative Psychology and Measurement
Volume 15 - 2024 | https://doi.org/10.3389/fpsyg.2024.1280311
Introduction: The COVID-19 pandemic has profoundly changed university teaching and learning formats, leading to a significant increase in online learning. Consequently, the crisis has facilitated the potential development of this educational modality. However, researchers need adapted and validated instruments to assess the online learning climate in universities.
Aim: This study aimed to adapt and psychometrically validate the Online Learning Climate Scale (OLCS) for Chilean university students.
Method: Quantitative research was conducted with a non-experimental and cross-sectional, design executed in two phases: the first was oriented to the cultural adaptation of the instrument, and the second was focused on analyzing its psychometric properties in a sample of 491 university students.
Results: A translated and culturally adapted version was obtained, composed of 15 items distributed in a factorial structure composed of four dimensions that showed excellent adjustment to the data [χ2 (84) = 189.628; p < 0.001; CFI = 0.979; TLI = 0.973; RMSEA = 0.051 (IC90% 0.044–0.059); SRMR = 0.028]; internal consistency was estimated through Cronbach’s alpha and ranged between 0.892 and 0.955, and strict invariance between men and women was achieved.
Discussion: The Online Learning Climate Scale (OLCS) is a valid and reliable measure for measuring the online learning climate within the Chilean higher education context so that it can be used both in research and in monitoring management programs in educational environments.
The pandemic associated with the SARS-CoV-2 virus drastically transformed various areas of people’s lives worldwide, including educational environments (Aristovnik et al., 2020). To reduce the spread of the virus, universities worldwide had to rapidly transform face-to-face courses to virtual mode (Ali, 2020; Sahu, 2020). However, for many students, virtual teaching is strange and even threatening because it requires higher levels of self-regulation to achieve optimal learning outcomes (Aristovnik et al., 2020), especially when considering social interaction through participation in online discussions or group work (Thomas, 2012; Whittaker, 2015).
Some students adapt more quickly than others to virtual learning environments and promptly handle the ambiguities and uncertainties that these scenarios may represent (Cole et al., 2019). Adaptability to a virtual classroom environment depends on several factors. Still, one of the main ones is the learning climate, which is conceptualized as the bonding, synchronization, and interaction that is generated between students, instructors, and the course structure from the social relationships that occur within the context of synchronous or asynchronous learning on digital platforms (Derakhshesh et al., 2022). Considering that virtual learning environments entail different challenges than traditional face-to-face teaching (e.g., geographical separation between participants), it has been of interest to other research groups to determine the components of the construct and to analyze its relationship with outcomes of interest within institutions such as well-being and academic achievement (Johnson, 2006; Wang et al., 2020).
So far, most of the research in this area has been conducted primarily in the United States, Europe, and Australia, with few reports of research conducted in other parts of the world (Stanley and Montero Fortunato, 2022). This is particularly relevant when considering that online learning does not have the same meaning in different cultures, given that cultural values influence elements of instruction, collaboration, and academic behavior (Liu et al., 2010). Therefore, a vital issue is to have culturally sensitive and psychometrically validated instruments to measure and assess the online learning climate in higher education students, especially in broader contexts, such as Latin American countries, where research is very recent and challenging (Okoye et al., 2023). This article will propose and discuss the theoretical and empirical background highlighting the need to adapt the Online Learning Climate Scale (OLCS) linguistically and culturally to assess the online teaching climate in higher education.
The concept of classroom climate in face-to-face settings (hereafter, “traditional classroom climate”) is understood as the perceived connection between instructor and students (Cooper, 1995; Myers, 1995; Dwyer et al., 2004). However, Frisby and Martin (2010) describe classroom climate as the perception of connectedness and includes course organization, arguing that the perceived relationship with instructors and classmates is related to perceptions of connectedness in the classroom. López González and Bisquerra Alina (2013) have indicated that classroom climate involves organization, performance, and socio-affective quality.
It is worth noting that while there is evidence of traditional classroom climate, less is known about what constitutes the learning climate in online environments for university students. Online learning combines synchronous and asynchronous classes where instructors and students are physically separated (Brophy et al., 2021). Synchronous courses are delivered using computer-based tools, while asynchronous classes correspond to self-study that can be supplemented with interactions via email and platforms (Bogolepova, 2021). Unlike face-to-face courses, Kaufmann et al. (2015) have posited that interaction between instructors and students in online courses can occur asynchronously, synchronously, or in a mixed form. However, at an early stage, educators have raised concerns about the lack of communication and participation in the online learning environment (Allen, 2006).
The instructional beliefs model (IBM) provides a conceptual framework that enables a clear understanding of the components that can shape the online learning climate and how it can be related to other psychological and behavioral variables (Kaufmann et al., 2015; Kaufmann and Vallade, 2022). This model proposes that there are first-order variables, such as teacher behavior, student characteristics, and structural-instructional aspects of the course, which influence second-order variables, such as student perceptions associated with self-efficacy, self-concept, or general beliefs about the learning process, and these in turn influence relevant variables in schools such as learning, critical thinking, and time use (Weber et al., 2011).
Teacher behaviors refer to the actions adopted to establish effective and affective interactions with their students, which, for Myers et al. (2018), requires two perspectives: rhetorical and relational. On the one hand, the instructor’s rhetorical perspective is focused on communicating the course content most clearly and understandably to contribute to learning achievements and academic grades, thus referring to the more objective aspects of the instructional process. On the other hand, the relational perspective aims to develop and maintain connections and relationships within the classroom over time, i.e., these objectives are associated with the effect of interpersonal connections that ultimately represent the subjective dimension in virtual learning contexts (Mottet et al., 2006; Frisby and Martin, 2010; Frisby et al., 2013, 2014; Frisby and Gaffney, 2015).
