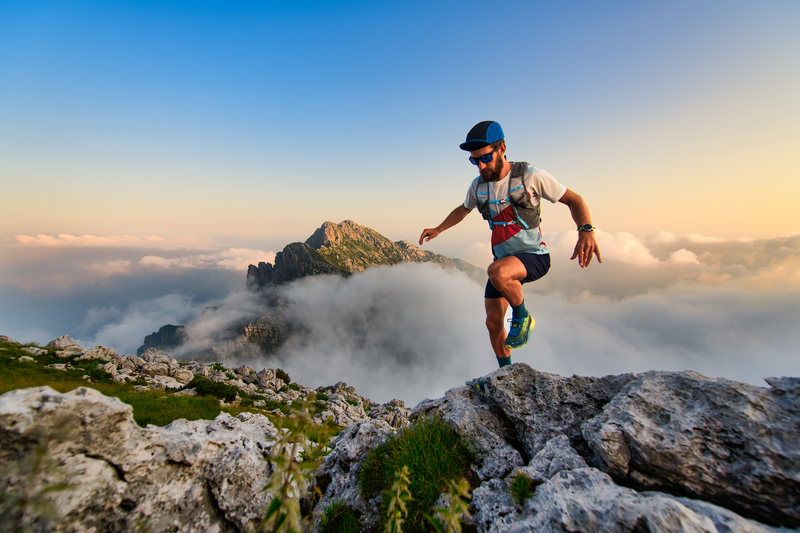
95% of researchers rate our articles as excellent or good
Learn more about the work of our research integrity team to safeguard the quality of each article we publish.
Find out more
ORIGINAL RESEARCH article
Front. Psychol. , 25 July 2023
Sec. Organizational Psychology
Volume 14 - 2023 | https://doi.org/10.3389/fpsyg.2023.1155302
Global e-commerce is growing rapidly during the COVID pandemic. Previous research on customers' online shopping decisions rarely considered social distancing. To investigate customers' continued intention toward online purchases while socially isolated, we propose a framework based on the UTAUT model. A survey of 330 valid samples was collected through an online survey among internet users during a period of social distancing in Indonesia. Hypotheses were validated using a structural equation modeling approach. The results showed that social contingency is the most influential factor on customers' intention to repurchase online under social restriction conditions, followed by customer perceived value and other significant factors. The findings contribute to providing a new understanding of customers' online repurchase intentions when they are in a contingency situation.
The growth of e-commerce has brought about significant modifications in personal online buying behavior. The advantage of e-commerce over traditional retail is that it eliminates geographical boundaries at any time (Mohanty et al., 2007). Since 2019, the COVID pandemic triggered tremendous growth in online sales as physical store visits were restricted, leading consumers to shift to online shopping (Dannenberg et al., 2020). Governments worldwide have implemented social distancing policies in response to the COVID outbreak to curb its spread. Social distancing measures aim to increase physical space between individuals to reduce the risk of virus transmission (Gross and Padilla, 2020). While this policy has restricted brick-and-mortar businesses, it has accelerated the growth of e-commerce (Fahrizal, 2020). This evolution has resulted in the maturation of online shopping as a retail channel and significant changes in people's purchasing habits (Le et al., 2022). Social distancing has emerged as the new norm that affects consumer purchasing behavior globally (Wang et al., 2021). According to Von Abrams (2021), total global online retail sales reached 17.9% in 2020 and rose to 20.3% in 2022.
Many governments implemented a social distancing policy which limited people's activities in public areas and facilities (Winanti et al., 2022). Consequently, workplaces have implemented work-from-home (WFH) measures to comply with social distancing policies, including in Indonesia. As Indonesian consumers practice social distancing to avoid the risk of COVID infection, online shopping has become increasingly popular in Indonesia (Adam et al., 2022). The rise of online and digital transactions (Prasetya et al., 2021) has led to a 25–30% increase in e-commerce in Indonesia during the pandemic (Pink, 2021).
Research conducted prior to the COVID pandemic using the Unified Theory of Acceptance and Use of Technology (UTAUT) revealed several factors that influenced customers' intention to shop online, such as performance expectations, effort expectations, social influence, and facilitating conditions (Venkatesh et al., 2003), as well as hedonic motivation, price value, and habit (Venkatesh et al., 2012). However, studies conducted during the early stages of the pandemic found that effort expectancy (Kadir and Ismail, 2022) and social influence (Erjavec and Manfreda, 2022) did not significantly impact customers' online shopping intentions. Recent research conducted during the pandemic suggests that major factors influencing customers' online purchasing decisions include performance expectancy (Zhao and Bacao, 2021), effort expectancy (Musyaffi, 2021), habit (Sheikh et al., 2017), and customer perceived value (Asti et al., 2021). These four UTAUT constructs were frequently identified in studies conducted both before and at the beginning of the COVID-19 outbreak.
The impact of social distancing on customers' online repurchase decisions has become an important concern, as highlighted by Maulidina et al. (2020). Given that social distancing measures affect the external environment rather than situational factors (Gehrt and Yan, 2004), the factors that influence customers' online repurchasing may differ from previous findings. However, recent research on consumer online repurchase has largely overlooked the context of social distancing. This study aims to address this gap by introducing a new variable, social contingency, as an external factor that can change customers' decisions to repurchase items online. As social distancing policy is crucial in mitigating the spread of epidemic diseases, it is important to gain a better understanding of the contextual factors that influence customers' online repurchasing decisions when socially isolated.
This study intends to investigate consumer online repurchase intentions during the pandemic, with a specific focus on developing countries, in addition to the UTAUT model. Prior research on online shopping has primarily concentrated on initial purchase intentions and paid less attention to frequent online shoppers or experienced customers. Therefore, this study aims to bridge this gap by applying the Expectation Confirmation Theory (ECT), a cognitive theory that explains post-purchase behavior, including repurchase intentions. ECT proposes that consumers repurchase intentions for a product or service are significantly influenced by their prior experience with it. By incorporating ECT, this study seeks to provide authentic factors that affect consumer repurchase intentions during the pandemic.
ECT emphasizes the importance of the confirmation construct. Hence, in this study, we integrate the UTAUT2 constructs (i.e., performance expectancy and effort expectancy) with the confirmation construct to create performance and effort confirmation. This integration attempts to enhance theoretical contributions to post-purchase consumer behavior in online shopping. The variables used in this study are performance confirmation, effort confirmation, customer perceived value, social contingency, and habit toward online shopping continuance intention. Although ECT provides a comprehensive theoretical framework, it remains unclear how social distancing impacts customers' online repurchase intentions. Additionally, theoretical models that consider social distancing and customer online repurchase intentions during the pandemic are scarce, particularly in e-commerce growing countries such as Indonesia. Therefore, this study seeks to explore the effects of social distancing on customers' online repurchase intentions during the pandemic. Two research questions are raised as below:
RQ1: What factors influence customers' continuous intentions toward online shopping during social distancing?
RQ2: What are the relationships among these factors that influence customers' intentions to continue online shopping?
This study holds significant importance as it introduces a model to measure customers' online shopping intentions under social contingencies like social distancing. Findings offer novel insights for online shopping research and practice in the post-COVID era. In addition to the core factors influencing customers' continuous online shopping intentions derived from UTAUT models, this study integrates a contextual variable called social contingency. The forthcoming sections present a literature review, hypothesis development, a methodology for validating the hypotheses, the results and implications, and the conclusion.
The Unified Theory of Acceptance and Use of Technology (UTAUT) was developed by Venkatesh et al. (2003). It is an integrated model based on socio-cognitive perspectives on users' initial acceptance of information technology. The original UTAUT model, also known as UTAUT1, proposes four constructs that affect users' intentions to use a specific technological product or service, namely performance expectancy, effort expectancy, social influence, and facilitating conditions. UTAUT1 has a 70% explained variance in behaviors, which outperforms other behavioral intention models (Venkatesh et al., 2003; Lu et al., 2009). Many studies have found that the four constructs of UTAUT1 can explain 71%−75% of the total variances influencing consumers' online purchase intentions (Adnan et al., 2018; Wijaya et al., 2022).
The original UTAUT model was later expanded with the inclusion of three additional constructs, namely hedonic motivation, price value, and habit, namely UTAUT2. This modification aimed to enhance its ability to predict consumer behavior. UTAUT2 demonstrated a significant improvement in its predictive power for consumption intentions compared to UTAUT1, with seven of the total UTAUT2 constructs contributing to an increase in the explained variance from 56% to 74% (Venkatesh et al., 2012). Table 1 provides the definitions of the core constructs in both UTAUT models.
Compared to other user behavioral models such as the theory of reasoned action (TRA), the theory of planned behavior (TPB), and the technology acceptance model (TAM), the UTAUT models show higher explanatory power in predicting customers' initial purchase intentions (Al-Qeisi et al., 2015; Taherdoost, 2018; Liu et al., 2022). This is because the UTAUT models integrate the above models into a unified framework (Venkatesh et al., 2003). Several studies have demonstrated that the UTAUT model is more effective in explaining consumer behavior related to technology acceptance compared to other models (Lin et al., 2019; Alghazi et al., 2021; Andrews et al., 2021; Bu et al., 2021), indicating its robustness. When compared to the previously mentioned theories, which explained only 17% to 42% of the variance in usage intention and behavior related to technology, the UTAUT model stands out with its high explanatory power of 70% in evaluating technology acceptance (Chao, 2019). Nevertheless, the efficacy of UTAUT constructs in predicting customer online repurchase intentions under social distancing remains unclear.
