- 1Department of Psychology, Université de Lorraine, 2LPN, Nancy, France
- 2Centre d’Économie de la Sorbonne, CNRS UMR 8174, Paris, France
- 3CNRS and Paris School of Economics, Paris, France
Over the last two decades, there has been a growing interest in the study of individual differences in how people’s judgments and decisions deviate from normative standards. We conducted a systematic review of heuristics-and-biases tasks for which individual differences and their reliability were measured, which resulted in 41 biases measured over 108 studies, and suggested that reliable measures are still needed for some biases described in the literature. To encourage and facilitate future studies on heuristics and biases, we centralized the task materials in an online resource: The Heuristics-and-Biases Inventory (HBI; https://sites.google.com/view/hbiproject). We discuss how this inventory might help research progress on major issues such as the structure of rationality (single vs. multiple factors) and how biases relate to cognitive ability, personality, and real-world outcomes. We also consider how future research should improve and expand the HBI.
1. Introduction
The heuristics-and-biases (HB) research program, introduced by Tversky and Kahneman in the early 1970s (Kahneman and Tversky, 1972; Tversky and Kahneman, 1973, 1974), is a descriptive approach to decision-making that consists of invoking heuristics (mental shortcuts) to explain systematic deviations from rational choice behavior. For instance, people may misestimate a numerical value because of an overreliance on information that comes to mind and insufficient adjustment (anchoring-and-adjustment heuristic; Tversky and Kahneman, 1974). Another well-known example of a cognitive bias is the framing effect, by which individuals respond differently to a choice problem when the possible outcomes are framed as gains or as losses.
Since its inception, research on HB has produced a large literature on errors in judgment and decision-making (Gilovich et al., 2002) and triggered much discussion. Important questions include, among others, whether deviations from rationality can be reduced to randomness in choice (Stanovich and West, 2000), and whether HB effects are universal or instead vary across situations (e.g., due to the ecological or non-ecological nature of the task; Gigerenzer, 1996, 2008) or across individuals (Stanovich and West, 1998; Baron, 2008). Indeed, not all HB effects are present to the same extent in all individuals. Some biases are more prevalent than others: loss aversion might be found in a large majority of individuals (Gächter et al., 2022), whereas framing effects might not (Li and Liu, 2008). Some individuals might be more susceptible than others. In the case of attribution bias, for instance, that is the observation that individuals are more prone to credit themselves for positive than for negative events, a large meta-analysis conducted by Mezulis et al. (2004) demonstrated significant variations across countries, across genders, as well as associations with clinical symptoms.
In addition, to go beyond establishing a list of biases, efforts have been made to describe how different biases relate to each other. In this line of work, some studies have argued for a common decision-making competence underlying several HB tasks (Bruine de Bruin et al., 2007), akin to the g-factor (Carroll, 1993), whereas other studies covering a more heterogeneous set of tasks have provided support for a more complex, multidimensional structure (Klaczynski, 2001; Weaver and Stewart, 2012; Aczel et al., 2015; Teovanović et al., 2015; Ceschi et al., 2019; Berthet et al., 2022; Erceg et al., 2022; Rieger et al., 2022; Burgoyne et al., 2023). This research illustrates how the cognitive structure underlying heuristics and biases in decision-making can be investigated using individual differences.
Individual differences, however, have not been the main focus of earlier research on HB effects. The first reason is that the goal of this research was to demonstrate the existence of HB effects in the first place, on average, across participants. A second reason was the methodological choice to do so using between-subjects designs. This choice was notably motivated by the assumption that between-subjects designs favor spontaneous, intuitive answers in individuals, which are precisely the phenomenon of interest in HB research. As Kahneman (2000, p. 682) puts it: “much of life resembles a between-subjects experiment.” By contrast, within-subject designs would be more transparent to participants, emphasizing the comparison between the conditions of interest, which might trigger the engagement of a slower, more deliberative system, and the override of intuitive answers, thereby reducing HB effects (Kahneman and Tversky, 2000; Kahneman and Frederick, 2005).
Critically though, the assumption that within-subject designs would produce smaller HB effects has not always been supported in empirical studies (Piñon and Gambara, 2005; Aczel et al., 2018; Gächter et al., 2022). In addition, regarding transparency, participants may remain unable to identify the research hypothesis in within-subject designs (Lambdin and Shaffer, 2009). In addition, they offer better statistical power than between-subject designs, and they eliminate confounds related to potential differences between participants in the different experimental conditions. Thus, within-subject designs seem appropriate tools to examine HB effects. As they allow for measuring biases at the individual level, these designs are particularly suited for individual differences. However, the measurement of individual differences in HB raises a practical and methodological issue: Finding such measures can be difficult and time-consuming while we still do not know much about their reliability.