The student characteristics component refers to those personal attributes that differentiate students from each other (Weber et al., 2011). These differential characteristics predispose to shaping each student’s perceptions of peers and teachers and include intellectual, motivational, and emotional abilities (McCroskey et al., 2006). Therefore, these characteristics also contribute to shaping the assessment made of the relationships established with other course participants, which is particularly challenging in an online classroom environment in which interactions do not usually occur in the same geographical space and often do not occur synchronously so that the impressions generated among participants are conditioned by a scenario in which feedback does not necessarily happen immediately (Serhan, 2010).
Finally, course-specific structural issues refer to formal aspects related to the content and structure of the course, mainly related to clear rules and instructions to establish a working agreement between teachers and students (Weber et al., 2011). The transparency of these aspects promotes a perception of fair treatment in students, which has been favorably linked to academic outcomes and learning (Mottet et al., 2006). In online learning, instructions have a fundamental role as they are the basis for participants to understand the use of technological platforms and the operating guidelines in classes and the evaluation system (Kaufmann et al., 2015).
The measurement of online classroom climate is mainly addressed using informatics, as it is a necessary resource for its implementation (Kaufmann et al., 2015; Bogolepova, 2021). Previous studies have provided insight into aspects related to online classroom climate; however, they present methodological limitations that impede the reporting of psychometric properties. For example, the Alqurashi (2019) and Cole et al. (2019) studies demonstrated the role of instructors and students in creating classroom climate environments, however, their sample size was small. In other cases, such as Swain et al. (2021), the participants belonged to computer science institutes, therefore, being related to a specific discipline it is not possible to generalize the results to other professions. Likewise, it is important to emphasize that for the measurement of variables of the educational context in virtual environments, it is not only enough to apply digital instruments, but it is also necessary to adapt them to the online environment, considering that many of these instruments have been developed to be applied in face-to-face classroom contexts (Hoi, 2022).
Therefore, evaluating the online learning climate from various perspectives and not only an informatic context is necessary. The educational context and the associated roles and interactions in this environment must also be evaluated. Previous literature, shows that few valid and reliable instruments measure classroom climate in virtual settings, one of them being the Online Learning Climate Scale (OLCS) by Kaufmann et al. (2015).
The OLCS is based on the premise that classroom climate comprises instructor behavior, student characteristics and behaviors, and course design/structure elements. Thus, this instrument explores how these dimensions articulate to establish the perception of classroom climate in online contexts (Kaufmann et al., 2015). The scale uses the IBM as its theoretical framework (Weber et al., 2011), and the initial bank of its items was created from the Classroom Communication Connectedness Inventory (Dwyer et al., 2004) and the Classroom Climate Scale (Gokcora’s, 1989).
This measurement was developed by Kaufmann et al. (2015), who, in a first study, generated a total of 47 items corresponding to the components of (1) instructor behaviors, (2) student characteristics and behaviors, and (3) specific structural aspects of the course; these items, in addition, were subjected to discussion in focus groups. The authors then conducted an exploratory factor analysis with a sample of 236 participants, from which they obtained the final version of the instrument composed of 15 items corresponding to four dimensions called Instructor Behavior (IB), Course Structure (CS), Course Clarity (CC), and Student Connectedness (SC). Subsequently, Kaufmann and Vallade (2022) conducted a confirmatory factor analysis of the scale, reaffirming the structure of four correlated factors. Although both studies showed excellent psychometric properties for the scale, they were developed with U.S. samples, so it would not be possible to guarantee a priori the cultural equivalence of the scale for its use in Latin America.
Digitalization has impacted various areas of society, and education is not exempt from this since digital platforms have allowed the development of flexible teaching methodologies that do not require face-to-face attendance (Borrego et al., 2017). In this regard, although in European countries and the United States, distance education covers about 20% of enrollment in higher education, in Chile, the scenario is different because until 2018, the number of students enrolled in online programs was still incipient; however, due to the pandemic, institutions were forced to incorporate online learning tools considering the confinement policies in force at the time (Villarroel et al., 2021).
The Higher Education Information Service [Servicio de Información de Educación Superior (SIES), 2023] reported that there are currently 1,341,439 people enrolled in higher education institutions, of which 11.2% correspond to the distance mode, representing an increase of 24.9% compared to 2022. Therefore, one of the serious problems is attrition from some online programs (Lee et al., 2015; Bawa, 2016; Hsu et al., 2019). The associated difficulty is primarily with low engagement in online learning, as students generally feel isolated and detached from learning platforms (Lee et al., 2015; Hoi and Hang, 2021).
Previous studies have shown that classroom climate influences other variables, such as self-efficacy, self-esteem, and depressive symptoms in university students (Hong et al., 2021). Notably, in online learning, it has been evidenced that a greater connection with other students and a clear course structure are linked to lower feelings of loneliness (Kaufmann and Vallade, 2022). Considering this, it is relevant to determine the elements that conform to the online classroom climate, the factors that promote positive climates, and how this influences student learning (Ko and Rossen, 2011; Ni, 2018).
Currently, little is known about what constitutes classroom climate in online learning contexts, which presents an opportunity for its study in South America, especially in Chile, where there are no valid and reliable tools to measure the learning climate in virtual educational environments. In addition, previous studies have shown that behaviors and perceptions in classes are different depending on gender (Lee and Mccabe, 2021; Koul et al., 2023); therefore, it is necessary to guarantee the metric equivalence of the measure between men and women so that possible differences obtained are directly due to the levels of the variable and not to metric equivalence biases.
