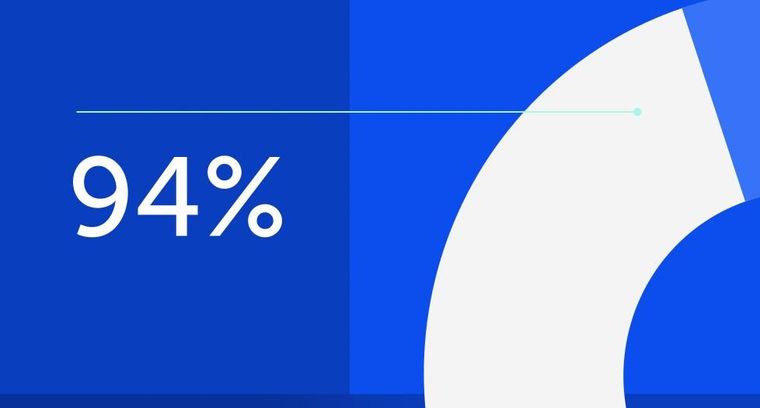
94% of researchers rate our articles as excellent or good
Learn more about the work of our research integrity team to safeguard the quality of each article we publish.
Find out more
REVIEW article
Front. Psychol., 27 April 2023
Sec. Emotion Science
Volume 14 - 2023 | https://doi.org/10.3389/fpsyg.2023.1158136
This article is part of the Research TopicReviews In Perception ScienceView all 4 articles
While approaching celebrations for the 150 years of “The Expression of the Emotions in Man and Animals”, scientists’ conclusions on emotion expression are still debated. Emotion expression has been traditionally anchored to prototypical and mutually exclusive facial expressions (e.g., anger, disgust, fear, happiness, sadness, and surprise). However, people express emotions in nuanced patterns and – crucially – not everything is in the face. In recent decades considerable work has critiqued this classical view, calling for a more fluid and flexible approach that considers how humans dynamically perform genuine expressions with their bodies in context. A growing body of evidence suggests that each emotional display is a complex, multi-component, motoric event. The human face is never static, but continuously acts and reacts to internal and environmental stimuli, with the coordinated action of muscles throughout the body. Moreover, two anatomically and functionally different neural pathways sub-serve voluntary and involuntary expressions. An interesting implication is that we have distinct and independent pathways for genuine and posed facial expressions, and different combinations may occur across the vertical facial axis. Investigating the time course of these facial blends, which can be controlled consciously only in part, is recently providing a useful operational test for comparing the different predictions of various models on the lateralization of emotions. This concise review will identify shortcomings and new challenges regarding the study of emotion expressions at face, body, and contextual levels, eventually resulting in a theoretical and methodological shift in the study of emotions. We contend that the most feasible solution to address the complex world of emotion expression is defining a completely new and more complete approach to emotional investigation. This approach can potentially lead us to the roots of emotional display, and to the individual mechanisms underlying their expression (i.e., individual emotional signatures).
“…Facial movement of expression impresses us through its changes, through its melody.
The characteristic of the person will always be the way they move, the melody of the expression; this can never be caught in snapshots…”
(Sir Ernst Gombrich, cited by Miller, 1983)
According to a growing crowd of influential researchers, we are now on the edge of a radical change of perspective about how we think of emotions (Adolphs et al., 2019; Barrett et al., 2019; Heaven, 2020). Emotion is one of the building blocks of human life. However, how humans dynamically and genuinely express their emotions with the whole body is still little investigated.
Over the last century, the science of emotion gradually anchored to six prototypical facial expressions: anger, disgust, fear, happiness, sadness, and surprise (i.e., the basic six; Ekman et al., 1969; Ekman and Friesen, 1971). These six expressions were described as unitary entities and conceived as if they were mutually exclusive categories. In particular, Basic emotion theory proposes that a limited number of emotions are manifested through organized and recurrent patterns of behavior in a kind of “one-to-one” correspondence, conserved by evolution to handle basic life situations (Ekman, 1992a,b, 2003; LeDoux, 1995, 2012; Ekman and Cordaro, 2011; Damasio and Carvalho, 2013). Jack et al. (2016) further narrowed this view by suggesting a four-scheme model of expression, each of which communicates a specific combination of valence, arousal and dominance, probably evolved from a simpler communication system (see also the “Three Primary Color Model of Basic Emotions,” Gu et al., 2019). Needless to say, all these elegant models leave most human expressions unexplored (Barrett, 2017; Adolphs et al., 2019; Barrett et al., 2019). Moreover, the term basic seems to underlie that the emotions are discrete, rather than a family of related states (Scherer and Ellgring, 2007; Tracy and Matsumoto, 2008; Roseman, 2011; Bänziger et al., 2012; Keltner and Cordaro, 2017; Cordaro et al., 2018). Instead of considering happiness as a single emotion, for instance, research should try to unpack emotional categories into their components: the happiness umbrella might cover joy, pleasure, compassion, pride, and so on. According to the Constructionist theory, a wide range of emotions have evolved, shaped by language and cognitive appraisal (Russell and Barrett, 1999). All the emotions can be located in a circle called circumplex (Russell, 1980), characterized by different amounts of valence (pleasure/displeasure axis) and arousal (high/low axis). Basic emotion and Constructionist theories have been pitted against each other for more than a century in the so-called 100-year war (Lindquist et al., 2013; for a review see Crivelli and Fridlund, 2019). Now, thanks to modern neuroscience, we are finally beginning to understand the complexity of the emotional world. Emotional expression might be far richer and more complex than the prototypical patterns of facial muscle movements so far considered.
The article is not intended to be a comprehensive review on emotions, but rather a focused review on the expression of emotions by presenting critical research suggesting that humans dynamically perform genuine and mixed expressions with face and body. We also propose a new integrated model capable of extracting multimodal algorithms applicable in ecological contexts for the assessment of inter-individual and cultural differences.
Humans have 43 facial muscles, with which they can produce up to 10,000 different expressions, making the human face one of the most powerful communicative tools our species has (Rinn, 1984). Note that even the expression of the same emotion may be conveyed by different neural systems. For instance, two anatomically and functionally different neural pathways sub-serve the expressions of genuine and posed facial expressions. The contraction of mimic muscles related to genuine emotion originates from subcortical brain areas that provide excitatory stimuli to the facial nerve nucleus in the brainstem via extrapyramidal motor tracts, which often involve the concomitant contraction of the ocular orbicular muscles. In contrast, posed smiles are controlled by impulses of the pyramidal tracts from the motor cortex (Frank et al., 1993; Schmidt et al., 2006; Sidequersky et al., 2014). Therefore, we have different pathways for posed (i.e., voluntarily controlled) and spontaneous (i.e., involuntarily produced) facial displays (Ross et al., 2016), so that the genuine pathway has been associated with more synchronized, smooth, and symmetrical expressions compared to the pyramidal voluntary system (Ross et al., 2019). Posed facial expressions are those displayed intentionally by a person who pretends to transmit a specific emotion (Namba et al., 2017), while spontaneous facial expressions are those elicited by true emotional content and usually correspond to a more genuine emotional experience (Niedenthal et al., 2010; Zloteanu and Krumhuber, 2021). For example, a smile is genuine when listening to a joke. However, people also try to smile when they feel angry, scared, tired or embarrassed, to hide these emotions in contexts where they are inappropriate. Notably, the upper face muscles (i.e., eye areas) are mainly controlled by the subcortical and extrapyramidal systems, whereas the lower face (i.e., mouth area) is under the voluntary control of the motor system (Gazzaniga and Smylie, 1990; Hopf et al., 1992; Ross et al., 2007; Krippl et al., 2015). This means that: (i) facial blends of expressions might occur across the horizontal axis (i.e., eyes vs. mouth areas, Ross et al., 2016); and (ii) muscles of the upper face are innervated bilaterally, whereas muscles of the lower face are cross-innervated prevalently from the contralateral side (Morecraft et al., 2004; Ross et al., 2016). Therefore, small changes in the dynamical development of a facial display may characterize and distinguish genuine from posed facial expressions, a topic still poorly investigated (but see Sowden et al., 2021). Investigating the relative contributes of upper and lower facial cues to emotion recognition is also particularly interesting in the light of the current COVID-19 pandemic. By 2020, medical facemasks occluding the lower portion of the face have become a pervasive feature in everyday life. These masks are clearly designed for preventing infection. However, there are concerns related to their possible impact on emotion recognition. Results from a just-published study (Marini et al., 2021) show that mask-wearing has two problematic side effects. First, by making the mouth invisible, they interfere with the recognition of emotional states. Moreover, they compromise facial mimicry reducing therefore emotional contagion (Dimberg et al., 2011; Hess and Fischer, 2013; Tramacere and Ferrari, 2016; Palagi et al., 2020).
When a genuine emotion is experienced, the expression of this emotion cannot be totally inhibited or modified, and it follows a rather stereotyped pattern (Baker et al., 2016). For example, a genuine smile – like automatic movements - can appear as fast as 0.30 s, and it usually fades away after 3 to 4 s (Schmidt et al., 2003). However, the diversity of appearance and dynamics of spontaneous smiles still requires a better understanding of a smile’s properties and patterns, to determine what features or temporal parameters are key in transmitting information and how they variate in different contexts (Schmidt et al., 2003). What is needed to make sense of emotional expressions is therefore a much richer taxonomy.
Experimental studies of emotional expressions, inaugurated by Ekman (1965) and continued by a large number of scholars, have focused on static and prototypical facial expressions with the goal of finding evidence for a universal theory of emotional expressions (with the help, for example, of facial expression analysis or FACS; Ekman et al., 2002). Past research, moreover, has favored the use of stereotyped emotional stimuli over more ecologically valid but less controllable expressions in order to better investigate the influence of variables such as gender, age, and personality traits. It was possible to show, for example, that observed emotion recognition performance is higher in females (e.g., Hoffmann et al., 2010; Saylik et al., 2018; Wingenbach et al., 2018; Connolly et al., 2019), decreases with age (e.g., Ruffman et al., 2008; West et al., 2012; Abbruzzese et al., 2019), and is particularly affected in people with alexithymia (e.g., Vermeulen et al., 2006; Scarpazza et al., 2014; Starita et al., 2018; Malykhin et al., 2023). The use of static, posed, and archetypical facial expressions has provided in fact high scientific control and repeatability, but at the cost of data variability that likely accounts for actual emotional manifestations (Kret and De Gelder, 2012). Past researchers have tried to balance these research priorities and have correctly identified the optimal ways of expressing different emotions (e.g., Ekman and Friesen, 1976; Wallbott and Scherer, 1986; Motley and Camden, 1988; Russell, 1994; Biehl et al., 1997; Tcherkassof et al., 2013). Now we can try to get on their shoulders and break down the question further, to investigate the distinction between authentic and posed emotions. It is time to increase complexity. We certainly do not deny the fact that using genuine and ecologically valid emotional stimuli will still have some problems too. For instance, showing combinations of different emotions might add complexity to the task of identifying emotional expressions. Indeed, as the prototypical quality of the stimuli lessens, the influence of perceiver-based processes (e.g., her/his current emotional state) becomes more prominent in the perceptual judgement process. We might ask: At what point does the benefit of using ecologically valid stimuli balance out the inverse increase in the influence of perceptual-based factors? We are aware that emotion science is now facing a classic trade-off between external validity of stimuli and their recognition properties. We believe, however, that if the science of emotions were to remain still anchored in prototypical displays, it would not rise to the level of understanding the recognition processes evolved in response to real stimuli during the phylogenetic development of the human species. Having a comprehensive taxonomy of real emotion expression will help to formulate new theories with a greater degree of complexity. Recently, some dataset including spontaneous emotions “in the wild” have been released (Guerdelli et al., 2022). However, the majority of emotional facial data sets of stimuli used in scientific research are based on static photographs of non-spontaneous facial expressions (Tcherkassof et al., 2013; O’Reilly et al., 2016; Dawel et al., 2022). This methodology has been questioned given the low generalizability of its results (Russell, 1994; Tcherkassof et al., 2013). People project their stereotypes in posed expressions, their common view of what they believe an emotional facial expression should look like (e.g., a scowling facial configuration to express anger), but these displays do not necessarily correspond to how people actually behave in real life (Barrett et al., 2019).
