- 1Department of Psychiatry and Clinical Psychology, Clínica Universidad de Navarra, Pamplona, Spain
- 2Instituto de Investigación Sanitaria de Navarra (IdiSNA), Pamplona, Spain
- 3Department of Psychiatry, Basurto University Hospital, Bilbao, Spain
- 4Colegio de Ciencias Sociales y Humanidades, Universidad San Francisco de Quito, Quito, Ecuador
- 5Instituto de Neurociencias, Universidad San Francisco de Quito, Quito, Ecuador
- 6Department of Radiology, Clínica Universidad de Navarra, Pamplona, Spain
Introduction: Over the past few years, several studies have described the brain activation pattern related to both time discrimination (TD) and change detection processes. We hypothesize that both processes share a common brain network which may play a significant role in more complex cognitive processes. The main goal of this proof-of-concept study is to describe the pattern of brain activity involved in TD and oddball detection (OD) paradigms, and in processes requiring higher cognitive effort.
Methods: We designed an experimental task, including an auditory test tool to assess TD and OD paradigms, which was conducted under functional magnetic resonance imaging (fMRI) in 14 healthy participants. We added a cognitive control component into both paradigms in our test tool. We used the general linear model (GLM) to analyze the individual fMRI data images and the random effects model for group inference.
Results: We defined the areas of brain activation related to TD and OD paradigms. We performed a conjunction analysis of contrast TD (task > control) and OD (task > control) patterns, finding both similarities and significant differences between them.
Discussion: We conclude that change detection and other cognitive processes requiring an increase in cognitive effort require participation of overlapping functional and neuroanatomical components, suggesting the presence of a common time and change detection network. This is of particular relevance for future research on normal cognitive functioning in the healthy population, as well as for the study of cognitive impairment and clinical manifestations associated with various neuropsychiatric conditions such as schizophrenia.
1. Introduction
Perception and processing of time plays a central role in human cognition and behavior (Allman and Meck, 2012). The ability to process time is described as an implicit aspect of our relationship with the world, how we perceive it and how we communicate and relate to it (Kononowicz et al., 2018; Nakajima et al., 2018; Sato, 2022), and occupies the highest level of the hierarchy of dimensions that forms our perception of the world (Navon, 1978). Time perception is involved in relatively basic activities (such as planning-sequencing actions), in processes of higher order (such as driving a car, play a musical instrument or performing physical activity), as well as in other sensory-perceptual processes (Gheorghiu and Erkelens, 2005; Pinheiro et al., 2019; Tonoyan et al., 2022).
Despite its relevance, the study of the subjective sense of time and the brain processes linked to it has been a challenge in recent years (Drayton and Furman, 2018). The experience of time (or time perception) is an abstract concept and difficult to define. It is also difficult to point out a specific anatomical area in charge of this process (Buzsáki and Llinás, 2017). Regardless of whether it is a real concept or a construct of our internal world, the processing of time relies on a network of specialized brain areas in charge of different aspects of timing (Nani et al., 2019; Lad et al., 2020).
According to the Scalar Expectancy Theory (SET), time perception involves three different cognitive processes: an internal clock, short and long term memory, and decisional processes (Gibbon et al., 1984). Neuroimaging studies have focused on microanalysis of specific and independent brain networks related to each of the three SET subcomponents (Pouthas and Perbal, 2004; Perbal et al., 2005; Allman and Meck, 2012). Multiple meta-analyses and task-based neuroimaging studies have highlighted the presence of a broad brain network involving both cortical (supplementary motor area, premotor cortex, inferior frontal gyrus, insula, inferior parietal cortex, superior temporal gyrus) and subcortical (left putamen, right thalamus and cerebellum) areas (Wiener et al., 2010; Ortuño et al., 2011; Lad et al., 2020). The findings show dimension-related differences, depending on the modality employed by the studies (motor or perceptual) or the duration of the time discrimination (sub-second or supra-second) (Nani et al., 2019). Some areas such as the supplementary motor area are consistently recruited regardless of modality (Coull et al., 2016) while others, such as the cerebellum, are activated in sub-second timing conditions (Bareš et al., 2019). Activation/deactivation of brain circuits underlying time processing have been reported to occur during several primary cognitive functions, such as working memory and certain executive functions (Stevens et al., 2007; Wiener et al., 2010; Radua et al., 2014; Wittmann, 2015; Davalos et al., 2018; Polti et al., 2018).
Time discrimination (TD) process allows us to perceive the duration of an event, that is, to calculate how much time has elapsed during a time interval. Thus, TD is basically and essentially a cognitive process related to the detection of change. Any non-temporary task requires processing changes, such as variations in the presentation of perceived stimuli or fluctuations in the level of demand. It is therefore expected to involve temporality processing and the involvement of the brain networks of temporality that support it (Livesey et al., 2007; Ortuño and Alústiza, 2014).
There is a growing consensus in the field of neurosciences about the fact that behavioral and cognitive functions are emergent properties, meaning that the combination and coordination of different brain regions give rise to new and complex functions that cannot be understood by simply looking at the individual regions roles (de Schotten and Forkel, 2022). These emergent properties are the result of the interactions and integration of different brain areas working together, which enables the performance of other functional roles such as perception, memory, decision-making, and more (Pessoa, 2022). Regarding time perception, several studies have documented this hypothesis, showing that the network associated with TD is involved in other processes, such as change detection (Stevens et al., 2000, 2007; Menon and Uddin, 2010; Alústiza et al., 2018; Justen and Herbert, 2018; Garcés et al., 2021) or cognitive control (Radua et al., 2014; Alústiza et al., 2017; Garcés et al., 2021), especially when execution of a task requires increases in cognitive effort (Alústiza et al., 2016; McTeague et al., 2016, 2017). Cognitive effort is an aspect of every cognitive process; it reflects the level of difficulty of a cognitive task and the resulting mental effort that a person must apply to achieve the cognitive goal (Radua et al., 2014).
The salience network, in charge of detecting salient or novel stimulus and guiding flexible behavior by regulating relevant functional networks, involves anterior insula, anterior cingulate cortex, amygdala, superior temporal gyrus, parietal cortex, ventral striatum and ventral tegmental area (Menon, 2015). One of the traditional paradigms to study this network involved in change detection is the oddball detection (OD) paradigm. This consists of an experimental technique in which a series of standard repetitive stimuli (auditory or visual) are presented and occasionally an infrequent stimulus (known as oddball or deviant) is included which evokes a reaction in the subject (Squires et al., 1975; Polich, 1986).
For several years, there has been growing research interest in temporal perception and its involvement in other cognitive processes (Head et al., 2008), both in healthy subjects and in relationship with psychopathology (Buhusi and Meck, 2005; Davalos et al., 2018). Both time and change processes are cognitive functions acquired gradually throughout human neurodevelopment (Buhusi and Meck, 2005; Brannon et al., 2008; Merchant et al., 2013). The interrelationship between TD and change detection processes may explain various aspects of normal and pathological cognition. With respect to normal cognition, we hypothesize the existence of a common time and change detection brain network that supports multiple non-temporal tasks. In a previous study, we conducted two independent signed differential mapping (SDM) meta-analysis of fMRI studies using timing and OD paradigms and a multimodal meta-analysis to elucidate whether there are brain regions common to these two processes, finding that the pattern of brain activation partially coincided (Garcés et al., 2021). These findings support the hypothesis that change detection paradigms, such as OD, could be related to a basic underlaying TD function and, eventually, to other non-temporary task and cognitive control processes, as far as they both implicate change.