There is a significant difference when comparing consumer online shopping behaviors before and during the COVID-19 pandemic. Before COVID, more customers went shopping in person even though it was not preferred (Bridges and Fowler, 2022). In contrast, social distancing policies and consumers' worries about shopping in crowded places have increased interests in online shopping (Ellison et al., 2020). Consumers have been abruptly compelled to alter their priorities for online shopping because of COVID-19 (Pan et al., 2020). During the pandemic, customers found that online shopping was convenient, affordable, and helps them overcome the stress brought on by new sanitary standards and restrictions at retail shops (Guthrie et al., 2021). Besides predicting consumers' initial acceptance, the UTAUT models have often used to investigate customers' online repurchase intentions. Thus, this study investigates the major influential factors influencing customers' online repurchase intentions based on the UTAUT model before and during COVID.
Prior to the COVID-19 pandemic, studies in e-commerce have identified several factors from the UTAUT models that significantly impact customers' online repurchase intentions. Tam et al. (2020) found that habit, performance expectancy, and effort expectancy were critical drivers of customers' intentions to purchase online using mobile payment. Lee et al. (2019) and Alalwan (2020) identified habit and performance expectation as significant factors in customers' continued intentions to use online food ordering services. Zhou (2011) indicated that performance expectancy, social influence, and facilitating conditions impacted customers' continued intention to use mobile shopping and payment services. Similarly, Purohit et al. (2022) found that effort expectancy and facilitating conditions significantly influenced customers' online repurchasing decisions. Additionally, perceived value significantly affected customers' repurchasing intentions in m-commerce (Marinković et al., 2019) and mobile payment services (Oh and Kim, 2020) in a similar context.
During the COVID pandemic, studies have utilized the UTAUT models to examine customers' continuous shopping intentions with m-commerce. The significant influential factors identified during this period include performance expectancy and effort expectancy (Ramos, 2022), social influence (Zhao and Bacao, 2020; Muangmee et al., 2021), facilitating conditions and hedonic motivations (Shoheib and Abu-Shanab, 2022; Vinerean et al., 2022), habit (Nam and An, 2021), and perceived value (Nguyen et al., 2022). In contrast to findings prior to and during COVID, the most commonly used constructs in UTAUT models for predicting customers' online shopping intentions include performance expectancy, effort expectancy, habit, and perceived value. The constructs of UTAUT1 and UTAUT2 are listed in Table 2.
While performance expectancy and effort expectancy are significant predictors of customers' initial purchase intentions, they may not be as relevant to customers' post-purchase decisions as they are to customers who have experience shopping online.
The formation of customer expectations and confirmation occurs at different stages of the purchasing process. Prior to making a purchase or during the initial use of a product or service, a customer's belief about the potential utility to be gained is referred to as expectation (Oliver, 1980). According to the expectation-confirmation theory, consumers evaluate a product's performance not only based on their initial expectations but also on performance confirmation. Confirmation refers to the consumer's perspective of the alignment between their use expectations and actual current results (Bhattacherjee, 2001). In this study, we integrate “confirmation” with the UTAUT constructs by defining performance confirmation as a consumer's perception of the alignment between online shopping expectations and actual outcomes, and effort confirmation as a consumer's perception of the alignment between expected online transactions and actual operations.
Hsu and Lin (2015) found confirmation was positively correlated with customer perceived value in mobile app usage and significantly impact on users' intention to purchase mobile apps. The effect of confirmation on customers' perceived value has been shown to influence consumers' continuous intention to use accommodation applications significantly (Kim et al., 2019). Customers are more likely to increase their perceived value of a product/service when its performance and effort are confirmed. Confirmation of performance and effort of online shopping experiences can be attributed to customer perceived value. Based on the above discussion, we argue that performance confirmation and effort confirmation are appropriately used as antecedents of CPV, replacing performance expectancy and effort expectancy in the UTAUT1 model in the context of consumers' online repurchase intention. Hence, two hypotheses are postulated as follows:
H1: Performance confirmation has a positive effect on customer perceived value.
H2: Effort confirmation has a positive effect on customer perceived value.
Perceived value, according to Zeithaml (1988) and Dodds et al. (1991) definition, is the overall evaluation of a product's or service's usefulness by consumers, based on their perceptions of what they receive and what they give, reflecting the trade-off between perceived benefit and perceived risk. Chae et al. (2020) suggest that perceived value is the customer's assessment of the product's utility, as well as their perception of personal effort and benefit. Similarly, Miao et al. (2022) defines perceived value as consumers' opinions of goods and services. Perceived value plays a critical role in the exchange transaction (Dhingra et al., 2020), as it is the point at which customers perceive that the benefits, they receive outweigh the price they pay (Sinha and Verma, 2020). Known as customer perceived value (CPV), Kotler and Keller (2006) explain that it is the gap between a potential customer's evaluation of all the benefits and drawbacks of a product or service compared to other options. Customers gauge the worth of the benefits they received in relation to the money they paid and are willing to pay more if they feel they are getting better value for their money.
The UTAUT2 model defines price value as the balance between the perceived advantages of using an application and its monetary cost, according to Venkatesh et al. (2012). In contrast, customer perceived value (CPV), as explained by Aini et al. (2019), incorporates not only benefits and costs but also the evaluation process that customers undergo. Gan and Wang (2017) explored CPV by considering consumers' perceptions of utilitarian, hedonic, and social benefits, as well as perceived risk. Meanwhile, Lin et al. (2018) expanded CPV to include consumer perceived usefulness, perceived ease of use, and perceived risk, which encompass economic and privacy risks. Their findings indicate that these factors significantly influence customers' intentions to continue purchasing online.
This study defines CPV as a customer's perception of the benefits and trade-offs associated with obtaining more tailored products or services that provide greater value for money (Parasuraman and Grewal, 2000). Previous research has shown that CPV is a key driver of online repurchasing behavior or intentions, as noted by Ali and Bhasin (2019) and Ijaz and Rhee (2018). Moreover, perceived value is an essential precursor to repurchasing intention, according to Gligor and Bozkurt (2020). As such, it is vital to sustain CPV to foster long-term customer relationships and encourage repurchasing intention, as outlined by Kim et al. (2012). Perceived value is a major consideration in online purchasing decisions (Chiu et al., 2014). CPV can be increased if e-commerce stores offer personalized goods or services to fit customers' individual needs (Jiang et al., 2015). When a product or service bought from the Internet provides a higher perceived value, customers will be more likely to repurchase it (Guo and Li, 2022). Past studies showed when customers receive a good deal for money from online shopping, they will likely purchase it in the future.
Customer perceived value (CPV) encompasses the efforts, costs, and risks that customers undertake when deciding to make repeat purchases. Previous studies have demonstrated that CPV positively impacts an individual's intention to repurchase networking services (Kuo et al., 2009), participate in repeat online group buying (Hsu et al., 2015), or revisit online stores (Fang et al., 2016). Furthermore, customer perceived value is a significant factor influencing online shopping repurchase intention (Yang and Lee, 2010; Pobee, 2021), and it plays a crucial role in enhancing online shopping continuance during the COVID-19 pandemic (Prasetyo et al., 2021). As a result, customers are more likely to engage in repeat online purchases once they perceive greater value from their previous online shopping experiences.
If customers perceive a high level of customer perceived value (CPV) from an online retail store, they are more likely to engage in repeat purchases from that store (Irshad, 2016). CPV has a significant impact on online shoppers' repurchase intentions, as noted by Wu et al. (2014). Furthermore, the more a consumer perceives the value of an online shopping experience, the more likely they are to reinforce the habit of shopping online (Aarts and Dijksterhuis, 2000). As customers derive more value from technology, such as online shopping during the pandemic, their online shopping habits are likely to become more entrenched (Nguyen et al., 2022). In the context of online shopping, CPV has a positive correlation with online shopping habits, as found by Chiu et al. (2012). Customers who frequently engage in online purchases are more susceptible to the influence of perceived value (Cheng et al., 2017). Therefore, the relationship among CPV, habit, and online shopping continuance intention can be postulated as follows:
H3: Customer perceived value has a positive effect on online shopping continuance intention.
H4: Customer perceived value has a positive effect on habit.
According to Chou and Hsu (2016), consumers' purchasing decisions are influenced by their circumstances and cognitive elaboration. When the COVID pandemic occurred, consumers became worried about supply shortages and price increases, leading to panic buying of daily necessities (Yoon et al., 2018; Wang et al., 2020). Social distancing is a new external environmental factor that can be classified as a large-scale social contingent occurrence, unlike situational factors that are unique to a particular time and place and do not stem from knowledge of personal stimuli that affect current behavior systematically (Belk, 1975). Due to social distancing restrictions, people may find themselves in social isolation, which refers to being physically and chronologically alone during emergencies (Al Amin et al., 2022).
This study introduces the term “social contingency” as a variable to describe how unexpected social environments can influence individual behaviors. Social distancing measures, such as lockdowns, social isolation, and restrictions due to COVID pandemic, are a prime example of social contingency. Therefore, social contingency is defined as external environmental factors that can quickly change people's behaviors, such as panic buying, remote work, distance learning, and others.
Vinerean et al. (2022) have reported that consumers have significantly adopted and depended on online shopping directly from e-stores or online shops during COVID lockdowns and restrictions that were characterized by confinement. Consequently, there has been a surge in online shopping due to the government's social distancing policies that imposed lockdowns and strict protocols during the pandemic (Gruntkowski and Martinez, 2022). The implementation of social distancing measures to curb COVID-19 transmission has influenced consumers' decisions to shift to contactless channels such as cashless payments, leading to a significant increase in home delivery and online shopping (Pantano et al., 2020; Wang et al., 2021; Alaimo et al., 2022).