The goal of the present study is to address these issues. First, we identify the currently available tasks that measure HB effects at the individual level. To do so, we conduct a systematic survey of empirical studies measuring one or more cognitive biases in a within-subject manner, focusing on studies in which the reliability of the measure used to quantify the bias is documented. Indeed, tasks optimized for large average effects might turn out to be less reliable at the individual level, producing a tradeoff between effect size and reliability (Hedge et al., 2018). We find that when reliability is documented, it is usually good. However, there are also a good number of HB effects for which no within-subject design has been tested or for which reliability is not known.
Second, we introduce an open online resource for the scientific community: the Heuristics-and-Biases Inventory (HBI1). This platform aims primarily at providing in a single location the experimental material for quantifying HB effects at the single subject level. The platform is meant to include new tasks as they are developed. Our hope is that this contribution will foster research on individual differences regarding cognitive heuristics and biases.
2. Methods
We conducted a systematic review in accordance with the PRISMA guidelines (Page et al., 2021).2
2.1. Search strategy
The following databases were searched for peer-reviewed empirical articles in June 2022: Web of Science, PsycINFO, and Pubmed. Our search strategy was based on the conjunction of two criteria: (1) the presence of “individual differences” in the title or in the abstract and (2) the presence of the terms “heuristics and biases” OR “cognitive bias” OR “cognitive biases” OR “behavioral biases” OR “rationality” OR “Decision-Making Competence” in the title or the abstract. All entries were imported in Zotero to remove duplicates, after which titles and abstracts were screened independently by two coders, according to predefined eligibility criteria.
Noteworthy, this search strategy had two implications. First, we likely missed relevant papers as we did not enter every single HB as a keyword, thereby limiting the comprehensiveness of our inventory. Second, our search strategy would not necessarily filter out studies that addressed psychological biases other than those pertaining to the heuristics-and-biases tradition (judgment and decision-making) such as health anxiety-related biases (e.g., interpretive bias and negativity bias) and the cognitive bias modification paradigm which aims at reducing them (e.g., Hallion and Ruscio, 2011).
2.2. Inclusion and exclusion criteria for studies
Included studies had to (1) be published in peer-reviewed scientific journals, (2) be written in English, and (3) be conducted on human participants. Reviews, conceptual or theoretical articles, book chapters, conference proceedings, dissertations, and editorial materials were excluded. We also excluded as follows: (1) Studies that addressed biases not pertaining to the HB tradition (e.g., health anxiety-related biases and implicit biases) for reasons previously mentioned, (2) studies in which self-report (questionnaires) rather than behavioral measures were used, (3) studies that merely applied the Adult Decision-Making Competence (ADMC), (4) studies in which a between-subject design was used. In addition, we chose not to include in the inventory two biases related to risk aversion (ambiguity aversion and zero-risk bias), which refers to a preference rather than a rationality failure (refer to the Discussion section).
2.3. Data collection and analysis
Relevant data was extracted by VB. The following information was collected for each study: author names, year of publication, title and journal where the study was published, study design, number of participants, inclusion/exclusion criteria, the HB task(s) used, whether the task(s) included single or multiple items, and the estimated reliability when reported. Discrepancies that emerged after full-text screening were resolved through a consensus meeting.
3. Results
Figure 1 displays the PRISMA flowchart with full detail of this process. The complete search resulted in 1429 articles, leaving 1,091 articles once duplicates were removed. After title and abstract screening, 109 articles met the inclusion criteria and were eligible for full-text assessment. One study was subsequently excluded because the author published the same data in another article. A total of 108 studies met eligibility criteria and were included in the review.
3.1. Study characteristics
Overall, the 108 studies included a total of 58,808 participants. Slightly more than half of the studies investigated a single HB (n = 56), while the rest addressed multiple HB (n = 51). Regarding the number of items, studies used one or several single-item tasks (n = 29), one or several multi-item tasks (n = 64), or both single and multi-item tasks (n = 14). Critically, out of the 78 studies that used multi-item tasks in the present survey, only 14 reported estimates of score reliability.