Genuine expressions differ specifically from posed expressions in both temporal and morphological features (Wehrle et al., 2000; Cohn and Schmidt, 2004; Sato and Yoshikawa, 2004; Ekman and Rosenberg, 2005; Yoshikawa and Sato, 2006; Valstar and Pantic, 2010). In first instance, genuine facial expressions can occur within a fraction of a second (i.e., micro expressions; Ekman, 2009). In second instance, they are usually less intense and more subtle than posed expressions classically used in laboratory (Tcherkassof et al., 2013). This disparity could explain why the recognition accuracy of posed emotions, characterized by prototypical and very intense facial configurations, is much higher than that of spontaneous emotions (Barrett et al., 2019). Thus, more genuine stimuli are needed in research. Unfortunately, such databases are still rare because of the practical (e.g., the methodology needed to collect these stimuli) and ethical difficulties (see Philippot, 1993 for initial considerations) of documenting and collecting genuine expressions (Tcherkassof et al., 2013). In fact, it is difficult to trigger authentic emotions with the same intensity as fake emotions and to validate the resulting dataset of posed and spontaneous displays (Krumhuber et al., 2017). For example, some datasets built on the performance of professional actors did not verify whether the expressions were then perceived as genuine by observers (McLellan et al., 2010). And even when it was verified, it missed the next step, which was to cross-reference the observers’ scoring with the emotion actually experienced by the actor (Dawel et al., 2017). Only recently, a dataset of authentic and inauthentic emotional expressions matched the emotion felt by the actor with that perceived by the observer in terms of intensity and genuineness (Miolla et al., 2022). The next step will be to create dataset including also the context of the emotional display. In fact, posed expressions often occur in everyday life (e.g., when mothers exaggerate their facial movements to be perceived accurately by their infant children) and they are nonetheless genuine and appropriate to the context.
A related problem in the study of emotions expressions is that the majority of the literature have employed static facial stimuli (Tcherkassof et al., 2007; McLellan et al., 2010, 2012; Douglas et al., 2012; Li et al., 2012; Dawel et al., 2015). Only the peak intensity of emotions was usually shown, while the time-course of facial expressions was substantially ignored. However, facial expressions are not an all-or-nothing phenomenon: the nature of facial expressions is that they are dynamic in presentation (Rymarczyk et al., 2019). Recent literature suggests that dynamic displays enhance the ability not only to correctly recognize facial expressions (Cunningham and Wallraven, 2009; Ceccarini and Caudek, 2013; Krumhuber et al., 2013), but also to discriminate genuine and posed facial expressions of emotion (Krumhuber et al., 2013; Namba et al., 2018, 2021; Lander and Butcher, 2020) and to elicit stronger muscle activation during mimicry (Rymarczyk et al., 2016). The use of dynamic emotional stimuli is more ecologically valid (Bernstein and Yovel, 2015), as an emotional message is usually reflected in dynamic complex action patterns and not in static facial clues (Tcherkassof et al., 2013; O’Reilly et al., 2016). This is probably because dynamic faces can transmit an evolving hierarchy of signals over time (Delis et al., 2016), thus providing much more information than static pictures (e.g., time course, change of speed, facial-feature amplitude, and irregularity of an expression; Tcherkassof et al., 2013). This effect has also been confirmed by the activation of a broader neural network in the observer when using dynamic stimuli compared to static emotion stimuli (Ambadar et al., 2005; Weyers et al., 2006; Trautmann et al., 2009). Only recently, an increasing number of dynamic emotion data sets have been developed, including, for instance, the Cohn–Kanade AU-Coded Facial Expression Database (Kanade et al., 2000; Lucey et al., 2010) and the Video Database of Moving Faces & People (O’Toole et al., 2005; for a review see Krumhuber et al., 2017). However, an aspect that has been largely neglected is the key role of temporal dynamics as a locus for investigating the encoding of facial displays. To date, little is known about the temporal course of facial expressions (Tcherkassof et al., 2013). Temporal parameters, such as the apex period (i.e., the time duration before the peak intensity starts decreasing) and movement time (i.e., the time from facial display onset until it disappears) of facial expressions, might allow unveiling the secret syntax of emotional language. For instance, recent research has shown that eyelid movements precede eyebrow movements in genuine surprise displays (Namba et al., 2017) and this could help to differentiate spontaneous from simulated expressions. In the case of smiles, shorter durations and more irregular onset have been associated with lower perceived genuineness (Krumhuber et al., 2013).
To sum up, research on emotion expression has been extensively conducted during passive observation of posed and static pictures (e.g., Karolinska Directed Emotional Faces; Lundqvist et al., 1998). More ecological and dynamic stimuli such as spontaneous recordings from real-time interactions have rarely been adopted. Crucially, posed expressions have lower ecological validity and differ in timing from spontaneous ones (Ekman and Rosenberg, 2005). Approaches based on static and simulated portrayals may, therefore, fail to generalize to real-world behavior (Zeng et al., 2009). Even distinguishing facial expressions into genuine or posed, depending on the manner and context in which they are produced, may be too simplistic, because they are just the poles of a broad spectrum with various gradations of color.
Many — even most — experiences of emotion are complex blends of emotion (Parr et al., 2005; Du et al., 2014). Multiple emotions can occur in a rapid sequence, again and again, or can merge in a mosaic. Humans have the capacity to produce facial blends of emotions in which the upper and lower face simultaneously display different expressions, suggesting that their underlying emotions are compound entities (Larsen et al., 2001; Scherer, 2009). Facial expressions are organized predominantly across the horizontal facial axis (i.e., upper-lower areas), but there are exemplars (e.g., surprise-frown or smile-grimace) in which the expression on the right and left sides of the face differs, thus providing evidence that facial blends of emotions may also occur across the vertical facial axis (i.e., left–right areas). In this vein, three major models of emotional processing address the so-called “hemispheric lateralization of emotions” topic in humans (Demaree et al., 2005; Killgore and Yurgelun-Todd, 2007). The Right Hemisphere Hypothesis asserts that all emotions and their associated expressions are a dominant and lateralized function of the right hemisphere. The Valence Hypothesis states that negative, avoidance or withdrawal-type emotions and their associated expressions are lateralized to the right hemisphere, whereas positive approach-type emotions and their associated expressions are lateralized to the left hemisphere. Finally, the Emotion-type Hypothesis (Ross et al., 2007, 2016) affirms that primary emotional responses are initiated by the right hemisphere on the left side of the face, whereas social emotional responses are initiated by the left hemisphere on the right side of the face. The most striking examples are expressions that display a “double peak” phenomenon (e.g., grimace-smile characterized by an initial movement followed by a slight relaxation and then a second movement to the final peak) as a result of dual or competing hemispheric motor control (Ross et al., 2016). In some instances, the initial movement starts on one side of the face and the second movement starts on the opposite side of the face. For instance, Duchenne and non-Duchenne are terms used to classify if a smile reflects a true emotional feeling versus a false smile (Ekman and Friesen, 1982; Ekman et al., 1988). A felt (Duchenne) smile is very expressive and it is classically described as causing the cheeks to lift, the eyes to narrow and wrinkling of the skin to produce crow’s feet. A false (non-Duchenne) smile, instead, would only involve the lower face area. However, recent research has shown that the difference between a felt (Duchenne) versus a fake smile might in fact be revealed by the side of the face initiating the smile (Ross et al., 2016).
Despite the importance of emotion in human functioning, scientists have been unable to reach a consensus on the debated issue concerning the lateralization of emotions. We believe that investigating the time course of facial blends of emotions, which can be controlled consciously only in part, would provide a useful operational test for comparing the different predictions of various models, thus allowing this long-standing conundrum to be solved.
Emotions can be described as responses to events that are important to an individual (Izard, 2010; Mulligan and Scherer, 2012; Ekman, 2016; for an overview see Scherer, 2009). They are usually expressed with the aim to be recognized by the addressee and might be expressed differently depending on who is the interlocutor. In this light, facial expressions are regarded as affective signals, which can convey social information regarding the expresser’s experience of an emotional event (Ekman, 2004; Scherer and Moors, 2019).
One of the hallmarks of social psychology is that in real life situations, body kinematics, gaze-related information, and contextual cues are all critical cues in guiding motor behavior (Sartori et al., 2011; Reader and Holmes, 2016). In the emotional world, the facial display is necessary but may be not sufficient to express and interpret correctly other’s emotions (Barrett et al., 2011). Humans do not interact with ‘bodyless’ or ‘contextless’ faces, as occurs in most of the current research: they constantly receive and integrate multimodal information. Needless to say, a facial expression could be misinterpreted when analyzed independently from the context in which is presented: for instance, tears of victory mean happiness.
Future studies should consider to adopt real-time naturalistic settings: for instance, involving participants in a dyadic interaction (i.e., authentic emotion induction; Zhang et al., 2014), while recording both their movements. Only the adoption of an ecological behavioral approach will allow to genuinely evaluate the effect of social context on emotional functioning. Participants’ spontaneous expressions should be video recorded at high frame rates using specialized recording equipment to provide a good resolution database, allowing the investigation of micro-expressions and subtle temporal features to be matched with self-reported feelings. Introspective measures constitute in fact an essential validation approach, as they provide insight into the elicitation effectiveness (Gray and Watson, 2007). Notably, the study of the neural underpinning of real-time contagious phenomena (e.g., the social transfer of pain) is now extremely relevant, as recently confirmed by Smith and colleagues (Smith et al., 2021). In this perspective, the existing literature has few or null ecologic validity. According to the influential article by Heaven (2020), researchers need to do what Darwin (1859) did for The Origin of Species: “Observe, observe, observe.” Watch what people actually do with their faces and their bodies in real-life contexts. More data and analytical techniques could help researchers to learn something new, instead of revisiting old data sets and experiments (Heaven, 2020).