The pandemic-induced social distancing measures have not only affected consumer behavior but have also accelerated the growth of e-commerce and online shopping (Ivascu et al., 2022). Recent studies suggest that social distancing has not only led to an increase in consumers' intentions to purchase online (Itani and Hollebeek, 2020) but also strengthened their commitment to do so (Nguyen et al., 2020; Toska et al., 2022). The concept of social contingency can be applied to consumer behavior as well as other external situations, such as people's responses to financial crises, conflicts between nations, and extreme weather conditions.
Social distancing has compelled consumers to switch from physical brick-and-mortar stores to online stores for their shopping needs (Reddy, 2020). These shifts have not only intensified customers' online shopping habits (Mejía-Trejo, 2021) but have also increased their intentions to repurchase online (Gefen and David, 2000). As a result, social distancing has discouraged people from visiting physical stores while encouraging them to shop online. Therefore, relationships among social contingency, habit, and online shopping continuance intention can be hypothesized as follows:
H5: Social contingency has a positive effect on habit.
H6: Social contingency has a positive effect on online shopping continuance intention.
As per previous literature, a habit can be described as an automatic behavioral inclination that an individual acquires over time (Limayem and Hirt, 2003). Habits are automatic responses that are constrained by circumstances or stimuli (Aarts et al., 1998). A person's psychological tendency to repeat a behavior is referred to as a habit (Neal et al., 2012). Habits are actions that become natural, and individuals are typically unaware of these patterns. Individuals who have a habit of online shopping will continue to make purchases, whether necessary or not (Ghias et al., 2022). Therefore, in this study, a habit is defined as a customer's routine of purchasing goods or services from online stores. Habit has been used to describe the development of customers' perceptions and consumer behavior, such as continued online shopping, in both online and offline shopping (Honkanen et al., 2005).
According to Liao et al. (2006), when using a website becomes habitual, it can reinforce the intention to continue using the internet for activities such as online shopping. The COVID pandemic led to more consumers switching to the Internet, resulting in increased spending on online shopping (McKinsey, 2020). This, in turn, reinforces customers' online purchasing habits (Tao et al., 2022) and strengthens the influence of their purchase intention (Chen et al., 2022). Nguyen et al. (2022) discovered that habit plays a crucial role in driving customers' unconscious response to continue shopping online, and it is a potent influence on their intention to keep shopping online.
During the COVID pandemic, people have gradually relied on shopping online (Rui, 2022). Lavuri (2021) and Gefen (2003) observed this habit directly influences consumers' intentions to shop online. In the context of online shopping, customer habits have a positive correlation with repurchase intentions (Khalifa and Liu, 2007; Huang and Chen, 2017) because the creation of online shopping habits plays a crucial role in determining customers' repurchase intentions (Nazir et al., 2023). Shoppers who have established online shopping habits are more likely to repurchase and enhance their online repurchase intentions (Anderson and Sullivan, 1993; Lin and Lekhawipat, 2014). Consequently, it can be inferred that the frequency with which buyers engage in online shopping directly impacts their intention to online repurchasing (Mohamed et al., 2014). Therefore, the relationship between habit and online shopping continuance intention is hypothesized as follows:
H7: Habit has a positive effect on online shopping continuance intention.
Based on literature prior to and during the COVID pandemic, it was found that the four primary factors of UTAUT—performance expectancy, effort expectancy, perceived value, and habit—were frequently utilized in studies of customers' online repurchasing behavior. However, these factors may not align with customers' current considerations when it comes to online repurchasing. It is hypothesized that an individual's intention to repeat an action is primarily influenced by their previous actions and their inner assessment and reaction to external circumstances, which triggers a behavioral intention. Thus, habit is viewed as a sustainable driver for online repurchases when online shopping becomes a routine for customers. Customer perceived value (CPV) is used due to its consolidated nature and its influence on habit and online repurchase intention. In addition, a new external variable called social contingency is introduced to stimulate habit and intention to continue shopping online. Performance confirmation and effort confirmation are identified as antecedents of CPV, replacing performance expectancy and effort expectancy in UTAUT models. Therefore, based on the above assumptions, a research framework is presented in Figure 1.
The research framework includes six variables, with operational definitions for each variable listed in Table 3.
Measurements for each variable were chosen from previous studies and adjusted according to its operational definition, as shown in Table 4.
The questionnaire was developed based on the relevant literature and includes demographic questions and various measurements. All measurements were measured with five-point Likert scales, ranging from 1 = “strongly disagree” to 5 =“strongly agree”. Since the respondents in this study are Indonesian consumers, the questionnaire items were translated into Indonesian language with proofreading. Next, to reduce language bias, a pilot study of bilingual questionnaires (English and Indonesian) was distributed to 30 participants who could read English. Based on the results, few items were revised to achieve the same meanings, removing response bias (Forza, 2002).
The e-commerce market in Southeast Asia has grown rapidly in recent years. According to Jakartaglobe (2021), Indonesia had the highest e-commerce transaction volume in Southeast Asia in 2021. During the COVID epidemic in 2021, the Indonesian government implemented a social segregation policy known as “Large-Scale Social Restrictions” (Nursalam et al., 2021). Data for this survey was collected in Indonesia during the social distancing period. To collect responses, a questionnaire was created and distributed through various online channels such as email and social media platforms like WhatsApp, Facebook, and Line.
To determine the necessary sample size for this study, the Slovin formula was employed, which uses the total population, error tolerance (5%), and minimum sample size to calculate the number of valid samples needed. The formula used was n = N / (1 + N e2), where n is the number of samples, e is the error tolerance, and N is the total population. The calculated minimum sample size required for this study was 286 samples. A total of 330 valid samples were collected, which is above the minimum requirement, it can be concluded that this sample size is representative of the population. This study invited a community of online shoppers in Indonesia to participate, with 1,000 invitations sent out, resulting in a response rate of 33% after screening for online shopping experience data.
To minimize sample selection bias and increase the likelihood of randomization in samples, data were primarily collected from three social media channels (Vehovar et al., 2016). To further reduce sample selection bias during data collection, a statement was included at the beginning of the questionnaire assuring participants that their answers would be anonymous and used only for this research. To ensure the absence of potential respondent biases, all samples were randomly divided into two groups and validated using the Kolmogorov-Smirnov (K-S) test (Martins et al., 2014). The K-S test results revealed no statistical difference, indicating no response bias.
To analyze the data, this study used partial least squares structural equation modeling (PLS-SEM) approach, which is known to have higher item loading, average variance extracted (AVE) and composite reliability (CR) compared to the covariance based-structural equation modeling (CB-SEM) approach (Dash and Paul, 2021). The PLS-SEM approach is also suitable for explanatory analysis, which is in line with the purpose of this study (Hair et al., 2017). The researchers used the SmartPLS 3.0 software package with PLS-SEM method to estimate the mean, significance of item factor loadings, and path coefficients for the hypothesized model, to test the feasibility of measurements and hypotheses. To ensure the validity of the results, the researchers randomly divided all samples into two groups and validated them using the Kolmogorov-Smirnov (K-S) test, which indicated no response bias.
Table 5 illustrates that the majority of young-Millennial respondents fell within the age range of 21 to 40. Additionally, nearly 90% of the participants made at least five online purchases on a monthly basis. Furthermore, over 87% of respondents reported monthly transactions totaling more than 300,000 Indonesian Rupiah (IDR).
Table 6 presents the descriptive statistics of the measurements. The means range from 3.648 (SD = 0.761) to 4.452 (SD = 0.713) for all measurements. Among the 23 indicators, Online Shopping Continuance Intention4 (OSCI4) had the highest average value of 4.452 (SD = 0.713), while Social Contingency3 (SC3) had the lowest average value of 3.648 (SD= 0.761). In the variable of online shopping continuance intention, OSCI1 had the lowest average value of 4.073 but the highest standard deviation value of 0.787. OSCI2 had a mean value of 4.394 and a standard deviation of 0.689, while OSCI4 had a mean value of 4.452 and a standard deviation of 0.713.
To ensure the reliability and validity of the variables, a measurement model was employed in this study. The measurement model test consisted of two parts: convergent validity and discriminant validity. Convergent validity was evaluated through cross-factor loading and average variance extracted (AVE). A factor loading value >0.7 and an AVE value >0.5 indicate good convergent validity for an item (Hair et al., 2014). Highly connected and positively correlated indicators within a construct with good factor loading values also indicate convergent validity (Ringle et al., 2015).
According to the results of the cross-factor loading test, two items (HA3 and EC4) had scores that were below 0.5. After removing these two items, the remaining items have cross-factor loadings above 0.7, which suggests that the construct validity is acceptable (Fornell and Larcker, 1981), as demonstrated in Table 7.
Table 8 indicates that all of the items have an AVE value that exceeds 0.5, which is a sign of acceptable convergent validity. Furthermore, the square root of the AVE value for each construct is greater than the correlations with other constructs, indicating good discriminant validity, as suggested by Hair et al. (2006).
To test reliability, Cronbach's Alpha and composite reliability were used. Table 9 displays the composite reliability range, which was between 0.845 and 0.912, as well as the Cronbach's Alpha range, which was between 0.724 and 0.855 for the various constructs. As per the guidelines provided by Hair et al. (2012), all items had a Cronbach's Alpha value >0.7, indicating that all constructs were reliable.
The goodness-of-fit (GOF) test determines whether a model adequately describes a study's empirical data (Sheppard and Meitner, 2005). To determine the GOF of a model, Tenenhaus et al. (2005) recommend using the geometric mean value of the AVE values and the average R2 value(s), as stated in Equation (1).
The results showed GOF was 0.594, with an average AVE of 0.671 and an average R2 of 0.353. It indicated that the empirical data used in this study was suitable for measurements (Baumgartner and Homburg, 1996). The standardized root means square residual (SRMR) was used to assess the overall goodness of model fit for PLS (Hair et al., 2017). The difference between the observed correlation and the correlation matrix implied by the model is known as the SRMR. A good fit is defined as an SRMR value < 0.1 (Hu and Bentler, 1998). Results showed SRMR value was 0.098, indicating that the measurement model fits the data well.