3.2. An inventory of tasks measuring heuristics and biases
Table 1 provides a reduced presentation of the outcome of this systematic survey. We identified 41 heuristics and biases for which there are tasks to measure individual differences. For each bias, we indicate the original paper introducing a typical task to measure the bias, the description of the task, the number of items, and estimated reliability when reported. A full version of the table is available in the supplementary material and on the HBI website, which also indicates, for each bias, the scoring rule and references of studies that merely used the task but did not report reliability. Note that there can be different measures available for some biases (e.g., anchoring) and that the same task can be associated with different scoring procedures (e.g., framing). The items for each task are available on the HBI website.
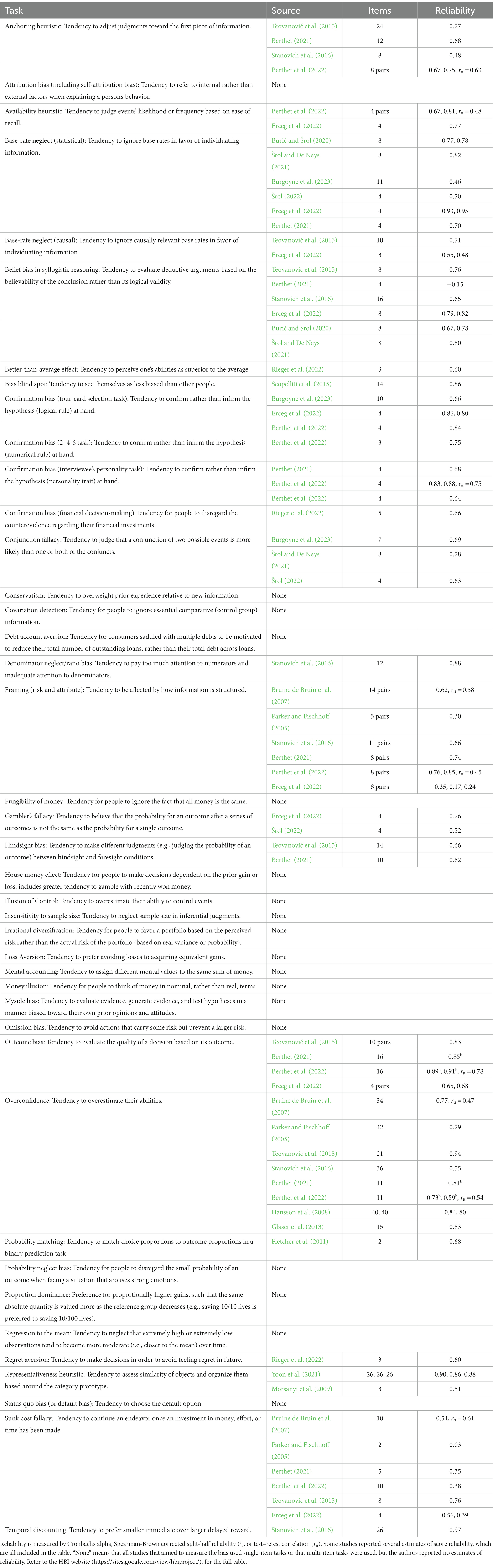
Table 1. Inventory and reliability of tasks measuring individual differences in heuristics and biases.
It turns out that the list includes the main biases studied in HB research. In fact, 18 out of the 41 HB are among the biases that violate normative models listed by Baron (2008). Our review points out, however, that there has been no attempt to measure individual differences for several significant biases, such as planning fallacy and prominence effect.
3.3. Reliability
Reliability (internal consistency) can be only estimated when multi-item tasks are used. Although this was the case for 23 of the HB tasks identified here, only 14 of the reviewed studies reported estimates of internal consistency, and two studies assessed test–retest reliability (Bruine de Bruin et al., 2007; Berthet et al., 2022). For instance, for the status quo bias, or for the insensitivity to sample size, the reliabilities of the measures are unknown. In addition, 11 HB have been measured only with single-item tasks so far (ambiguity aversion, attribution bias, conservatism, denominator neglect, illusion of control, loss aversion, mental accounting, myside bias, omission bias, proportion dominance, and regression to the mean).
Based on the available estimates of internal consistency (excluding estimates of test–retest reliability which are too infrequent), the reliability of HB scores is most often above the generally accepted standard of 0.70 (Nunnally and Bernstein, 1994). This finding is noteworthy and confirms that despite the “reliability paradox” described by Hedge et al. (2018), tasks that were primarily designed to produce robust between-subject experimental effects can be turned into reliable measures of individual differences (note, however, that our estimate might be inflated by publication bias). Some exceptions are the framing effects and sunk cost fallacy, for which low reliabilities have been repeatedly found.