When we are in the grip of an emotion, a cascade of changes occurs in the face, gaze, autonomic nervous system activity, and in our expressive body behavior (for review, see Keltner et al., 2016, 2019). In 2019, Barrett and colleagues published a benchmark review on emotion expression (Barrett et al., 2019). They considered over 1,000 papers and they reached an unambiguous conclusion: the face is not the whole picture. Other aspects, including body movement, gaze, and physiological changes (e.g., cardiovascular changes) are crucial in our expression and perception of emotions. Therefore, a pressing need in the study of emotional expression appears to be necessary to move beyond the narrow focus on facial displays.
In the real world, bodies and faces are almost never perceived in isolation, but rather as an integrated whole. Bodies contain valuable information about the actions and intentions of others, which often intensifies or conversely cancels out the emotion expressed by the face (de Gelder, 2009; Aviezer et al., 2012; de Gelder et al., 2015). When emotion facial expressions are paired with incongruent bodily expressions (e.g., anger facial expression with a fearful body pose), for instance, perceivers show distinct neural responses and impaired recognition, even when they are consciously focusing on the face alone (Meeren et al., 2005; Borgomaneri et al., 2020). These findings suggest that it might be fruitful to focus more attention on the body when considering emotion expression. For example, full-body expressions of fear communicate important information in an immediate, arousing, and contagious manner (de Gelder et al., 2004; Borgomaneri et al., 2015). Anger is commonly expressed with hands in fists, disgust with head tilted slightly forward, fear with hands raised to protect the body, sadness with shoulders slumped, surprise with arms raised, shame with downward head tilt (Izard, 1971; Keltner, 1995), while pride includes headed slightly tilted back and hands on hips (Tracy and Robins, 2007). Notably, the expression of emotions such as embarrassment, pride, and shame can only be recognized when body movements are combined with facial expressions. Body postures do, in fact, influence both the expression and the recognition of emotions (Shan et al., 2007; Dael et al., 2012). This issue becomes relevant when considering emotions such as guilt and love, which lack distinctive facial signals: they may display recognizable nonverbal expressions if body position is considered.
To sum up, emotional experience does not manifest itself in facial configurations alone, but rather in multimodal expressions involving head movements, gaze, and the body. The close connection and continuity of facial expressions with postural and gestural cues, however, has historically remained in the background (Lott et al., 2022). In recent decades, interest in the study of emotional body expressions has steadily increased (Lenzoni et al., 2020; Poyo Solanas et al., 2020; Watson and de Gelder, 2020), leading to the development of data sets on the emotional body with dynamic stimuli (e.g., Troje, 2002; Atkinson et al., 2004; Alaerts et al., 2011; de Gelder and Van den Stock, 2011). However, studies presenting facial and body expressions together (e.g., Rosenthal et al., 1979; Thoma et al., 2013) or investigating how body movements can express spontaneous and posed displays are still scarce. To obtain a more complete evaluation of emotional functionality, the synergistic actions of many different facial and body muscles, as well as gaze, physiological correlates and self-reports should be investigated with a triangulation approach. Triangulation is a strategy adopted by cartographers to map a new territory: three known points are defined and based on those, the unknown point is identified. In this case, the integration of reliable data from three different sources (i.e., physiological, psychological and behavioral) will allow answering questions such as: What are the neurophysiological processes that underlie the expression of emotion? Which physical features are globally encoded? What would a comprehensive atlas of human emotions’ expression include?
In a meta-analysis of physiological responses associated with a wide range of distinct emotions, several positive emotions (e.g., amusement, awe, contentment, desire, enthusiasm — all of which would be grouped under “happiness” by the classical basic six approach) were found to have subtly distinct patterns of peripheral physiological response linked to unique biological substrates (Kreibig, 2010). In this light, psychologists have uncovered that positive and negative valence information can increase pupil dilation (Bradley et al., 2008), making this measure a suitable proxy for understanding emotional load (Sirois and Brisson, 2014) in conjunction with high-frequency heart rate variability (HF-HRV), a biomarker of vagal-mediated parasympathetic activity able to detect states of distress (Dell’Acqua et al., 2020; da Estrela et al., 2021). We argue that only the combined recording of different and complementary techniques will provide a comprehensive emotional taxonomy.
Past research investigating emotional displays has mainly focused on the facial muscle activation occurring during an emotional event using manual coding approaches, such as the Facial Action Coding System (FACS; Ekman and Friesen, 1978; Ekman et al., 2002). Classically, two FACS coders decompose an observed expression into specific Action Units (AUs; i.e., contraction or relaxation of distinct facial muscles) that produce the movement, and their outcomes are eventually compared. Although this is the most widely used method to categorize emotion expressions, its primary drawback is that it analyzes each facial movement independently from other movements. Being many facial muscles closely related, they cannot move independently (Hao et al., 2018). Moreover, FACS codes have a fixed range of applications: for instance, they do not incorporate AUs for emotions like pride, which has a complex expression that involves the body as well as the face. Another weakness of this system is that being a human coder requires an extensive training and it is very time-consuming. A trained FACS operator can take hours to code 1 min of video data depending on the complexity and density of facial expressions.
To solve this issue, researchers created automatized algorithms (Chu et al., 2017; Martinez, 2017; Park et al., 2020), which work very well in the laboratory, when images can be controlled. However, their accuracy drops substantially when they detect less constricted facial expressions (Benitez-Quiroz et al., 2017). Moreover, both manual FACS coding by expert raters (van der Schalk et al., 2011) and automatic detection by means of computational algorithms (Lucey et al., 2010; Valstar et al., 2017) have been applied to dynamic expression databases only on the apex. The estimation at multiple time steps is vital because, in real life, expressions vary in intensity over time.
In this respect, in the last decade, Machine Learning (ML) has been applied to both static and dynamic emotional stimuli to investigate the possibility to automatically discriminate emotions basing on facial expression configuration. ML is one of the most promising fields in the area of artificial intelligence (Mitchell, 1997): it is a discipline associated with computational statistics that aims to create new knowledge or predictions through algorithms that - based on real observations, categorizes items into different categories. In particular, ML algorithms are trained on a portion of data (training set). Then once trained, they are tested on the remaining data (test set). Besides being applied in emotional discrimination, ML algorithms have also been recently applied to discriminate genuine from posed emotional expressions. The first example regarded pain (Bartlett et al., 2014): the authors showed that, while human observers could not discriminate spontaneous from posed expression of pain better than chance (55% of accuracy), ML algorithms that automatically detected facial movements were able to achieve 85% of accuracy. Similarly, in another study (Monaro et al., 2022), authors recorded participants’ face while they recalled a real or posed emotional event. Again, the ability of ML algorithms to discriminate the true from the false story based on facial movements was much higher than the one of human beings (78 vs. 57%). These pioneering studies suggest that, when relying on facial cues only, artificial intelligence performs better than humans in discriminating genuine from posed emotions even if humans have more information to rely upon.
Despite ML algorithms are considered a powerful tool, their use is not free from criticisms. Nowadays, a widespread criticism to Machine Learning (ML) algorithms is that they provide un-interpretable results (i.e., a percentage of classification accuracy without explaining the classification rules; see Carvalho et al., 2019). Recently, a paper tried to overcome this shortcoming by using interpretable ML models able to detect and describe differences between genuine and non-genuine emotional expressions (Cardaioli et al., 2022). Interpretable ML models are algorithms that, besides providing the scientists with a classification accuracy, also identify facial movements that mostly contribute to the classification of genuine and posed emotions. In Figure 1, for instance, are reported the results of decision tree ML algorithms with a mean of 82% of accuracy in discriminating genuine and posed emotions. Moreover, decision tree, identifies, for each specific emotions, the AUs critical for the classification of genuine and posed, thus providing important insights for the neuroscientific understanding of emotions.
Figure 1. Best Tree models and normalized features (Nmean, NSD). Graphs report tree decision paths and feature thresholds to predict fake (F) and genuine (G) expressions. Image is modified from Cardaioli et al. (2022).
Although several studies have reported promising detection accuracy with intra-dataset testing scenarios, another drawback of ML application is that the performance can vary widely applying the same detection method to different databases (Jia et al., 2021). The weak consistency among the results may be due to the high inter-individual variability in the facial displays of emotions (Holberg et al., 2006; Sangineto et al., 2014; Durán et al., 2017). In general, the datasets used for training models do not adequately consider the real-world scenarios variability, an effect called “dataset bias effect” (Khosla et al., 2012). Although researchers have strong incentives to reduce the impact of individual differences as much as possible (e.g., to increase effect size and improve statistical power), the inter-variability among individuals should not be neglected in favor of a more generalist approach. Facial displays are not identical for different subjects, nor even for the same emotion (Sadeghi et al., 2013; Sangineto et al., 2014; Durán et al., 2017). A recent paper (Cardaioli et al., 2022) capitalized the PEDFE dataset described above (Miolla et al., 2022). The PEDFE dataset is unique to explore inter-individual differences in emotional expression, as, besides including genuine and posed dynamic emotional expression, it also includes many emotional stimuli for each “actor,” where the same emotion is expressed with different intensities or response to different stimuli. This allows to have a wide range of genuine expressions of the same emotion for each participant and to test the ability of ML models to discriminate genuine and posed emotions at the level of the single individual. An overall accuracy of 84.4% was achieved when applying ML models at the level of the single individual (i.e., for each subject, ML models can correctly discriminate genuine or posed emotions in the 84% of cases), as compared to the 67.0% when applying group-level algorithms. In general, these results suggest that it could be more reliable to detect unique deceptive cues for each subject instead of identifying a common rule to discriminate spontaneous and posed emotional facial expressions.
Recently, ML techniques have been used by Cowen et al. (2021) to address the debate about universal facial expressions by analyzing more than 6 million YouTube videos. The researchers used a powerful ML method involving deep neural networks (DNNs) to assess the extent to which specific facial configurations could be reliably observed in the videos across cultures. They found that people around the world make similar facial expressions in similar social contexts. Needless to say, the result is extremely interesting. On the other hand, there is no guarantee about the actual emotions felt by the people in those videos. A marriage context, for example, may lead one to believe that the emotions manifested are of joy. But this is all to be proven. In fact, the DNN learnt from human evaluators, who annotated the facial movements contained in each videoclip by choosing from a set of English words. The raters were, in effect, offering inferences about the emotional meaning of the facial movements (Barrett, 2021). Data science and algorithms can work very well on large numbers, but if the source lacks an accurate and reliable indication about the real emotion experienced by people, the whole analysis is tainted. In general, the great limitation of ML technology is that how you train the algorithm will determine the outcome, in a self-referential way. That is why we propose to train ML algorithms with large - already separated - datasets of authentic and posed expressions, scientifically controlled and rich in psychophysiological information (e.g., ECG, EDA, self-report). Google can offer emotion science huge sets of real, but uncontrolled expressions, totally devoid of psychophysiological correlates and distorted by the personal beliefs of the researcher who selected the specific stimuli for the experiment. The solution we propose is to make a major collective effort at the level of the scientific community to create large, rich, multifaceted ecological datasets to take full advantage of the enormous potential of ML. Once these datasets have been acquired in controlled environments, it will be possible to use them to train algorithms that can also function in natural environments, which are by definition less controlled. Indeed, the ultimate goal of this process will be to create increasingly complex algorithms capable of extracting key features even from data collected in natural scenarios. Including dynamic and interactive information instead of limiting the science of emotions to an “individual peak” would be important in modern emotion research, especially considering today’s technological capabilities. We believe that only accurate triangulation of physiological, behavioral, and self-report data can ensure accurate identification of the emotion experienced. And only this data can then be properly used by the ML for the generation of predictive algorithms.