The Normed Fit Index (NFI) was used as an indicator of global model fit in surveys with small sample sizes. NFI has a value between 0 and 1, with a higher NFI indicating a better fit (Bentler and Bonett, 1980). The chi-square goodness of fit test is estimated when comparing a sample to a population with known parameters on a specific variable. Chi-square statistics with values ranging from 0 to infinity are commonly used as goodness-of-fit indices (Wheaton, 1987). Results showed NFI = 0.709 and Chi-Square = 1293.762 indicating that the model had an acceptable fit level.
The exact model fit criteria are formed by combining two model fit indices, namely the squared Euclidean distance (d_ULS) and the geodesic distance (d_G) (Dijkstra and Henseler, 2015). Results showed that d_ULS (2.239) and d_G (0.712) had values lower than the 95% bootstrapped quantile, indicating a good fit between the measurement model and data.
This study estimated the structural model using path coefficients, which indicate the strength of the relationships between independent and dependent variables (Sarstedt et al., 2017). A bootstrapping procedure was used to determine the significance of these coefficients. The adjusted R2 value, which adjusts for the number of predictors in the model (Miles, 2014), was also calculated. The results, as shown in Figure 2, revealed that all paths had positive coefficients that were statistically significant. The highest beta-value was for habit (β = 0.328***), followed by social contingency (β = 0.259***) and customer perceived value (β = 0.217**). Effort confirmation (β = 0.515***) had a greater effect on customer perceived value than performance confirmation (β= 0.404***). Overall, the validated model had a moderate explanatory power on consumers' online repurchase intentions, with an adjusted R2 value of 0.527. The results of the structural model supported all hypotheses, as shown in Table 10.
This study calculated effect size (ES) coefficients for each path in the validated model to compare the total effect of different variables on customers' online repurchase intentions. Table 11 presents the total ES for each path, which includes performance confirmation (ES = 0.087), effort confirmation (ES = 0.111), customer perceived value (ES = 0.330), social contingency (ES = 0.414), and habit (ES = 0.328). According to Cohen (1988), an ES of 0.35, 0.15, and 0.02 indicates a strong, moderate, and weak effect, respectively. The results indicated that social contingency had a strong total ES on online shopping continuation intention, followed by customer perceived value and habit, both of which had a moderate impact on customers' online repurchase intentions. Two external variables, performance confirmation and effort confirmation, had a weak indirect ES on online shopping retention intention.
This study aimed to explore how contextual factors related to social distancing influence customers' online repurchase intentions. Empirical data was collected and analyzed to validate the research model, and the results support the hypotheses. Specifically, this study found that antecedents of customer perceived value, such as performance confirmation and effort confirmation, had a significant impact on customer perceived value. This study replaced the constructs of performance expectancy and effort expectancy from the UTAUT model with performance confirmation and effort confirmation based on ECT perspective, and this approach was found to be valid. This study showed that customers' perception of the congruence between their expectations and actual outcomes significantly influences their perceived value. Higher levels of performance confirmation and effort confirmation lead to higher perceived value.
This study's second finding was that customer perceived value had a significant impact on both habit and online shopping continuance intention. The result supported prior research (Wu et al., 2014; Pham et al., 2018; Guo and Li, 2022; Miao et al., 2022), indicating that customers are more likely to repurchase online if they received greater perceived value during their prior internet shopping experience. Moreover, this study found that customer perceived value significantly affects habit, which is consistent with prior studies (Chiu et al., 2012; Cheng et al., 2017; Nguyen et al., 2022). This result suggests that higher customer perceived value leads to more frequent habit repetition. Thus, improving customer perceived value is crucial to encourage online shopping habits and maintain customers' continued intention to shop online.
As a new factor in this study, social contingency refers to the influence of social distancing on consumer behavior, had a significant impact on habit and online shopping continuance intention. Interestingly, social contingency had the greatest effect on consumers' intention to continue online shopping compared to other variables in the model. This suggests that social contingency was a crucial aspect of online shopping during the COVID pandemic. These findings are consistent with previous research indicating that the pandemic has forced consumers to adapt their shopping habits to online channels due to social contingency (Briedis et al., 2020; Alhaimer, 2022; Kao and André L'Huillier, 2022). Therefore, it is essential for e-retailers on e-commerce platforms to consider social contingency as a contextual factor that plays a vital role in online shopping behavior, particularly during a pandemic.
Social contingency is the most significant factor influencing customers' intention to continue online shopping during the pandemic. This could be attributed to the anxiety triggered by the COVID situation, and the implementation of social distancing measures limiting people's ability to shop in physical stores. As a result, many Indonesian respondents in this study increased their online shopping, particularly for health products, due to fear of a shortage of goods resulting from panic buying. This finding builds upon previous research on Indonesian consumers (Iriani et al., 2021; Elisa et al., 2022), which demonstrated that social restrictions during the COVID pandemic have led to an increase in online sales of health products in Indonesia.
This study also found that habit has a significant impact on customers' intention to continue online shopping. This result is consistent with previous research (Khalifa and Liu, 2007; Urueña-López et al., 2012; Huang and Chen, 2017). Additionally, this research demonstrated that habit has the greatest direct influence on online shopping continuance intention compared to customer perceived value and social contingency. This finding suggests that habit is a more robust predictor of technology continuance intention, which is supported by prior research (Zhang et al., 2015; Mirkovski et al., 2018; Nguyen et al., 2022). The strong impact of habit on online shopping continuance intention could be due to consumers increasing their use of technology to shop online during unpredictable situations such as the pandemic.
This study's results suggest that the habit of online shopping among Indonesian consumers is likely to continue even after the pandemic ends. This is supported by a survey conducted by SIRCLO in 2021 (SIRCLO and Ravenry, 2021), which found that more than 40% of new Indonesian online shoppers intend to continue shopping online, indicating that online shopping has become a permanent habit during the pandemic. This finding is consistent with the current situation in Indonesia, which is one of the largest e-commerce markets globally, with an estimated increase in the number of e-commerce shoppers to 221 million IDR by 2025 (Statista, 2020).
This study makes several distinct theoretical contributions to the existing academic literature on consumer behavior and online repurchase intention compared to previous research. Firstly, it proposes a model that considers customer perceived value (CPV) and habit as factors influencing continuous online shopping intention in the context of social distancing. Few studies have examined post-purchase consumer decisions using the UTAUT model under social distancing conditions. As such, this study establishes an influencing factors model of customers' continued online shopping intention under social distancing conditions, and modifies the UTAUT2 model based on the ECT construct to provide a more comprehensive explanation of post-purchase online consumer behavior.
Secondly, as discussed in the literature review, this study justifies the use of CPV rather than the price value construct in the UTAUT2 model. The rationale for this is that CPV has a more significant impact on repurchase intention than price value. This suggests that customers are more likely to make repeat purchases online once they have confirmed the higher value of their previous online shopping experience. Numerous studies have demonstrated the significant direct effects of CPV on online repurchase intention (Wu et al., 2014; Fang et al., 2016; Ali and Bhasin, 2019; Guo and Li, 2022; Nguyen et al., 2022). However, very few studies have explored the antecedents of CPV derived from the perspectives of ECT and UTAUT2 constructs, which significantly affect online repurchase intentions. Therefore, by drawing on theories from both the ECT and UTAUT models, this study expands on both theories by proposing a more comprehensive model.
In conclusion, this study contributes to the existing literature on online repurchase intention in three main ways that differ from related studies. Firstly, it proposes a model based on CPV and habit for continuous online shopping intention under the context of social distancing, which is an area that has not been explored in previous studies. Secondly, it argues for the use of CPV instead of price value in the UTAUT2 model, as CPV has a greater impact on repurchase intention. Finally, this study introduces social contingency as a new variable that affects online repurchase decisions and suggests that it can be applied to other social occurrences in future research. By addressing these gaps in the literature, this study provides a foundation on post-purchase behavior in online consumer decision-making.
To retain customers in the post-COVID era, online retailers should take note of the practical implications from this study. The results suggest that improving performance confirmation and effort confirmation can significantly affect customer perceived value. This can be achieved by enhancing the speed of online order delivery, simplifying the ordering process, streamlining payment processing, and improving overall convenience and practicability of the online shopping experience. Furthermore, providing excellent customer service can help online retailers better understand their customers and strengthen their perceived value. These measures can increase customer satisfaction and lead to higher repurchase intentions, ultimately benefiting online retailers in the long run.
The results of this study indicate that customer perceived value (CPV) plays a crucial role in online shopping habits and repurchase intentions in the context of e-commerce. The findings suggest that customers are more likely to make repeat purchases online when they perceive higher value for money and desired benefits. Therefore, online retailers should focus on providing good value for money and offer various promotions and discounts to encourage customers to develop online shopping habits and make repeat purchases in the future.
Social contingency was found to have the largest effect on consumers' online shopping continuance intention compared to all other variables in this study. Hence, online retailers should prioritize ensuring the availability of necessities such as medicine, masks, and other health products during social distancing in the pandemic. Online retailer managers could encourage the regular use of their platforms by offering incentives such as promotional items like masks, hand antiseptic, or disinfectant. They could also offer free protective suits for COVID to reinforce their brand image and increase customer loyalty.
This study revealed that habit is a significant factor in online shopping continuance intention. It was found that habit has a stronger direct effect on customers' online shopping intentions compared to perceived value and social contingency. As a result, online retailers can implement loyalty programs that incentivize repeat purchases and customer retention. This can include reward points, discounts, and exclusive offers for loyal customers, which can help reshape their online shopping habits and encourage repurchase intention. Additionally, online retailers should focus on providing a seamless and personalized shopping experience to enhance customer satisfaction and loyalty. Online retailers can use endorsers or influencers to promote their products through live-stream shopping to attract, interact and maintain engagement with customers to stimulate customers to make repeat online purchases.