4. Discussion
The aim of the present study was to provide a systematic review of individual difference measures used in heuristics-and-biases research. Based on 108 studies, we listed 41 biases for which at least one behavioral task allows one to calculate individual scores. While it is apparent that some of the tasks belong to a particular category (e.g., availability heuristic, conjunction fallacy, gambler’s fallacy, probability matching, and base-rate neglect all assess biases in probability), we did not organize the tasks according to a particular theoretical taxonomy (e.g., Baron, 2008; Stanovich et al., 2008). Indeed, a key aim of the HBI is to help researchers build a robust empirical classification of HB by allowing them to include a large number of tasks in the study design and, therefore, to test the validity of the existing theoretical taxonomies (Refer to the following text).
Noteworthy, our review raised the issue of the reliability of such scores. Indeed, a significant number of HB have been measured only with single-item tasks, which does not allow checking reliability. When multi-item tasks are used, the reliability of scores is not systematically reported. In addition, low-reliability estimates have been repeatedly found for some biases (e.g., framing and sunk cost fallacy). However, based on the available estimates of internal consistency, the reliability of HB scores turns out to be most often above the generally accepted standard of 0.70. We encourage researchers to (1) use multi-item tasks and systematically report score reliability, (2) avoid calculating composite scores derived from single-item HB tasks as such scores are unreliable (West et al., 2008; Toplak et al., 2011; Aczel et al., 2015).
In the following subsections, we discuss the limits of our systematic review, how the HBI relates to existing taxonomies, and how it could be used to further address the impact of cognitive biases on real-life behavior.
4.1. Limitations of the systematic review
There are several limitations of this systematic review worth considering. First, the comprehensiveness of our inventory is limited by our search strategy. In order to cover all papers that addressed individual differences in HB, one should enter every single heuristic and bias as a keyword, which we did not do for practical reasons. Note, however, that our review was not meant to be exhaustive but rather to lay the foundation for listing HB tasks that are suited for the measurement of individual differences. As a collaborative and evolutive repository, the HBI may become more exhaustive over time.
The second limit relates to the selection of biases. As mentioned in the Methods section, we excluded psychological biases that do not fall within the category of heuristics and biases, defined as rationality failures. In particular, health anxiety-related biases such as interpretive bias (the tendency to inappropriately analyze ambiguous stimuli) and negativity bias (the tendency to pay more attention or give more weight to negative experiences over neutral or positive experiences) are typically not considered in the classification of biases in the heuristics-and-biases approach (Baron, 2008). Similarly, we did not include in our inventory two biases related to risk aversion (ambiguity aversion and zero-risk bias), which refers to a preference rather than a rationality failure (an individual is considered risk averse if she prefers a certain or risky option to a riskier option with equal or higher expected value while an individual who prefers a risky option to a certain or less risky option with higher expected value will be considered risk-seeking; Fox et al., 2015). However, one could argue that the exclusion of such biases is somewhat arbitrary as there is no objective criterion to qualify a bias under the heuristics-and-biases approach. Based on how the HBI is used by researchers, we will consider the possibility of expanding the scope of the inventory to include other types of biases.
4.2. HBI and existing inventories
We discuss here how the HBI compares with two related tools, the ADMC and the Comprehensive Assessment of Rational Thinking (CART; Stanovich et al., 2016). The ADMC is a set of six behavioral tasks measuring different aspects of decision-making (resistance to framing, recognizing social norms, overconfidence, applying decision rules, consistency in risk perception, and resistance to sunk costs) (Parker and Fischhoff, 2005; Bruine de Bruin et al., 2007)3. Three of the ADMC tasks can be identified as HB tasks (resistance to framing, overconfidence, and resistance to sunk costs). The full-form CART includes 20 subtests, some of them measuring HB (e.g., gambler’s fallacy, four-card selection task, and anchoring). Noteworthy, the CART and the HBI have different aims. The CART is an instrument that aims to provide an overall measure of rational thinking (the same way IQ tests measure intelligence): A given number of points is attributed to each subtest, and an overall rational thinking score (Rationality Quotient) is calculated (the full-form CART takes about 3 h to complete). Indeed, each subtest is thought to reflect a single subconstruct within the concept of rationality. Accordingly, the CART subtests are not thought to be used separately. On the other hand, the HBI follows a more basic and practical aim: Providing researchers with an open, collaborative, and evolutive inventory of HB tasks, each of which can be used separately.
