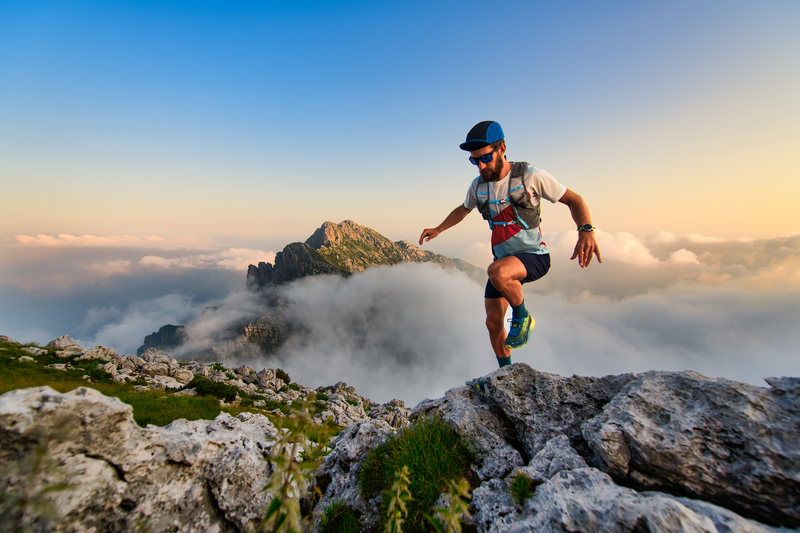
95% of researchers rate our articles as excellent or good
Learn more about the work of our research integrity team to safeguard the quality of each article we publish.
Find out more
ORIGINAL RESEARCH article
Front. Psychol. , 07 February 2024
Sec. Educational Psychology
Volume 15 - 2024 | https://doi.org/10.3389/fpsyg.2024.1268549
This study is centered on investigating the acceptance and utilization of AI Chatbot technology among graduate students in China and its implications for higher education. Employing a fusion of the UTAUT (Unified Theory of Acceptance and Use of Technology) model and the ECM (Expectation-Confirmation Model), the research seeks to pinpoint the pivotal factors influencing students’ attitudes, satisfaction, and behavioral intentions regarding AI Chatbots. The study constructs a model comprising seven substantial predictors aimed at precisely foreseeing users’ intentions and behavior with AI Chatbots. Collected from 373 students enrolled in various universities across China, the self-reported data is subject to analysis using the partial-least squares method of structural equation modeling to confirm the model’s reliability and validity. The findings validate seven out of the eleven proposed hypotheses, underscoring the influential role of ECM constructs, particularly “Confirmation” and “Satisfaction,” outweighing the impact of UTAUT constructs on users’ behavior. Specifically, users’ perceived confirmation significantly influences their satisfaction and subsequent intention to continue using AI Chatbots. Additionally, “Personal innovativeness” emerges as a critical determinant shaping users’ behavioral intention. This research emphasizes the need for further exploration of AI tool adoption in educational settings and encourages continued investigation of their potential in teaching and learning environments.
AI chatbots, such as OpenAI’s ChatGPT, utilize natural language processing and machine learning to simulate human-like conversations. ChatGPT, powered by a vast pre-trained dataset and reinforcement learning, demonstrates proficient language understanding, text generation, and high performance in diverse tasks like translation, composition, questioning, and summarization (Kasneci et al., 2023; Qadir, 2023). Since its launch in November 2022, ChatGPT has gained global attention for its human-like output quality, social capabilities, and extensive user base. Achieving 100 million active users within two months, it holds the record as the fastest-growing application in history (Harrison, 2023). The newest version, GPT-4, released in March 2023, extends its capabilities to image processing, significantly expanding its functionalities (Achiam, 2023).
The advent and ongoing progress of AI chatbots have catalyzed significant transformations across various fields, spanning education, finance, law, healthcare, media, and telecommunications (Aung et al., 2021). Particularly in education, the integration of large language models like ChatGPT has revolutionized the landscape, enabling personalized learning, cross-language learning methodologies, intelligent teaching strategies, efficient academic planning, and optimized management systems. They serve as essential aids for educators and virtual tutors for students, facilitating tasks like lesson planning, language translation, homework assignments, and essay composition (Qadir, 2023). Their versatility extends to addressing technical (e.g., engineering technology and computer programming) and non-technical issues (e.g., language and literature) across diverse disciplines. For example, in computer science, they provide explanations for various code, aiding students’ programming comprehension (MacNeil et al., 2022); in chemistry, they assist in evaluating answer quality (Moore et al., 2022); in language teaching, they offer personalized interaction, reducing learner anxiety (Bao, 2019); and in medical education, they aid in auto-grading, auxiliary teaching, information retrieval, and case-scenario generation (Thunström, 2022; Hamoudi, 2023).
Though AI Chatbots offer advantages in education, they have limitations such as potential inaccuracies, biases, lack of critical thinking, and ethical, privacy, and legal risks (Rahman and Watanobe, 2023; Zhu et al., 2023). Surprisingly, ChatGPT passed exams at the University of Minnesota Law School (Samantha, 2023). Concerns also exist about students misusing AI tools for plagiarism, exam cheating, and research data abuse (van Dis et al., 2023).
The emergence and ongoing evolution of AI Chatbots have catalyzed a global wave of AI innovation, with major industry players such as Google, Anthropic, and Meta launching their large language model products, such as Google’s Bard, Gemini, and Anthropic’s Claude. Simultaneously, in China, a multitude of generative AI tools have been introduced. Baidu unveiled “Wenxin Yiyan,” their large language model, in March 2023, while Alibaba initiated beta testing of “Tongyi Qianwen” in April, and Sensetime Technology released “Shangliang.” Additionally, various AI projects are in the pipeline, including JD Cloud’s “ChatJD,” Huawei’s “Pangu,” Tencent’s “Hunyuan,” and the Chinese Academy of Sciences Institute of Automation’s “Zidong Taichu.”
In China, highly educated young individuals form the primary cohort dedicated to the extensive use of AI chatbots, using new technologies to underscore their social status. Their adoption is fueled by this demographic emphasis. Furthermore, qualitative studies by Qiang and Hu (2023) have highlighted the allure of AI chatbots, identifying user attitudes as fervent admiration for innovation, objective acceptance of technological shocks, and understanding and tolerance for imperfections.
Chinese researchers acknowledge the dual nature of AI Chatbots, recognizing both their potential for human development and associated concerns (Hou and Li, 2023). These concerns include the potential for social biases, ethical issues, algorithmic enclosure, diminished user autonomy (Zhang and Jia, 2023), as well as privacy infringement, data security risks, and challenges related to intellectual property rights (Tan, 2023).
Prior research primarily focuses on AI Chatbot acceptance among undergraduate students, with limited attention to graduate students, particularly in China. Given that graduate students are essential to scientific research and innovation, and their research progress holds significant societal implications, this study aims to explore the acceptance and usage of AI Chatbots among Chinese graduate students, addressing this literature gap:
1. What is the factorial structure of the acceptance and utilization of AI Chatbot technology among graduate students in China, considering the combined framework of the UTAUT model and the ECM model?
2. What are the structural relationships among the predictors of graduate students’ attitudes, satisfaction, and behavioral intentions (BI) towards AI Chatbots in the context of higher education in China?
The emergence and progression of AI chatbots as technological constructs signify a substantial advancement in the realm of human-computer interaction. As postulated by Xu et al. (2020), AI chatbots embody sophisticated systems capable of assimilating real-time data from diverse sources to provide customized responses, suggestions, and resolutions to complex customer inquiries. This functional capacity is deeply rooted in the historical underpinnings of AI chatbots, dating back to the seminal inquiry posed by Alan Turing in the 1950s, “Can machines think?”—a foundational question within the field of AI, as extensively documented by Medina and Ferrer (2022).
The evolution of AI chatbots has significantly revolutionized customer service, offering real-time, personalized support by integrating data from various touchpoints, thus enhancing customer engagement and satisfaction. Through constant learning and adaptation, AI chatbots can better understand and respond to the nuanced needs and preferences of users, reshaping the landscape of human-computer interaction and challenging traditional models of customer engagement. This versatility and adaptability underscore the transformative power of AI chatbots, positioning them as pivotal assets in reshaping the dynamic between technology and human interaction.
The field has seen the emergence and persistent use of various chatbots, with contemporary models like ChatGPT and those developed by Chinese entities showcasing advanced features such as voice recognition and synthesis. These advancements significantly expand the practical scope of chatbots across a wide array of industries, including the crucial domain of education and language acquisition.