To enhance the model's applicability, several limitations need addressing in future research. Firstly, it is necessary to validate the generalizability of the findings to other countries outside Indonesia. Future studies should investigate the proposed model's applicability to other Asian countries. Secondly, this study's cross-sectional data sampling during the COVID pandemic makes it challenging to understand the effects over time. Thus, future research should incorporate longitudinal data to examine the differences in the pre-COVID, COVID, and post-COVID periods. Finally, to gain a better understanding of online repurchase behaviors, future studies may consider including moderating variables.
The original contributions presented in the study are included in the article/supplementary material, further inquiries can be directed to the corresponding author.
WW and GW conceptualized the study. WW contributed to the study design, reviewed, edited, and managed the manuscript. GW contributed to the study design, collected and analyzed the data, and wrote the original draft. DR reviewed and edited the manuscript.
The authors declare that the research was conducted in the absence of any commercial or financial relationships that could be construed as a potential conflict of interest.
All claims expressed in this article are solely those of the authors and do not necessarily represent those of their affiliated organizations, or those of the publisher, the editors and the reviewers. Any product that may be evaluated in this article, or claim that may be made by its manufacturer, is not guaranteed or endorsed by the publisher.
Aarts, H., and Dijksterhuis, A. (2000). Habits as knowledge structures: automaticity in goal-directed behavior. J. Pers. Soc. Psychol., 78, 53–63. doi: 10.1037/0022-3514.78.1.53
Aarts, H., Verplanken, B., and Van Knippenberg, A. (1998). Predicting behavior from actions in the past: repeated decision making or a matter of habit? J. Appl. Soc. Psychol.28, 1355–1374. doi: 10.1111/J.1559-1816.1998.TB01681.X
Adam, M., Ibrahim, M., Idris, S., Saputra, J., and Putra, T. R. I. (2022). An investigation of e-marketing and its effect on the consumer buying decision during Covid-19 pandemic in Aceh Province, Indonesia: a mediating role of perceived risk. Int. J. Data Network. 6, 115–126. doi: 10.5267/J.IJDNS.2021.9.016
Adnan, N., Md Nordin, S., bin Bahruddin, M. A., and Ali, M. (2018). How trust can drive forward the user acceptance to the technology? In-vehicle technology for autonomous vehicle. Transport. Res. Part A Pol. Pract. 118, 819–836. doi: 10.1016/J.TRA.2018.10.019
Aini, Q., Rahardja, U., and Hariguna, T. (2019). The antecedent of perceived value to determine of student continuance intention and student participate adoption of ilearning. Proced. Comp. Sci. 161, 242–249. doi: 10.1016/j.procs.2019.11.120
Al Amin, M., Arefin, M. S., Alam, M. S., and Rasul, T. F. (2022). Understanding the predictors of rural customers' continuance intention toward mobile banking services applications during the COVID-19 pandemic. J. Global Mark. 35, 324–347. doi: 10.1080/08911762.2021.2018750
Alaimo, L. S., Fiore, M., and Galati, A. (2022). Measuring consumers' level of satisfaction for online food shopping during COVID-19 in Italy using POSETs. Socio-Econ. Plann. Sci. 82, 101064. doi: 10.1016/j.seps.2021.101064
Alalwan, A. A. (2020). Mobile food ordering apps: an empirical study of the factors affecting customer e-satisfaction and continued intention to reuse. Int. J. Inform. Manag. 50, 28–44. doi: 10.1016/j.ijinfomgt.2019.04.008
Alghazi, S. S., Kamsin, A., Almaiah, M. A., Wong, S. Y., and Shuib, L. (2021). For sustainable application of mobile learning: an extended utaut model to examine the effect of technical factors on the usage of mobile devices as a learning tool. Sustainability, 13, 1–23. doi: 10.3390/su13041856
Alhaimer, R. (2022). Fluctuating attitudes and behaviors of customers toward online shopping in times of emergency: the case of Kuwait during the COVID-19 pandemic. J. Internet Comm. 21, 26–50. doi: 10.1080/15332861.2021.1882758
Ali, A., and Bhasin, J. (2019). Understanding customer repurchase intention in e-commerce: role of perceived price, delivery quality, and perceived value. Jindal J. Bus. Res. 8, 142–157. doi: 10.1177/2278682119850275
Al-Qeisi, K., Dennis, C., Hegazy, A., and Abbad, M. (2015). How viable is the UTAUT model in a non-Western context? Int. Bus. Res. 8, 204–219. doi: 10.5539/ibr.v8n2p204
Anderson, E. W., and Sullivan, M. W. (1993). The antecedents and consequences of customer satisfaction for firms. Mark. Sci. 12, 125–143. doi: 10.1287/mksc.12.2.125
Andrews, J. E., Ward, H., and Yoon, J. W. (2021). UTAUT as a model for understanding intention to adopt AI and related technologies among librarians. J. Acad. Librarian. 47, 102437. doi: 10.1016/J.ACALIB.2021.102437
Asti, W. P., Handayani, P. W., and Azzahro, F. (2021). Influence of trust, perceived value, and attitude on customers' repurchase intention for e-grocery. J. Food Products Marketing. doi: 10.1080/10454446.2021.1922325
Baumgartner, H., and Homburg, C. (1996). Applications of structural equation modeling in marketing and consumer research: a review. Int. J. Res. Mark. 13, 139–161.
Belk, R. W. (1975). Situational variables and consumer behavior. J. Cons. Res. 2, 157–164. doi: 10.1086/208627
Bentler, P. M., and Bonett, D. G. (1980). Significance tests and goodness of fit in the analysis of covariance structures. Psychol. Bullet. 88, 588–606. doi: 10.1037/0033-2909.88.3.588
Bhattacherjee, A. (2001). Understanding information systems continuance: expectation-confirmation mode. MIS Quart. 25, 351–370. doi: 10.2307/3250921
Bridges, E., and Fowler, K. (2022). Grocery shopping before, during and after the Pandemic: a qualitative study. Family Cons. Sci. Res. J. 51, 35–50. doi: 10.1111/FCSR.12453
Briedis, H., Kronschnabl, A., Rodriguez, A., and Ungerman, K. (2020). Adapting to the next normal in retail: The customer experience imperative. McKinsey. Available online at: https://www.mckinsey.com/industries/retail/our-insights/adapting-to-the-next-normal-in-retail-the-customer-experience-imperative (accessed January 29, 2022).
Bu, F., Wang, N., Jiang, B., and Jiang, Q. (2021). Motivating information system engineers' acceptance of Privacy by Design in China: An extended UTAUT model. Int. J. Inform. Manag. 60, 102358. doi: 10.1016/J.IJINFOMGT.2021.102358
Chae, H., Kim, S., Lee, J., and Park, K. (2020). Impact of product characteristics of limited edition shoes on perceived value, brand trust, and purchase intention; focused on the scarcity message frequency. J. Bus. Res. 120, 398–406. doi: 10.1016/j.jbusres.2019.11.040
Chao, C. M. (2019). Factors determining the behavioral intention to use mobile learning: an application and extension of the UTAUT model. Front. Psychol. 10, 1–14. doi: 10.3389/fpsyg.2019.01652
Chen, H., Chen, H., and Tian, X. (2022). The dual-process model of product information and habit in influencing consumers' purchase intention: the role of live streaming features. Elect. Comm. Res. Appl. 53, 101150. doi: 10.1016/J.ELERAP.2022.101150
Cheng, S., Lee, K.-R., and Lee, S.-J. (2017). Study on Chinese repurchase intention of group-buying social commerce:the moderating role of shopping habit. J. Korea Converg. Soc. 8, 169–181. doi: 10.15207/jkcs.2017.8.2.169
Chiu, C. M., Hsu, M. H., Lai, H., and Chang, C. M. (2012). Re-examining the influence of trust on online repeat purchase intention: the moderating role of habit and its antecedents. Dec. Supp. Sys. 53, 835–845. doi: 10.1016/j.dss.2012.05.021
Chiu, C. M., Wang, E. T. G., Fang, Y. H., and Huang, H. Y. (2014). Understanding customers' repeat purchase intentions in B2C e-commerce. Inform. Sys. J. 24, 85–114. doi: 10.1111/J.1365-2575.2012.00407
Chou, S. W., and Hsu, C. S. (2016). Understanding online repurchase intention: social exchange theory and shopping habit. Inform. Sys. E-Bus. Manag. 14, 19–45. doi: 10.1007/s10257-015-0272-9
Cohen, J. (1988). Cohen, J. (1988). Statistical Power Analysis for the Behavioral Sciences (2nd ed.). Hillsdale, NJ: Lawrence Erlbaum Associates, Publishers.