Regarding pathological conditions and considering TD as a primary cognitive domain, this time and change detection network could clarify and explain some common features of brain disorders of different natures and pathophysiology. Multiple neuroimage studies and reviews point to the involvement of brain regions related to temporality and cognitive control functions in schizophrenia (Radua et al., 2014; de Montalembert et al., 2015; Alústiza et al., 2016, 2017; Ciullo et al., 2016; Lošák et al., 2016), neurodegenerative processes (Alústiza et al., 2016; Lad et al., 2020; Cope et al., 2022) and other neuropsychiatric conditions (Piras et al., 2014; Wise and Barnett-Cowan, 2018).
In this study we focus on brain activity involved in TD processes in neurotypical (healthy) people. Could TD and OD paradigm based tasks reveal the existence of a common brain network? Is this common network involved in processes of higher cognitive effort? To try to answer these questions, we designed our own experimental auditory test to assess TD and OD paradigms. In this proof-of-concept study our main goal is to describe the pattern of brain activity involved in TD and OD paradigms and in processes requiring higher cognitive effort. We hypothesize that the activation pattern of the TD network coincides to some degree with that of brain activity during the performance of OD and with the activation patterns of other brain networks involved in cognitive control processes.
2. Materials and methods
2.1. Design
We designed an experimental, descriptive, cross-sectional and quantitative study. Participants carried out tasks whilst undergoing fMRI of the brain. Tasks were based on different established paradigms (with different conditions) but were adapted so that subjects could carry them out within the fMRI machine. The objective was to scan brain activity generated during execution of experimental tasks.
2.2. Subjects
Recruitment was of healthy individuals. Inclusion and exclusion criteria are listed in Table 1. Possible participants completed an initial interview, which included sociodemographic information, and underwent detailed clinical assessment. All participants gave informed written consent to the participation. The study protocol was approved by the Research Ethics Committee of the University of Navarra.
The study sample was formed by 14 healthy volunteers (6 women and 8 men; mean age was 36 years, with standard deviation of 9.29 years), right-handed (except one left-handed subject). None of the volunteers had personal or first-degree family history of psychosis, or any other mental disorder (common or severe).
From the original sample (n = 14), a total of 13 participants successfully completed the study. The data from one of the subjects could not be analyzed due to technical problems, and therefore this subject was excluded from the final results of the study.
2.3. Experimental task
We designed our own test to assess TD and OD paradigms. In both paradigms, we built a cognitive control component by introducing two levels of difficulty (difficult and easy). Thus, participants were required to do two tasks (TD and OD) at two levels of difficulty: a total of four tasks (TD easy; TD difficult; OD easy; OD difficult).
2.3.1. Time discrimination (TD) paradigm
We designed a TD test (Table 2; Figure 1) based on the repetition of a sound (a pure sine wave at a frequency of 1,000 Hz and a sound pressure level of 90 dB/SPL) of 50 ms duration. The subject was required to indicate a change in the length of the silence between the sound beeps: the inter-stimulus interval (ISI). The test included both frequent and infrequent ISIs. The frequent ISI was 1,350 ms long, while infrequent ISIs varied in duration. The test was divided into two levels of difficulty:
• At the easy level (TD easy), we included a total of 21 auditory stimuli and 20 ISIs, with 80% being the frequent ISI and 20% (positions 3, 7, 13, and 17) being infrequent (2000 ms long).
• At the difficult level (TD difficult), we included 23 sounds and 22 ISIs. The frequent ISI had a frequency of occurrence of 73.3%, while 22.7% (positions 4, 7, 13, 16, 21) of the ISIs were infrequent, with a duration of 1,500 ms.
2.3.2. Oddball detection (OD) paradigm
The OD test (Table 3; Figure 2), like the TD test, was based on repetition of a sound (a pure sine wave at a sound pressure level of 90 dB/SPL) of 50 ms duration, with an ISI of 1,350 ms. The subject was required to detect and indicate change in pitch of the sound. The test included a total of 22 stimuli, both frequent and infrequent. The frequent stimulus (with an 80% probability of occurrence) had a frequency of 1,000 Hz. The infrequent stimulus (with a 20% probability) was divided into two levels of difficulty:
• At the easy level (OD easy), the infrequent stimulus had a frequency of 1,300 Hz and appeared at positions 5, 8, 13, 17, and 19
• A t the difficult level (OD difficult), the infrequent stimulus had a frequency of 1,200 Hz and appeared at positions 4, 7, 12, 16, and 19.
2.3.3. Task presentation
The above tasks were adapted and conditioned for fMRI. Tasks were done in blocks of 9 min duration. There was one block for the TD paradigm and another for the OD paradigm. After a rest period, the two blocks were repeated and so in total the test took about 40 min and we obtained 18 min of BOLD data for each paradigm (TD and OD). We alternated the order of the paradigms between participants, with some starting with the TD paradigm and others with the OD paradigm (generating two possible order of presentations: TD-OD-TD-OD or OD-TD-OD-TD).
Each block comprised 10 iterations of a single task (five easy and five difficult, alternating). Before each individual task, subjects did a “control task” in which they had to perform a non-discriminatory action. The stimulus sequence for the control task was the same as that for the subsequent discrimination/detection task. After a rest period, the two blocks were repeated, and so in total the test took about 40 min and we obtained 18 min of BOLD data for each paradigm (TD and OD).
Each block of trials began with an oral statement of the name of the paradigm (“time discrimination” or “oddball detection”) and a brief reminder of the action to be performed (pressing one of two buttons on a simple hand-held controller). Upon hearing the command “control,” subjects were to press the “1” button after each beep in the stimulus sequence, regardless of its characteristics, without making any discriminative effort. On hearing “task,” they were to press “1” after hearing the frequent form of the stimulus and “2” after hearing an infrequent form of the stimulus (i.e., a longer interval than usual, in TD, or higher frequency than usual, in OD) (Figure 3).