In education, AI chatbots are gaining prominence as dynamic instructional agents, proficient in delivering educational content, engaging learners in dialogue, and providing instant feedback, as observed by Mageira et al. (2022). Their role is notably complementary to that of human educators, offering continuous assistance and support to students—a function that has gained significant importance in the context of remote learning and language acquisition. This dualistic role has been explored by scholars such as Shawar (2017), who have identified the potential of chatbots in alleviating language-related anxiety, enhancing student engagement, and facilitating iterative learning opportunities enriched by multimodal interactions.
The research conducted by Wu and Yu (2023), employing meta-analytical methodologies to explore the influence of AI chatbots on educational outcomes, confirms the considerable efficacy of chatbots within higher education, in contrast to the more modest effects observed in primary and secondary education settings. However, the incorporation of chatbots in education is not without its challenges. Notably, the work of Wang et al. (2023) highlights prominent concerns related to privacy, cultural considerations, and language proficiency barriers, offering a critical examination of AI’s integration into educational administration and pedagogy.
Meanwhile, ChatGPT represents a novel chatbot deeply rooted in the Generative Pre-training Transformer architecture. Extensive analyses by Dwivedi et al. (2023), Farrokhnia et al. (2023), Su et al. (2023), and Tlili et al. (2023) confirm its superiority over early chatbots in the realms of understanding and generating human-like texts, and providing comprehensive feedback on lengthy textual inputs. These distinctive capabilities position ChatGPT as a formidable writing assistant and tool, as supported by research from Barrot (2023), Dergaa et al. (2023), and Imran and Almusharraf (2023). Furthermore, Taecharungroj’s (2023) analysis of early reactions on Twitter underscores ChatGPT’s predominant use in writing applications such as the composition of essays and articles. This widespread recognition and adoption of ChatGPT within writing tasks signal its instrumental role in facilitating and enhancing the writing process.
The collective evidence underscores ChatGPT’s transformative impact on writing and its substantial advancement over previous chatbot models. Its sophisticated text generation capabilities have positioned it as an invaluable resource in assisting writers across various domains, thereby underscoring the influential role it plays in shaping the landscape of writing and content generation. Assessing the scholarly discourse on ChatGPT, Dergaa et al. (2023) and Imran and Almusharraf (2023) underscore the necessity of harnessing ChatGPT as a valuable writing assistant tool in bolstering the writing process and enriching academic composition. Their contributions spotlight the pivotal role that ChatGPT assumes in elevating the quality of academic writing and streamlining the writing process within academic settings.
While the preceding user cases and academic discourse provide valuable insights into the potentials and challenges of integrating AI chatbots in education, it is critical to note that research in this area is still in its nascent phase (Barrot, 2023). Empirical research investigating the socio-technical dimensions of utilizing AI chatbots in higher education remains limited. There exists a need to delve into and assess the intention of postgraduate students regarding AI chatbots in learning and research, in addition to examining the influential factors at play. Such investigations hold the potential to elucidate postgraduate students’ acceptance of AI chatbots in educational and research contexts, offering valuable insights to leverage AI chatbots effectively within higher education.
Amid this landscape, it becomes evident that there is a pressing need to advance empirical research focusing on the integration of AI chatbots in higher education. Understanding postgraduate students’ perceptions and intentions towards AI chatbots can offer nuanced insights into the potential benefits and challenges associated with their utilization, thereby guiding the strategic integration of AI chatbots to augment the scholarly landscape within higher education. This underscores the necessity for further, in-depth investigations to not only understand postgraduates’ readiness to adopt AI chatbots but also to identify effective strategies to harness AI chatbots in advancing research and learning pursuits within higher education. Through such explorations, educators and researchers can gain critical insights essential for the effective leveraging of AI chatbots as pedagogical aids and in scholarly research within the higher education domain.
In 2003, Venkatesh and others constructed the Unified Theory of Acceptance and Use of Technology (UTAUT) model based on the integration and expansion of eight theories and models, including the Theory of Reasoned Action (TRA) and the Technology Acceptance Model (TAM). The UTAUT model introduces a more comprehensive theoretical framework to understand user technology acceptance and usage behavior by consolidating four core variables (Venkatesh et al., 2003, 447–453):
1. Performance Expectancy (PE): This refers to “the degree to which an individual believes that using the system will help him or her to attain gains in job performance”;
2. Effort Expectancy (EE): This refers to “the degree of ease associated with the use of the system”;
3. Social Influence (SI): This refers to “the degree to which an individual perceives that important others believe he or she should use the new system.”
4. Facilitating Conditions (FC): This refers to “the degree to which an individual believes that an organisational and technical infrastructure exists to support the use of the system.”
Moreover, four moderating variables were introduced: gender, age, experience, and voluntariness (Venkatesh et al., 2003).
While TAM models explain at most 40% of Behavioral Intentions (BI), UTAUT explains up to 70% of variability in user acceptance and usage intention (Holden and Karsh, 2009).
Multiple studies applied the UTAUT model to various digital landscapes.
1. In E-learning, PE, SI, and FC directly influenced students’ attitudes towards the usage of Moodle, with PE as the strongest determinant of students’ attitude (Šumak et al., 2010).
2. In E-Government, significant positive correlations were found between FC, PE, EE, SI, System Trust, and Net Ethics, influencing the Behavioral Intentions (BI) to use e-government services. Age, education level, and the BI to use e-government services have significant differences, excluding gender (Zeebaree et al., 2022).
3. In M-payment, the best predictor of the intention to use a mobile payment system was PE, followed by SI, EE, Perceived Trust, Perceived Cost, and Self-efficacy (Al-Saedi et al., 2020).
4. In AI, the correlation was found to be significantly positive between PE and BI to utilize AI in recruitment, with no significant impact discovered in terms of gender, age, experience, and education level (Horodyski, 2023).
5. In AI-assisted education, EE, PE, and SI were positively correlated with university students’ usage of AI-assisted learning, with Psychological Risk being a significant negative influence on the students’ BI (Wu et al., 2022).
6. In Web-based learning, PE, EE, Computer Self-efficacy, Achievement Value, Utility Value, and Intrinsic value were significant predictors of individuals’ intention to continue using web-based learning. Anxiety has a significant negative influence. Positive subjective task value is just as important as PE and EE in motivating learners’ intentions to continue using web-based learning (Chiu and Wang, 2008).
In sum, these studies stressed the significant influence of the UTAUT model in predicting and explaining the usage behavior across various digital technology domains.
In 2001, Bhattacherjee merged the Technology Acceptance Model (TAM) and Expectation Confirmation Theory (ECT) to create a new model for the continued utilization of information systems—the Expectation Confirmation Model (ECM). The ECM explains users’ post-adoption Use Behavior (UB) and the factors affecting their continued use intentions from aspects like expectation confirmation, perceived usefulness, and satisfaction. Comparing the actual utility after initial training with the expected usage, expectation confirmation impacts users’ perceived usefulness and satisfaction, thus indirectly affecting their sustained use. Perceived usefulness can also have a direct positive influence on user satisfaction. User Satisfaction is a crucial factor in influencing perceived usefulness and sustained use, while expectation confirmation and perceived usefulness are key prerequisites for satisfaction (Bhattacherjee, 2001).
Currently, the application of ECM primarily centers on information technology, encompassing the utilization of mobile applications and online platforms (Tam et al., 2020), acceptance of mobile advertising (Lu et al., 2019), and use of smart devices (Pal et al., 2020). Researchers often explore users’ satisfaction and their continued intent and behavior towards different technologies, products, or services by adjusting variables or integrating other models based on their research conditions. Jung-Chieh Lee and others used ECM to study how AI functions influence user’s continued intention to use mobile banking applications. The study indicated that both AI and anthropomorphic services can enhance user satisfaction by improving expectation confirmation and perceived usefulness, thus promoting continued mobile banking use (Lee et al., 2023). Neeraj Dhiman integrated the Task-Technology Fit (TTF) model with ECM to delve into the continued intent of users to use service chatbots during travelling. The results showed that when users find the technical characteristics of chatbots fit their tasks, their expectations are confirmed and directly impact their perceived usefulness. Both perceived usefulness and expectation confirmation positively influence user satisfaction towards chatbots, with perceived usefulness having the stronger impact (Neeraj and Jamwal, 2022). Baker-Eveleth used ECM to investigate students’ continued intent to use e-textbooks. The findings showed that students’ willingness to use e-textbooks is driven by satisfaction and perceived usefulness. Additionally, students’ expectation confirmation and the availability of e-textbooks have a positive impact on satisfaction and perceived usefulness, thus influencing the intention to continue using e-textbooks (Lori and Stone, 2015).