Given this, the objective of this study was to culturally adapt the Online Learning Climate Scale (OLCS) and analyze its psychometric properties in Chilean university students. The present research was divided into two studies. The objective of the first study was to adapt the OLCS instrument linguistically and culturally in Chilean university students, while the second study had the objective of determining the internal structure, reliability indicators, and gender invariance of the questionnaire in a sample of Chilean university students. The steps followed in the two studies are specified in Figure 1.
This study was conducted entirely online and following the guidelines on test adaptation that have been compiled by international organizations such as the International Test Commission1 and systematized in the scientific literature (Elosua et al., 2014; Muñiz et al., 2015). In addition, the research protocol was previously approved by the university’s Scientific Ethics Committee, in which voluntary participation was guaranteed through informed consent and data safeguarding under confidentiality and anonymity.
The Online Learning Climate Scale (OLCS; Kaufmann et al., 2015) is a 15-item scale that measures online classroom climate through four dimensions, which are Instructor Behavior (IB), Course Structure (CS), Course Clarity (CC), and Student Connectedness (SC), with internal consistency indices ranging from 0.81 to 0.90. The response scale is a 7-point scale ranging from “strongly disagree” to “strongly agree.” Reliability in that study, estimated through Cronbach’s alpha, oscillated between 0.81 and 0.90.
Expert committee: A multidisciplinary team comprising three professional translators from the university’s language coordination and research departments. Also, four researchers from the educational field: two English professors from the English Pedagogy degree program, a Doctor of Education with experience in evaluation instruments, and a PhD in Applied Linguistics. In addition, a psychologist with training in measurement.
Pilot test and cognitive interview: A sample of 13 participants between 18 and 21 years (61.5% female) was obtained through non-probability sampling. The inclusion criteria were to be a student enrolled and taking an undergraduate course at the university in virtual mode.
A robust linguistic and cultural adaptation procedure was performed through the application of the analytical-rational procedures for instrument adaptation (Muñiz et al., 2013, 2015; Elosua et al., 2014) to guarantee the adaptation and equivalence of the instrument.
Initially, the instrument’s authors were contacted by e-mail to obtain permission and guarantee the legality of the adapted version. Next, the translation process of the OLCS instrument was initiated through three independent translators from the original language, American English, to the target language, Spanish (forward translation). After that, the three versions were discussed by a team of four experts in education and a psychologist specialized in measurement. This committee of experts evaluated, first individually and then as a group, the translation of the instrument through verification criteria focused on the cultural relevance of the items and the equivalence of the items for the Chilean context (see Supplementary material 1). The instrument was considered culturally relevant and coherent for measuring the dimensions proposed in the theory (Elosua and López-jaúregui, 2007; Hambleton and Zenisky, 2011).
Following the agreement of this committee, the version underwent a back-translation process by two native speakers to complement the study concerning grammatical and semantic equivalence, cultural relevance, linguistic appropriateness, format, and instrument design (Muñiz et al., 2013, 2015; Elosua et al., 2014). These translators also approved the translation’s equivalence based on standardized criteria (see Supplementary material 2). For this step, inter-rater concordance indices were calculated through Aiken’s V, which is considered adequate if it is greater than 0.70.
Finally, a cognitive interview was conducted with a small sample of undergraduate university students (n = 13) to pilot the instrument and ensure it was correctly understood; this included instructions, content, and type of response (Elosua et al., 2014) (see Supplementary material 3). These participants evaluated the scale’s usability using a guideline with a total score from 0 to 20, with 14 points or more being interpreted as high usability.
The main grammatical agreements were related to the differences between English and Spanish regarding personal pronouns and discourse markers for gender and number. In English, the third-person singular pronouns “he” and “she” refer to people according to gender. However, Spanish uses gendered pronouns for all people, including the third person singular “él” (he) for masculine and “ella” (she) for feminine. This can generate challenges in cross-cultural research when using translated scales (Padrón et al., 2017; Pérez et al., 2019).
The central language adaptations were implemented after the cognitive interview regarding linguistic adequacy. In this aspect, the adjustments were made in the Student Connectedness dimension, and they respond mainly to cultural elements that reflect the students’ perception of greater closeness to their peers: “mis compañeros (as)” (my classmates) instead of referring to “los estudiantes” (the students) as was proposed in the translation. The inter-rater agreement indices for translation and back-translation were.83 and.88, respectively, and are therefore considered adequate.
Likewise, the instructions and the response scale that accompany each of the four dimensions of the instrument were translated and adapted grammatically and linguistically to be understood by the target population. Regarding usability, the report indicated that the OLCS presents high usability because the average of the evaluations was 18.2 on a 20-point scale. The results show that the experience of answering the scale and the design were comfortable and accessible. In addition, the statements are clear and easy to understand, which reduces the response time required to complete it, which averaged 12 min. The process ended with a pilot version of the original 15 items adapted to Spanish (see Table 1).
The sample of Study 2 was obtained through non-probability sampling. Four hundred ninety-one undergraduate students of the Universidad de La Frontera were between 18 and 25 years old (54.1% female). The inclusion criteria were to be a student enrolled and taking an undergraduate course at the university in virtual mode. The adapted version of the instrument obtained in the first study was applied to this sample through the QuestionPro® platform.