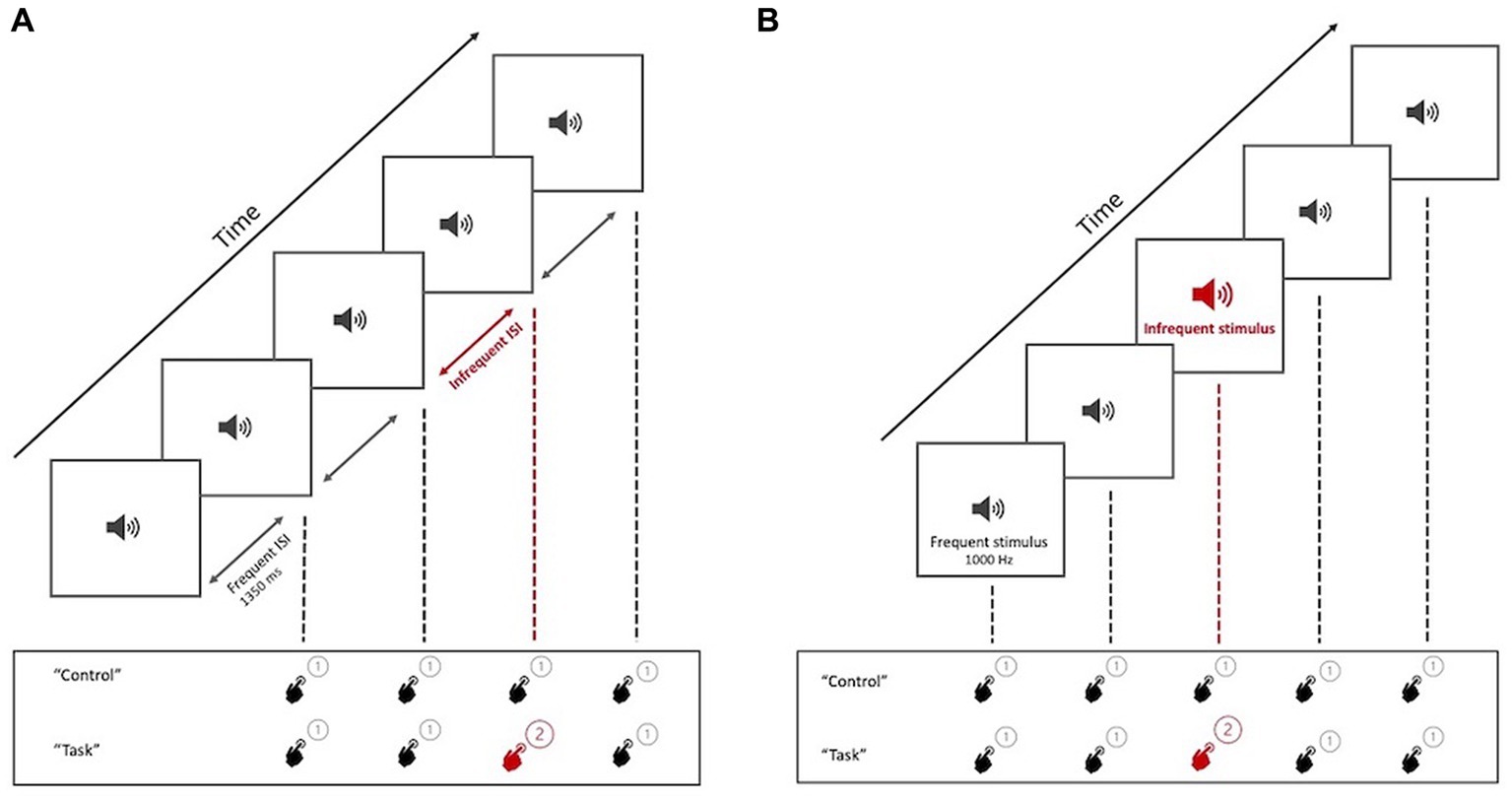
Figure 3. Graphical representation of the task presentation. (A) TD paradigm test, with the two tasks to be performed by the participant (Control and Task). (B) OD paradigm test, with the two tasks to be performed by the participant (Control and Task). When hearing the command “control,” participants press “1” regardless of the perceived stimulus (non-discriminatory activity). At the command “task,” participants must press “2” for the infrequent trait.
All participants did a training session of the test on a computer, prior to the fMRI session.
2.4. fMRI scanning
Studies were performed on a 3 Tesla MRI scanner (Siemens Skyra; RRID:SCR_020530) using a 20-channel head coil. A T2* weighted echo-planar imaging (EPI) sequence was employed to acquire the BOLD fMRI images. Each volume consisted of 46 slices, with a slice thickness of 3 mm and slice gap of 15%. Time interval between two consecutive acquisitions (TR) was 3 s and echo time (TE) was 30 ms. In-plane image resolution was 3.4 × 3.4 mm2, field-of-view (FOV) was 220 × 220 mm2, and flip angle was 90°. An anatomical image was also acquired using a 3D T1-weighted MPRAGE sequence with imaging parameters: TR = 2,320 ms, TE = 3.68 ms, inversion time (TI) = 1,170 ms, FOV = 208 × 256× 192 mm3, flip angle = 8°, number of slices = 192, and voxel size = 1 mm isotropic.
2.5. fMRI data analysis
BOLD images were pre-processed and analyzed using “Statistical Parametric Mapping” software, version SPM12 (RRID:SCR_007037), in MATLAB (RRID:SCR_001622). All EPI volumes were realigned to the mean and co-registered to the anatomical image. The anatomical image was then normalized to the Montreal Neurological Institute (MNI) template and the normalization parameters were applied to the EPI images. In this step, the EPI images were resampled to an isotropic voxel size of 2 mm. A 3D Gaussian smoothing filter of 8 mm full-width at half maximum was applied to the EPI images.
Statistical analysis was performed at two levels. At the first level, individual subject data were entered into a general linear model (GLM) (Friston et al., 1994). The design matrix was composed of 16 task/control regressors, 6 movement parameters as nuisance regressors and a constant regressor. The 16 task/control regressors model the occurrence of an event type. There were 8 event types: 2 paradigms (TD and OD) x 2 difficulty levels (easy and difficult) x 2 conditions (task and control). The regressors were convolved with a hemodynamic response function (canonical HRF). After model estimation, we calculated statistical contrasts in order to compare brain activation patterns.
For each individual, we performed the following t-contrasts with different objectives:
• To assess the brain activity evoked by each of the paradigms:
1. TD task contrast (TD task > TD control).
2. OD task contrast (OD task > OD control).
• To determine the differential/specific activation pattern of each of the paradigms:
1. TD specificity contrast (TD task > OD task).
2. OD specificity contrast (OD task > TD task).
• To measure the cognitive effort component in each of the paradigms:
1. TD effort contrast (TD task difficult > TD task easy).
2. OD effort contrast (OD task difficult > OD task easy).
• To analyze the differential/specific activation pattern of the TD paradigm in the cognitive effort component:
1. TD specificity in cognitive effort contrast [(TD task difficult > TD task easy) > (OD task difficult > OD task easy)].
The first level analysis was followed by group inference using the random effects model (Penny and Holmes, 2003). Random-effects group t-maps were generated for each of the first-level contrasts by applying a one-sample t-test for the contrast parameter values of all the subjects at each voxel. The significance level was set at a cluster-corrected p value of 0.05, with a cluster-generating threshold of 0.001. If map-wise analysis at this corrected level of significance failed to identify areas of activation, results were evaluated using an uncorrected p value of 0.001 with a minimum cluster size of 50.
Additionally, conjunction analysis was performed to determine areas of overlap between TD task and OD task (contrasts 1 and 2). This was done in two steps: first, thresholded activation maps were saved for both contrasts (i1 for TD and i2 for OD, respectively). Subsequently the imcalc tool was used in SPM with the following equation: (i1 > 0) + 2*(i2 > 0). The result was a new image with a value of 3 in areas activated by both tasks, a value of 1 in areas activated by TD only and a value of 2 in areas activated by OD only.
3. Results
The analysis of the fMRI data revealed distinct patterns of brain activity in response to the different planned contrasts.
3.1. TD and OD activity
Upon examining the TD task contrast (TD task > TD control), we were able to study the activation relative to performing the discriminatory task (TD task) compared to non-discriminatory activity (TD control). We applied a family-wise error (FWE) correction at the cluster level at a significance level of p = 0.05, with a cluster-defining threshold set at p = 0.001, which resulted in a minimum cluster size of 328 voxels (k = 328). We observed significant activation in several frontal cortical regions. Specifically, the right frontal orbital cortex, inferior frontal gyrus, superior frontal gyrus, and paracingulate gyrus displayed pronounced engagement during the TD task. Additionally, other areas such as the insular cortex and the cerebellum exhibited notable activity, suggesting their contribution in task execution (see Figure 4 and Supplementary Table S1).