AI chatbots, considered landmarks in the new era of AI, has attracted considerable attention for its potential to revolutionize AI education. It is crucial to examine the factors that contribute to the sustained usage of AI chatbots by users, which has emerged as an important research inquiry. Media reports have highlighted the integration of AI chatbots in higher education institutions, providing valuable support to students in various academic activities such as essay writing assistance and question answering (Zhu and Wang, 2023). Consequently, investigating the determinants of AI chatbots adoption among university students, especially graduate students, holds paramount significance in this field.
Previous research has extensively utilized the UTAUT model to empirically examine the factors that influence users’ BI. These studies have established that the UTAUT model offers a more precise and effective explanation of users’ adoption and utilization behaviors towards technology (Holden and Karsh, 2009). For instance, El-Masri and Tarhini’s (2017) study on the acceptance of e-learning systems reveals a significant association between PE and BI. Similarly, Bao (2017) demonstrates that PE positively influences the adoption of mobile learning by open educational learners, while EE negatively impacts their willingness to adopt it. Wang and Mao (2016) assert that PE and EE serve as influential determinants affecting students’ engagement in online learning.
Building upon these findings, Lori and Stone (2015) highlight the considerable impact of PE, EE, and FC on the formation of students’ BI. These factors collectively shape students’ perceptions and attitudes towards online learning platforms. Meanwhile, El-Masri and Tarhini (2017) emphasize the crucial mediating role of “EE” in students’ intentions to continue using electronic learning systems, thus enhancing our understanding of the factors influencing sustained usage. Hu et al. (2020) and Raza et al. (2022) further support the critical influence of “EE” within the contexts of mobile learning and learning management systems, underscoring its significant impact on user acceptance and system adoption.
Furthermore, Zhang et al. (2023) provide compelling evidence of the causal relationship between social factors and users’ intention to adopt and utilize technology-mediated learning systems, thereby advancing our understanding of the intricate interplay between sociocultural elements and users’ BI. Wu et al. (2022) posit a positive correlation between variables of PE, SI, and FC with the adoption of AI-assisted learning among university students. Similarly, Zhai et al. (2022) proposes that FC indirectly impact the sustained usage intention of AI-assisted learning. Additionally, Zhang et al. (2016) examine the behavioral usage patterns of teachers in online learning environments, revealing significant influences of PE, EE, and SI on their sustained usage, with EE not substantially affecting their usage intention. In the domain of mobile learning, Wang et al. (2009) and Donaldson (2010) investigate the factors influencing users’ acceptance of mobile learning, emphasizing the importance of PE, EE, and SI. Moreover, the role of gender and age as moderating variables in these associations has been emphasized. Various studies, such as those conducted by Nikolopoulou et al. (2020) in the realm of mobile learning, Samsudeen and Mohamed (2019) concerning e-learning platforms, and Ain et al. (2016) focusing on learning management systems, underscore the significance of FC as determinant of learners’ BI and UB. These factors play a critical role in individual technology usage. Additionally, in the context of mobile learning (Kang et al., 2015), e-learning platforms, and augmented reality, FC has been recognized as pivotal factors influencing the adoption of diverse educational technologies in higher education. Furthermore, Wan and Zhao (2016) have identified substantial impacts of both FC and BI on UB. Drawing upon the insights, this study formulates the following hypotheses to examine the causal relations between PE, EE, SI, FC and BI when AI Chatbots are used:
H1: PE has direct and significant impact on BI.
H2: EE has direct and significant impact on BI.
H3: SI has direct and significant impact on BI.
H4: FC has direct and significant impact on BI.
H5: FC has direct and significant impact on UB.
H12: BI has direct and significant impact on UB.
The concept of “Satisfaction (SA)” in the context of information technology usage pertains to the subjective evaluation by users, reflecting their overall contentment with the technology (Gan and Wang, 2015). Notably, Gan and Wang (2015) reveals a strong positive correlation between satisfaction and sustained UB. This finding aligns with the work of Cidral et al. (2018), who emphasize the crucial role of SA as a fundamental construct for assessing the long-term adoption and continued usage of electronic learning systems. Furthermore, Zhang and Bai (2017) assert the prominent influence of SA as a critical factor impacting users’ enduring engagement with such systems. Overall, these scholarly insights underscore the significance of SA as a key determinant shaping users’ sustained UB in the context of information technology adoption.
In ECM, Confirmation (CF) is defined as “the users’ level of the appropriateness between their actual performance and expectation of the usage of information systems and services” (Hsu and Lin, 2015). ECM posits that users’ perceived “Confirmation (CF)” indirectly influences their BI by impacting factors of SA and “Perceived ease of use (PEU)” (Bhattacherjee, 2001). Notably, within this study, the selected variable representing “PEU” aligns with the concept of “PE” in the UTAUT model. This deliberate choice enhances the theoretical continuity and integration between the Technology Acceptance Model (TAM) and the UTAUT model, ensuring a comprehensive examination of user behavior in the present research context.
Li and Zhao (2016) highlight the pivotal roles of CF and SA in shaping UB. Zhu (2017) conducted a rigorous empirical study in the domain of online learning spaces, yielding significant findings regarding the relationship between SA and students’ intention to maintain their usage. This study demonstrates the influential role of SA in fostering a positive inclination among students to continue utilizing online learning platforms. Expanding on these foundations, Bhattacherjee (2001) emphasizes the significant impact of CF on user SA, thus indirectly affecting their sustained usage. Additionally, Yang et al. (2015) also underscores the consequences of CF on both user satisfaction and the intention to continue using. It is not clear whether those findings apply for the adoption of AI Chatbots. Accordingly, the following hypotheses were proposed:
H6: SA positively influences BI.
H7: PE positively influences SA.
H8: EE positively influences SA.
H9: CF positively influences PE.
H10: CF positively influences SA.
“Personal innovativeness (PI)” refers to an inherent characteristic that reflects an individual’s cognitive orientation and propensity for action (Xiong, 2015). Notably, Agarwal and Karahanna (2000) introduced the concept of PI in the context of information technology to shed light on users’ willingness to adopt novel technological advancements. Subsequent research in various information technology domains consistently acknowledges the significant influence of personal innovativeness on users’ acceptance of emerging technologies. For example, Twum et al. (2022) demonstrate the substantial impact of personal innovativeness on users’ adoption of electronic platforms, while Dajani and Abu Hegleh (2019) emphasize its pivotal role as a determinant in university students’ utilization of animation. Additionally, Sitar-Taut and Mican (2021) highlight the distinct significance of PI in the domain of mobile learning.
Within the higher education context, personal innovativeness serves as a crucial supplementary construct in the UTAUT model. The UTAUT model posits that user characteristics, such as gender, age, experience, and voluntariness, act as influential moderators across the core dimensions of acceptance and use behavior. Considering the specific context of the present study where participants engaged voluntarily with AI Chatbots and possessed limited experience with the technology, the moderator variables of voluntariness and experience were excluded. Building upon these considerations, the present study proposes the following hypothesis:
H11: Personal innovativeness has positive impact on BI.
Figure 1 displays the study’s hypothesized model, which includes seven predictors, four of which are originally from UTAUT model, two from ECM model, and an additional external variable, “Personal innovativeness.”
The survey encompassed master’s and doctoral students from various Chinese universities like Xinjiang University, Ocean University of China, Dalian University of Science and Technology. It was conducted via the “Wenjuanxing” platform from May 30, 2023, and extended for two weeks. The survey was administered three times to different cohorts of graduate students, resulting in the collection of 637 questionnaires. After data cleaning, 373 valid responses remained (only those completed in at least 100 s and with distinct scores for all items were retained), yielding an effective response rate of 58.6%.
Table 1 presents the demographic characteristics of the study participants. Out of the 373 respondents, there were 193 males and 180 females. The majority of participants were master’s students, with an average age range of 20–25 years. Master’s students constituted 84.2% of the total graduate student population, while the ratio of master’s to doctoral students was approximately 5.3:1. Comparing this with data from the National Bureau of Statistics of China (NBSC) (2022), which reported that master’s students comprised about 84.7% of the graduate student population in 2021, with a master’s to doctoral student ratio of approximately 5.5:1 (National Bureau of Statistics of China (NBSC), 2022), it suggests that the sampling distribution in our study aligns well with the actual population and demonstrates strong representativeness.
The research instrumentation in this study consisted of two components. The first component captured demographic information such as participants’ cities, gender, ages, and educational background. The second component utilized a seven-point Likert scale, ranging from “1 = strongly disagree/unsatisfied” to “7 = strongly agree/satisfied,” to collect responses. This scale aimed to assess the factorial structure of UTAUT and ECM in relation to Chinese graduates’ willingness to utilize an AI chatbot. To ensure consistent estimation, we established a numerical metric scale from 1 to 7, associating specific frequencies with each point on the scale. This scale is defined as follows: “Never” corresponds to 1, “Once a month” corresponds to 2, “Several times a month” corresponds to 3, “Once a week” corresponds to 4, “Several times a week” corresponds to 5, “Once a day” corresponds to 6, and “Several times a day” corresponds to 7.