4.3. HBI and future research
We argue that the HBI has the potential to help researchers in their investigation of several issues. The first one is the structure of rationality. Similar to other topics in psychology (e.g., intelligence, personality, executive functions, and risk preference), early studies on HB that followed an individual differences approach aimed to explore whether single or multiple factors accounted for the correlations between performance on various tasks. While some studies have suggested the existence of a single rationality factor (Stanovich and West, 1998; Bruine de Bruin et al., 2007; Erceg et al., 2022), several factor analytic studies supported multiple-factor solutions, which relate more or less to existing taxonomies of HB (e.g., Klaczynski, 2001; Weaver and Stewart, 2012; Aczel et al., 2015; Teovanović et al., 2015; Ceschi et al., 2019; Berthet, 2021; Rieger et al., 2022).
Irrespective of their results, virtually all studies that explored the structure of rationality suffered from two limitations. First, scores for some HB tasks (even multi-item ones) failed to reach satisfactory levels of reliability (e.g., Ceschi et al., 2019; Erceg et al., 2022), thereby questioning the robustness of the factorial solution. Second, the sample of HB tasks submitted to factor analysis was limited (mainly because of practical limits such as total testing duration) and not being representative of all biases listed in the literature. That limitation is important as one could reasonably expect that a higher number of tasks would result in a higher number of factors extracted. Indeed, Berthet et al. (2022) showed that there was no longer evidence of a general decision-making competence when adding four HB tasks to the six ADMC tasks while ensuring satisfactory levels of score reliability. By providing researchers with more HB tasks producing reliable scores, the HBI will further shed light on the structure of rationality. Indeed, performing factor analysis on more exhaustive samples of tasks might eventually lead to more robust empirical taxonomies of biases (Ceschi et al., 2019).
Second, the HBI will allow researchers to further address how heuristics and biases correlate with cognitive ability (Stanovich and West, 2008; Oechssler et al., 2009; Stanovich, 2012; Teovanović et al., 2015; Erceg et al., 2022; Burgoyne et al., 2023), personality traits (Soane and Chmiel, 2005; McElroy and Dowd, 2007; Weller et al., 2018), and real-life behavior (Toplak et al., 2017). Regarding the latter, Bruine de Bruin et al. (2007) reported that the ADMC components predicted significant and unique (after controlling for cognitive ability) variance on the Decision Outcome Inventory (DOI), a self-report questionnaire measuring the tendency to avoid negative real-life decision outcomes (e.g., rented a movie and returned it without having watched it at all) (refer to also Parker et al., 2015). However, Erceg et al. (2022) found no evidence that performance on HB tasks predicts various self-reported real-life decision outcomes (DOI, job and career satisfaction, peer-rated decision-making quality). In particular, personality traits (conscientiousness and emotional stability) were the most predictive of DOI scores (Berthet et al., 2022, found similar—unpublished—results). It is worth noting, however, that these studies included relatively few HB so how they relate to real-life behavior remains an issue to be further addressed.
5. Conclusion
As highlighted by Gertner et al. (2016, p. 3), “the study of bias within an individual difference framework is still largely in its infancy.” The present article aims to introduce the HBI, an exhaustive inventory of behavioral tasks that allow for a reliable measurement of individual differences in heuristics and biases. The aim of the HBI is to foster individual differences research in heuristics and biases by improving the visibility and accessibility of the relevant measures. As a collaborative and evolutive repository of all available measures, the success of the HBI project depends on the scientific community. Indeed, we invite researchers to support the HBI by reporting any use of the tasks (published or unpublished) and submit their own—new or alternative—measures of heuristics and biases. This open and collaborative approach will allow us to share results and continually expand the inventory.
Large-scale studies will allow to establish norm data from the general population and specific groups (e.g., documenting effects of gender and age) for each bias. Thus, our hope is that the HBI can help the research on individual differences in heuristics and biases to progress from infancy to adulthood.
Data availability statement
The original contributions presented in the study are included in the article/supplementary material, further inquiries can be directed to the corresponding author.
Author contributions
VB: conceptualization, methodology, and writing. VG: conceptualization and writing. All authors contributed to the article and approved the submitted version.
Acknowledgments
The author is grateful to Predrag Teovanović for feedback on an earlier draft of this study.
Conflict of interest
The authors declare that the research was conducted in the absence of any commercial or financial relationships that could be construed as a potential conflict of interest.
Publisher’s note
All claims expressed in this article are solely those of the authors and do not necessarily represent those of their affiliated organizations, or those of the publisher, the editors and the reviewers. Any product that may be evaluated in this article, or claim that may be made by its manufacturer, is not guaranteed or endorsed by the publisher.