Following the recommendations of Ferrando et al. (2022), a preliminary analysis of the data was performed to determine the existence of missing data or outliers, the latter being determined by a p-value below 0.05 in the Mahalanobis distance. Next, the univariate descriptive statistics were explored to ensure that the items provided variability and that none of the response categories had zero value in the frequency of responses; likewise, the values of skewness and kurtosis were inspected, whose values between 1 and −1 were suggestive of univariate normality, a scenario in which conventional estimators could be used (Muthent and Kaplan, 1992).
Next, multivariate normality was explored through Mardia’s statistics, considering that the assumption was fulfilled when the p-value of these was above 0.05. Confirmatory factor analysis (CFA) was then performed, based on the original structure of the instrument using the maximum likelihood (ML) estimator or its robust alternative (maximum likelihood robust, MLR) in case of non-compliance with normality; the choice of this alternative is due to the most recent recommendations of Li (2021) concerning the extraction of polytomous data.
The fit of the measurement model was evaluated using conventional indicators such as chi-square (χ2), comparative fit index (CFI), Tucker-Lewis index (TLI), root mean square error of approximation (RMSEA), and standardized root mean squared residual (SRMR). To consider a good model fit based on the sample size and the number of observable indicators, non-significant χ2 values, CFI and TLI ≥ 0.94, along with RMSEA and SRMR <0.07, were used as reference; in addition, factor loadings (λ) should be greater than 0.40 (Hair et al., 2019).
Next, the reliability coefficients for the total scale and its dimensions were estimated using a conventional estimator such as Cronbach’s alpha (α); in addition, McDonald’s omega (ω) was incorporated as it is a more accurate estimator in multidimensional structures (Trizano-Hermosilla and Alvarado, 2016). The coefficients are acceptable above 0.70 and good above 0.80 (Campo-Arias and Oviedo, 2008).
Finally, and complementarily, sex invariance analyses were performed; invariance analyses incorporate equality restrictions between groups to different model parameters (factor loadings, intercepts, and residuals). In this study, configural, metric (also known as weak), scalar (also known as strong), and residual (also known as strict) invariance models were tested (Kline, 2015). The criteria when comparing models were the change in CFI (ΔCFI) and RMSEA (ΔRMSEA) since the classical criterion of change in chi-square is sensitive to sample size; two models are considered to differ from each other and, therefore, the level of invariance is rejected when ΔCFI >0.010 (Cheung and Rensvold, 2002) and ΔRMSEA >0.015 (Chen, 2007).
Descriptive and univariate analyses were performed in the SPSS v26.0 statistical program, while multivariate estimations were performed using the lavaan package in RStudio.
Preliminary analyses showed no missing data or outliers in the participants’ responses, whose frequencies and percentages are presented in Table 2. On the other hand, univariate and multivariate analyses showed that the principle of normality was not met, so the models were run using the robust alternative of the maximum likelihood estimator (MLR).
First, a model with four correlated factors (oblique) was estimated according to the original structure of the instrument; this model obtained an excellent fit to the data [χ2 (84) = 189.628; p < 0.001; CFI = 0.979; TLI = 0.973; RMSEA = 0.051 (IC90% 0.044–0.059); SRMR = 0.028] and the factor loadings can be considered adequate (see Table 3). Given that the correlations between the first three factors were high (r > 0.80), it was plausible to hypothesize the existence of a unidimensional structure; however, this model had a poor fit to the data [χ2 (90) = 1044.597; p < 0.001; CFI = 0.794; TLI = 0.759; RMSEA = 0.147 (IC90% 0.139–0.155); SRMR = 0.025] and the factor loadings of items 12, 13, and 14 were below the established cutoff point (see Table 3).
Following the recommendations of Zhang et al. (2016) to avoid underestimation of unidimensional models due to possible multicollinearity, a second-order model was estimated in which the four factors that compose the scale do not correlate with each other but are explained by a second-order latent factor; this model obtained an excellent fit to the data [χ2 (86) = 211.226; p < 0.001; CFI = 0.975; TLI = 0.969; RMSEA = 0.055 (IC90% 0.048–0.062); SRMR = 0.038]; in terms of factor loadings, all items loaded significantly on their factors and these in turn on the second-order factor (see Table 3). Considering the fit of all the models, the one that best reflects the behavior of the data and is the most parsimonious is the four-factor oblique model; therefore, the remaining analyses were conducted with this model. In addition, excellent average variance extracted indicators were obtained for Instructor Behavior (AVE = 0.792), Course Structure (AVE = 0.856), Course Clarity (AVE = 0.853), and Student Connectedness (AVE = 0.716).
The estimated reliability coefficients were excellent for the dimensions of Instructor Behavior (α = 0.955; ω = 0.959), Course Structure (α = 0.948; ω = 0.948), and Course Clarity (α = 0.947; ω = 0.946), and good for Student Connectedness (α = 0.892; ω = 0.877). Likewise, the invariance analyses reached the residual (strict) level by gender. This means that the scale allows reliable comparisons between men and women and affirms that the differences are due to the data and not elements inherent to the measuring instrument (see Table 4).
According to Kaufmann et al. (2015), the OLCS explores how instructor behaviors, student characteristics and behaviors, and course design/structure elements articulate to establish the perceived classroom climate in online contexts. Likewise, in the context of Higher Education in Latin America, research on the online learning climate is scarce, and in Chile, there are no valid and reliable tools to measure it. Therefore, the present research was divided into two studies: (a) to adapt the OLCS linguistically and culturally for Chilean university students and (b) to psychometrically validate the OLCS instrument.