In the second part of the instrumentation, a total of 29 items were developed by drawing from previous research studies conducted by Agarwal and Prasad (1998), Bhattacherjee (2001), Venkatesh et al. (2003), Lee (2010), Venkatesh and Xu (2012), and Yang (2016). These items covered various constructs, including “PE” (four items), “EE” (three items), “SI” (three items), “FC” (four items), “CF” (three items), “SA” (four items), “PI” (four items), “BI” (three items), and “UB” (one item). To ensure linguistic equivalence, a forward-backward translation procedure was employed, following the method suggested by Li et al. (2019) and Brislin (1986). Firstly, an English teacher translated the items into Chinese, and then another English teacher back-translated the Chinese version into English. Any discrepancies in the translations were resolved through negotiation and adjustments in wording. The accuracy of the Chinese translations was confirmed by the high similarity observed between the two versions. For detailed information on the measurement scale and descriptive statistics, please refer to Table 2.
Data analysis in this study was conducted using the Statistical Package for the Social Sciences (SPSS) and the PLS-SEM (Partial Least Squares Structural Equation Modeling) method. PLS-SEM combines principal component analysis with ordinary least squares regression and was employed to estimate the hypothesized study model. The selection of PLS-SEM was based on two reasons: First, the study adopted a composite-based model for theory development, which is both causal and predictive (Hair et al., 2021). Second, PLS-SEM is robust to data distribution assumptions, making it suitable for analysis in this study (Hair et al., 2021).
The PLS-SEM entails two main phases: the measurement model and the structural model. The measurement model establishes the relationships between latent variables (unobserved variables) and measurement variables (observed variables) to explain causal relationships (Wang and Cao, 2020). PLS-SEM, a non-parametric structural equation modeling method, is suitable for non-normal, small sample, and exploratory studies. It does not strictly assume sample distribution and handles complex structural equation models. A minimum sample size of 10 times the number of indicators is recommended (Haenlein and Kaplan, 2004; Hair et al., 2021).
With 373 valid samples and 29 items, the sample-to-indicator ratio in this study met the PLS-SEM requirement of at least a 10:1 ratio (Kock, 2018).
In assessing the measurement model, this study conducted an evaluation of factor loadings, reliability, convergence validity, and discriminant validity, following the approach outlined by Hair et al. (2021). The assessment utilized Cronbach’s alpha, AVE (average variance extracted), and CR (composite reliability) as reliability indicators, in line with the guidance provided by Bagozzi and Yi (1988). These metrics were computed using the PLS Algorithm in Smart PLS, with the results detailed in Table 3. Reliability serves as an indicator of the questionnaire’s consistency and stability, with higher values signaling greater questionnaire reliability and internal consistency.
Cronbach’s alpha is commonly used as a measure of overall scale reliability, with a value greater than 0.70 considered acceptable. A higher Cronbach’s alpha coefficient indicates a higher level of reliability (Hair et al., 2021). According to Fornell and Larcker (1981), AVE (average variance extracted) value greater than 0.50 is considered ideal, while a range of 0.30–0.50 is deemed acceptable. When both AVE and CR exceed 0.50 and 0.70 respectively, it indicates good reliability of the factors. Table 3 presents the results, indicating that the lowest values for Cronbach’s alpha (0.717), AVE (0.633), and CR (0.842) in this study surpass the general standards (Cronbach’s alpha >0.70, AVE > 0.50, and CR > 0.70). Moreover, considering that the questionnaire was adapted from a mature model, it demonstrates a desirable level of reliability and consistency in its structure.
The questionnaire in this study has undergone meticulous development, drawing on empirical studies within the same thematic or disciplinary domain, which significantly contributes to its high content validity. Hair et al. (2021) emphasizes the importance of both convergent validity and discriminant validity. Convergent validity is established when factor loadings exceed 0.70, and it is considered acceptable when they exceed 0.50. From Figure 2 and Table 2, all factor loadings exceed 0.70, except for FC4, which exceeds 0.50, indicating high convergent validity. Additionally, Fornell and Larcker (1981) suggest an AVE value of at least 0.50 for convergent validity. As shown in Table 3, all AVE values surpass 0.50, further supporting high convergent validity.
Discriminant validity was assessed using the heterotrait-monotrait ratio of correlations (HTMT) proposed by Henseler et al. (2015). The HTMT threshold of 0.90 was used to evaluate effective discrimination. Table 4 shows that all item measurement values are below 0.90, indicating good discriminant validity.
Through the higher-order structural model, we examined the causal relationships between latent variables and evaluated the model’s predictive power. To assess this, we employed various measures such as the variance inflation factor (VIF), path coefficients, f-squared, and R-squared (Stone, 1976). The model’s path parameters were estimated, and the significance of the path coefficients was evaluated using the Bootstrapping algorithm (Chin, 1998) with 5,000 resamples from valid data, as shown in Table 5. Additionally, we tested the moderation effects of “gender” and “age” in the model and assessed their significance, as presented in Table 6. Collinearity was assessed using VIF, with a criterion of VIF values less than 5 for all endogenous variables, as outlined by Hair et al. (2021). In our analysis, all path VIF values were below 5, indicating the absence of collinearity, as shown in Table 7.
Table 7 provides statistical confirmation for each path in the model. The f-squared analysis was used to estimate the strength of the relationships between variables, with values greater than 0.02, 0.15, and 0.35 indicating small, medium, and large effects, respectively (Hair et al., 2021). The table also displays collinearity results, showing the relationships between endogenous variables and their regression weights with exogenous variables (path coefficients), indicating the presence of multicollinearity among the indicators.
Table 8 evaluates the model’s accuracy using the coefficient of determination, R-squared, which measures the explanatory power of the model. Ranging from 0 to 1, a higher value indicates stronger explanatory power, where 0.75, 0.50, and 0.25 are considered substantial, moderate, and weak, respectively (Hair et al., 2021). The endogenous variables BI (R2 = 0.639), PE (R2 = 0.447), and SA (R2 = 0.570) exhibit strong explanatory power, while UB1 (R2 = 0.216) has weaker explanatory power. Q-squared values are employed to assess the impact of exogenous variables on endogenous constructs (Stone, 1976). Q-squared values greater than 0.02, 0.15, and 0.35 indicate small, medium, and large predictive relevance, respectively (Hair et al., 2021). As shown in Table 8, the endogenous variables BI (Q2 = 0.453), PE (Q2 = 0.444), SA (Q2 = 0.469), and UB1 (Q2 = 0.131) demonstrate strong predictive capability. The model fit was assessed using the Goodness of Fit (GOF) proposed by Tenenhaus et al. (2004) and the Standardized Root Mean Square Residual (SRMR) proposed by Henseler and Sarstedt (2012). Both GOF and SRMR were used to evaluate the model’s fit, as researchers have debated their relative importance (Henseler and Sarstedt, 2012).
Table 8 provides the GOF values and SRMR values to evaluate the model fit. GOF values of 0.01, 0.25, and 0.36 are considered small, medium, and large, respectively. For this study, the computed GOF value is approximately 0.419, indicating a good overall fit of the model. The SRMR, which should be less than 0.08 for a good fit, is calculated as 0.052 in this study.
Confirmation of hypotheses relies on the t-values and p-values presented in Table 5. A hypothesis can be confirmed if the p-value is ≤0.05 and the t-value is ≥1.96. Based on Table 5, eight hypotheses (H1, H6, H7, H8, H9, H10, H11, H12) are supported, while the remaining four hypotheses (H2, H3, H4, H5) are not supported. The path coefficients for the structural model are shown in Figure 3, and the t-values for the structural model are displayed in Figure 4.
Our study investigates the integration of AI Chatbots among Chinese postgraduate students, merging the UTAUT and ECM models. Despite the limited prior exploration of this subject, particularly in higher education settings, our findings hold significant implications for advancing the understanding of AI chat technologies as educational tools. Notably, our results confirm a positive correlation between “Performance Expectancy (PE)” and “Behavioral Intention (BI)” among postgraduates regarding AI Chatbot usage, aligning with the observations made by El-Masri and Tarhini (2017) in their study of e-learning system acceptance. This positive association between “PE” and “BI” has been consistently observed in various domains, including learning management software (Kang et al., 2015), mobile learning (Kumar and Bervell, 2019), and online learning (Chiu and Wang, 2008). Previous research consistently identifies “PE” as a critical predictor of “BI.”