Supplementary material
The Supplementary material for this article can be found online at: https://www.frontiersin.org/articles/10.3389/fpsyg.2023.1145246/full#supplementary-material
Footnotes
1. ^https://sites.google.com/view/hbiproject/
2. ^The original ADMC included a seventh task (path independence) which has been removed due to low reliability and validity.
3. ^Studies included in the systematic review are referenced in the online material only: https://osf.io/5xg92/.
References
Aczel, B., Bago, B., Szollosi, A., Foldes, A., and Lukacs, B. (2015). Measuring individual differences in decision biases: methodological considerations. Front. Psychol. 6:1770. doi: 10.3389/fpsyg.2015.01770
Aczel, B., Szollosi, A., and Bago, B. (2018). The effect of transparency on framing effects in within-subject designs. J. Behav. Decis. Mak. 31, 25–39. doi: 10.1002/bdm.2036
Berthet, V. (2021). The measurement of individual differences in cognitive biases: a review and improvement. Front. Psychol. 12:630177. doi: 10.3389/fpsyg.2021.630177
Berthet, V., Autissier, D., and de Gardelle, V. (2022). Individual differences in decision-making: a test of a one-factor model of rationality. Personal. Individ. Differ. 189:111485. doi: 10.1016/j.paid.2021.111485
Berthet, V., Teovanović, P., and de Gardelle, V. (2022). Confirmation bias in hypothesis testing: A unitary phenomenon?
Bruine de Bruin, W., Parker, A. M., and Fischhoff, B. (2007). Individual differences in adult decision-making competence. J. Pers. Soc. Psychol. 92, 938–956. doi: 10.1037/0022-3514.92.5.938
Burič, R., and Šrol, J. (2020). Individual differences in logical intuitions on reasoning problems presented under two-response paradigm. Journal of Cognitive Psychology 32, 460–477.
Burgoyne, A. P., Mashburn, C. A., Tsukahara, J. S., Hambrick, D. Z., and Engle, R. W. (2023). Understanding the relationship between rationality and intelligence: a latent-variable approach. Think. Reason. 23, 1–42. doi: 10.1080/13546783.2021.2008003
Carroll, J. B. (1993). Human cognitive abilities: A survey of factor-analytic studies. New York: Cambridge University Press
Ceschi, A., Costantini, A., Sartori, R., Weller, J., and Di Fabio, A. (2019). Dimensions of decision-making: an evidence-based classification of heuristics and biases. Personal. Individ. Differ. 146, 188–200. doi: 10.1016/j.paid.2018.07.033
Erceg, N., Galić, Z., and Bubić, A. (2022). Normative responding on cognitive bias tasks: some evidence for a weak rationality factor that is mostly explained by numeracy and actively open-minded thinking. Intelligence 90:101619. doi: 10.1016/j.intell.2021.101619
Fletcher, J. M., Marks, A. D. G., and Hine, D. W. (2011). Working memory capacity and cognitive styles in decision-making Personality and Individual Differences. 50, 1136–1141.
Fox, C. R., Erner, C., and Walters, D. (2015). “Decision under risk: from the field to the lab and back” in Handbook of judgment and decision making. eds. G. Keren and G. Wu (New York: Wiley), 43–88.
Gächter, S., Johnson, E. J., and Herrmann, A. (2022). Individual-level loss aversion in riskless and risky choices. Theor. Decis. 92, 599–624. doi: 10.1007/s11238-021-09839-8
Gertner, A., Zaromb, F., Schneider, R., Roberts, R. D., and Matthews, G. (2016). The assessment of biases in cognition: Development and evaluation of an assessment instrument for the measurement of cognitive bias (MITRE technical report MTR160163). McLean, VA: The MITRE Corporation.
Gigerenzer, G. (1996). On narrow norms and vague heuristics: a reply to Kahneman and Tversky. Psychol. Rev. 103, 592–596. doi: 10.1037/0033-295X.103.3.592
Gigerenzer, G. (2008). Why heuristics work. Perspect. Psychol. Sci. 3, 20–29. doi: 10.1111/j.1745-6916.2008.00058.x
Gilovich, T., Griffin, D., and Kahneman, D. (Eds.). (2002). Heuristics and biases: The psychology of intuitive judgment. New York: Cambridge University Press
Glaser, M., Langer, T., and Weber, M. (2013). True overconfidence in interval estimates: Evidence based on a new measure of miscalibration. Journal of Behavioral Decision Making 26, 405–407.