Dannenberg, P., Fuchs, M., Riedler, T., and Wiedemann, C. (2020). Digital transition by COVID-19 pandemic? The German food online retail. Tijdschrift Voor Econ. En Soc. Geog. 111, 543–560. doi: 10.1111/tesg.12453
Dash, G., and Paul, J. (2021). CB-SEM vs PLS-SEM methods for research in social sciences and technology forecasting. Technol. Forecast. Soc. Change, 173, 121092. doi: 10.1016/j.techfore.2021.121092
Dhingra, S., Gupta, S., and Bhatt, R. (2020). A study of relationship among service quality of E-commerce websites, customer satisfaction, and purchase intention. Int. J. E-Business Res. 16, 42–59. doi: 10.4018/IJEBR.2020070103
Dijkstra, T. K., and Henseler, J. (2015). Consistent and asymptotically normal PLS estimators for linear structural equations. Computat. Stat. Data Anal. 81, 10–23. doi: 10.1016/j.csda.2014.07.008
Dodds, W. B., Monroe, K. B., and Grewal, D. (1991). Effects of price, brand, and store information on buyers' product evaluations. J. Marketing Research, 28, 307. doi: 10.2307/3172866
Elisa, H. P., Fakhri, M., and Pradana, M. (2022). The moderating effect of social media use in impulsive buying of personal protective equipments during the COVID-19 pandemic. Cogent Soc. Sci. 8, 94. doi: 10.1080/23311886.2022.2062094
Ellison, B., McFadden, B., Rickard, B. J., and Wilson, N. L. W. (2020). Examining food purchase behavior and food values during the COVID-19 pandemic. Appl. Econ. Perspect. Policy 3, 3118. doi: 10.1002/aepp.13118
Erjavec, J., and Manfreda, A. (2022). Online shopping adoption during COVID-19 and social isolation: extending the UTAUT model with herd behavior. J. Retail. Consumer Serv. 65, 2867. doi: 10.1016/j.jretconser.2021.102867
Fahrizal, R. (2020). Penggunaan Aplikasi Belanja dan Produktivitas Meningkat Karena Social Distancing- Info Komputer. Available online at: https://infokomputer.grid.id/read/122103021/penggunaan-aplikasi-belanja-dan-produktivitas-meningkat-karena-social-distancing?page=all (accessed October 8, 2021).
Fang, J., Wen, C., George, B., and Prybutok, V. R. (2016). Consumer heterogeneity, perceived value, and repurchase decision-making in online shopping: the role of gender, age, and shopping motives. J. Elect. Comm. Res. 17, 116–131.
Fornell, C., and Larcker, D. F. (1981). Evaluating structural equation models with unobservable variables and measurement error. J. Mark. Res. 18, 39.
Forza, C. (2002). Survey research in operations management: a process-based perspective. Int. J. Operat. Prod. Manag. 22, 152–194. doi: 10.1108/01443570210414310
Gan, C., and Wang, W. (2017). The influence of perceived value on purchase intention in social commerce context. Int. Res. 27, 772–785. doi: 10.1108/IntR-06-2016-0164
Gefen, D. (2003). TAM or just plain habit: a look at experienced online shoppers. J. End User Computing 15, 1–13.
Gehrt, K. C., and Yan, R. N. (2004). Situational, consumer, and retailer factors affecting Internet, catalog, and store shopping. Int. J. Retail Distribut. Manag. 32, 5–18. doi: 10.1108/09590550410515515
Ghias, S., Ahmed, K. A., and Khan, A. A. (2022). Impact of e-retailing ethics on consumer repurchase intention. J. Bus. Soc. Rev. Emerg. Econ. 8, 251–264. doi: 10.26710/jbsee.v8i1.2194
Gligor, D., and Bozkurt, S. (2020). FsQCA versus regression: the context of customer engagement. J. Retail. Consum. Serv. 52, 101929. doi: 10.1016/J.JRETCONSER.2019.101929
Gross, J., and Padilla, M. (2020). Coronavirus Glossary: Flattening the Curve, Pandemic, Covid-19 and More- The New York Times. Available online at: https://www.nytimes.com/2020/03/18/us/coronavirus-terms-glossary.html (accessed February 23, 2023).
Gruntkowski, L. M., and Martinez, L. F. (2022). Online grocery shopping in Germany: assessing the impact of COVID-19. J. Theoret. Appl. Elect. Comm. Res. 17, 984–1002. doi: 10.3390/jtaer17030050
Guo, J., and Li, L. (2022). Exploring the relationship between social commerce features and consumers' repurchase intentions: the mediating role of perceived value. Front. Psychol. 12, 5056. doi: 10.3389/fpsyg.2021.775056
Guthrie, C., Fosso-Wamba, S., and Arnaud, J. B. (2021). Online consumer resilience during a pandemic: an exploratory study of e-commerce behavior before, during and after a COVID-19 lockdown. J. Retail. Consum. Serv. 61, 102570. doi: 10.1016/j.jretconser.2021.102570
Hair, J. F., Black, W. C., Babin, B. J., Anderson, R. E., and Tatham, R. L. (2006). Multivariate Data Analysis. Vol. 6. Upper Saddle River: Pearson Prentice Hall.
Hair, J. F., Hult, G. T. M., Ringle, C. M., and Sarstedt, M. (2017). A Primer on Partial Least Squares Structural Equation Modeling (PLS-SEM), 2nd Edn. Thousand Oaks, CA: Sage Publications Inc.
Hair, J. F., Sarstedt, M., Hopkins, L., and Kuppelwieser, V. G. (2014). Partial least squares structural equation modeling (PLS-SEM): an emerging tool in business research. Eur. Bus. Rev. 26, 106–121. doi: 10.1108/EBR-10-2013-0128
Hair, J. F., Sarstedt, M., Ringle, C. M., and Mena, J. A. (2012). An assessment of the use of partial least squares structural equation modeling in marketing research. J. the Acad. Market. Sci. 40, 414–433. doi: 10.1007/s11747-011-0261-6
Honkanen, P., Olsen, S. O., and Verplanken, B. (2005). Intention to consume seafood—The importance of habit. Appetite, 45, 161–168. doi: 10.1016/j.appet.2005.04.005
Hsu, C. L., and Lin, J. C. C. (2015). What drives purchase intention for paid mobile apps? An expectation confirmation model with perceived value. Elect. Comm. Res. Appl. 14, 46–57. doi: 10.1016/j.elerap.2014.11.003
Hsu, M. H., Chang, C. M., and Chuang, L. W. (2015). Understanding the determinants of online repeat purchase intention and moderating role of habit: the case of online group-buying in Taiwan. Int. J. Inf. Manage. 35, 45–56.
Hu, L. T., and Bentler, P. M. (1998). Fit indices in covariance structure modeling: sensitivity to underparameterized model misspecification. Psychol. Methods 3, 424–453.
Huang, M., and Chen, T. (2017). Tourism website customers' Repurchase intention: information system success model. DEStech Transact. Engin. Technol. Res. 426–430. doi: 10.12783/dtetr/amma2017/13399
Ijaz, M. F., and Rhee, J. (2018). Constituents and consequences of online-shopping in sustainable e-business: an experimental study of online-shopping malls. Sustainability 10, 3756. doi: 10.3390/su10103756
Iriani, S. S., Nuswantara, D. A., Kartika, A. D., and Puwohandoko, P. (2021). the impact of government regulations on consumers behaviour during the COVID-19 pandemic: a case study in Indonesia. J. Asian Finance Econ. Bus. 8, 939–948. doi: 10.13106/jafeb.2021.vol8.no4.0939
Irshad, S. (2016). Mediating role of customer value between innovative self-service technology (ISST) factors and online repurchase intention. J. Internet Bank. Comm. 21, 2.
Itani, O. S., and Hollebeek, L. D. (2020). Consumers' health-locus-of-control and social distancing in pandemic-based e-tailing services. J. Services Marketing 35, 1073–1091. doi: 10.1108/JSM-10-2020-0410
Ivascu, L., Domil, A. E., Artene, A. E., Bogdan, O., Burcă, V., and Pavel, C. (2022). Psychological and behavior changes of consumer preferences during COVID-19 pandemic times: an application of GLM regression model. Front. Psychol. 13, 1–18. doi: 10.3389/fpsyg.2022.879368
Jakartaglobe. (2021). E-Commerce Transaction Value to Hit $27.5b in 2021. Available online at: https://jakartaglobe.id/business/ecommerce-transaction-value-to-hit-275b-in-2021 (accessed January 26, 2022).
Jiang, L., Jun, M., and Yang, Z. (2015). Customer-perceived value and loyalty: how do key service quality dimensions matter in the context of B2C e-commerce? Serv. Bus. 10, 301–317. doi: 10.1007/s11628-015-0269-y
Kadir R. D. and Ismail J. (2022). Millennials adpot online delivery: analysis using UTAUT2 model during pandemic COVID-19. J. Princ. Manag. Bus. 1, 18–26. doi: 10.55657/jpmb.v1i01.54
Kao, W. K., and André L'Huillier, E. (2022). The moderating role of social distancing in mobile commerce adoption. Elect. Comm. Res. Appl. 52. doi: 10.1016/j.elerap.2021.101116
Khalifa, M., and Liu, V. (2007). Online consumer retention: contingent effects of online shopping habit and online shopping experience. Eur. J. Inform. Syst. 16, 780–792. doi: 10.1057/PALGRAVE.EJIS.3000711
Kim, C., Galliers, R. D., Shin, N., Ryoo, J. H., and Kim, J. (2012). Factors influencing internet shopping value and customer repurchase intention. Electron. Commer. Res. Appl. 11, 374–387. doi: 10.1016/j.elerap.2012.04.002
Kim, S. H., Bae, J. H., and Jeon, H. M. (2019). Continuous intention on accommodation apps: Integrated value-based adoption and expectation-confirmation model analysis. Sustainability 11, 1–17. doi: 10.3390/su11061578
Kim, S. H., and Yoo, B. K. (2020). Factors influencing the reuse of mobile payment services in retail. J. Disturb. Sci. 18, 53–65. doi: 10.15722/jds.18.3.202003.53
Kotler, P., and Keller, K. L. (2006). Marketing Management. 12th edition. Upper Saddle River, New Jersey: Prentice-Hall.