The first study provided a consensus version of 15 items, and there was no need to eliminate any of them. In the same way, the instructions and the response scale accompanying each of the four dimensions of the instrument were translated grammatically and linguistically adapted for comprehension. Thus, the linguistic and cultural adaptation process yielded an instrument that maintains the four dimensions, number of items, and instructions of the one reported by Kaufmann et al. (2015), which shows the equivalence of the instruments at the semantic level.
A culturally sensitive adaptation of the measurement instruments guarantees the reduction of biases. It allows the comparability of results between studies that may be carried out in different countries since it is based on a robust process that is not limited to the direct translation of the questions of the original questionnaire but contextualizes them by recognizing the semantic particularities of the language and culture to which the target sample belongs ((Muñiz et al., 2013, 2015; Elosua et al., 2014;). Therefore, having an adapted version that shows excellent usability indicators is the basis for future studies that wish to include the measurement of the construct of their models, especially when considering that much of the literature in this area has been developed in English-speaking countries (Okoye et al., 2023), so that the evidence obtained in this background is not completely extrapolated to the Chilean reality; therefore, studies are needed to account for the phenomenon in this context, being one of the first requirements, to have valid and reliable instruments.
The second study sought to validate the instrument psychometrically. The results showed that the OLCS instrument is a four-factor scale, according to the original structure of the instrument, which presented a better fit to the data than the unidimensional model and an alternative second-order proposal. These four dimensions account for relevant aspects within the learning beliefs model and incorporate objective (e.g., classroom norms) and subjective (e.g., interactions perception) elements of didactic interaction that become central to the development of an adequate classroom climate, especially in those contexts where the dynamics ultimately differ due to the lack of a common physical environment (Weber et al., 2011; Kaufmann et al., 2015).
Likewise, the OLCS with its 15 items proved to be reliable, even improving the indicators obtained in previous psychometric analyses (Kaufmann et al., 2015; Kaufmann and Vallade, 2022), and the analyses reached the highest level of invariance (residual) when restricting parameters between men and women. Consequently, it can be stated that this is an accurate instrument whose items are consistent with each other and that it allows future research proposals to compare latent averages between men and women with the assurance that differences are directly due to the trait measured and not to methodological artifices inherent to the research instrument (Kline, 2015).
From this perspective, the OLCS scale makes it possible to gather information on what is happening in virtual classroom learning environments and thus enhance learning in the virtual environment (Laurillard, 2012). Likewise, it represents inputs for developing lines of research and the possibility of developing guidelines and improvement resources for online teaching programs (Thomas, 2012; Whittaker, 2015; Aristovnik et al., 2020). From this perspective, understanding the effects of online classroom climate on learning contributes to improving the teaching and learning process and well-being in the online classroom for both students and professors, especially in the current post-pandemic context. The OLCS scale as a valid and reliable measure has the potential to collaborate in higher education with the main problems that the literature has shown in online learning environments, i.e., dropout rates (Lee et al., 2015; Bawa, 2016; Hsu et al., 2019) and low engagement (Lee et al., 2015; Hoi and Hang, 2021), and contribute to the development and management of a positive online classroom climate (Ko and Rossen, 2011; Ni, 2018).
Although the present study has strengths, there are also some weaknesses, the first of which was the non-probability sampling, which prevents the generalization of the data, especially because the sample studied corresponds to a university that exceptionally maintained online classes since the modality of study is entirely face-to-face; therefore, future studies should test the scale in samples of universities oriented to distance education. Likewise, other scales were not included to analyze the convergent validity of the instrument with other measures. Considering those mentioned above, future studies with the OLCS should test its concurrent validity with measures such as loneliness, subjective well-being, or academic dropout. Likewise, future lines of work could make longitudinal measurements to increase the measurements’ precision and evaluate the measure’s stability, i.e., whether the measure is consistent and stable over time. Longitudinal measurements would make it possible to detect changes in the measured variable. Finally, considering that virtual education increasingly allows people to connect in different parts of the world, it would be interesting to study the invariance of the test between countries.
The above evidence from the validity analyses supports that the OLCS is a scale that works as theoretically expected, and it can be concluded that it is a valid and reliable instrument to measure the different dimensions of the online learning climate in the Chilean context.
The raw data supporting the conclusions of this article will be made available by the authors, without undue reservation.
The studies involving humans were approved by Comité Ético Científico de la Universidad de La Frontera ACTA N° 062_21. The studies were conducted in accordance with the local legislation and institutional requirements. The participants provided their written informed consent to participate in this study.
MB-S: Conceptualization, Funding acquisition, Investigation, Project administration, Resources, Validation, Visualization, Writing – original draft, Writing – review & editing. RM: Data curation, Formal analysis, Methodology, Software, Writing – original draft. OT-M: Data curation, Formal analysis, Methodology, Software, Supervision, Validation, Writing – original draft. MM-C: Methodology, Validation, Writing – review & editing. LC: Investigation, Methodology, Resources, Writing – original draft.
The author(s) declare financial support was received for the research, authorship, and/or publication of this article. This study was supported by the Fondo Nacional de Desarrollo Científico y Tecnológico, FONDECYT REGULAR with grant 1220166, whose responsible researcher is Dr. Monica Bravo-Sanzana and by the Universidad de La Frontera, under Project DI21-011.
We appreciate the support of the UNESCO Chair Researcher: Childhood, Youth, Education, and Society.
The authors declare that the research was conducted in the absence of any commercial or financial relationships that could be construed as a potential conflict of interest.