To sum up, these results indicate that both manual and automated coding have temporal, spatial and reliability limitations. We suggest that the true move towards an objective analysis of emotional function will begin with the 3-D tracking of small configurations of points (landmarks) to define a unique set of universal and easily recognizable reference points for extracting the kinematics of face and body movements in a replicable manner. A simple model would allow to analyze separately the upper and lower face and to compare the left and right faces (see Figure 2). Moreover, considering the relative position of couples of points instead of single points would allow to neutralize possible head movements. This methodological step is nowadays crucial, since the variety of evaluation methods and evaluated movements that are present in the literature does not suggest a unique and easily applicable standardized method. An exhaustive quantitative analysis of facial and body motion kinematics shall then be integrated with EMG (Hess et al., 2017; Beringer et al., 2019), physiological indexes such as pupil dilation (Brod, 2021), gaze (D’Mello et al., 2012), heart rate variability (Dell’Acqua et al., 2020; da Estrela et al., 2021), and self-reports (Durán et al., 2017). Such a fully integrated database would allow to extract multi-modal detection algorithms able to discriminate the specific patterns of a wide range of blended emotion displays, and to assess the efficiency of emotional expressivity also in pathological conditions (e.g., ASD syndrome). Interestingly, these algorithms would be easily applicable in ecological contexts (e.g., with smartphone Apps; see Figure 2) for the assessment of inter-individual and cultural differences.
Figure 2. We propose an integrated methodology (i.e., kinematical 3-D analysis of movement, pupil dilation, gaze, EMG, heart rate variability, self-reports, and machine learning) to extract multi-modal detection algorithms able to discriminate the specific patterns of a wide range of blended emotion displays even in ecological contexts (e.g., with smartphone Apps).
New databases of genuine displays will also allow to overcome a critical issue related to emotion discrimination tasks (Barrett et al., 2019). Although the forced-choice paradigm classically adopted in emotion recognition tasks might yield robust results, it lacks ecological validity since it forces the use of labels that might not otherwise be selected. An essential improvement in the actual literature would be obtained by combining free-response tasks with the kinematic analysis of emotional movements, which may allow to better understand and describe participants’ emotional reactions. In the free-response tasks, participants would be allowed to decode the type of observed emotion into continuous emotion ratings as expressions progress over time, while judging dimensions of valence and intensity. Accurately assessing kinematic parameters in these tasks would then provide an implicit measure of the association between observed emotions and participants’ reactions (i.e., motor contagion; Borgomaneri et al., 2020).
One reason for the relative lack of previous research attention to whole-body displays is the absence of a precise navigation system for coding emotions from movements. Targeting the 3-dimensional space to expand our understanding of how facial and body displays unfold over time is therefore of primary importance. Studying the dynamic aspects of emotional expressions will open a new stage in understanding how they are expressed and recognized. Furthermore, we maintain that the most viable solution to start better addressing the ecological validity aspect is defining an innovative approach to emotional testing. Novel and more integrated databases of genuine dynamic stimuli will meet new demands in research on human communication, machine recognition, and human-computer interaction. This approach can potentially lead us to the roots of emotional display, and to the individual mechanisms underlying their expression (i.e., emotional signatures). Kinematics, moreover, might allow to disentangle, from a very innovative perspective, the relative role of experience and culture in shaping emotional expression. By opening up the field to a richer description, new fields of neuroscientific inquiry will consequently emerge.
In applicative terms, providing a new database that can span a large range of spontaneous emotion expressions would pave the way for human-computer interaction research (Pantic and Bartlett, 2007). Increasing efforts are nowadays targeted towards developing robotic systems able to recognize and respond to emotional signals, which can be applied in fields such as security, medicine, education, and digital communication. A high-dimensional taxonomy will open a new stage in understanding how emotional expressions are recognized, allowing to develop new algorithms which would be an alternative to those commonly used to detect emotions. Companies and governments are spending billions of dollars in trying to improve the way emotions are detected. Tech giants strive to improve algorithms designed to detect a person’s emotions to assess the suitability of job candidates, detect lies, make adverts more alluring and diagnose disorders from dementia to depression. Estimates place the industry’s value for this research at tens of billions of dollars. However, we shall look at the full picture, as faces alone do not reveal much about emotions. In the future, cooperative efforts between psychology and computer science are indispensable (e.g., Valstar et al., 2015). For knowledge transfer and dialogue to increase, researchers from both sides will have to embrace unique and rich stimulus datasets.
Creating a high-dimensional and total-body taxonomy of emotion expression will also offer invaluable information to programs that seek to train children who live with autism (Wieckowski and White, 2017) and other conditions defined by difficulties in representing and reading one’s own and others’ emotions (e.g., Alexithymia). Such a taxonomy will allow investigations throughout life span – from childhood to old age – and will allow to identify functionally-relevant biomarkers that can early reveal disease onset. For instance, in stroke patients - where the hemispheric damage translates into asymmetries in contralateral facial expressions of emotion.
At present, there is still no standardized method to evaluate the accuracy and efficiency of full-body emotion expressivity, which could help in diagnosis, treatment planning, and post-treatment follow-up (Trotman et al., 2005). The absence of an accurate and universally accepted grading system for assessing the severity of emotional impairment makes comparisons of results invalid.
Among the quantitative instruments recently developed for the assessment of emotional movements, 3-D motion analyzers appear the most suitable for the collection of data in a great range of patients. They allow a complete and detailed assessment of motion in all parts of the face and body, and quantitative data can be compared between and within individuals (Coulson et al., 2002; Mishima et al., 2004; Nooreyazdan et al., 2004). A full understanding of emotional expression requires an appreciation of a wide degree of variability in display behavior, both within and across emotion categories. By introducing the concept of “individual emotional performance,” researchers will also provide a reference to compare long-term performance.
A few years ago, Chris Anderson - the editor of Wired - wrote an article titled “The end of theory: the data deluge makes the scientific method obsolete” (Anderson, 2005). Anderson argued the provocative thesis that with the advent of digital and the computational capabilities of supercomputers, theory is now useless. He also claimed that correlation prevails over causation, and science can progress even without coherent models or unique theories. In short, why should we waste time searching for causal relationships that explain what happens in the world when it is intellectually less demanding to entrust the machine (e.g., AI, ML) with the search for highly effective correlations? Indeed, because the characteristic feature of the digital is to record everything it comes into contact with, humanity is now awash in an unprecedented deluge of data: a huge archive of all human life forms.
We speculate that it is precisely this immense growth of data that requires theories capable of governing what would otherwise be chaos from a cognitive point of view. No one would be satisfied to explain the workings of the universe by resorting to simple correlations, because at that point there would be no difference between an astronomer and an astrologer. Mankind has made huge progress thanks to experimental science and unique theories.
The fact that of many correlations we cannot understand the cause does not eliminate the need for the theory, quite the contrary. The social world and its emotions, in particular, has long been considered the domain of the unpredictable. But now that human actions can be recorded in minute detail, human behavior can be understood and interpreted. Here is where collaboration between researchers, philosophers and engineers becomes essential.
A growing body of evidence suggests that each emotional display is a complex, multi-component, motoric event. Human face is never static, but it continuously acts and reacts to internal and environmental stimuli, with the coordinated action of the facial muscles (Calvo et al., 2018). Most research on facial expression, however, has used static and posed expressions as stimuli, obtained from standardized databases (for a review, see Calvo and Nummenmaa, 2016). Yet, dynamic changes in the facial expression of emotions are a particularly valuable source of information: they indicate changes in the emotional state of other individuals. Our understanding of such dynamic information and the corresponding dynamic expression databases are so far very limited (for a review, see Krumhuber et al., 2017). Moreover, no study has yet combined EMG with 3-D motion analysis to provide a full spatio-temporal characterization of blended emotional expression at both the muscular and kinematic levels. We propose that richer and larger data sets - including facial blends of emotions, will provide an ideal test case for studying emotion expressions. The search for this emotional taxonomy, coupled with more powerful quantitative approaches, will in turn allow a better understanding of the rules governing the syntax of facial expressions.
This knowledge might eventually have large implications in the strongly debated issue concerning the role of nature and culture on the expression of emotions. Note that this debate arose exactly 150 years ago, when Darwin (1872) proposed facial expressions of emotion to be universal. We believe it is time to overhaul the science of emotion with better tools and more valid experimental designs.
All authors contributed to conception of the review. LS and ES wrote the first draft of the manuscript. CS wrote sections of the manuscript. All authors contributed to the article and approved the submitted version.
The present work was carried out within the scope of the research programme “Dipartimenti di Eccellenza” (art.1, commi 314-337 legge 232/2016), which was supported by a grant from MIUR to the Department of General Psychology, University of Padova.
The authors declare that the research was conducted in the absence of any commercial or financial relationships that could be construed as a potential conflict of interest.
All claims expressed in this article are solely those of the authors and do not necessarily represent those of their affiliated organizations, or those of the publisher, the editors and the reviewers. Any product that may be evaluated in this article, or claim that may be made by its manufacturer, is not guaranteed or endorsed by the publisher.
Abbruzzese, L., Magnani, N., Robertson, I. H., and Mancuso, M. (2019). Age and gender differences in emotion recognition. Front. Psychol. 10:2371. doi: 10.3389/fpsyg.2019.02371
Adolphs, R., Mlodinow, L., and Barrett, L. F. (2019). What is an emotion? Curr. Biol. 29, R1060–R1064. doi: 10.1016/j.cub.2019.09.008
Alaerts, K., Nackaerts, E., Meyns, P., Swinnen, S. P., and Wenderoth, N. (2011). Action and emotion recognition from point light displays: an investigation of gender differences. PLoS One 6:e20989. doi: 10.1371/journal.pone.0020989
Ambadar, Z., Schooler, J. W., and Cohn, J. F. (2005). Deciphering the enigmatic face: the importance of facial dynamics in interpreting subtle facial expressions. Psychol. Sci. 16, 403–410. doi: 10.1111/j.0956-7976.2005.01548.x
Anderson, C. (2005). The end of theory: the data deluge makes the scientific method obsolete. Wired Available at: https://www.wired.com/2008/06/pb-theory/ (Accessed February 17, 2023).