Kuo, Y. F., Wu, C. M., and Deng, W. J. (2009). The relationships among service quality, perceived value, customer satisfaction, and post-purchase intention in mobile value-added services. Comp. Human Behav. 25, 887–896. doi: 10.1016/J.CHB.2009.03.003
Lavuri, R. (2021). Intrinsic factors affecting online impulsive shopping during the COVID-19 in emerging markets. Int. J. Emerg. Mark. doi: 10.1108/IJOEM-12-2020-1530
Le, H. T. K., Carrel, A. L., and Shah, H. (2022). Impacts of online shopping on travel demand: a systematic review. Transport Rev. 42, 273–295. doi: 10.1080/01441647.2021.1961917
Lee, S. W., Sung, H. J., and Jeon, H. M. (2019). Determinants of continuous intention on food delivery apps: extending UTAUT2 with information quality. Sustainability, 11, 3141. doi: 10.3390/su11113141
Liao, C., Palvia, P., and Lin, H. N. (2006). The roles of habit and web site quality in e-commerce. Int. J. Inform. Manag. 26, 469–483. doi: 10.1016/J.IJINFOMGT.2006.09.001
Limayem, M., and Hirt, S. (2003). Force of habit and information systems usage: theory and initial validation. J. Assoc. Inform. Sys. 4, 65–97. doi: 10.17705/1jais.00030
Lin, C., and Lekhawipat, W. (2014). Factors affecting online repurchase intention. Indust. Manag. Data Sys. 114, 597–611. doi: 10.1108/IMDS-10-2013-0432
Lin, W., Xu, A., Zheng, Q., Ke, L., and Lin, J. (2018). Influence of customer perceived value on the online shopping intention of aquatic products under B2C E-commerce. J. Disc. Mathemat. Sci. Cryptography 21, 1189–1192.
Lin, X., Wu, R. Z., Lim, Y. T., Han, J., and Chen, S. C. (2019). Understanding the sustainable usage intention of mobile payment technology in Korea: cross-countries comparison of Chinese and Korean users. Sustainability, 11, 1–23. doi: 10.3390/su11195532
Liu, C. H., Chen, Y. T., Kittikowit, S., Hongsuchon, T., and Chen, Y. J. (2022). Using unified theory of acceptance and use of technology to evaluate the impact of a mobile payment app on the shopping intention and usage behavior of middle-aged customers. Front. Psychol. 13, 1–11. doi: 10.3389/fpsyg.2022.830842
Lu, J., Yu, C. S., and Liu, C. (2009). Mobile data service demographics in urban China. J. Com. Inform. Sys. 50, 117–126. doi: 10.1080/08874417.2009.11645390
Marinković, V., Ð*ordević, A., and Kalinić, Z. (2019). The moderating effects of gender on customer satisfaction and continuance intention in mobile commerce: a UTAUT-based perspective. Technol. Anal. Strat. Manag. 32, 306–318. doi: 10.1080/09537325.2019.1655537
Martins, C., Oliveira, T., and Popovi,č, A. (2014). Understanding the internet banking adoption: a unified theory of acceptance and use of technology and perceived risk application. Int. J. Inform. Manag. 34, 1–13. doi: 10.1016/j.ijinfomgt.2013.06.002
Maulidina, P. R., Sarno, R., Sungkono, K. R., and Giranita, T. A. (2020). Using extended UTAUT2 Model to Determine Factors Influencing the Use of Shopee E-commerce. Proceedings-−2020 International Seminar on Application for Technology of Information and Communication: IT Challenges for Sustainability, Scalability, and Security in the Age of Digital Disruption, iSemantic 2020. Institute of Electrical and Electronics Engineers Inc (pp. 493–498). doi: 10.1109/iSemantic50169.2020.923425
McKinsey. (2020). Adapting to the next normal in retail | McKinsey. Available online at: https://www.mckinsey.com/industries/retail/our-insights/adapting-to-the-next-normal-in-retail-the-customer-experience-imperative (accessed January 29, 2022).
Mejía-Trejo, J. (2021). COVID-19 ads on purchase intention of online consumer behavior as business innovation activity: a contribution to the uses and gratification theory. Electron. Commer. Res. Appl. 49, 101086. doi: 10.1016/J.ELERAP.2021.101086
Miao, M., Jalees, T., Zaman, S. I., Khan, S., Hanif, N., ul, A., et al. (2022). The influence of e-customer satisfaction, e-trust and perceived value on consumer's repurchase intention in B2C e-commerce segment. Asia Pacific J. Mark. 34, 2184–2206. doi: 10.1108/APJML-03-2021-0221
Miles, J. (2014). R squared, adjusted R squared. Wiley StatsRef: Stat. Ref. Online. doi: 10.1002/9781118445112.stat06627
Mirkovski, K., Jia, Y., Liu, L., and Chen, K. (2018). Understanding microblogging continuance intention: The directed social network perspective. Inform. Technol. People, 31, 215–238. doi: 10.1108/ITP-07-2015-0168
Mohamed, N., Hussein, R., Hidayah Ahmad Zamzuri, N., and Haghshenas, H. (2014). Insights into individual's online shopping continuance intention. Indust. Manag. Data Sys. 114, 1453–1476. doi: 10.1108/imds-07-2014-0201
Mohanty, R. P., Seth, D., and Mukadam, S. (2007). Quality dimensions of E-commerce and their implications. Total Qual. Manag. Bus. Excell. 18, 219–247. doi: 10.1080/14783360601149992
Muangmee, C., Kot, S., Meekaewkunchorn, N., Kassakorn, N., and Khalid, B. (2021). Factors determining the behavioral intention of using food delivery apps during COVID-19 pandemics. J. Theoret. Appl. Elect. Comm. Res. 16, 1297–1310. doi: 10.3390/jtaer16050073
Musyaffi, A. M. (2021). Understanding of digital payment usage during COVID-19 pandemic: a study of UTAUT extension model in Indonesia. The J. Asian Finance Econ. Bus. 8, 475–482. doi: 10.13106/jafeb.2021.vol8.no6.0475
Nam, L. G., and An, N. T. T. (2021). Factors affecting the continuance intention to use food delivery apps of the millennials in Ho Chi Minh City. Technium Soc. Sci. J. 18, 404–417. doi: 10.47577/TSSJ.V18I1
Nazir, S., Khadim, S., Ali Asadullah, M., and Syed, N. (2023). Exploring the influence of artificial intelligence technology on consumer repurchase intention: the mediation and moderation approach. Technol. Soc. 72, 102190. doi: 10.1016/j.techsoc.2022.102190
Neal, D. T., Wood, W., Labrecque, J. S., and Lally, P. (2012). How do habits guide behavior? Perceived and actual triggers of habits in daily life. J. Exp. Soc. Psychol. 48, 492–498. doi: 10.1016/J.JESP.2011.10.011
Nguyen, D. M., Chiu, Y. T. H., Vu, H. Q., and Nguyen, T. (2022). Toward a unified theory of technology continuance: Effects of conscious versus unconscious factors. J. Consumer Behav. 21, 1219–1235. doi: 10.1002/cb.2073
Nguyen, H., Xuan Tran, H., Van Huy, L., Nhi Nguyen, X., Thanh Do, M., and Nguyen, N. (2020). Online book shopping in Vietnam: the impact of the COVID-19 pandemic situation. Publish. Res. Quart. 36, 437–445. doi: 10.1007/s12109-020-09732-2
Nursalam, N., Sulaeman, S., and Mustafa, I. (2021). Analisis istilah wacana kebijakan pembatasan sosial Covid-19 di Indonesia. KEMBARA: J. Keilmuan Bahasa, Sastra, dan Pengajarannya 7, 388–405. doi: 10.22219/kembara.v7i2.16500
Oh, J., and Kim, W. (2020). The effects of mobile payment system on consumer attitude and behavioral intention. J. Internet Elect. Comm. Res. 20, 35–50. doi: 10.37272/jiecr.2020.04.20.2.35
Oliver, R. L. (1980). A cognitive model of the antecedents and consequences of satisfaction decisions. J. Marketing Research, 17, 460. doi: 10.2307/3150499
Pan, S. L., Cui, M., and Qian, J. (2020). Information resource orchestration during the COVID-19 pandemic: a study of community lockdowns in China. Int. J. Inform. Manag. 54, 102143. doi: 10.1016/j.ijinfomgt.2020.102143
Pantano, E., Pizzi, G., Scarpi, D., and Dennis, C. (2020). Competing during a pandemic? Retailers' ups and downs during the COVID-19 outbreak. J. Business Research, 116, 209–213. doi: 10.1016/j.jbusres.2020.05.036
Parasuraman, A., and Grewal, D. (2000). The impact of technology on the quality-value-loyalty chain: a research agenda. J. the Acad. Market. Sci. 28, 168–174. doi: 10.1177/0092070300281015
Pham, Q. T., Tran, X. P., Misra, S., Maskeliunas, R., and Damaševičius, R. (2018). Relationship between convenience, perceived value, and repurchase intention in online shopping in Vietnam. Sustainability, 10, 156. doi: 10.3390/su10010156
Pink, B. (2021). YLKI catat selama pandemi aktivitas belanja online meningkat hingga 30%. Kontan. Available online at: https://nasional.kontan.co.id/news/ylki-catat-selama-pandemi-aktivitas-belanja-online-meningkat-hingga-30 (accessed March 5, 2023).
Pobee, F. (2021). Assessing online repurchase intention in a developing country. Int. J. Strat. Decis. Sci, 12, 61–76. doi: 10.4018/ijsds.2021010104
Prasetya, M. E., Mohamed, I. S., Shuhidan, S. M., and Wasistha, G. H. (2021). The COVID-19 pandemic as a driving force for E-wallet adoption in Indonesia. Bus. Manag. Horizons 9, 40. doi: 10.5296/bmh.v9i2.19335
Prasetyo, J. H., Prakoso, B. S., Wiharso, G., and Fabrianto, L. (2021). E-Commerce: The importance role of customer perceived value in increasing online repurchase intention. Dinasti Int. J. Digital Bus. Manag. 2, 955–962.