Hallion, L. S., and Ruscio, A. M. (2011). A meta-analysis of the effect of cognitive bias modification on anxiety and depression. Psychol. Bull. 137, 940–958. doi: 10.1037/a0024355
Hansson, P., Rönnlund, M., Juslin, P., and Nilsson, L. G. (2018). Adult age differences in the realism of confidence judgments: overconfidence, format dependence, and cognitive predictors. Psychology and aging 23, 531–544.
Hedge, C., Powell, G., and Sumner, P. (2018). The reliability paradox: why robust cognitive tasks do not produce reliable individual differences. Behav. Res. Methods 50, 1166–1186. doi: 10.3758/s13428-017-0935-1
Kahneman, D. (2000). A psychological point of view: violations of rational rules as a diagnostic of mental processes. Behav. Brain Sci. 23, 681–683. doi: 10.1017/S0140525X00403432
Kahneman, D., and Frederick, S. (2005). “A model of heuristic judgment” in The Cambridge handbook of thinking and reasoning. eds. K. J. Holyoak and R. Morrison (Cambridge: Cambridge University Press), 267–293.
Kahneman, D., and Tversky, A. (1972). Subjective probability: a judgment of representativeness. Cogn. Psychol. 3, 430–454. doi: 10.1016/0010-0285(72)90016-3
Kahneman, D., and Tversky, A. (Eds.). (2000). Choices, values and frames. New York: Cambridge University Press
Klaczynski, P. A. (2001). Analytic and heuristic processing influences on adolescent reasoning and decision-making. Child Dev. 72, 844–861. doi: 10.1111/1467-8624.00319
Lambdin, C., and Shaffer, V. A. (2009). Are within-subjects designs transparent? Judgm. Decis. Mak. 4, 544–566. doi: 10.1017/S1930297500001133
Li, S., and Liu, C.-J. (2008). Individual differences in a switch from risk-averse preferences for gains to risk-seeking preferences for losses: can personality variables predict the risk preferences? J. Risk Res. 11, 673–686. doi: 10.1080/13669870802086497
McElroy, T., and Dowd, K. (2007). Susceptibility to anchoring effects: how openness-to-experience influences responses to anchoring cues. Judgm. Decis. Mak. 2, 48–53. doi: 10.1017/S1930297500000279
Mezulis, A. H., Abramson, L. Y., Hyde, J. S., and Hankin, B. L. (2004). Is there a universal positivity bias in attributions? A meta-analytic review of individual, developmental, and cultural differences in the self-serving attributional bias. Psychol. Bull. 130, 711–747. doi: 10.1037/0033-2909.130.5.711
Morsanyi, K., Primi, C., Chiesi, F., and Handley, S. (2009). The effects and side-effects of statistics education: Psychology students’ (mis-)conceptions of probability. Contemporary Educational Psychology, 34, 210–220.
Oechssler, J., Roider, A., and Schmitz, P. W. (2009). Cognitive abilities and behavioral biases. J. Econ. Behav. Organ. 72, 147–152. doi: 10.1016/j.jebo.2009.04.018
Page, M. J., McKenzie, J. E., Bossuyt, P. M., Boutron, I., Hoffmann, T. C., Mulrow, C. D., et al. (2021). The PRISMA 2020 statement: an updated guideline for reporting systematic reviews. BMJ 372:n71 doi: 10.1136/bmj.n71
Parker, A. M., de Bruin, W. B., and Fischhoff, B. (2015). Negative decision outcomes are more common among people with lower decision-making competence: an item-level analysis of the decision outcome inventory (DOI). Front. Psychol. 6:363. doi: 10.3389/fpsyg.2015.00363
Parker, A. M., and Fischhoff, B. (2005). Decision-making competence: external validation through an individual-differences approach. J. Behav. Decis. Mak. 18, 1–27. doi: 10.1002/bdm.481
Piñon, A., and Gambara, H. (2005). A meta-analytic review of framing effect: risky, attribute and goal framing. Psicothema 17, 325–331. doi: 10.1136/bmj.n71
Rieger, M. O., Wang, M., Huang, P.-K., and Hsu, Y.-L. (2022). Survey evidence on core factors of behavioral biases. J. Behav. Exp. Econ. 100:101912. doi: 10.1016/j.socec.2022.101912
Scopelliti, I., Morewedge, C. K., McCormick, E., Min, H., Lebrecht, S., and Kassam, K. S. (2015). Bias blind spot: Structure, measurement, and consequences. Management Science. 61:2468–2486.