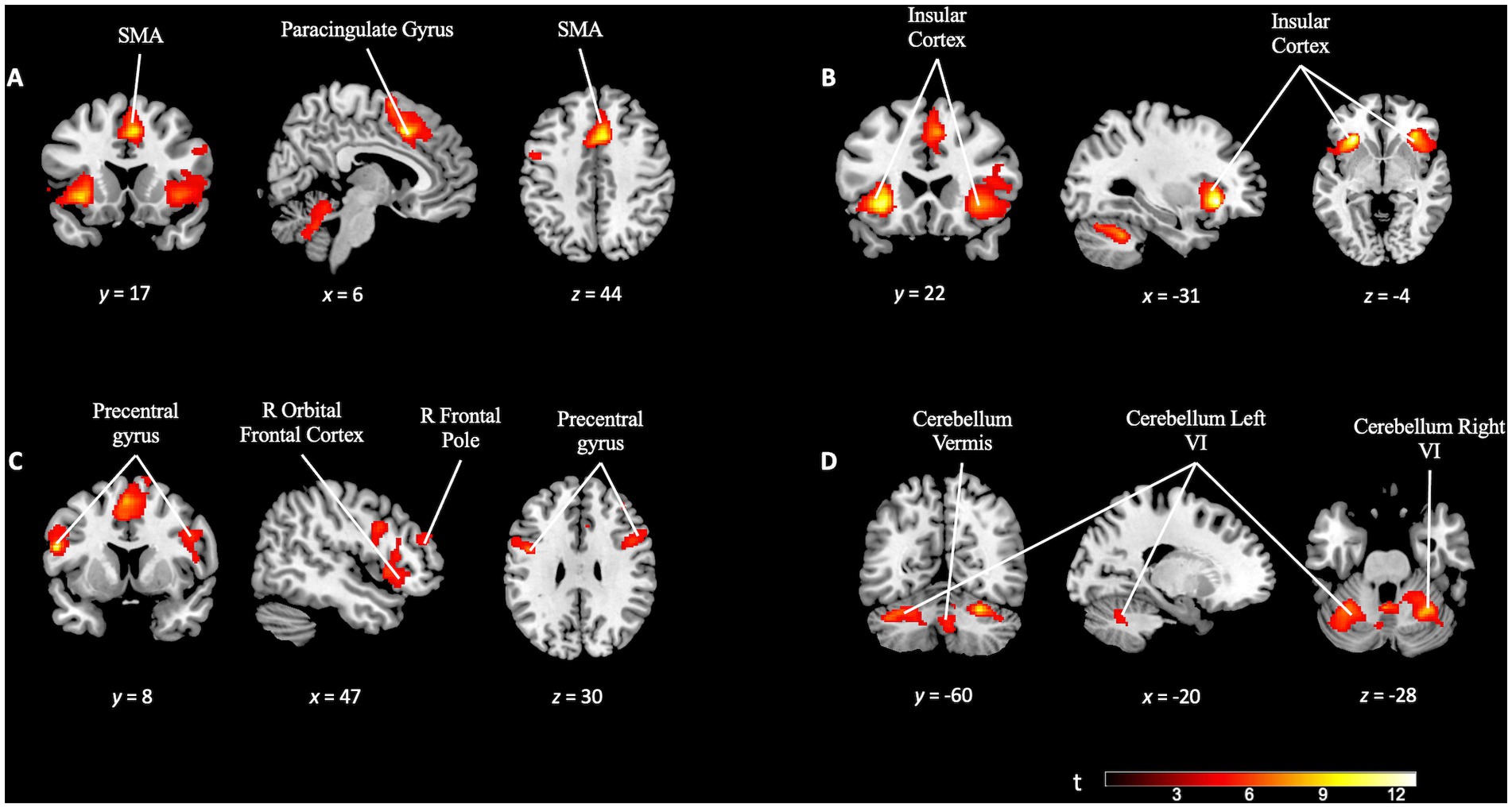
Figure 4. Brain activation pattern of TD task contrast (TD task > TD control; FWE cluster-corrected p = 0.05; cluster-defining p = 0.001; k = 328). (A) Activation of the paracingulate gyrus and supplementary motor area (SMA). (B) The insular cortex, bilaterally, involved in the same TD task. (C) Increased activation in the right prefrontal areas (right frontal pole, right orbital frontal cortex) and bilaterally (precentral gyrus). (D) Activation pattern in the cerebellum. For more detailed information, see Supplementary Table S1.
Meanwhile, the OD task contrast (OD task > OD control) was subjected to FWE cluster correction with a significance threshold of p = 0.05. The cluster-defining threshold was set at p = 0.001, resulting in a minimum cluster size (k) of 402 voxels. This analysis revealed extensive activation across various brain regions. Increased activity was observed in prefrontal areas, including the supplementary motor area (SMA) and the anterior cingulate cortex. In opposition to the TD task contrast, we observed significant activation in subcortical areas, such as the bilateral putamen. Notably, the cerebellum and posterior regions, such as the precuneus, also showed increased activity. Moreover, there was widespread activation in the bilateral temporal cortex (see Figure 5 and Supplementary Table S2).
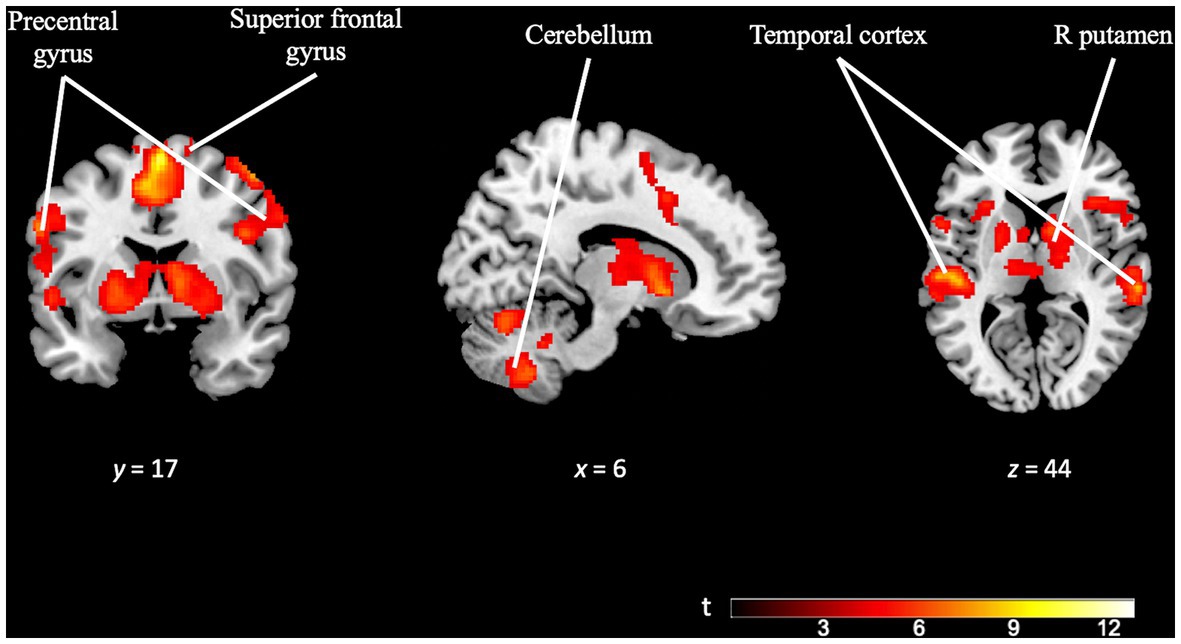
Figure 5. Brain activation pattern of OD task contrast (OD task > OD control; FWE cluster-corrected p = 0.05; cluster-defining p = 0.001; k = 402). Image showing significant activation in superior frontal gyrus, precentral gyrus, right putamen, temporal cortex (bilaterally) and cerebellum (bilaterally). For more detailed information on activation areas, see Supplementary Table S2.