Atkinson, A. P., Dittrich, W. H., Gemmell, A. J., and Young, A. W. (2004). Emotion perception from dynamic and static body expressions in point-light and full-light displays. Perception 33, 717–746. doi: 10.1068/p5096
Aviezer, H., Trope, Y., and Todorov, A. (2012). Body cues, not facial expressions, discriminate between intense positive and negative emotions. Science 338, 1225–1229. doi: 10.1126/science.1224313
Baker, A., Black, P. J., and Porter, S. (2016). “The truth is written all over your face! Involuntary aspects of emotional facial expressions,” in The expression of emotion: Philosophical, psychological and legal perspectives Studies in Emotion and Social Interaction. eds. C. Abell and J. Smith (Cambridge: Cambridge University Press), 219–244.
Bänziger, T., Mortillaro, M., and Scherer, K. R. (2012). Introducing the Geneva multimodal expression corpus for experimental research on emotion perception. Emotion 12, 1161–1179. doi: 10.1037/a0025827
Barrett, L. F. (2017). How emotions are made: The secret life of the brain. Boston, MA: Houghton Mifflin Harcourt.
Barrett, L. F. (2021). AI weighs in on debate about universal facial expressions. Nature 589, 202–203. doi: 10.1038/d41586-020-03509-5
Barrett, L. F., Adolphs, R., Marsella, S., Martinez, A. M., and Pollak, S. D. (2019). Emotional expressions reconsidered: challenges to inferring emotion from human facial movements. Psychol. Sci. Public Interest J. Am. Psychol. Soc. 20, 1–68. doi: 10.1177/1529100619832930
Barrett, L. F., Mesquita, B., and Gendron, M. (2011). Context in emotion perception. Curr. Dir. Psychol. Sci. 20, 286–290. doi: 10.1177/0963721411422522
Bartlett, M. S., Littlewort, G. C., Frank, M. G., and Lee, K. (2014). Automatic decoding of facial movements reveals deceptive pain expressions. Curr. Biol. 24, 738–743. doi: 10.1016/j.cub.2014.02.009
Benitez-Quiroz, C. F., Wang, Y., and Martinez, A. M. (2017). “Recognition of action units in the wild with deep nets and a new global-local loss.” in 2017 IEEE International Conference on Computer Vision (ICCV). Venice: IEEE, 3990–3999.
Beringer, M., Spohn, F., Hildebrandt, A., Wacker, J., and Recio, G. (2019). Reliability and validity of machine vision for the assessment of facial expressions. Cogn. Syst. Res. 56, 119–132. doi: 10.1016/j.cogsys.2019.03.009
Bernstein, M., and Yovel, G. (2015). Two neural pathways of face processing: a critical evaluation of current models. Neurosci. Biobehav. Rev. 55, 536–546. doi: 10.1016/j.neubiorev.2015.06.010
Biehl, M., Matsumoto, D., Ekman, P., Hearn, V., Heider, K., Kudoh, T., et al. (1997). Matsumoto and Ekman’s Japanese and Caucasian facial expressions of emotion (JACFEE): reliability data and cross-national differences. J. Nonverbal Behav. 21, 3–21. doi: 10.1023/A:1024902500935
Borgomaneri, S., Vitale, F., and Avenanti, A. (2020). Early motor reactivity to observed human body postures is affected by body expression, not gender. Neuropsychologia 146:107541. doi: 10.1016/j.neuropsychologia.2020.107541
Borgomaneri, S., Vitale, F., Gazzola, V., and Avenanti, A. (2015). Seeing fearful body language rapidly freezes the observer’s motor cortex. Cortex 65, 232–245. doi: 10.1016/j.cortex.2015.01.014
Bradley, M. M., Miccoli, L., Escrig, M. A., and Lang, P. J. (2008). The pupil as a measure of emotional arousal and autonomic activation. Psychophysiology 45, 602–607. doi: 10.1111/j.1469-8986.2008.00654.x
Brod, G. (2021). Predicting as a learning strategy. Psychon. Bull. Rev. 28, 1839–1847. doi: 10.3758/s13423-021-01904-1
Calvo, M. G., Fernández-Martín, A., Recio, G., and Lundqvist, D. (2018). Human observers and automated assessment of dynamic emotional facial expressions: KDEF-dyn database validation. Front. Psychol. 9:2052. doi: 10.3389/fpsyg.2018.02052
Calvo, M. G., and Nummenmaa, L. (2016). Perceptual and affective mechanisms in facial expression recognition: an integrative review. Cognit. Emot. 30, 1081–1106. doi: 10.1080/02699931.2015.1049124
Cardaioli, M., Miolla, A., Conti, M., Sartori, G., Monaro, M., Scarpazza, C., et al. (2022). “Face the truth: interpretable emotion genuineness detection,” in 2022 International Joint Conference on Neural Networks (IJCNN). 1–8.
Carvalho, D. V., Pereira, E. M., and Cardoso, J. S. (2019). Machine learning interpretability: a survey on methods and metrics. Electronics 8:832. doi: 10.3390/electronics8080832
Ceccarini, F., and Caudek, C. (2013). Anger superiority effect: the importance of dynamic emotional facial expressions. Vis. Cogn. 21, 498–540. doi: 10.1080/13506285.2013.807901
Chu, W., Torre, F. D.la, and Cohn, J. F. (2017). “Learning spatial and temporal cues for multi-label facial action unit detection.” in 2017 12th IEEE International Conference on Automatic Face Gesture Recognition (FG 2017). pp. 25–32.
Cohn, J. F., and Schmidt, K. L. (2004). The timing of facial motion in posed and spontaneous smiles. Int. J. Wavelets Multiresolution Inf. Process 2, 121–132. doi: 10.1142/S021969130400041X
Connolly, H. L., Lefevre, C. E., Young, A. W., and Lewis, G. J. (2019). Sex differences in emotion recognition: evidence for a small overall female superiority on facial disgust. Emotion 19, 455–464. doi: 10.1037/emo0000446
Cordaro, D. T., Sun, R., Keltner, D., Kamble, S., Huddar, N., and McNeil, G. (2018). Universals and cultural variations in 22 emotional expressions across five cultures. Emotion 18, 75–93. doi: 10.1037/emo0000302
Coulson, S. E., Croxson, G. R., and Gilleard, W. L. (2002). Three-dimensional quantification of the symmetry of normal facial movement. Otol. Neurotol. Off. Publ. Am. Otol. Soc. Am. Neurotol. Soc. Eur. Acad. 23, 999–1002. doi: 10.1097/00129492-200211000-00032
Cowen, A. S., Keltner, D., Schroff, F., Jou, B., Adam, H., and Prasad, G. (2021). Sixteen facial expressions occur in similar contexts worldwide. Nature 589, 251–257. doi: 10.1038/s41586-020-3037-7
Crivelli, C., and Fridlund, A. J. (2019). Inside-out: from basic emotions theory to the behavioral ecology view. J. Nonverbal Behav. 43, 161–194. doi: 10.1007/s10919-019-00294-2
Cunningham, D. W., and Wallraven, C. (2009). Dynamic information for the recognition of conversational expressions. J. Vis. 9:7. doi: 10.1167/9.13.7
D’Mello, S., Olney, A., Williams, C., and Hays, P. (2012). Gaze tutor: a gaze-reactive intelligent tutoring system. Int. J. Hum.-Comput. Stud. 70, 377–398. doi: 10.1016/j.ijhcs.2012.01.004
da Estrela, C., MacNeil, S., and Gouin, J.-P. (2021). Heart rate variability moderates the between- and within-person associations between daily stress and negative affect. Int. J. Psychophysiol. 162, 79–85. doi: 10.1016/j.ijpsycho.2021.02.001
Dael, N., Mortillaro, M., and Scherer, K. R. (2012). Emotion expression in body action and posture. Emotion 12, 1085–1101. doi: 10.1037/a0025737
Damasio, A., and Carvalho, G. B. (2013). The nature of feelings: evolutionary and neurobiological origins. Nat. Rev. Neurosci. 14, 143–152. doi: 10.1038/nrn3403
Darwin, C. (1872). The expression of the emotions in man and animals. Cambridge: Cambridge University Press.
Dawel, A., Miller, E. J., Horsburgh, A., and Ford, P. (2022). A systematic survey of face stimuli used in psychological research 2000–2020. Behav. Res. Methods 54, 1889–1901. doi: 10.3758/s13428-021-01705-3
Dawel, A., Palermo, R., O’Kearney, R., and McKone, E. (2015). Children can discriminate the authenticity of happy but not sad or fearful facial expressions, and use an immature intensity-only strategy. Front. Psychol. 6:462. doi: 10.3389/fpsyg.2015.00462
Dawel, A., Wright, L., Irons, J., Dumbleton, R., Palermo, R., O’Kearney, R., et al. (2017). Perceived emotion genuineness: normative ratings for popular facial expression stimuli and the development of perceived-as-genuine and perceived-as-fake sets. Behav. Res. Methods 49, 1539–1562. doi: 10.3758/s13428-016-0813-2
de Gelder, B. (2009). Why bodies? Twelve reasons for including bodily expressions in affective neuroscience. Philos. Trans. R. Soc. Lond. Ser. B Biol. Sci. 364, 3475–3484. doi: 10.1098/rstb.2009.0190
de Gelder, B., de Borst, A. W., and Watson, R. (2015). The perception of emotion in body expressions. Wiley Interdiscip. Rev. Cogn. Sci. 6, 149–158. doi: 10.1002/wcs.1335
de Gelder, B., Snyder, J., Greve, D., Gerard, G., and Hadjikhani, N. (2004). Fear fosters flight: a mechanism for fear contagion when perceiving emotion expressed by a whole body. Proc. Natl. Acad. Sci. 101, 16701–16706. doi: 10.1073/pnas.0407042101
de Gelder, B., and Van den Stock, J. (2011). The bodily expressive action stimulus test (BEAST). Construction and validation of a stimulus basis for measuring perception of whole body expression of emotions. Front. Psychol. 2:181. doi: 10.3389/fpsyg.2011.00181
Delis, I., Chen, C., Jack, R. E., Garrod, O. G. B., Panzeri, S., and Schyns, P. G. (2016). Space-by-time manifold representation of dynamic facial expressions for emotion categorization. J. Vis. 16:14. doi: 10.1167/16.8.14
Dell’Acqua, C., Dal Bò, E., Messerotti Benvenuti, S., and Palomba, D. (2020). Reduced heart rate variability is associated with vulnerability to depression. J. Affect. Disord. Rep. 1:100006. doi: 10.1016/j.jadr.2020.100006
Demaree, H. A., Everhart, D. E., Youngstrom, E. A., and Harrison, D. W. (2005). Brain lateralization of emotional processing: historical roots and a future incorporating “dominance”. Behav. Cogn. Neurosci. Rev. 4, 3–20. doi: 10.1177/1534582305276837
Dimberg, U., Andréasson, P., and Thunberg, M. (2011). Emotional empathy and facial reactions to facial expressions. J. Psychophysiol. 25, 26–31. doi: 10.1027/0269-8803/a000029
Douglas, K. M., Porter, R. J., and Johnston, L. (2012). Sensitivity to posed and genuine facial expressions of emotion in severe depression. Psychiatry Res. 196, 72–78. doi: 10.1016/j.psychres.2011.10.019
Du, S., Tao, Y., and Martinez, A. M. (2014). Compound facial expressions of emotion. Proc. Natl. Acad. Sci. 111, E1454–E1462. doi: 10.1073/pnas.1322355111
Durán, J. I., Reisenzein, R., and Fernández-Dols, J.-M. (2017). “Coherence between emotions and facial expressions: a research synthesis” in The science of facial expression Oxford series in social cognition and social neuroscience (New York, NY, US: Oxford University Press), 107–129.