Soane, E., and Chmiel, N. (2005). Are risk preferences consistent? The influence of decision domain and personality. Personal. Individ. Differ. 38, 1781–1791. doi: 10.1016/j.paid.2004.10.005
Šrol, J. (2022). Individual differences in epistemically suspect beliefs: The role of analytic thinking and susceptibility to cognitive biases Thinking and reasoning. 28, 125–162.
Šrol, J., and De Neys, W. (2021). Predicting individual differences in conflict detection and bias susceptibility during reasoning. Thinking and Reasoning. 27, 38–68.
Stanovich, K. E. (2012). “On the distinction between rationality and intelligence: implications for understanding individual differences in reasoning” in The Oxford handbook of thinking and reasoning. eds. K. J. Holyoak and R. G. Morrison (New York: Oxford University Press), 433–455.
Stanovich, K. E., Toplak, M. E., and West, R. F. (2008). “The development of rational thought: a taxonomy of heuristics and biases” in Advances in child development and behavior. ed. R. V. Kail (San Diego, CA: Elsevier Academic Press), 251–285.
Stanovich, K. E., and West, R. F. (1998). Individual differences in rational thought. J. Exp. Psychol. Gen. 127, 161–188. doi: 10.1037/0096-3445.127.2.161
Stanovich, K. E., and West, R. F. (2000). Individual differences in reasoning: implications for the rationality debate? Behav. Brain Sci. 23, 645–665. doi: 10.1017/S0140525X00003435
Stanovich, K. E., and West, R. F. (2008). On the relative independence of thinking biases and cognitive ability. J. Pers. Soc. Psychol. 94, 672–695. doi: 10.1037/0022-3514.94.4.672
Stanovich, K. E., West, R. F., and Toplak, M. E. (2016). The rationality quotient: Toward a test of rational thinking. Cambridge, MA: MIT Press
Teovanović, P., Knežević, G., and Stankov, L. (2015). Individual differences in cognitive biases: evidence against one-factor theory of rationality. Intelligence 50, 75–86. doi: 10.1016/j.intell.2015.02.008
Toplak, M. E., West, R. F., and Stanovich, K. E. (2011). The cognitive reflection test as a predictor of performance on heuristics-and-biases tasks. Mem. Cogn. 39, 1275–1289. doi: 10.3758/s13421-011-0104-1
Toplak, M. E., West, R. F., and Stanovich, K. E. (2017). Real-world correlates of performance on heuristics and biases tasks in a community sample. J. Behav. Decis. Mak. 30, 541–554. doi: 10.1002/bdm.1973
Tversky, A., and Kahneman, D. (1973). Availability: a heuristic for judging frequency and probability. Cogn. Psychol. 5, 207–232. doi: 10.1016/0010-0285(73)90033-9
Tversky, A., and Kahneman, D. (1974). Judgment under uncertainty: heuristics and biases. Science 185, 1124–1131. doi: 10.1126/science.185.4157.1124
Weaver, E. A., and Stewart, T. R. (2012). Dimensions of judgment: factor analysis of individual differences. J. Behav. Decis. Mak. 25, 402–413. doi: 10.1002/bdm.748
Weller, J., Ceschi, A., Hirsch, L., Sartori, R., and Costantini, A. (2018). Accounting for individual differences in decision-making competence: personality and gender differences. Front. Psychol. 9:2258. doi: 10.3389/fpsyg.2018.02258
West, R. F., Toplak, M. E., and Stanovich, K. E. (2008). Heuristics and biases as measures of critical thinking: associations with cognitive ability and thinking dispositions. J. Educ. Psychol. 100, 930–941. doi: 10.1037/a0012842
Keywords: individual differences, heuristics and biases, rationality, decision-making, measures
Citation: Berthet V and de Gardelle V (2023) The heuristics-and-biases inventory: An open-source tool to explore individual differences in rationality. Front. Psychol. 14:1145246. doi: 10.3389/fpsyg.2023.1145246
Edited by:
Dario Monzani, University of Palermo, ItalyReviewed by:
Rick Thomas, Georgia Institute of Technology, United StatesRichard S. John, University of Southern California, United States
Copyright © 2023 Berthet and de Gardelle. This is an open-access article distributed under the terms of the Creative Commons Attribution License (CC BY). The use, distribution or reproduction in other forums is permitted, provided the original author(s) and the copyright owner(s) are credited and that the original publication in this journal is cited, in accordance with accepted academic practice. No use, distribution or reproduction is permitted which does not comply with these terms.
*Correspondence: Vincent Berthet, dmluY2VudC5iZXJ0aGV0QHVuaXYtbG9ycmFpbmUuZnI=