All claims expressed in this article are solely those of the authors and do not necessarily represent those of their affiliated organizations, or those of the publisher, the editors and the reviewers. Any product that may be evaluated in this article, or claim that may be made by its manufacturer, is not guaranteed or endorsed by the publisher.
The Supplementary material for this article can be found online at: https://www.frontiersin.org/articles/10.3389/fpsyg.2024.1280311/full#supplementary-material
Ali, W. (2020). Online and remote learning in higher education institutes: a necessity in light of COVID-19 pandemic. High. Educ. Stud. 10, 16–25. doi: 10.5539/hes.v10n3p16
Allen, T. H. (2006). Raising the question #1 is the rush to provide on-line instruction setting our students up for failure? Commun. Educ. 55, 122–126. doi: 10.1080/03634520500343418
Alqurashi, E. (2019). Predicting student satisfaction and perceived learning within online learning environments. Distance Educ. 40, 133–148. doi: 10.1080/01587919.2018.1553562
Aristovnik, A., Keržič, D., Ravšelj, D., Tomaževič, N., and Umek, L. (2020). Impacts of the COVID-19 Pandemic on Life of Higher Education Students: A Global Perspective. Sustainability, 12, 1–2. doi: 10.3390/su12208438
Bawa, P. (2016). Retention in online courses: exploring issues and solutions — a literature review. SAGE Open 6:177. doi: 10.1177/2158244015621777
Bogolepova, S. V. (2021). Emergency transition: have tertiary language students’ needs been met? Prof. Discourse Commun. 3, 49–61. doi: 10.24833/2687-0126-2021-3-1-49-61
Borrego, D. D., Olivares, N. R., and Cervantes, D. C. (2017). Educación a distancia y TIC. Bloomington, IN: Palibrio.
Brophy, N. S., Broeckelman-Post, M. A., Nordin, K., Miller, A. D., Buehl, M. M., and Vomund, J. (2021). Pandemic pedagogy: elements of online supportive course design. J. Commun. Pedagogy 5, 95–114. doi: 10.31446/JCP.2018.02
Campo-Arias, A., and Oviedo, H. C. (2008). Propiedades Psicométricas de una Escala: la Consistencia Interna. Revista de Salud Publica 10, 831–839. doi: 10.1590/s0124-00642008000500015
Chen, F. F. (2007). Structural equation Modeling: a multidisciplinary sensitivity of goodness of fit indexes to lack of measurement invariance sensitivity of goodness of fit indexes to lack of measurement invariance. Struct. Equ. Model. Multidiscip. J. 14, 464–504. doi: 10.1080/10705510701301834
Cheung, G. W., and Rensvold, R. B. (2002). Structural equation Modeling: a evaluating goodness-of- fit indexes for testing measurement invariance. Struct. Equ. Model. Multidiscip. J. 9, 233–255. doi: 10.1207/S15328007SEM0902_5
Cole, A. W., Lennon, L., Weber, N. L., Cole, A. W., Lennon, L., Student, N. L. W., et al. (2019). Student perceptions of online active learning practices and online learning climate predict online course engagement learning climate predict online course engagement. Interact. Learn. Environ. 29, 866–880. doi: 10.1080/10494820.2019.1619593
Cooper, J. L. (1995). Cooperative Learning and Critical Thinking. Teaching of Psychology, 22, 7–9. doi: 10.1207/s15328023top2201_2
Derakhshesh, A., Fathi, J., Hosseini, H. M., and Mehraein, S. (2022). An investigation of the structural model of online course satisfaction, online learning self-efficacy, and online learning climate in the EFL context. Comput. Assist. Lang. Learn. Electron. J. 23, 261–281.
Dwyer, K. K., Bingham, S. G., Carlson, R. E., Prisbell, M., Cruz, A. M., and Fus, D. A. (2004). Communication and connectedness in the classroom: development of the connected classroom climate inventory. Commun. Res. Rep. 21, 264–272. doi: 10.1080/08824090409359988
Elosua, P., and López-jaúregui, A. (2007). Potential sources of differential item functioning in the adaptation of tests. Int. J. Test. 7, 39–52. doi: 10.1080/15305050709336857
Elosua, P., Mujika, J., Almeida, L., and Hermosilla, D. (2014). Procedimientos analítico-racionales en la adaptación de tests. Adaptación al español de la batería de pruebas de razonamiento. Revista Latinoamericana de Psicología 46, 117–126. doi: 10.1016/S0120-0534(14)70015-9
Ferrando, P. J., Lorenzo-seva, U., Hernández-dorado, A., and Muñiz, J. (2022). Decálogo para el Análisis Factorial de los Ítems de un Test. Psicothema 34, 7–17. doi: 10.7334/psicothema2021.456
Frisby, B. N., Berger, E., Burchett, M., Herovic, E., and Strawser, M. G. (2014). Participation apprehensive students: the influence of face support and instructor–Student rapport on classroom participation. Commun. Educ. 63, 105–123. doi: 10.1080/03634523.2014.881516
Frisby, B. N., and Gaffney, A. L. H. (2015). Understanding the role of instructor rapport in the college classroom understanding the role of instructor rapport in the college classroom. Commun. Res. Rep. 32, 340–346. doi: 10.1080/08824096.2015.1089847
Frisby, B. N., Limperos, A. M., and Downs, E. (2013). Students’ perceptions of social presence: rhetorical and relational goals across three mediated instructional designs. MERLOT J. Online Learn. Teach. 9, 468–480.