Ekman, P. (1965). Differential communication of affect by head and body cues. J. Pers. Soc. Psychol. 2, 726–735. doi: 10.1037/h0022736
Ekman, P. (1992a). An argument for basic emotions. Cognit. Emot. 6, 169–200. doi: 10.1080/02699939208411068
Ekman, P. (1992b). Are there basic emotions? Psychol. Rev. 99, 550–553. doi: 10.1037/0033-295X.99.3.550
Ekman, P. (2003). Emotions inside out. 130 years after Darwin’s “the expression of the emotions in man and animal”. Ann. N. Y. Acad. Sci. 1000, 1–6. doi: 10.1196/annals.1280.002
Ekman, P. (2009). “Lie Catching and Micro Expressions,” in The Philosophy of Deception. ed. C. Martin (New York, NY: Oxford University Press).
Ekman, P. (2016). What scientists who study emotion agree about. Perspect. Psychol. Sci. 11, 31–34. doi: 10.1177/1745691615596992
Ekman, P., and Cordaro, D. (2011). What is meant by calling emotions basic. Emot. Rev. 3, 364–370. doi: 10.1177/1754073911410740
Ekman, P., and Friesen, W. V. (1971). Constants across cultures in the face and emotion. J. Pers. Soc. Psychol. 17, 124–129. doi: 10.1037/h0030377
Ekman, P., and Friesen, W. V. (1976). Pictures of facial affect. Palo Alto, Calif: Consulting Psychologists Press.
Ekman, P., and Friesen, W. (1978). Facial action coding system: A technique for the measurement of facial movement. Palo Alto: Consulting Psychologists Press.
Ekman, P., and Friesen, W. V. (1982). Felt, false, and miserable smiles. J. Nonverbal Behav. 6, 238–252. doi: 10.1007/BF00987191
Ekman, P., Friesen, W. V., and Hager, J. C. (2002). Facial action coding system. Manual and investigator’s guide. Salt Lake City, UT: Research Nexus.
Ekman, P., Friesen, W. V., and O’Sullivan, M. (1988). Smiles when lying. J. Pers. Soc. Psychol. 54, 414–420. doi: 10.1037/0022-3514.54.3.414
Ekman, P., and Rosenberg, E. L. (2005). What the face reveals: Basic and applied studies of spontaneous expression using the facial action coding system (FACS) Oxford University Press.
Ekman, P., Sorenson, E. R., and Friesen, W. V. (1969). Pan-cultural elements in facial displays of emotion. Science 164, 86–88. doi: 10.1126/science.164.3875.86
Frank, M. G., Ekman, P., and Friesen, W. V. (1993). Behavioral markers and recognizability of the smile of enjoyment. J. Pers. Soc. Psychol. 64, 83–93. doi: 10.1037//0022-3514.64.1.83
Gazzaniga, M. S., and Smylie, C. S. (1990). Hemispheric mechanisms controlling voluntary and spontaneous facial expressions. J. Cogn. Neurosci. 2, 239–245. doi: 10.1162/jocn.1990.2.3.239
Gray, E. K., and Watson, D. (2007). “Assessing positive and negative affect via self-report” in Handbook of emotion elicitation and assessment series in affective science. eds. J. A. Coan and J. B. Allen (New York, NY, US: Oxford University Press), 171–183.
Gu, S., Wang, F., Patel, N. P., Bourgeois, J. A., and Huang, J. H. (2019). A model for basic emotions using observations of behavior in drosophila. Front. Psychol. 10:781. doi: 10.3389/fpsyg.2019.00781
Guerdelli, H., Ferrari, C., Barhoumi, W., Ghazouani, H., and Berretti, S. (2022). Macro- and micro-expressions facial datasets: a survey. Sensors 22:1524. doi: 10.3390/s22041524
Hao, L., Wang, S., Peng, G., and Ji, Q. (2018). “Facial action unit recognition augmented by their dependencies.” in 2018 13th IEEE International Conference on Automatic Face Gesture Recognition (FG 2018). pp. 187–194.
Heaven, D. (2020). Why faces don’t always tell the truth about feelings. Nature 578, 502–504. doi: 10.1038/d41586-020-00507-5
Hess, U., Arslan, R., Mauersberger, H., Blaison, C., Dufner, M., Denissen, J. J. A., et al. (2017). Reliability of surface facial electromyography. Psychophysiology 54, 12–23. doi: 10.1111/psyp.12676
Hess, U., and Fischer, A. (2013). Emotional mimicry as social regulation. Personal. Soc. Psychol. Rev. 17, 142–157. doi: 10.1177/1088868312472607
Hoffmann, H., Kessler, H., Eppel, T., Rukavina, S., and Traue, H. C. (2010). Expression intensity, gender and facial emotion recognition: women recognize only subtle facial emotions better than men. Acta Psychol. 135, 278–283. doi: 10.1016/j.actpsy.2010.07.012
Holberg, C., Maier, C., Steinhäuser, S., and Rudzki-Janson, I. (2006). Inter-individual variability of the facial morphology during conscious smiling. J. Orofac. Orthop. Fortschritte Kieferorthopädie 67, 234–243. doi: 10.1007/s00056-006-0518-8
Hopf, H. C., Md, W. M.-F., and Hopf, N. J. (1992). Localization of emotional and volitional facial paresis. Neurology 42:1918. doi: 10.1212/WNL.42.10.1918
Izard, C. E. (2010). The many meanings/aspects of emotion: definitions, functions, activation, and regulation. Emot. Rev. 2, 363–370. doi: 10.1177/1754073910374661
Jack, R. E., Sun, W., Delis, I., Garrod, O. G. B., and Schyns, P. G. (2016). Four not six: revealing culturally common facial expressions of emotion. J. Exp. Psychol. Gen. 145, 708–730. doi: 10.1037/xge0000162
Jia, S., Wang, S., Hu, C., Webster, P. J., and Li, X. (2021). Detection of genuine and posed facial expressions of emotion: databases and methods. Front. Psychol. 11:580287. doi: 10.3389/fpsyg.2020.580287
Kanade, T., Cohn, J. F., and Tian, Y. (2000). “Comprehensive database for facial expression analysis.” in Proceedings Fourth IEEE International Conference on Automatic Face and Gesture Recognition (Cat. No. PR00580). pp. 46–53.
Keltner, D. (1995). Signs of appeasement: evidence for the distinct displays of embarrassment, amusement, and shame. J. Pers. Soc. Psychol. 68, 441–454. doi: 10.1037/0022-3514.68.3.441
Keltner, D., and Cordaro, D. T. (2017). “Understanding multimodal emotional expressions: recent advances in basic emotion theory,” in The science of facial expression, Oxford series in social cognition and social neuroscience (New York, NY, US: Oxford University Press), 57–75.
Keltner, D., Sauter, D., Tracy, J., and Cowen, A. (2019). Emotional expression: advances in basic emotion theory. J. Nonverbal Behav. 43, 133–160. doi: 10.1007/s10919-019-00293-3
Keltner, D., Sauter, D., Tracy, J., McNeil, G., and Cordaro, D. T. (2016). “Expression,” in Handbook of emotion. eds. L. F. Barrett, M. Lewis and J. Haviland-Jones (New York, NY: Guilford Press), 467–482.
Khosla, A., Zhou, T., Malisiewicz, T., Efros, A. A., and Torralba, A. (2012). “Undoing the damage of dataset bias,” in Computer vision – ECCV 2012 lecture notes in computer science. eds. A. Fitzgibbon, S. Lazebnik, P. Perona, Y. Sato, and C. Schmid (Berlin, Heidelberg: Springer), 158–171.
Killgore, W. D. S., and Yurgelun-Todd, D. A. (2007). The right-hemisphere and valence hypotheses: could they both be right (and sometimes left)? Soc. Cogn. Affect. Neurosci. 2, 240–250. doi: 10.1093/scan/nsm020
Kreibig, S. D. (2010). Autonomic nervous system activity in emotion: a review. Biol. Psychol. 84, 394–421. doi: 10.1016/j.biopsycho.2010.03.010
Kret, M. E., and De Gelder, B. (2012). A review on sex differences in processing emotional signals. Neuropsychologia 50, 1211–1221. doi: 10.1016/j.neuropsychologia.2011.12.022
Krippl, M., Karim, A. A., and Brechmann, A. (2015). Neuronal correlates of voluntary facial movements. Front. Hum. Neurosci. 9:598. doi: 10.3389/fnhum.2015.00598
Krumhuber, E. G., Kappas, A., and Manstead, A. S. R. (2013). Effects of dynamic aspects of facial expressions: a review. Emot. Rev. 5, 41–46. doi: 10.1177/1754073912451349
Krumhuber, E. G., Skora, L., Küster, D., and Fou, L. (2017). A review of dynamic datasets for facial expression research. Emot. Rev. 9, 280–292. doi: 10.1177/1754073916670022
Lander, K., and Butcher, N. L. (2020). Recognizing genuine from posed facial expressions: exploring the role of dynamic information and face familiarity. Front. Psychol. 11:1378. doi: 10.3389/fpsyg.2020.01378
Larsen, J. T., McGraw, A. P., and Cacioppo, J. T. (2001). Can people feel happy and sad at the same time? J. Pers. Soc. Psychol. 81, 684–696. doi: 10.1037//0022-3514.81.4.684
LeDoux, J. E. (1995). Emotion: clues from the brain. Annu. Rev. Psychol. 46, 209–235. doi: 10.1146/annurev.ps.46.020195.001233
LeDoux, J. E. (2012). Rethinking the emotional brain. Neuron 73, 653–676. doi: 10.1016/j.neuron.2012.02.004
Lenzoni, S., Bozzoni, V., Burgio, F., de Gelder, B., Wennberg, A., Botta, A., et al. (2020). Recognition of emotions conveyed by facial expression and body postures in myotonic dystrophy (DM). Cortex J. Devoted Study Nerv. Syst. Behav. 127, 58–66. doi: 10.1016/j.cortex.2020.02.005
Li, Y., Li, F., Chen, J., and Li, H. (2012). An ERP study on the understanding of the distinction between real and apparent emotions. Neurosci. Lett. 529, 33–38. doi: 10.1016/j.neulet.2012.08.063
Lindquist, K. A., Siegel, E. H., Quigley, K. S., and Barrett, L. F. (2013). The hundred-year emotion war: are emotions natural kinds or psychological constructions? Comment on. Psychol. Bull. 139, 255–263. doi: 10.1037/a0029038
Lott, L. L., Spengler, F. B., Stächele, T., Schiller, B., and Heinrichs, M. (2022). EmBody/EmFace as a new open tool to assess emotion recognition from body and face expressions. Sci. Rep. 12:14165. doi: 10.1038/s41598-022-17866-w
Lucey, P., Cohn, J. F., Kanade, T., Saragih, J., Ambadar, Z., and Matthews, I. (2010). “The extended Cohn-Kanade dataset (CK+): a complete dataset for action unit and emotion-specified expression.” in 2010 IEEE Computer Society Conference on Computer Vision and Pattern Recognition – Workshops. pp. 94–101.