Purohit, S., Arora, R., and Paul, J. (2022). The bright side of online consumer behavior: Continuance intention for mobile payments. J. Consumer Behav. 21, 523–542. doi: 10.1002/CB.2017
Ramos, K. (2022). Factors influencing customers' continuance usage intention of food delivery apps during COVID-19 quarantine in Mexico. Br. Food J. 124, 833–852. doi: 10.1108/BFJ-01-2021-0020
Reddy, A. (2020). Covid-19 impact: Consumers move more towards digital - The Hindu Business Line. Available online at: https://www.thehindubusinessline.com/opinion/covid-19-impact-consumers-move-more-towards-digital/article31337127.ece (accessed September 22, 2021).
Rui, F. (2022). Research on the influence of interaction with the streamer on customers' purchase intention in the context of live-streaming e-commerce. Soc. Sci. 11, 373. doi: 10.11648/j.ss.20221106.14
Sarstedt, M., Ringle, C. M., and Hair, J. F. (2017). Partial least squares structural equation modeling. Handbook Market Res. 3, 1–40. doi: 10.1007/978-3-319-05542-8_15-1
Sheikh, Z., Islam, T., Rana, S., Hameed, Z., and Saeed, U. (2017). Acceptance of social commerce framework in Saudi Arabia. Telemat. Inform. 34, 1693–1708. doi: 10.1016/j.tele.2017.08.003
Sheppard, S. R. J., and Meitner, M. (2005). Using multi-criteria analysis and visualisation for sustainable forest management planning with stakeholder groups. Forest Ecol. Manag. 1–2, 171–187. doi: 10.1016/J.FORECO.2004.10.032
Shoheib, Z., and Abu-Shanab, E. A. (2022). Adapting the UTAUT2 model for social commerce context. Int. J. E-Bus. Res. 18, 293. doi: 10.4018/IJEBR.293293
Sinha, S. K., and Verma, P. (2020). Impact of sales Promotion's benefits on perceived value: does product category moderate the results? J. Retail. Consum. Serv. 52, 101887. doi: 10.1016/j.jretconser.2019.101887
SIRCLO Ravenry (2021). Navigating Indonesia's E-Commerce: COVID-19 Impact and The Rise of Social Commerce. SIRCLO and Ravenry, 55. Available online at: https://insights.sirclo.com/ (accessed March 7, 2023).
Statista (2020). E-commerce users in Indonesia 2025. Available online at: https://www.statista.com/forecasts/251635/e-commerce-users-in-indonesia (accessed March 5, 2023).
Taherdoost, H. (2018). A review of technology acceptance and adoption models and theories. Procedia Manufactur. 22, 960–967. doi: 10.1016/j.promfg.2018.03.137
Tam, C., Santos, D., and Oliveira, T. (2020). Exploring the influential factors of continuance intention to use mobile apps: extending the expectation confirmation model. Inform. Sys. Front. 22, 243–257. doi: 10.1007/s10796-018-9864-5
Tao, H., Sun, X., Liu, X., Tian, J., and Zhang, D. (2022). The impact of consumer purchase behavior changes on the business model design of consumer services companies over the course of COVID-19. Front. Psychol. 13. doi: 10.3389/FPSYG.2022.818845
Tenenhaus, M., Vinzi, V. E., Chatelin, Y. M., and Lauro, C. (2005). PLS path modeling. Computat. Stat. Data Anal. 48, 159–205. doi: 10.1016/J.CSDA.2004.03.005
Toska, A., Zeqiri, J., Ramadani, V., and Ribeiro-Navarrete, S. (2022). Covid-19 and consumers' online purchase intention among an older-aged group of Kosovo. Int. J. Emerg. Markets, 3, 1875. doi: 10.1108/IJOEM-12-2021-1875/FULL/XML
Urueña-López, A., Pascual-Miguel, F., and Iglesias-Pradas, S. (2012). Value, quality, purchasing habits and repurchase intention in B2C: differences between frequent and occasional purchaser. Direccion y Organ. 47, 70–80.
Vehovar, V., Toepoel, V., and Steinmetz, S. (2016). “Non-probability sampling,” in The SAGE Handbook of Survey Methodology, eds C. Wolf, D. Joye, T. W. Smith, and Y.C., Fu (pp. 329-345). (London: Sage Publications).
Venkatesh, V., Morris, M. G., Davis, G. B., and Davis, F. D. (2003). User Acceptance of Information technology: toward a unified view. MIS Quart.Manag. Inform. Sys. 27, 425–478. doi: 10.2307/30036540
Venkatesh, V., Thong, J. Y. L., and Xu, X. (2012). Consumer acceptance and use of information technology: Extending the unified theory of acceptance and use of technology. MIS Quart. Manag. Inform. Sys. 36, 157–178. doi: 10.2307/41410412
Vinerean, S., Budac, C., Baltador, L. A., and Dabija, D. C. (2022). Assessing the effects of the COVID-19 pandemic on M-commerce adoption: an adapted UTAUT2 approach. Elect. 11, 1296. doi: 10.3390/electronics11081269
Von Abrams, K. (2021). Global Ecommerce Forecast 2021. Avaible online at: l https://www.emarketer.com/content/global-ecommerce-forecast-2021 (accessed October 11, 2022).
Wang, E., An, N., Gao, Z., Kiprop, E., and Geng, X. (2020). Consumer food stockpiling behavior and willingness to pay for food reserves in COVID-19. Food Sec. 12, 739–747. doi: 10.1007/s12571-020-01092-1
Wang, X., Wong, Y. D., Qi, G., and Yuen, K. F. (2021). Contactless channel for shopping and delivery in the context of social distancing in response to COVID-19 pandemic. Elect. Comm. Res. Appl. 48, 101075. doi: 10.1016/j.elerap.2021.101075
Wheaton, B. (1987). Assessment of fit in overidentified models with latent variables. Sociol. Methods Res. 16, 118–154. doi: 10.1177/0049124187016001005
Wijaya, T. T., Cao, Y., Weinhandl, R., Yusron, E., and Lavicza, Z. (2022). Applying the UTAUT model to understand factors affecting micro-lecture usage by mathematics teachers in China. Mathematics, 10, 1–20. doi: 10.3390/math10071008
Winanti, A., Sulastri, S., and Bakhtiar, H. S. (2022). The roles of governments in implementing large-scale social restrictions to accelerate Covid-19 handling. J. Commun. Posit. Pract. 22, 77–85. doi: 10.35782/JCPP.2022.1.05
Wu, L. Y., Chen, K. Y., Chen, P. Y., and Cheng, S. L. (2014). Perceived value, transaction cost, and repurchase-intention in online shopping: a relational exchange perspective. J. Bus. Res., 67, 2768–2776. doi: 10.1016/J.JBUSRES.2012.09.007
Yang, K., and Lee, H. J. (2010). Gender differences in using mobile data services: Utilitarian and hedonic value approaches. J. Res. Inter. Mark 4, 142–156. doi: 10.1108/17505931011051678
Yoon, J., Narasimhan, R., and Kim, M. K. (2018). Retailer's sourcing strategy under consumer stockpiling in anticipation of supply disruptions. Int. J. Prod. Res. 56, 3615–3635. doi: 10.1080/00207543.2017.1401748
Zeithaml, V. A. (1988). Consumer perceptions of price, quality, and value: a Means-end model and synthesis of evidence. J. Mark. 52, 2–22. doi: 10.1177/002224298805200302
Zhang, H., Zhang, K. Z. K., Lee, M. K. O., and Feng, F. (2015). Brand loyalty in enterprise microblogs: Influence of community commitment, IT habit, and participation. Inform. Technol. People, 28, 304–326. doi: 10.1108/ITP-03-2014-0047
Zhao, Y., and Bacao, F. (2020). What factors determining customer continuingly using food delivery apps during 2019 novel coronavirus pandemic period? Int. J. Hospital. Manag.91, 102683. doi: 10.1016/j.ijhm.2020.102683
Zhao, Y., and Bacao, F. (2021). How does the pandemic facilitate mobile payment? An investigation on users' perspective under the COVID-19 pandemic. Int. J. Environ. Res. Public Health 18, 1–22. doi: 10.3390/ijerph18031016
Keywords: online repurchase intention, social contingency, performance confirmation, effort confirmation, customer perceived value, habit
Citation: Wu W, Widiatmo G and Riantama D (2023) What motivates customers to repurchase online under social distancing?. Front. Psychol. 14:1155302. doi: 10.3389/fpsyg.2023.1155302
Received: 31 January 2023; Accepted: 23 March 2023;
Published: 25 July 2023.
Edited by:
Omkar Dastane, UCSI University, MalaysiaReviewed by:
Wasim Ahmad, UCSI University, MalaysiaCopyright © 2023 Wu, Widiatmo and Riantama. This is an open-access article distributed under the terms of the Creative Commons Attribution License (CC BY). The use, distribution or reproduction in other forums is permitted, provided the original author(s) and the copyright owner(s) are credited and that the original publication in this journal is cited, in accordance with accepted academic practice. No use, distribution or reproduction is permitted which does not comply with these terms.
*Correspondence: Gumilang Widiatmo, Z3VtaWxhbmd3aWRpYXRtb0BnbWFpbC5jb20=
Disclaimer: All claims expressed in this article are solely those of the authors and do not necessarily represent those of their affiliated organizations, or those of the publisher, the editors and the reviewers. Any product that may be evaluated in this article or claim that may be made by its manufacturer is not guaranteed or endorsed by the publisher.
Research integrity at Frontiers
Learn more about the work of our research integrity team to safeguard the quality of each article we publish.