3.2. TD and OD specificity
After analyzing the contrasts for each paradigm, we performed additional analyses to explore the unique and specific aspects of each task. The TD specificity contrast map (TD task > OD task), utilizing a minimum cluster size (k) of 50 voxels, revealed distinctive activation localized in the right lateral occipital cortex. Interestingly, this region is not typically associated with temporal discrimination (see Figure 6 and Supplementary Table S3).
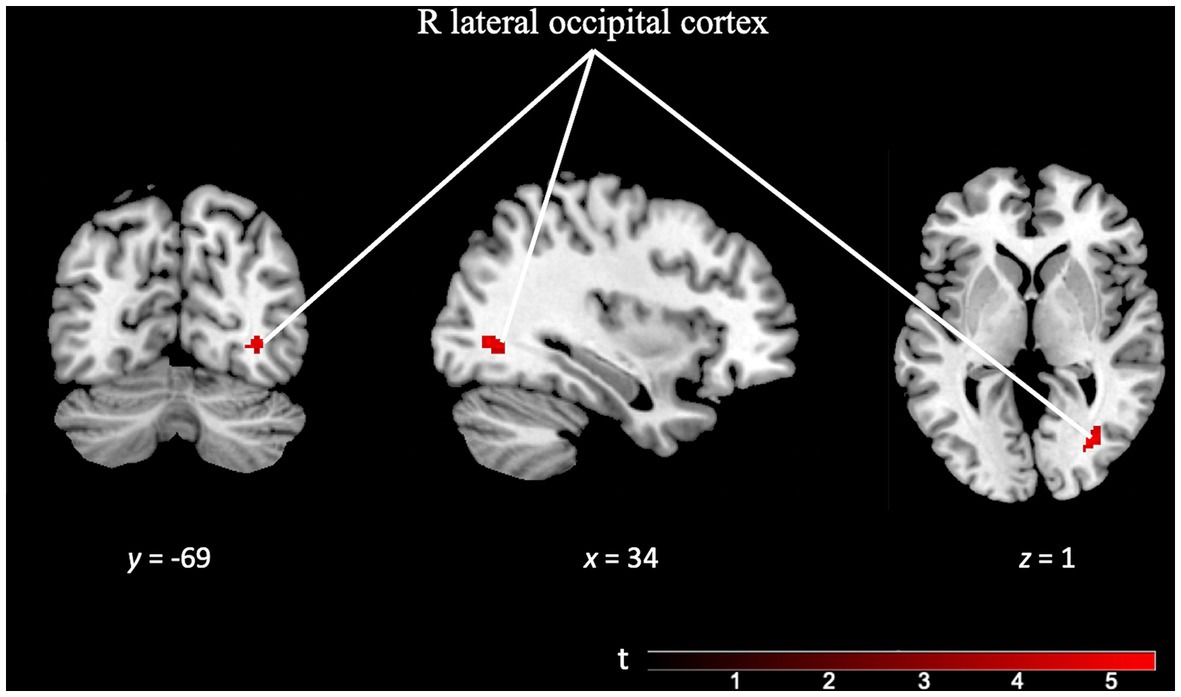
Figure 6. Brain activation pattern of TD specificity contrast (TD Task > OD Task; FWE cluster-corrected p = 0.05; cluster-defining p = 0.001; k = 50 vox) showing greater activity in right lateral occipital cortex (see Supplementary Table S3).
On the other hand, the OD specificity contrast map (OD task > TD task; k = 223 voxels) revealed significant activation across multiple brain regions. The precuneus cortex, temporal areas, pre-supplementary motor area, occipital cortex, and subcortical areas such as the right putamen exhibited prominent activity. Notably, the brain regions recruited by this task were significantly larger than those engaged in the TD task, confirming the differences observed in the separate analyses of each paradigm (see Figure 7 and Supplementary Table S4).
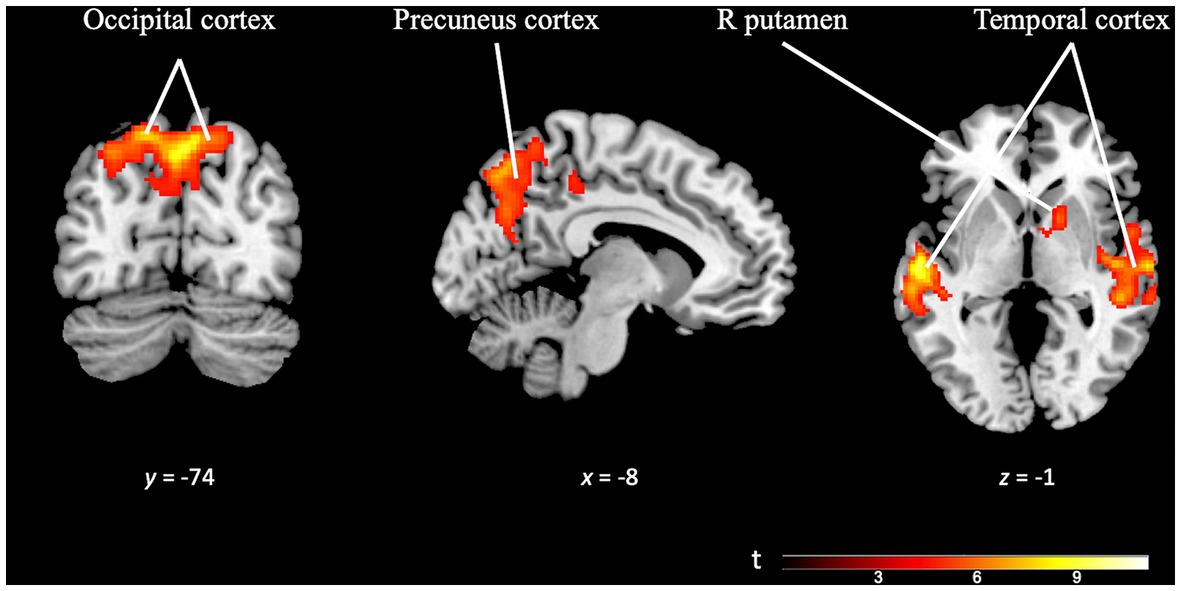
Figure 7. Brain activation pattern of OD specificity contrast (OD Task > TD Task; FWE cluster-corrected p = 0.05; cluster-defining p = 0.001; k = 223 vox). The selected slides in this image show greater activity in occipital cortex, precuneus cortex, temporal areas (bilaterally) and right putamen (see Supplementary Table S4).
In addition to investigating the specific differences between the tasks, a conjunction analysis was performed between the TD task and OD task contrast maps. This analysis aimed to examine the overlapping activation and identify common areas of engagement in greater detail. The analysis revealed significant activation overlap in several key regions, including the precentral gyrus, juxtapositional lobule cortex, superior frontal gyrus, paracingulate gyrus, bilateral frontal orbital cortex, bilateral insular cortex, and the cerebellum (see Figure 8). This suggests shared neural substrates and potential interactions between these regions during the execution of both tasks.