This substantive association between “PE” and “BI” underscores the critical role of performance expectancy in shaping the behavioral intention towards technology adoption, a pattern evident across diverse educational realms. Understanding this relationship within the context of AI chatbot integration among postgraduate students not only provides insights into their acceptance, but also highlights the influential role of user experience in driving their intention to utilize AI chatbots as educational resources. Moreover, this analysis emphasizes the transferability of these insights from prior technological adoption studies to the domain of AI chatbot implementation within higher education, signifying the overarching significance of performance expectancy in shaping behavioral intent within educational technology adoption.
Meanwhile, our examination reveals that “Effort Expectancy (EE)” demonstrates no statistically significant impact on “Behavioral Intention (BI)” of postgraduates on using AI Chatbot for academic research, aligning with the findings of EE on BI reported by Bao (2017). This indicates that the adoption of AI Chatbots in higher education necessitates minimal effort without significantly influencing users’ intention to utilize them. Additionally, a substantial positive relationship is observed between “Performance Expectancy (PE)” and “Effort Expectancy (EE)” with “Satisfaction (SA).” Specifically, the support offered by AI Chatbots to graduate students in their research and learning endeavors, along with their comprehension of the feedback received, impacts the levels of satisfaction following actual usage. “Satisfaction” emerges as the primary and most influential predictor of “BI.”
Furthermore, our study unveils that both “Social Influence (SI)” and “Effort Expectancy (EE)” do not exert a significant impact on “Behavioral Intention (BI),” delineating insignificant effect sizes and deviating from the UTAUT model. The lack of substantial influence from “Social Influence (SI)” on the adoption of AI Chatbots by graduate students can be attributed to two primary factors. First, this outcome is likely associated with the heightened educational background of the participants, indicative of a higher level of discernment and reduced susceptibility to external influences. Second, it can be ascribed to the relatively limited usage and uptake of AI Chatbot technology among the target population in China, which has not gained widespread acceptance and recognition in society.
This observation aligns with previous studies (Kumar and Bervell, 2019; Alotumi, 2022), which similarly reported no significant impact of “Social Influence (SI)” on users’ sustained usage. These findings collectively underscore the complex interplay of factors influencing the adoption of AI Chatbots in higher education and pave the way for a deeper understanding of the nuanced dynamics at play. Further exploration of these layered dynamics can provide essential insights for refining the implementation and utilization of AI Chatbots within the educational landscape.
In addition, the impact of “Facilitating Conditions (FC)” on users’ sustained usage of AI Chatbots is not found to be statistically significant. This can be attributed to the perceived ease of use and comprehension among graduate students, who belong to a generation well-versed in internet technology, when interacting with AI Chatbots. Simple prompts suffice for them to complete tasks without necessitating additional resources or equipment. Consequently, “FC” does not significantly influence users’ intention for continued use. Our study also reaffirms the noteworthy impact of “Satisfaction” on “Behavioral Intention (BI)” of postgraduates on using AI Chatbots, in line with earlier research (Gan and Wang, 2015; Li and Zhao, 2016) of “Satisfaction” on “Behavioral Intention (BI).”
Moreover, “Confirmation (CF)” positively influences both “Performance Expectancy (PE)” and “Satisfaction (SA)” on graduate students using AI Chatbots on research. Within the ECM model, the level of confirmation users receive regarding their expectations before usage assumes a critical role in shaping their satisfaction. Greater confirmation leads to heightened satisfaction, subsequently driving continued usage. Notably, in our investigation, “CF” emerges as the primary and most influential predictor of “SA.” Additionally, “Personal Innovativeness (PI)” exhibits a significant influence on “BI” while serving as the second most influential predictor. AI Chatbots represent not only an innovation in traditional learning methods but also a technological innovation, positioning “PI” as a crucial individual difference factor.
Regarding moderating variables, our analysis indicates that the connections between the predictors and the dependent variable are not substantially impacted by the moderating variables of “Gender” and “Age.” This indicates the robustness and consistency of the observed patterns in influencing users’ behavioral intentions towards AI Chatbots, irrespective of gender and age, underlining the broad applicability and reliability of the identified predictors in shaping user intent and behavior. This comprehensive understanding of the multifaceted factors and their interplay not only enriches our comprehension of AI chatbots adoption but also offers valuable insights for tailoring strategies to optimize their effective implementation within higher education settings.
In our current investigation, we have empirically verified 12 hypotheses and revealed a robust relationship between CF, SA, and BI in the ECM model, surpassing the strength of the relationship between UTAUT constructs and BI. This suggests that users of information technology, notably AI Chatbots as examined in our study of Chinese graduate students, engage in decision-making processes akin to consumer decisions for repeated consumption and purchases in marketing. The alignment between users’ expectations of an AI Chatbot and their initial experience significantly impacts their decision and intention to continue usage. Moreover, users’ actual satisfaction with an AI Chatbot is pivotal in shaping their subsequent intentions. This highlights the parallel mechanisms between consumer behavior and technology acceptance, emphasizing the relevance of user experience and satisfaction in shaping continued usage intentions.
The existing literature predominantly focuses on the future development of AI Chatbots and the industry’s response to their impact, particularly ChatGPT. However, there is a dearth of research examining students’ perspectives and acceptance of AI Chatbots, specifically in China. Students express a strong desire for the integration of AI Chatbots in higher education, expecting them to positively impact their educational development. Some researchers have conducted interviews with ChatGPT (Lund and Wang, 2023) to explore its influence on education. The utilization of AI Chatbots in academia and higher education is relatively new and still in the exploratory stage. In this study, we adopted the UTAUT model as the foundational framework, while also incorporating elements from the ECM to further enhance our evaluation of Chinese graduate students’ attitudes and intentions towards using AI Chatbots.
Interestingly, we found that certain items within the UTAUT model inadequately captured the perspectives of Chinese graduate students on the acceptance and utilization of AI Chatbots. Conversely, constructs such as “Confirmation” and “Satisfaction” from the ECM model demonstrated better efficacy in assessing the technological acceptance levels among Chinese graduate students, particularly their perceptions of AI Chatbots. Notably, “Satisfaction” and “Personal innovativeness” emerged as critical factors significantly influencing Chinese graduate students’ intentions to accept and utilize AI Chatbots. It is essential to highlight the scarcity of previous research on the adoption and acceptance of AI Chatbots in Chinese higher education, underscoring the novelty and significance of our study. As a result, the findings from this study will contribute to enhancing our understanding of AI Chatbot deployment in higher education and facilitating the advancement and optimization of AI Chatbot applications within educational contexts.
The integration of AI chatbots in higher education pedagogy, as instrumental adjuncts in fostering research writing aptitudes, affords a multifarious linguistic repertoire and proffers critical feedback, thereby mitigating the anxieties surrounding academic writing endeavors (Guo et al., 2022). The study at hand substantiates that “Satisfaction” is a cardinal determinant in the behavioral intention (BI) of postgraduate scholars’ engagement with AI chatbots for research. To amplify scholarly rigor and assimilate AI chatbots seamlessly into the pedagogical milieu of Chinese graduate students, a pivot towards user-centric methodologies is imperative. Such approaches mandate an intimate acquaintance with academic exigencies and a relentless pursuit of strategically adaptive solutions.
Key considerations also include ensuring the authenticity and factual accuracy of interactions, ensuring data confidentiality, and exploring heightened collaboration between human intellect and computational systems to achieve a synergy of human-machine intelligence. The practical implications of these strategies for the educational sector, particularly within the investigated acceptance of AI Chatbots among graduate students for research and study, are multifaceted. Firstly, the emphasis on user-centric methodologies highlights the need for educational institutions to customize AI Chatbot interventions to correspond with students’ specific research and learning requisites. This underlines the importance of understanding postgraduates’ unique research demands, ensuring that AI Chatbots are tailored to enhance and streamline the research process, ultimately contributing to improved research outcomes and student satisfaction.
Moreover, the recognition of student satisfaction as a decisive factor in their intent to leverage AI Chatbots for research emphasizes the significance of AI Chatbots in enriching the research experience, thereby potentially enhancing academic productivity and knowledge creation. From a policy perspective, the practical implications necessitate the formulation of guidelines that promote ethical utilization of AI technologies, ensuring data privacy, and fostering transparent interactions. This underlines the imperative for educational policymakers to adopt a balanced approach that encourages innovation while prioritizing student well-being and ethical considerations. Additionally, fostering a regulatory framework that emphasizes the ethical and effective integration of AI technologies within the scholarly research landscape is pivotal in ensuring the responsible implementation of AI Chatbots in higher education.