Frisby, B. N., and Martin, M. M. (2010). Instructor–Student and Student–Student rapport in the classroom. Commun. Educ. 59, 146–164. doi: 10.1080/03634520903564362
Gokcora, D. (1989). A descriptive study of communication and teaching strategies used by two types of international teaching assistants at the University of Minnesota, and their cultural perceptions of teaching and teachers. Communication Strategies. University of Minnesota: Minneapolis, MN.
Hair, J. F., Black, W. C., Babin, B. J., Anderson, R. E., and Tatham, R. (2019). Multivariate data analysis. 8th Edn. Boston, MA: Cengage.
Hambleton, R. K., and Zenisky, A. L. (2011). Translating and adapting tests for cross-cultural assessments. In D. Matsumoto and F. J. R. Vijvervan de (Eds.), Cross-cultural research methods in psychology (pp. 46–74). Cambridge: Cambridge University Press.
Hoi, V. N. (2022). Measuring students’ perception of an engaging online learning environment: an argument – based scale validation study. Educ. Technol. Res. Dev. 70, 2033–2062. doi: 10.1007/s11423-022-10155-3
Hoi, V. N., and Hang, H. L. (2021). The structure of student engagement in online learning: a bi-factor exploratory structural equation modelling approach. J. Comput. Assist. Learn. 37, 1141–1153. doi: 10.1111/jcal.12551
Hong, F. Y., Chiu, S. I., Huang, D. H., and Chiu, S. L. (2021). Correlations among classroom emotional climate, social self-efficacy, and psychological health of university students in Taiwan. Education and Urban Society, 53, 446–468. doi: 10.1177/0013124520931458
Hsu, H. K., Wang, C. V., and Levesque-Bristol, C. (2019). Reexamining the impact of self-determination theory on learning outcomes in the online learning environment. Educ. Inf. Technol. 24, 2159–2174. doi: 10.1007/s10639-019-09863-w
Johnson, G. M. (2006). Perception of classroom climate, use of web CT, and academic achievement. J. Comput. High. Educ. 17, 25–46. doi: 10.1007/BF03032697
Kaufmann, R., Sellnow, D. D., and Frisby, B. N. (2015). The development and validation of the online learning climate scale (OLCS). Commun. Educ. 65, 307–321. doi: 10.1080/03634523.2015.1101778
Kaufmann, R., and Vallade, J. I. (2022). Exploring connections in the online learning environment: student perceptions of rapport, climate, and loneliness perceptions of rapport, climate, and loneliness. Interact. Learn. Environ. 30, 1794–1808. doi: 10.1080/10494820.2020.1749670
Kline, R. B. (2015). Principles and practice of structural equation Modeling. 4th Edn. New York: Guilford Press.
Koul, R. B., McLure, F. I., and Fraser, B. J. (2023). Gender differences in classroom emotional climate and attitudes among students undertaking integrated STEM projects: a Rasch analysis. Res. Sci. Technol. Educ. 41, 1051–1071. doi: 10.1080/02635143.2021.1981852
Laurillard, D. (2012). Teaching as a design science. Building Pedagogical Patterns for Learning and Technology. New York: Routledge.
Lee, J. J., and Mccabe, J. M. (2021). Who speaks and who listens: revisiting the chilly climate in college classrooms. Gend. Soc. 35, 32–60. doi: 10.1177/0891243220977141
Lee, E., Pate, J. A., and Cozart, D. (2015). Autonomy support for online students. Tech Trends 59, 54–61. doi: 10.1007/s11528-015-0871-9
Li, C. H. (2021). Statistical estimation of structural equation models with a mixture of continuous and categorical observed variables. Behav. Res. Methods 53, 2191–2213. doi: 10.3758/s13428-021-01547-z
Liu, X., Liu, S., Lee, S. H., and Magjuka, R. J. (2010). Cultural differences in online learning: international student perceptions. J. Educ. Technol. Soc. 13, 177–188.
López González, L., and Bisquerra Alina, R. (2013). Validación y análisis de una escala breve para evaluar el clima de clase en educación secundaria. Psicopedagogía, 62–77.
McCroskey, J. C., Richmond, V. P., and Bennett, V. E. (2006). The relationships of student end-of-class motivation with teacher communication behaviors and instructional outcomes. Commun. Educ. 55, 403–414. doi: 10.1080/03634520600702562
Mottet, T. P., Frymier, A. B., and Beebe, S. A. (2006). “Theorizing about instructional communication” in Handbook of instructional communication: Rhetorical and relational perspectives. eds. T. P. Mottet, V. P. Richmond, and J. C. McCroskey (Boston, MA: Allyn & Bacon), 255–282.
Muñiz, J., Elosua, P., and Hambleton, R. K. (2013). International Test Commission Guidelines for test translation and adaptation. Psicothema, 25, 151–157. doi: 10.7334/psicothema2013.24
Muñiz, J., Hernández, A., and Ponsoda, V. (2015). Nuevas directrices sobre el uso de los tests: investigación, control de calidad y seguridad. Papeles Del Psicólogo 36, 161–173.
Muthent, B., and Kaplan, D. (1992). A comparison of some methodologies for the factor analysis of non-normal Likert variables: a note on the size of the model. Br. J. Math. Sfaflslical Psychol. 45, 19–30. doi: 10.1111/j.2044-8317.1992.tb00975.x
Myers, S. A. (1995). Student perceptions of teacher affinity – seeking and classroom climate. Commun. Res. Rep. 12, 192–199. doi: 10.1080/08824099509362056
Myers, S. A., Garlitz, K. T., Kromka, S. M., Nicholson, A. L., Sutherland, A. D., and Thomas, M. J. (2018). Using rhetorical/relational goal theory to examine millennial students’ academic and relational needs. Atay, A., and Ashlock, M. Z. Millennial culture and communication pedagogies: Narratives from the classroom and higher education. Rowman & Littlefield: Lanham, MD. 121–141.