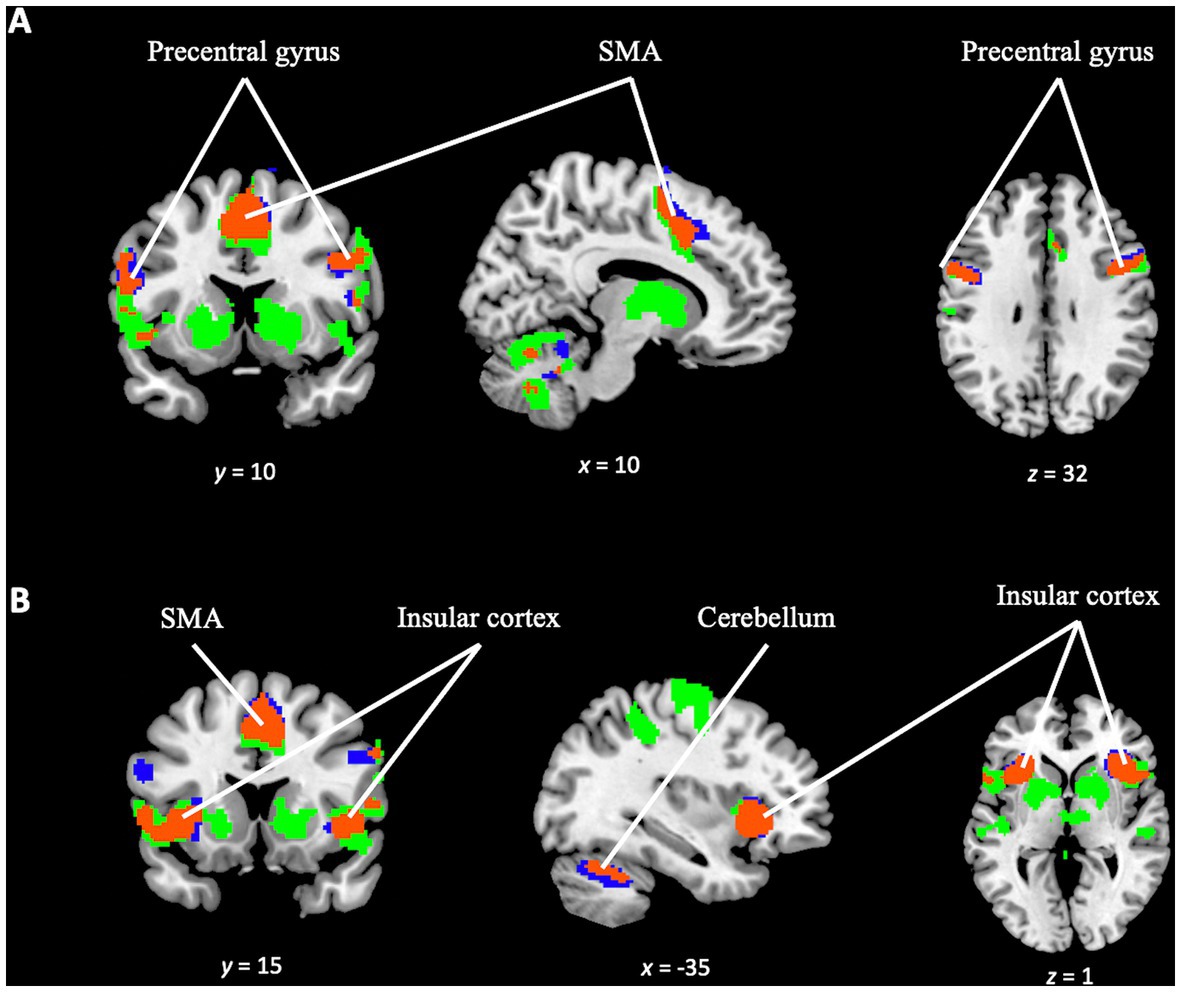
Figure 8. Conjunction analysis between TD task and OD task contrast. Blue color for TD task activation. Green color for OD task activation. In orange color, overlapping areas between the two contrasts. (A) Overlapping (orange) in supplementary motor area, precentral gyrus (bilaterally and cerebellum). (B) Overlapping (orange) in insular cortex (bilaterally), supplementary motor area and cerebellum.
3.3. Cognitive control
The component of cognitive effort was examined by analyzing the differences in activation patterns in response to increasing difficulty levels within each paradigm. The TD effort contrast (TD Task Difficult > TD Task Easy), employing a minimum cluster size of 50 voxels, revealed activation in right prefrontal areas, specifically the right frontal pole and right frontal operculum. These findings suggest increased cognitive engagement and resource allocation in these regions during the more challenging TD task conditions (see Figure 9 and Supplementary Table S5).
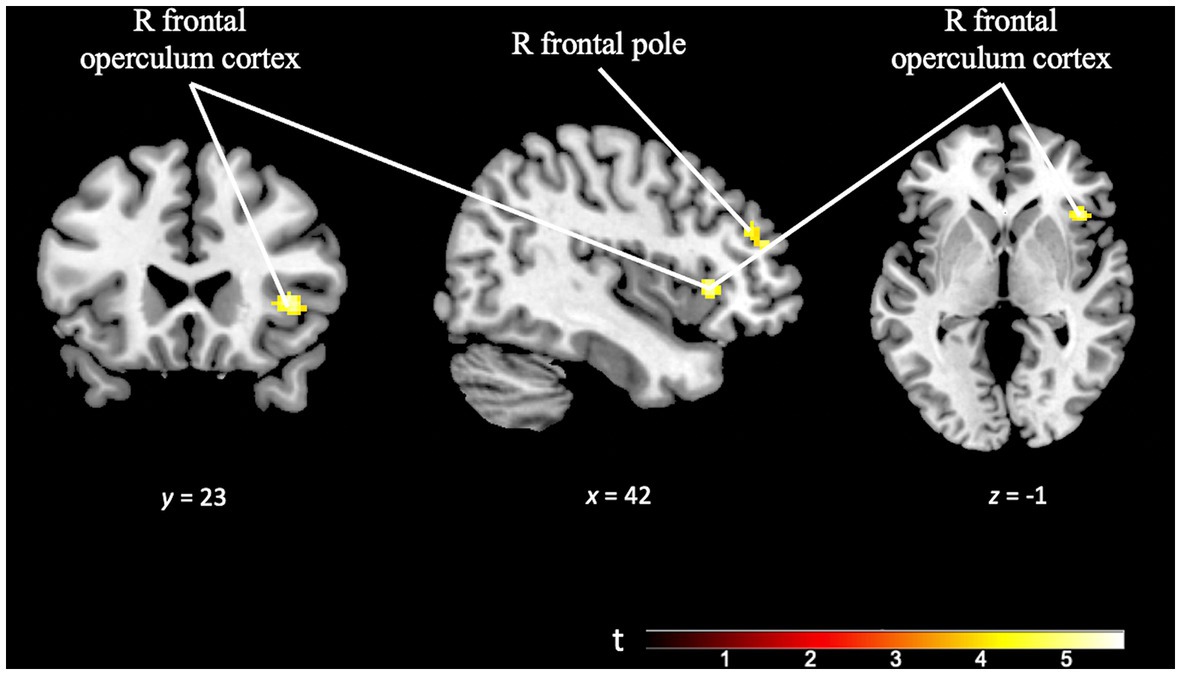
Figure 9. Brain activation pattern of TD effort contrast (TD task difficult > TD task easy; p = 0.001; k = 50 vox) showing greater activation in right frontal pole and right frontal operculum cortex (see Supplementary Table S5).
However, the OD paradigm did not reveal discernible differences between various levels of difficulty. When analyzing the OD effort contrast (OD Task Difficult > OD Task Easy), no statistically significant differences in the activation patterns were observed. This indicates that the OD task may not induce distinct levels of cognitive effort as detected by the fMRI measurements employed in this study.