This study is not without limitations, all of which merit consideration. Firstly, it is essential to acknowledge the limitations of the UTAUT model, given that the majority of empirical research validating this model has been primarily conducted in developed countries. This raises the critical necessity for expanded empirical scrutiny to ascertain its applicability in distinct contexts, particularly in China, as underscored by Xiong (2015). Secondly, this study did not account for the respondents’ prior experience or duration of use with AI Chatbots, generating concerns regarding the uniformity and depth of users’ familiarity and engagement with the technology. Additionally, the geographical focus of the study primarily centered on provincial capitals and major cities, which potentially restricted the representation of perspectives from smaller cities. Furthermore, one must acknowledge that the level of economic development in these cities may significantly impact users’ acceptance and adoption of new technologies. To augment the scholarly rigor of future research in this burgeoning field, it is advisable to conduct a comprehensive assessment of the scales employed in this study and contemplate the integration of contextual factors to enhance the study’s validity and generalizability.
Considering the limitations, future research endeavors could delve into rectifying the identified shortcomings and further illuminating the understanding of AI Chatbot acceptance among graduate students for research and study purposes. Given the significance of contextual factors, subsequent research could aim to analyze the nuanced impacts of economic development and regional disparities on AI Chatbot acceptance within varied geographical settings in China. Additionally, delving into the role of prior experience and the duration of AI Chatbot use among respondents could elucidate the influence of familiarity and proficiency with the technology on their acceptance and utilization. Furthermore, future studies could endeavor to validate and refine the UTAUT model within the specific context of Chinese higher education, offering insights into its cross-cultural applicability and potential areas for modification or extension to suit the Chinese educational landscape. Through these future research efforts, the scholarly community would gain a more comprehensive understanding of AI Chatbot integration within scholarly settings, thereby refining pedagogical strategies and technological implementation to better serve graduate students’ academic needs.
The original contributions presented in the study are included in the article/supplementary material, further inquiries can be directed to the corresponding author.
WT: Methodology, Project administration, Supervision, Writing – original draft, Writing – review & editing, Formal analysis, Funding acquisition, Investigation. JG: Methodology, Project administration, Supervision, Writing – original draft, Writing – review & editing, Conceptualization. YZ: Visualization, Writing – original draft. XZ: Visualization, Writing – review & editing.
The author(s) declare financial support was received for the research, authorship, and/or publication of this article. This work was supported by Basic research funds for colleges and universities in Autonomous region (XJEDU2022P031), 2022 Autonomous Region ‘Tianchi Talent’ Introduction Plan Project, and Shandong Social Science Planning Project (21DYYJ05).
We would like to express our gratitude to editors and reviewers for their extraordinarily helpful comments.
The authors declare that the research was conducted in the absence of any commercial or financial relationships that could be construed as a potential conflict of interest.
All claims expressed in this article are solely those of the authors and do not necessarily represent those of their affiliated organizations, or those of the publisher, the editors and the reviewers. Any product that may be evaluated in this article, or claim that may be made by its manufacturer, is not guaranteed or endorsed by the publisher.
Achiam, J. (2023). GPT-4 Technical Report. Available at: https://openai.com/research/gpt-4 (Accessed July 02, 2023).
Agarwal, R., and Karahanna, E. (2000). Time flies when you’re having fun: cognitive absorption and beliefs about information technology usage. MIS Q. 24, 665–694. doi: 10.2307/3250951
Agarwal, R., and Prasad, J. (1998). A conceptual and operational definition of personal innovativeness in the domain of information technology. Inf. Syst. Res. 9, 204–215. doi: 10.1287/isre.9.2.204
Ain, N., Kaur, K., and Waheed, M. (2016). The influence of learning value on learning management system use. Inf. Dev. 32, 1306–1321. doi: 10.1177/0266666915597546
Alotumi, M. (2022). Factors influencing graduate students’ behavioral intention to use Google classroom: case study-mixed methods research. Educ. Inf. Technol. 27, 10035–10063. doi: 10.1007/s10639-022-11051-2
Al-Saedi, K., Al-Emran, M., Ramayah, T., and Abusham, E. (2020). Developing a general extended UTAUT model for M-payment adoption. Technol. Soc. 62:101293. doi: 10.1016/j.techsoc.2020.101293
Aung, Y. Y. M., Wong, D. C. S., and Ting, D. S. W. (2021). The promise of artificial intelligence: a review of the opportunities and challenges of artificial intelligence in healthcare. Br. Med. Bull. 139, 4–15. doi: 10.1093/bmb/ldab016
Bagozzi, R. P., and Yi, Y. (1988). On the evaluation of structural equation models. J. Acad. Mark. Sci. 16, 74–94. doi: 10.1007/BF02723327
Bao, M. (2019). Can home use of speech-enabled artificial intelligence mitigate foreign language anxiety–investigation of a concept. Arab World Engl. J. (AWEJ) Special Issue on CALL. 5, 28–40. doi: 10.24093/awej/call5.3
Bao, R. (2017). Factors influencing the intention to use mobile learning among open education learners. J. Dist. Educ. 3, 102–112. doi: 10.15881/j.cnki.cn33-1304/g4.2017.03.012
Barrot, J. S. (2023). Using ChatGPT for second language writing: pitfalls and potentials. Assess. Writ. 57:100745. doi: 10.1016/j.asw.2023.100745
Bhattacherjee, A. (2001). Understanding information systems continuance: an expectation-confirmation model. MIS Q. 25, 351–370. doi: 10.2307/3250921
Brislin, R. W. (1986). “The wording and translation of research instruments,” In Field methods in cross-cultural research. eds. W. Lonner and J. Berry (Beverly Hills, CA: Sage), 37–164.
Chin, W. W. (1998). The partial least squares approach for structural equation modeling. Adv. Hosp. Leis. 8, 295–336.
Chiu, C.-M., and Wang, E. T. G. (2008). Understanding web-based learning continuance intention: the role of subjective task value. Inf. Manag. 45, 194–201. doi: 10.1016/j.im.2008.02.003
Cidral, W. A., Oliveira, T., Di Felice, M., and Aparicio, M. (2018). E-learning success determinants: Brazilian empirical study. Comput. Educ. 122, 273–290. doi: 10.1016/j.compedu.2017.12.001
Dajani, D., and Abu Hegleh, A. S. (2019). Behavioral intention of animation usage among university students. Heliyon 5:e02536. doi: 10.1016/j.heliyon.2019.e02536
Dergaa, I., Chamari, K., Zmijewski, P., and Saad, H. B. (2023). From human writing to artificial intelligence generated text: examining the prospects and potential threats of ChatGPT in academic writing. Biol. Sport 40, 615–622. doi: 10.5114/biolsport.2023.125623
Donaldson, R. L. (2010). Student acceptance of mobile learning. Tallahassee, FL: The Florida State University.
Dwivedi, Y. K., Kshetri, N., Hughes, L., Slade, E. L., Jeyaraj, A., Kar, A. K., et al. (2023). “So what if ChatGPT wrote it?” multidisciplinary perspectives on opportunities, challenges and implications of generative conversational AI for research, practice and policy. Int. J. Inf. Manag. 71:102642. doi: 10.1016/j.ijinfomgt.2023.102642
El-Masri, M., and Tarhini, A. (2017). Factors affecting the adoption of e-learning systems in Qatar and USA: extending the unified theory of acceptance and use of technology 2 (UTAUT2). Educ. Technol. Res. Dev. 65, 743–763. doi: 10.1007/s11423-016-9508-8
Farrokhnia, M., Banihashem, S. K., Noroozi, O., and Wals, A. (2023). A SWOT analysis of ChatGPT: implications for educational practice and research. Innov. Educ. Teach. Int., 1–15. doi: 10.1080/14703297.2023.2195846
Fornell, C., and Larcker, D. (1981). Structural equation models with unobservable variables and measurement error: algebra and statistics. J. Mark. Res. 18, 382–388. doi: 10.1177/002224378101800313
Gan, C., and Wang, W. (2015). Continuance intention of academic blogs: the effects of interactivity, immersion, and satisfaction. Inf. Sci. 3, 70–74. doi: 10.13833/j.cnki.is.2015.03.014
Guo, K., Wang, J., and Chu, S. K. W. (2022). Using chatbots to scaffold EFL students’ argumentative writing. Assess. Writ. 54:100666. doi: 10.1016/j.asw.2022.100666
Haenlein, M., and Kaplan, A. M. (2004). A beginner’s guide to partial least squares analysis. Underst. Stat. 3, 283–297. doi: 10.1207/s15328031us0304_4
Hair, J. F., Hult, G. T. M., Ringle, C. M., and Sarstedt, M. (2021). A primer on partial least squares structural equation modeling (PLS-SEM). Thousand Oaks, CA: Sage.