Lundqvist, D., Flykt, A., and Öhman, A. (1998). Karolinska directed emotional faces. Cognit. Emot. doi: 10.1037/2Ft27732-000
Malykhin, N., Pietrasik, W., Aghamohammadi-Sereshki, A., Ngan Hoang, K., Fujiwara, E., and Olsen, F. (2023). Emotional recognition across the adult lifespan: effects of age, sex, cognitive empathy, alexithymia traits, and amygdala subnuclei volumes. J. Neurosci. Res. 101, 367–383. doi: 10.1002/jnr.25152
Marini, M., Ansani, A., Paglieri, F., Caruana, F., and Viola, M. (2021). The impact of facemasks on emotion recognition, trust attribution and re-identification. Sci. Rep. 11:5577. doi: 10.1038/s41598-021-84806-5
Martinez, A. M. (2017). Computational models of face perception. Curr. Dir. Psychol. Sci. 26, 263–269. doi: 10.1177/0963721417698535
McLellan, T., Johnston, L., Dalrymple-Alford, J., and Porter, R. (2010). Sensitivity to genuine versus posed emotion specified in facial displays. Cognit. Emot. 24, 1277–1292. doi: 10.1080/02699930903306181
McLellan, T., Wilcke, J. C., Johnston, L., Watts, R., and Miles, L. K. (2012). Sensitivity to posed and genuine displays of happiness and sadness: a fMRI study. Neurosci. Lett. 531, 149–154. doi: 10.1016/j.neulet.2012.10.039
Meeren, H. K. M., van Heijnsbergen, C. C. R. J., and de Gelder, B. (2005). Rapid perceptual integration of facial expression and emotional body language. Proc. Natl. Acad. Sci. 102, 16518–16523. doi: 10.1073/pnas.0507650102
Miolla, A., Cardaioli, M., and Scarpazza, C. (2022). Padova emotional dataset of facial expressions (PEDFE): a unique dataset of genuine and posed emotional facial expressions. Behav. Res. Methods, 1–16. doi: 10.3758/s13428-022-01914-4
Mishima, K., Yamada, T., Fujiwara, K., and Sugahara, T. (2004). Development and clinical usage of a motion analysis system for the face: preliminary report. Cleft Palate Craniofac. J. 41, 559–564. doi: 10.1597/03-079.1
Mitchell, T. (1997). Machine learning textbook. McGraw-Hill. Available at: http://www.cs.cmu.edu/~tom/mlbook.html (Accessed August 30, 2022).
Monaro, M., Maldera, S., Scarpazza, C., Sartori, G., and Navarin, N. (2022). Detecting deception through facial expressions in a dataset of videotaped interviews: a comparison between human judges and machine learning models. Comput. Hum. Behav. 127:107063. doi: 10.1016/j.chb.2021.107063
Morecraft, R. J., Stilwell–Morecraft, K. S., and Rossing, W. R. (2004). The motor cortex and facial expression:: new insights from neuroscience. Neurologist 10, 235–249. doi: 10.1097/01.nrl.0000138734.45742.8d
Motley, M. T., and Camden, C. T. (1988). Facial expression of emotion: a comparison of posed expressions versus spontaneous expressions in an interpersonal communication setting. West. J. Speech Commun. 52, 1–22. doi: 10.1080/10570318809389622
Mulligan, K., and Scherer, K. R. (2012). Toward a working definition of emotion. Emot. Rev. 4, 345–357. doi: 10.1177/1754073912445818
Namba, S., Kabir, R. S., Miyatani, M., and Nakao, T. (2018). Dynamic displays enhance the ability to discriminate genuine and posed facial expressions of emotion. Front. Psychol. 9:672. doi: 10.3389/fpsyg.2018.00672
Namba, S., Makihara, S., Kabir, R. S., Miyatani, M., and Nakao, T. (2017). Spontaneous facial expressions are different from posed facial expressions: morphological properties and dynamic sequences. Curr. Psychol. 36, 593–605. doi: 10.1007/s12144-016-9448-9
Namba, S., Matsui, H., and Zloteanu, M. (2021). Distinct temporal features of genuine and deliberate facial expressions of surprise. Sci. Rep. 11:3362. doi: 10.1038/s41598-021-83077-4
Niedenthal, P. M., Mermillod, M., Maringer, M., and Hess, U. (2010). The Simulation of Smiles (SIMS) model: Embodied simulation and the meaning of facial expression. Behav. Brain Sci. 33, 417–433; 433–480. doi: 10.1017/S0140525X10000865
Nooreyazdan, M., Trotman, C.-A., and Faraway, J. J. (2004). Modeling facial movement: II. A dynamic analysis of differences caused by orthognathic surgery. J. Oral Maxillofac. Surg. 62, 1380–1386. doi: 10.1016/j.joms.2004.03.015
O’Reilly, H., Pigat, D., Fridenson, S., Berggren, S., Tal, S., Golan, O., et al. (2016). The EU-emotion stimulus set: a validation study. Behav. Res. Methods 48, 567–576. doi: 10.3758/s13428-015-0601-4
O’Toole, A. J., Harms, J., Snow, S. L., Hurst, D. R., Pappas, M. R., Ayyad, J. H., et al. (2005). A video database of moving faces and people. IEEE Trans. Pattern Anal. Mach. Intell. 27, 812–816. doi: 10.1109/TPAMI.2005.90
Palagi, E., Celeghin, A., Tamietto, M., Winkielman, P., and Norscia, I. (2020). The neuroethology of spontaneous mimicry and emotional contagion in human and non-human animals. Neurosci. Biobehav. Rev. 111, 149–165. doi: 10.1016/j.neubiorev.2020.01.020
Pantic, M., and Bartlett, M. S. (2007). “Machine analysis of facial expressions” in Face Recognition. eds. K. Delac and M. Grgic (Vienna, Austria: I-Tech Education and Publishing), 377–416.
Park, S., Lee, K., Lim, J.-A., Ko, H., Kim, T., Lee, J.-I., et al. (2020). Differences in facial expressions between spontaneous and posed smiles: automated method by action units and three-dimensional facial landmarks. Sensors 20:1199. doi: 10.3390/s20041199
Parr, L. A., Cohen, M., and de Waal, F. (2005). Influence of social context on the use of blended and graded facial displays in chimpanzees. Int. J. Primatol. 26, 73–103. doi: 10.1007/s10764-005-0724-z
Philippot, P. (1993). Inducing and assessing differentiated emotion-feeling states in the laboratory. Cognit. Emot. 7, 171–193. doi: 10.1080/02699939308409183
Poyo Solanas, M., Vaessen, M. J., and de Gelder, B. (2020). The role of computational and subjective features in emotional body expressions. Sci. Rep. 10:6202. doi: 10.1038/s41598-020-63125-1
Reader, A. T., and Holmes, N. P. (2016). Examining ecological validity in social interaction: problems of visual fidelity, gaze, and social potential. Cult. Brain 4, 134–146. doi: 10.1007/s40167-016-0041-8
Rinn, W. E. (1984). The neuropsychology of facial expression: a review of the neurological and psychological mechanisms for producing facial expressions. Psychol. Bull. 95, 52–77. doi: 10.1037/0033-2909.95.1.52
Roseman, I. J. (2011). Emotional behaviors, emotivational goals, emotion strategies: multiple levels of organization integrate variable and consistent responses. Emot. Rev. 3, 434–443. doi: 10.1177/1754073911410744
Rosenthal, R., Hall, J. A., DiMatteo, M. R., Rogers, P. L., and Archer, D. (1979). Sensitivity to nonverbal communication: The PONS test. Baltimore: The Johns Hopkins University Press.
Ross, E. D., Gupta, S. S., Adnan, A. M., Holden, T. L., Havlicek, J., and Radhakrishnan, S. (2016). Neurophysiology of spontaneous facial expressions: I. motor control of the upper and lower face is behaviorally independent in adults. Cortex. J. Devoted Study Nerv. Syst. Behav. 76, 28–42. doi: 10.1016/j.cortex.2016.01.001
Ross, E. D., Gupta, S. S., Adnan, A. M., Holden, T. L., Havlicek, J., and Radhakrishnan, S. (2019). Neurophysiology of spontaneous facial expressions: II. Motor control of the right and left face is partially independent in adults. Cortex 111, 164–182. doi: 10.1016/j.cortex.2018.10.027
Ross, E. D., Prodan, C. I., and Monnot, M. (2007). Human facial expressions are organized functionally across the upper-lower facial Axis. Neuroscientist 13, 433–446. doi: 10.1177/1073858407305618
Ruffman, T., Henry, J. D., Livingstone, V., and Phillips, L. H. (2008). A meta-analytic review of emotion recognition and aging: implications for neuropsychological models of aging. Neurosci. Biobehav. Rev. 32, 863–881. doi: 10.1016/j.neubiorev.2008.01.001
Russell, J. A. (1980). A circumplex model of affect. J. Pers. Soc. Psychol. 39, 1161–1178. doi: 10.1037/h0077714
Russell, J. A. (1994). Is there universal recognition of emotion from facial expression? A review of the cross-cultural studies. Psychol. Bull. 115, 102–141. doi: 10.1037/0033-2909.115.1.102
Russell, J. A., and Barrett, L. F. (1999). Core affect, prototypical emotional episodes, and other things called emotion: dissecting the elephant. J. Pers. Soc. Psychol. 76, 805–819. doi: 10.1037/0022-3514.76.5.805
Rymarczyk, K., Żurawski, Ł., Jankowiak-Siuda, K., and Szatkowska, I. (2016). Do dynamic compared to static facial expressions of happiness and anger reveal enhanced facial mimicry? PLoS One 11:e0158534. doi: 10.1371/journal.pone.0158534
Rymarczyk, K., Żurawski, Ł., Jankowiak-Siuda, K., and Szatkowska, I. (2019). Empathy in facial mimicry of fear and disgust: simultaneous EMG-fMRI recordings during observation of static and dynamic facial expressions. Front. Psychol. 10:701. doi: 10.3389/fpsyg.2019.00701
Sadeghi, H., Raie, A.-A., and Mohammadi, M.-R. (2013). “Facial expression recognition using geometric normalization and appearance representation.” in 2013 8th Iranian Conference on Machine Vision and Image Processing (MVIP). pp. 159–163.
Sangineto, E., Zen, G., Ricci, E., and Sebe, N. (2014). “We are not all equal: personalizing models for facial expression analysis with Transductive parameter transfer.” in Proceedings of the 22nd ACM international conference on Multimedia MM ‘14. New York, NY, USA: Association for Computing Machinery. pp. 357–366.