Ni, A. Y. (2018). Comparing the effectiveness of classroom and online learning: teaching research methods. J. Public Affairs Educ. 19, 199–215. doi: 10.1080/15236803.2013.12001730
Okoye, K., Hussein, H., Arrona-palacios, A., Quintero, H. N., Omar, L., Ortega, P., et al. (2023). Impact of digital technologies upon teaching and learning in higher education in Latin America: an outlook on the reach, barriers, and bottlenecks. Educ. Inf. Technol. 28, 2291–2360. doi: 10.1007/s10639-022-11214-1
Padrón, E., Dakanalis, A., Carrera, O., Calogero, R., Fernández-Aranda, F., and Clerici, M. (2017). The Spanish Version of the Body Dissatisfaction Scale: Psychometric Properties, Gender Invariance, and the Moderating Role of Eating Disorder Symptoms. Assessment, 24, 155–170. doi: 10.1177/1073191115587873
Pérez, J. F., Sánchez, E., Olmo, R., and García, F. E. (2019). Adaptación y validación de la Escala de Estrés Parental en padres y madres mexicanos. Revista Iberoamericana de Diagnóstico y Evaluación e Avaliação Psicológica, 51, 47–60. doi: 10.21865/RIDEP51.2.04
Sahu, P. (2020). Closure of universities due to coronavirus disease 2019 (COVID-19): Impact on Education and mental health of students and academic staff. Cureus 12:e7541. doi: 10.7759/cureus.7541
Servicio de Información de Educación Superior (SIES) (2023). Informe 2023, Matrícula en Educación Superior. Available at: https://www.mifuturo.cl/wp-content/uploads/2023/07/Matricula-_en_Educacion_Superior_2023_SIES.pdf
Stanley, D., and Montero Fortunato, Y. R. (2022). The efficacy of online higher education in Latin America: a systematic literature review. Revista Iberoamericana de Tecnologias Del Aprendizaje 17, 262–269. doi: 10.1109/RITA.2022.3191299
Swain, D., Jena, L. K., Dash, S. S., and Yadav, R. S. (2021). Motivation to learn, mobile learning and online learning climate: moderating role of learner interaction. European J. Train. Dev. 47, 123–140. doi: 10.1108/EJTD-06-2021-0077
Thomas, L. (2012). Building student engagement and belonging in higher education at a time of change: A summary of findings and recommendations from the what works? Student Retention & Success programme. London: Paul Hamlyn Foundation.
Trizano-Hermosilla, I., and Alvarado, J. M. (2016). Best alternatives to Cronbach’s alpha reliability in realistic conditions: congeneric and asymmetrical measurements. Front. Psychol. 7:769. doi: 10.3389/fpsyg.2016.00769
Villarroel, V., Pérez, C., Rojas-Barahona, C. A., and García, R. (2021). Educación remota en contexto de pandemia: caracterización del proceso educativo en las universidades chilenas. Formación universitaria 14, 65–76. doi: 10.4067/S0718-50062021000600065
Wang, M. T., Degol, J. L., Amemiya, J., Parr, A., and Guo, J. (2020). Classroom climate and children’s academic and psychological wellbeing: a systematic review and meta-analysis. Dev. Rev. 57:100912. doi: 10.1016/j.dr.2020.100912
Weber, K., Martin, M. M., and Myers, S. A. (2011). The development and testing of the instructional beliefs model. Commun. Educ. 60, 51–74. doi: 10.1080/03634523.2010.491122
Whittaker, A. A. (2015). Effects of team-based learning on self-regulated online learning. Int. J. Nurs. Educ. Scholarsh. 12, 45–54. doi: 10.1515/ijnes-2014-0046
Keywords: online learning, classroom climate, distance education, psychometric analysis, reliability, validity
Citation: Bravo-Sanzana M, Miranda R, Terán-Mendoza O, Mieres-Chacaltana M and Carabantes L (2024) Cultural adaptation and psychometric properties of the online learning climate scale for Chilean university students. Front. Psychol. 15:1280311. doi: 10.3389/fpsyg.2024.1280311
Received: 20 August 2023; Accepted: 22 January 2024;
Published: 14 February 2024.
Edited by:
Lluís Coromina Soler, University of Girona, SpainReviewed by:
Imanuel Hitipeuw, State University of Malang, IndonesiaCopyright © 2024 Bravo-Sanzana, Miranda, Terán-Mendoza, Mieres-Chacaltana and Carabantes. This is an open-access article distributed under the terms of the Creative Commons Attribution License (CC BY). The use, distribution or reproduction in other forums is permitted, provided the original author(s) and the copyright owner(s) are credited and that the original publication in this journal is cited, in accordance with accepted academic practice. No use, distribution or reproduction is permitted which does not comply with these terms.
*Correspondence: Mónica Bravo-Sanzana, bW9uaWNhdml2aWFuYS5icmF2b0B1ZnJvbnRlcmEuY2w=
Disclaimer: All claims expressed in this article are solely those of the authors and do not necessarily represent those of their affiliated organizations, or those of the publisher, the editors and the reviewers. Any product that may be evaluated in this article or claim that may be made by its manufacturer is not guaranteed or endorsed by the publisher.
Research integrity at Frontiers
Learn more about the work of our research integrity team to safeguard the quality of each article we publish.