Hamoudi, R. (2023). Artificial intelligence in medicine and medical education. Adv. Biomed. Health Sci. 2:1. doi: 10.4103/abhs.abhs_69_22
Harrison, M., (2023). ChatGPT’s explosive popularity makes it the fastest-growing app in human history. The Byte. Available at: https://futurism.com/the-byte/chatgpts-fastest-growing-app-human-history
Henseler, J., Ringle, C. M., and Sarstedt, M. (2015). A new criterion for assessing discriminant validity in variance-based structural equation modeling. J. Acad. Mark. Sci. 43, 115–135. doi: 10.1007/s11747-014-0403-8
Henseler, J., and Sarstedt, M. (2012). Goodness-of-fit indices for partial least squares path modeling. Comput. Stat. 28, 565–580. doi: 10.1007/s00180-012-0317-1
Holden, R. J., and Karsh, B. T. (2009). The technology acceptance model: its past and its future in health care. J. Biomed. Inform. 43, 159–172. doi: 10.1016/j.jbi.2009.07.002
Horodyski, P. (2023). Recruiter's perception of artificial intelligence (AI)-based tools in recruitment. Comput. Hum. Behav. Rep. 10:100298. doi: 10.1016/j.chbr.2023.100298
Hou, L., and Li, Z. (2023). ChatGPT legal challenges and institutional responses in academic use. J. Northeast Norm. Univ. (Philos. Soc. Sci. Edition) 324, 29–39. doi: 10.16164/j.cnki.22-1062/c.2023.04.004
Hsu, C.-L., and Lin, J. C.-C. (2015). What drives purchase intention for paid mobile apps? An expectation confirmation model with perceived value. Electron. Commer. Res. Appl. 14, 46–57. doi: 10.1016/j.elerap.2014.11.003
Hu, S., Laxman, K., and Lee, K. (2020). Exploring factors affecting academics’ adoption of emerging mobile technologies-an extended UTAUT perspective. Educ. Inf. Technol. 25, 4615–4635. doi: 10.1007/s10639-020-10171-x
Imran, M., and Almusharraf, N. (2023). Analyzing the role of ChatGPT as a writing assistant at higher education level: a systematic review of the literature. Contemp. Educ. Technol. 15:ep464. doi: 10.30935/cedtech/13605
Kang, M., Liew, B. Y. T., Lim, H., Jang, J., and Lee, S. (2015). Lecture notes in educational technology. 9783662441879: 209–216.
Kasneci, E., Sessler, K., Küchemann, S., Bannert, M., Dementieva, D., Fischer, F., et al. (2023). ChatGPT for good? On opportunities and challenges of large language models for education. Learn. Individ. Differ. 103, 1–9. doi: 10.1016/j.lindif.2023.102274
Kock, N. (2018). “Minimum sample size estimation in PLS-SEM: An application in tourism and hospitality research,” In Applying partial least squares in tourism and hospitality research. Emerald Publishing Limited. 1–16.
Kumar, J. A., and Bervell, B. (2019). Google classroom for mobile learning in higher education: modelling the initial perceptions of students. Educ. Inf. Technol. 24, 1793–1817. doi: 10.1007/s10639-018-09858-z
Lee, M. C. (2010). Explaining and predicting users’ continuance intention toward e-learning: An extension of the expectation-confirmation model. Comput. Educ. 54, 506–516. doi: 10.1016/j.compedu.2009.09.002
Lee, J., Tang, Y., and Jiang, S. (2023). Understanding continuance intention of artificial intelligence (AI)-enabled mobile banking applications: an extension of AI characteristics to an expectation confirmation model. Humanit. Soc. Sci. Commun. 10, 1–12. doi: 10.1057/s41599-023-01845-1
Li, R., Meng, Z., Tian, M., Zhang, Z., Ni, C., and Xiao, W. (2019). Examining EFL learners’ individual antecedents on the adoption of automated writing evaluation in China. Comput. Assist. Lang. Learn. 32, 784–804. doi: 10.1080/09588221.2018.1540433
Li, W., and Zhao, X. (2016). Research on the continuance intention and mechanism of social reading app usage among college students. J. Chin. Libr. Inf. Sci. 1, 52–65. doi: 10.13530/j.cnki.jlis.160005
Lori, B.-E., and Stone, R. W. (2015). Usability, expectation, confirmation, and continuance intentions to use electronic textbooks. Behav. Inform. Technol. 34, 992–1004. doi: 10.1080/0144929X.2015.1039061
Lu, C.-C., Wu, I.-L., and Hsiao, W.-H. (2019). Developing customer product loyalty through mobile advertising: affective and cognitive perspectives. Int. J. Inf. Manag. 47, 101–111. doi: 10.1016/j.ijinfomgt.2018.12.020
Lund, B. D., and Wang, T. (2023). Chatting about ChatGPT: how may AI and GPT impact academia and libraries? Library Hi Tech News 40, 26–29. doi: 10.1108/LHTN-01-2023-0009
MacNeil, S., Tran, A., Mogil, D., Bernstein, S., Ross, E., and Huang, Z. (2022). “Generating diverse code explanations using the GPT-3 large language model,” In Proceedings of the 2022 ACM Conference on International Computing Education Research. 2, 37–39. doi: 10.1145/3501709.3544280
Mageira, K., Pittou, D., Papasalouros, A., Kotis, K., Zangogianni, P., and Daradoumis, A. (2022). Educational AI chatbots for content and language integrated learning. Appl. Sci. 12:3239. doi: 10.3390/app12073239
Medina, J. B., and Ferrer, J. R. C. (2022). Using Chatbots as AI conversational partners in language learning. Appl. Sci. 12:8427. doi: 10.3390/app12178427
Moore, S., Nguyen, H. A., Bier, N., Domadia, T., and Stamper, J. (2022). “Assessing the quality of student-generated short answer questions using GPT-3[C],” In European conference on technology enhanced learning. Cham: Springer International Publishing, 243–257.
National Bureau of Statistics of China (NBSC). (2022). Statistical Communiqué of the People’s Republic of China on the 2021 National Economic and social development. Available at: https://www.gov.cn/xinwen/2022-02/28/content_5676015.htm (Accessed July 2, 2023)
Neeraj, D., and Jamwal, M. (2022). Tourists’ post-adoption continuance intentions of chatbots: integrating task–technology fit model and expectation–confirmation theory. Foresight 25, 209–224. doi: 10.1108/FS-10-2021-0207
Nikolopoulou, K., Gialamas, V., and Lavidas, K. (2020). Acceptance of mobile phone by university students for their studies: an investigation applying UTAUT2 model. Educ. Inf. Technol. 25, 4139–4155. doi: 10.1007/s10639-020-10157-9
Pal, D., Funilkul, S., and Vanijja, V. (2020). The future of smartwatches: assessing the end-users’ continuous usage using an extended expectation-confirmation model. Univ. Access Inf. Soc. 19, 261–281. doi: 10.1007/s10209-018-0639
Qadir, J. (2023). Engineering education in the era of ChatGPT: promise and pitfalls of generative AI for education. IEEE Global Engineering Education Conference.
Qiang, Y., and Hu, Q. (2023). Adoption of new technologies under the myth of the electronic revolution and identity identification: a user study of ChatGPT based on grounded theory. News Univ. 4, 59–74. doi: 10.20050/j.cnki.xwdx.2023.04.008
Rahman, M. M., and Watanobe, Y. (2023). ChatGPT for education and research: opportunities, threats, and strategies. Appl. Sci. 13, 57–83. doi: 10.3390/app13095783
Raza, S. A., Qazi, Z., Qazi, W., and Ahmed, M. (2022). E-learning in higher education during COVID-19: evidence from black-board learning system. J. Appl. Res. High. Educ. 14, 1603–1622. doi: 10.1108/JARHE-02-2021-0054
Samantha, M. K. (2023). ChatGPT passes exams from law and business schools. CNN. Available at: https://edition.cnn.com/2023/01/26/tech/chatgpt-passes-exams/index.html
Samsudeen, S. N., and Mohamed, R. (2019). University students’ intention to use e-learning systems. Interact. Technol. Smart Educ. 16, 219–238. doi: 10.1108/ITSE-11-2018-0092
Shawar, B. A. (2017). Integrating CALL systems with chatbots as conversational partners. Comput. Sist. 21, 615–626. doi: 10.13053/cys-21-4-2868
Sitar-Taut, D.-A., and Mican, D. (2021). Mobile learning acceptance and use in higher education during social distancing circumstances: an expansion and customization of UTAUT2. Online Inf. Rev. 45, 1000–1019. doi: 10.1108/OIR-01-2021-0017
Stone, M. (1976). Cross-validatory choice and assessment of statistical predictions (with discussion). J. R. Stat. Soc., Ser. B Methodol. 38:102.