Finally, the TD specificity in cognitive effort contrast [(TD task difficult > TD task easy) > (OD task difficult > OD task easy)], minimum cluster size (k) of 50 voxels, revealed activation in the left dorsal dentate nucleus of the cerebellum. This finding suggests that the observed activation in this region is specific to the cognitive effort required during the more challenging TD task (see Figure 10 and Supplementary Table S6).
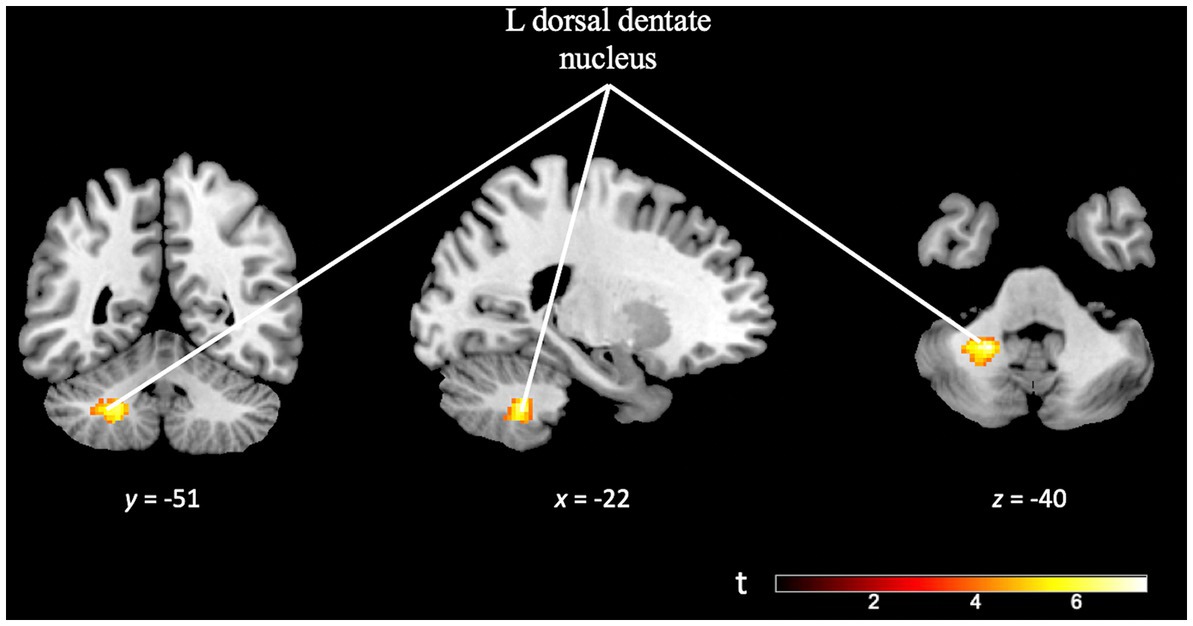
Figure 10. Brain activation pattern of TD specificity in cognitive effort contrast, [(TD task difficult > TD task easy) > (OD task difficult > OD task easy)]; FWE cluster-corrected (p = 0.05; cluster-defining p = 0.001, k = 50), showing greater activity in the left dorsal dentate nucleus of the cerebellum (see Supplementary Table S6).
4. Discussion
The findings obtained in this original research contribute to the endeavor to understand time perception. Within the different dimensions encompassed by temporality, in this study we decided to focus on brain activity related to TD in a healthy population. We have attempted to describe the brain regions involved in TD and its interrelation with change detection processes, especially by including a component of higher cognitive load.
Previous studies have pointed out the relationship of temporality with other cognitive processes, such as change detection (Ortuño et al., 2005; Livesey et al., 2007; Alústiza et al., 2018; Garcés et al., 2021). This hypothesized connection becomes more evident when studying processes that require greater cognitive demand, since any cognitive task involves salience and change detection processes (Wiener et al., 2010; Niendam et al., 2012; Ortuño and Alústiza, 2014; Radua et al., 2014; Alústiza et al., 2016, 2017). Therefore, we hypothesize that there is a common brain network underlying time and change detection processes. Following this idea, we conducted a meta-analysis study (Garcés et al., 2021) in which we provided evidence that timing and salience processes are connected. Considering the limitations of a meta-analysis study and in order to corroborate this hypothesis, we considered it worthwhile to perform our own experimental functional neuroimaging experiment in the small-scale proof-of-concept study reported here.
Instead of studying only TD function-specific brain activity, as an isolated and independent brain function, in this experimental study we included tasks for different paradigms (TD and OD) and conditions (cognitive control). This design, based on the interrelation and connection of different brain areas, is closer to the scientific consensus about the real functioning and structure of the brain (de Schotten and Forkel, 2022). Our group has designed and is working on the validation studies of a new experimental computerized perceptual testing tool for TD-OD as a feasible way of studying time perception and its implications. In this proof-of-concept study, we applied a fMRI adaptation of this tool, to examine our hypotheses and evaluate our methodological approach, which has wide applicability in other relevant populations (Kendig, 2015). We consider that the TD process, and the study of the brain circuits involved betters our understanding of cognitive and perceptual processes in the healthy brain and in different neuropsychiatric conditions, especially in schizophrenia (Andreasen et al., 1999; Davalos et al., 2011; Ortuño et al., 2011; Roy et al., 2012; Gómez et al., 2014; Alústiza et al., 2016, 2018; Ciullo et al., 2016; Lošák et al., 2016; Parker et al., 2021; Todd et al., 2022).
4.1. TD and OD activity
For each of the two paradigms (TD and OD) we decided to include a control task. During this task (passive listening), participants did not have to perform any discriminative activity (passive listening), but only pressed the same button (“1”), regardless of the perceived stimulus (frequent or infrequent). In this way, comparing the discriminative task with the control task, we were able to assess the evoked activity in each subject in a more detailed way (Sadaghiani et al., 2009; Justen and Herbert, 2018; Kamp et al., 2018).
We describe the pattern of brain activation corresponding to the performance of the TD paradigm. The activation patterns observed (Figure 4) are consistent with those previously reported in the literature (Stevens et al., 2007; Wiener et al., 2010; Ortuño et al., 2011; Nani et al., 2019). In a previous meta-analysis, our research team observed the existence of a cortico-cerebellar-thalamic-cortical circuit, related to time estimation (Ortuño et al., 2011). The same brain areas are the focus of attention for other studies researching temporal processing (Wiener et al., 2010; Matthews and Meck, 2016; Petter et al., 2016; Eichenbaum, 2017; Nobre and van Ede, 2017; Coull and Droit-Volet, 2018; Gili et al., 2018; Nani et al., 2019; Lad et al., 2020; Cona et al., 2021). In conjunction, these findings are consistent with previous evidence for the existence of a brain circuit responsible for temporality.
In this study, we included a non-temporal cognitive task based on the OD paradigm. The brain activity we observed during the performance of OD tasks (greater activity in areas such as the pre-SMA, cerebellum, cingulate cortex and bilateral putamen), was consistent with what is reported in the literature (Ortuño et al., 2005; Stevens et al., 2007; Wiener et al., 2010; Menon, 2015).