Sartori, L., Becchio, C., and Castiello, U. (2011). Cues to intention: the role of movement information. Cognition 119, 242–252. doi: 10.1016/j.cognition.2011.01.014
Sato, W., and Yoshikawa, S. (2004). BRIEF REPORT the dynamic aspects of emotional facial expressions. Cognit. Emot. 18, 701–710. doi: 10.1080/02699930341000176
Saylik, R., Raman, E., and Szameitat, A. J. (2018). Sex differences in emotion recognition and working memory tasks. Front. Psychol. 9:1072. doi: 10.3389/fpsyg.2018.01072
Scarpazza, C., di Pellegrino, G., and Làdavas, E. (2014). Emotional modulation of touch in alexithymia. Emotion 14, 602–610. doi: 10.1037/a0035888
Scherer, K. R. (2009). The dynamic architecture of emotion: evidence for the component process model. Cognit. Emot. 23, 1307–1351. doi: 10.1080/02699930902928969
Scherer, K. R., and Ellgring, H. (2007). Multimodal expression of emotion: affect programs or componential appraisal patterns? Emotion 7, 158–171. doi: 10.1037/1528-3542.7.1.158
Scherer, K. R., and Moors, A. (2019). The emotion process: event appraisal and component differentiation. Annu. Rev. Psychol. 70, 719–745. doi: 10.1146/annurev-psych-122216-011854
Schmidt, K. L., Ambadar, Z., Cohn, J. F., and Reed, L. I. (2006). Movement differences between deliberate and spontaneous facial expressions: zygomaticus major action in smiling. J. Nonverbal Behav. 30, 37–52. doi: 10.1007/s10919-005-0003-x
Schmidt, K. L., Cohn, J. F., and Tian, Y. (2003). Signal characteristics of spontaneous facial expressions: automatic movement in solitary and social smiles. Biol. Psychol. 65, 49–66. doi: 10.1016/S0301-0511(03)00098-X
Shan, C., Gong, S., and Mcowan, P. (2007). “Beyond facial expressions: learning human emotion from body gestures,’’ in BMV. 1-10.
Sidequersky, F. V., Verzé, L., Mapelli, A., Ramieri, G. A., and Sforza, C. (2014). Quantification of facial movements by optical instruments: surface laser scanning and optoelectronic three-dimensional motion analyzer. J. Craniofac. Surg. 25:e65. doi: 10.1097/SCS.0000000000000379
Sirois, S., and Brisson, J. (2014). Pupillometry. WIREs Cogn. Sci. 5, 679–692. doi: 10.1002/wcs.1323
Smith, M. L., Asada, N., and Malenka, R. C. (2021). Anterior cingulate inputs to nucleus accumbens control the social transfer of pain and analgesia. Science 371, 153–159. doi: 10.1126/science.abe3040
Sowden, S., Schuster, B. A., Keating, C. T., Fraser, D. S., and Cook, J. L. (2021). The role of movement kinematics in facial emotion expression production and recognition. Emotion 21, 1041–1061. doi: 10.1037/emo0000835
Starita, F., Borhani, K., Bertini, C., and Scarpazza, C. (2018). Alexithymia is related to the need for more emotional intensity to identify static fearful facial expressions. Front. Psychol. 9:929. doi: 10.3389/fpsyg.2018.00929
Tcherkassof, A., Bollon, T., Dubois, M., Pansu, P., and Adam, J.-M. (2007). Facial expressions of emotions: a methodological contribution to the study of spontaneous and dynamic emotional faces. Eur. J. Soc. Psychol. 37, 1325–1345. doi: 10.1002/ejsp.427
Tcherkassof, A., Dupré, D., Meillon, B., Mandran, N., Dubois, M., and Adam, J.-M. (2013). DynEmo: a video database of natural facial expressions of emotions. Int. J. Multimed. Its Appl. 5, 61–80. doi: 10.5121/ijma.2013.5505
Thoma, P., Soria Bauser, D., and Suchan, B. (2013). BESST (Bochum emotional stimulus set)--a pilot validation study of a stimulus set containing emotional bodies and faces from frontal and averted views. Psychiatry Res. 209, 98–109. doi: 10.1016/j.psychres.2012.11.012
Tracy, J. L., and Matsumoto, D. (2008). The spontaneous expression of pride and shame: evidence for biologically innate nonverbal displays. Proc. Natl. Acad. Sci. 105, 11655–11660. doi: 10.1073/pnas.0802686105
Tracy, J. L., and Robins, R. W. (2007). The prototypical pride expression: development of a nonverbal behavior coding system. Emotion 7, 789–801. doi: 10.1037/1528-3542.7.4.789
Tramacere, A., and Ferrari, P. F. (2016). Faces in the mirror, from the neuroscience of mimicry to the emergence of mentalizing. J. Anthropol. Sci. 94, 113–126. doi: 10.4436/JASS.94037
Trautmann, S. A., Fehr, T., and Herrmann, M. (2009). Emotions in motion: dynamic compared to static facial expressions of disgust and happiness reveal more widespread emotion-specific activations. Brain Res. 1284, 100–115. doi: 10.1016/j.brainres.2009.05.075
Troje, N. F. (2002). Decomposing biological motion: a framework for analysis and synthesis of human gait patterns. J. Vis. 2, 371–387. doi: 10.1167/2.5.2
Trotman, C.-A., Faraway, J. J., and Phillips, C. (2005). Visual and statistical modeling of facial movement in patients with cleft lip and palate. Cleft Palate Craniofac. J. 42, 245–254. doi: 10.1597/04-010.1
Valstar, M. F., Almaev, T., Girard, J. M., McKeown, G., Mehu, M., Yin, L., et al. (2015). “FERA 2015 - second facial expression recognition and analysis challenge.” in 2015 11th IEEE International Conference and Workshops on Automatic Face and Gesture Recognition (FG) (IEEE). pp. 1–8.
Valstar, M. F., and Pantic, M. (2010). “Induced disgust, happiness and surprise: an addition to the mmi facial expression database.” in Proc. 3rd Intern. Workshop on EMOTION (satellite of LREC): Corpora for Research on Emotion and Affect (Paris, France). p. 65.
Valstar, M. F., Sánchez-Lozano, E., Cohn, J. F., Jeni, L. A., Girard, J. M., Zhang, Z., et al. (2017). “FERA 2017 - addressing head pose in the third facial expression recognition and analysis challenge.” in 2017 12th IEEE International Conference on Automatic Face Gesture Recognition (FG 2017). pp. 839–847.
van der Schalk, J., Hawk, S. T., Fischer, A. H., and Doosje, B. (2011). Moving faces, looking places: validation of the Amsterdam dynamic facial expression set (ADFES). Emotion 11, 907–920. doi: 10.1037/a0023853
Vermeulen, N., Luminet, O., and Corneille, O. (2006). Alexithymia and the automatic processing of affective information: evidence from the affective priming paradigm. Cognit. Emot. 20, 64–91. doi: 10.1080/02699930500304654
Wallbott, H. G., and Scherer, K. R. (1986). Cues and channels in emotion recognition. J. Pers. Soc. Psychol. 51, 690–699. doi: 10.1037/0022-3514.51.4.690
Watson, R., and de Gelder, B. (2020). The representation and plasticity of body emotion expression. Psychol. Res. 84, 1400–1406. doi: 10.1007/s00426-018-1133-1
Wehrle, T., Kaiser, S., Schmidt, S., and Scherer, K. R. (2000). Studying the dynamics of emotional expression using synthesized facial muscle movements. J. Pers. Soc. Psychol. 78, 105–119. doi: 10.1037//0022-3514.78.1.105
West, J. T., Horning, S. M., Klebe, K. J., Foster, S. M., Cornwell, R. E., Perrett, D., et al. (2012). Age effects on emotion recognition in facial displays: from 20 to 89 years of age. Exp. Aging Res. 38, 146–168. doi: 10.1080/0361073X.2012.659997
Weyers, P., Mühlberger, A., Hefele, C., and Pauli, P. (2006). Electromyographic responses to static and dynamic avatar emotional facial expressions. Psychophysiology 43, 450–453. doi: 10.1111/j.1469-8986.2006.00451.x
Wieckowski, A. T., and White, S. W. (2017). Eye-gaze analysis of facial emotion recognition and expression in adolescents with ASD. J. Clin. Child Adolesc. Psychol. 46, 110–124. doi: 10.1080/15374416.2016.1204924
Wingenbach, T. S. H., Ashwin, C., and Brosnan, M. (2018). Sex differences in facial emotion recognition across varying expression intensity levels from videos. PLoS One 13:e0190634. doi: 10.1371/journal.pone.0190634
Yoshikawa, S., and Sato, W. (2006). Enhanced perceptual, emotional, and motor processing in response to dynamic facial expressions of emotion1. Jpn. Psychol. Res. 48, 213–222. doi: 10.1111/j.1468-5884.2006.00321.x
Zeng, Z., Pantic, M., Roisman, G. I., and Huang, T. S. (2009). A survey of affect recognition methods: audio, visual, and spontaneous expressions. IEEE Trans. Pattern Anal. Mach. Intell. 31, 39–58. doi: 10.1109/TPAMI.2008.52
Zhang, X., Yin, L., Cohn, J. F., Canavan, S., Reale, M., Horowitz, A., et al. (2014). BP4D-spontaneous: a high-resolution spontaneous 3D dynamic facial expression database. Image Vis. Comput. 32, 692–706. doi: 10.1016/j.imavis.2014.06.002
Keywords: expressed emotion, kinematics, face perception, laterality of motor control, happiness, fear, anger, dynamic patterns
Citation: Straulino E, Scarpazza C and Sartori L (2023) What is missing in the study of emotion expression? Front. Psychol. 14:1158136. doi: 10.3389/fpsyg.2023.1158136
Received: 03 February 2023; Accepted: 06 April 2023;
Published: 27 April 2023.
Edited by:
Mariella Pazzaglia, Sapienza University of Rome, ItalyReviewed by:
Shaun Halovic, The University of Sydney, AustraliaCopyright © 2023 Straulino, Scarpazza and Sartori. This is an open-access article distributed under the terms of the Creative Commons Attribution License (CC BY). The use, distribution or reproduction in other forums is permitted, provided the original author(s) and the copyright owner(s) are credited and that the original publication in this journal is cited, in accordance with accepted academic practice. No use, distribution or reproduction is permitted which does not comply with these terms.
*Correspondence: Elisa Straulino, ZWxpc2Euc3RyYXVsaW5vQHBoZC51bmlwZC5pdA==; Luisa Sartori, bHVpc2Euc2FydG9yaUB1bmlwZC5pdA==
Disclaimer: All claims expressed in this article are solely those of the authors and do not necessarily represent those of their affiliated organizations, or those of the publisher, the editors and the reviewers. Any product that may be evaluated in this article or claim that may be made by its manufacturer is not guaranteed or endorsed by the publisher.
Research integrity at Frontiers
Learn more about the work of our research integrity team to safeguard the quality of each article we publish.