Šumak, B., Hericko, M., Polančič, G., and Pušnik, M. (2010). Investigation of E-learning system acceptance using UTAUT. Int. J. Eng. Educ. 26, 1327–1342.
Su, Y., Lin, Y., and Lai, C. (2023). Collaborating with ChatGPT in argumentative writing classrooms. Assess. Writ. 57:100752. doi: 10.1016/j.asw.2023.100752
Taecharungroj, V. (2023). “What can ChatGPT do?” analyzing early reactions to the innovative AI Chatbot on twitter. Big Data Cogn. Comp. 7:35. doi: 10.3390/bdcc7010035
Tam, C., Santos, D., and Oliveira, T. (2020). Exploring the influential factors of continuance intention to use mobile apps: extending the expectation confirmation model. Inf. Syst. Front. 22, 1–15. doi: 10.1007/s10796-018-9864-5
Tan, Z. (2023). Legal risks and governance pathways of ChatGPT. J. Hunan Univ. Sci. Technol. (Soc. Sci. Edition) 26, 117–125.
Tenenhaus, M., Silvano, A., and Vinzi Vincenzo, E. (2004). A global goodness-of-fit index for PLS structural equation modelling. In Proceedings of the XLII SIS Scientific Meeting, 739–742.
Thunström, A. O. (2022). We asked GPT-3 to write an academic paper about itself: then we tried to get it published. Scientific American.
Tlili, A., Shehata, B., Adarkwah, M. A., Bozkurt, A., Hickey, D. T., Huang, R., et al. (2023). What if the devil is my guardian angel: ChatGPT as a case study of using chatbots in education. Smart Learn. Environ. 10:15. doi: 10.1186/s40561-023-00237-x
Twum, K. K., Ofori, D., Keney, G., and Korang-Yeboah, B. (2022). Using the UTAUT, personal innovativeness and perceived financial cost to examine student’s intention to use E-learning. J. Sci. Technol. Policy Manag. 13, 713–737. doi: 10.1108/JSTPM-12-2020-0168
van Dis, E. A. M., Bollen, J., Zuidema, W., van Rooij, R., and Bockting, C. L. (2023). ChatGPT: five priorities for research. Nature 614, 224–226. doi: 10.1038/d41586-023-00288-7
Venkatesh, T., and Xu, X. (2012). Consumer acceptance and use of information technology: extending the unified theory of acceptance and use of technology. MIS Q. 36:157. doi: 10.2307/41410412
Venkatesh, V., Morris, M. G., Davis, G. B., and Davis, F. D. (2003). User acceptance of information technology: toward a unified view. MIS Q. 27:425. doi: 10.2307/30036540
Wan, L., and Zhao, C. (2016). Research on factors influencing the adoption and use of information technology by primary and secondary school teachers in ethnic regions based on the UTAUT model. Mod. Distance Educ. 2, 70–75. doi: 10.13927/j.cnki.yuan.2016.0021
Wang, Q., and Mao, H. (2016). Analysis of factors influencing MOOC learning behavior based on the UTAUT model. Res. Digit. Educ. 6, 43–48. doi: 10.13811/j.cnki.eer.2016.06.006
Wang, T., Lund, B. D., Marengo, A., Pagano, A., Mannuru, N. R., Teel, Z. A., et al. (2023). Exploring the potential impact of artificial intelligence (AI) on international students in higher education: generative AI, Chatbots, analytics, and international student success. Appl. Sci. 13:6716. doi: 10.3390/app13116716
Wang, Y. S., Wu, M. C., and Wang, H. Y. (2009). Investigating the determinants and age and gender differences in the acceptance of mobile learning. Br. J. Educ. Technol. 40, 92–118. doi: 10.1111/j.1467-8535.2007.00809.x
Wang, Z., and Cao, S. (2020). Factors influencing relevance judgment in video retrieval: an empirical study based on PLS-path analysis. J. Inf. Sci. 39, 926–937. doi: 10.3772/j.issn.1000-0135.2020.09.005
Wu, R., and Yu, Z. G. (2023). Do AI chatbots improve students learning outcomes? Evidence from a meta-analysis. Br. J. Educ. Technol. 1–24. doi: 10.1111/bjet.13334
Wu, W., Zhang, B., Li, S., and Liu, H. (2022). Exploring factors of the willingness to accept AI-assisted learning environments: an empirical investigation based on the UTAUT model and perceived risk theory. Front. Psychol. 13:870777. doi: 10.3389/fpsyg.2022.870777
Xiong, M. (2015). Research on factors influencing acceptance of Mobile learning among college students. Suzhou University.
Xu, Y., Shieh, C. H., Esch, P. V., and Ling, I. L. (2020). AI customer service: task complexity, problem-solving ability, and usage intention. Australas. Mark. J. 28, 189–199. doi: 10.1016/j.ausmj.2020.03.005
Yang, G. F. (2016). Factors influencing the sustained usage behavior of MOOC users. Open. Educ. Res. 1, 100–111.
Yang, W., Zhang, J., Liu, M., and You, H. (2015). An empirical study on continued usage behavior of digital educational resources: based on an extended ECM-ISC model. Chinese Electrified Educ. 11, 54–61. doi: 10.3969/j.issn.1006-9860.2015.11.008
Zeebaree, M., Agoyi, M., and Aqel, M. (2022). Sustainable adoption of E-government from the UTAUT perspective. Sustain. For. 14:5370. doi: 10.3390/su14095370
Zhai, S., Chen, H., and Wang, Z. (2022). Research on factors influencing user intention to use ChatGPT. Inf. Theory Pract. 2, 28–35. doi: 10.13833/j.issn.1007-7634.2022.02.004
Zhang, A., and Jia, L. (2023). Algorithmic "comfort zone" and its breakthrough: a discussion on the algorithmic content of ChatGPT. Party Government Stud. 3, 22–33. doi: 10.13903/j.cnki.cn51-1575/d.20230220.001
Zhang, H., Liu, C., Wang, D., and Zhao, Z. (2023). Research on factors influencing user intention to use ChatGPT. Inf. Theory Pract. 4, 15–22. doi: 10.16353/j.cnki.1000-7490.2023.04.003
Zhang, S., Liu, Q., Huang, J., and Wu, P. (2016). Research on factors influencing the use of online learning spaces by primary and secondary school teachers: a survey based on the UTAUT model. Chinese Digit. Educ. 3, 99–106. doi: 10.3969/j.issn.1006-9860.2016.03.017
Zhang, W., and Bai, L. (2017). Research on continued usage behavior of crowdsourcing community users: based on ECM-ISC and commitment-trust theory. Inf. Resourc. Manag. 2, 54–62. doi: 10.3969/j.issn.1002-0314.2017.02.008
Zhu, K. (2017). Research on the effects of interactivity and immersion in online learning spaces on students' continuance intention. Chinese Digit. Educ. 2, 89–95. doi: 10.3969/j.issn.1006-9860.2017.02.014
Zhu, C., Sun, M., Luo, J., and Li, T. (2023). How to harness the potential of ChatGPT in education?[J]. Knowl. Manag. E-Learn. 15:133. doi: 10.34105/j.kmel.2023.15.008
Keywords: AI Chatbot, Chinese graduate students, technology acceptance, UTAUT, ECM
Citation: Tian W, Ge J, Zhao Y and Zheng X (2024) AI Chatbots in Chinese higher education: adoption, perception, and influence among graduate students—an integrated analysis utilizing UTAUT and ECM models. Front. Psychol. 15:1268549. doi: 10.3389/fpsyg.2024.1268549
Received: 28 July 2023; Accepted: 22 January 2024;
Published: 07 February 2024.
Edited by:
Petra Poulova, University of Hradec Králové, CzechiaReviewed by:
Dag Øivind Madsen, University of South-Eastern Norway (USN), NorwayCopyright © 2024 Tian, Ge, Zhao and Zheng. This is an open-access article distributed under the terms of the Creative Commons Attribution License (CC BY). The use, distribution or reproduction in other forums is permitted, provided the original author(s) and the copyright owner(s) are credited and that the original publication in this journal is cited, in accordance with accepted academic practice. No use, distribution or reproduction is permitted which does not comply with these terms.
*Correspondence: Jingshen Ge, Z2VqaW5nc2hlbkBvdWMuZWR1LmNu
Disclaimer: All claims expressed in this article are solely those of the authors and do not necessarily represent those of their affiliated organizations, or those of the publisher, the editors and the reviewers. Any product that may be evaluated in this article or claim that may be made by its manufacturer is not guaranteed or endorsed by the publisher.
Research integrity at Frontiers
Learn more about the work of our research integrity team to safeguard the quality of each article we publish.