4.2. Is there a common brain network underlying the TD and OD paradigms?
Given these two contrasts (the TD activity and the OD activity contrasts) and according to the results of the conjunction analysis between them, we can state that the brain activity corresponding to each of these tasks partially coincides, involving the supplementary motor area, primary motor cortex, prefrontal cortex, insula and cerebellum. However, there are some differences in the activation pattern of both paradigms. In the activity related to the TD paradigm (and not in the OD task contrast), we observed greater activity in the right lateral occipital area, in principle, an area not previously described as involved in temporality. In a previous meta-analysis study (Radua et al., 2014) we found a similar finding, although in that study the additional area can be explained by the fact that the task stimuli used there were visual (and not auditory, as they are in this study). In the study, the presence of the additional activation area can plausibly be explained, as follows, in terms of a complication introduced by the test design. While conducting the experiment, both the researchers and participants felt that the TD paradigm was more difficult than the OD paradigm. We suspect that the possible difference in difficulty between tasks (Alexiou et al., 2009) might trigger the recruitment of other brain areas not related to temporality in order to accomplish the TD task successfully. Occipital cortex activation has been described in both auditory and visual oddball tasks (Stevens et al., 2000; Goldman et al., 2009; McDonald et al., 2013; Alústiza et al., 2018; Justen and Herbert, 2018), which would reinforce the idea that it is precisely in tasks that demand greater cognitive effort that network overlap is more evident. Regarding future studies, we believe that it would be convenient to evaluate in a more objective way, not only the performance in execution, but also the difficulty perceived by the participants during the task (Alexiou et al., 2009; Hay et al., 2022).
When we compare the activation pattern for OD with that for TD, we observe that during OD there was greater activation in temporal regions, bilaterally, and in the precuneus. This observation and the differences in general between TD and OD activation patterns may be due to the study design and the ways tasks were presented. In particular, for the TD paradigm tasks, participants had to focus on the duration of the silence between two sounds, while for the OD paradigm tasks, participants had to focus on the frequency of a sound. So, it is to be expected that brain areas involved in auditory processes would be activated in OD paradigm tasks, as we observed.
Despite the limitations of the study, in view of the fact that the differences between brain activation patterns were small, predictable and also explainable by the limitations of the study design, our results support the hypothesis that the network defined under the TD paradigm has areas in common with other cognitive networks and processes such as OD (Alústiza et al., 2016, 2017, 2018; Garcés et al., 2021).
4.3. Cognitive effort
By establishing easy and difficult modes of the two experimental tasks, we aimed to study the effect of cognitive control on activation patterns. Regarding TD, in the TD effort contrast we found that increased difficulty resulted in implication of right prefrontal regions (specifically, the right frontal pole and the right frontal operculum cortex), which is suggestive of involvement of the dorsolateral prefrontal cortex (DLPFC), which has been previously described as part of a multi-demand system related to more complex cognitive functions and tasks (Tregellas et al., 2006; Lewandowska et al., 2010; Koizumi et al., 2018; Chen et al., 2020; Hertrich et al., 2021). These findings are consistent with the hypothesis that brain networks invoked in TD are engaged in tasks that require greater cognitive difficulty (Livesey et al., 2007; Davalos et al., 2011; Niendam et al., 2012; Alústiza et al., 2016, 2017, 2018).
The OD effort contrast did not show statistically significant differences. This could be because the increase of difficulty in OD task (difference between OD difficult and OD easy) is perhaps not demanding enough to activate the regions involved in greater cognitive effort. In addition, the small sample size in this preliminary study makes it difficult to obtain robust results.
Is there any brain region specific to TD, not active during OD, whose role becomes more important with increasing cognitive load? Interestingly, comparison of the contrast map for increase in difficulty in the TD task with the contrast map for increase in difficulty in the OD task, reveals greater activation in cerebellar regions (see Figure 10). Numerous studies have pointed out the relevant role of the cerebellum in temporal processing and its relationship with areas such as the SMA, parietal cortex, thalamus and basal ganglia (Bengtsson et al., 2005; Koziol et al., 2014; Finnerty et al., 2015; Petter et al., 2016; Bareš et al., 2019). Particularly, detection of the involvement of the cerebellum and subcortical areas in temporal processing is clearer when using tasks of sub-second timing conditions, as in the present study (Petter et al., 2016; Nani et al., 2019).
The pattern of cerebellar activity detected has been reported to be related to motor functions and complex cognitive functions, especially those that involve prefrontal areas (Bernard et al., 2014). This result points at the possibility of the TD network not only being involved in time perception processes, but also being relevant and perhaps playing a specific mediating role in more complex cognitive processes (such as executive functions) (Niendam et al., 2012; Radua et al., 2014; Lošák et al., 2016; Bareš et al., 2019; Pinheiro et al., 2021).
4.4. Limitations
Some of the limitations of this study – the modest sample size, in particular – are inherent to a proof-of-concept study (Kendig, 2015). The proposed theoretical framework of the brain circuits involved in TD processes and their implication in other cognitive functions, being derived from a small sample size, must be taken with caution. Nevertheless, we believe that the insights gained in our experimental study are applicable to larger samples and clinical populations for future research oriented to the implementation and validation of our test-tool. Despite these limitations, the present results are consistent with previous evidence from other task-based neuroimaging studies (Wiener et al., 2010; Niendam et al., 2012; Nani et al., 2019; Garcés et al., 2021), which may help promote larger scale studies.
Another limitation of our study is the age range of participants. To increase sample size and generalizability to the population, we included healthy subjects aged 18 to 65 in our study protocol. In the final sample of participants, the age range was between 23 and 49 years old. However, it is important to note that age-related changes in time discrimination can affect both test performance and neuroimaging results in older subjects (Mioni et al., 2021). In future studies examining temporality and other cognitive functions, it would be beneficial to consider the impact of participant age on the findings. A possible solution could be to include younger subjects, or to expand the sample size to study the effect of age in more detail as a possible confounding factor. An alternative approach would be to compare the findings with performance in other domains (such as processing speed), which have been known to be influenced by age (Baudouin et al., 2018).
In this study, the nature of the tests implied certain limitations: some of the differences in the TD and OD task activation patterns can be attributed to the greater auditory component of the latter task; it is possible that TD task was more difficult to execute than the OD task; and it is hard to determine accurately the increased cognitive load between the two levels of the OD task. We believe, however, that such limitations have not been entirely detrimental but also provided us with valuable information on brain functioning.
The general heterogeneity in neuroimaging research is a big concern. Although this heterogeneity is partly attributable to the range of experimental task employed, a priority in our opinion is to describe differences between individual subjects. To do this, there is a need for further fMRI studies involving more participants and participants with different neuropsychiatric conditions.
Despite recent improvements in the field of neuroimaging, each of the available imaging techniques provides us with a partial view of the real brain structure and function. Integrating different techniques and associating them with fMRI studies can be valuable to study primary domains such as TD. Having looked at the relationship between time perception and change detection, it would be interesting to study the evoked potentials, such as, mismatch negativity (MMN) (Moore et al., 2021). The combinations of findings from task-based fMRI studies and studies that focus on brain structure (i.e., diffusion techniques) may help us to better understand brain connectivity and functioning (de Schotten and Forkel, 2022) especially in disorders such as schizophrenia where aberrant temporal processing has been described (Alústiza et al., 2016, 2017, 2018; Ciullo et al., 2016) and may be related to abnormal structural brain connectivity (white matter impairment) (Ortuño et al., 2011; Gómez et al., 2014; Kochunov et al., 2017, 2022).
5. Conclusion
Our study contributes to knowledge about the brain network responsible for temporal perception. In particular, we were interested in the brain areas related to TD and their interrelation with cognitive processes involving change detection in the healthy population. To that end, based on the TD and OD paradigms, we designed a test-tool including a cognitive control component and adapted it for use within MRI equipment. Our results indicate the existence of a relationship between the brain circuits of TD and other discriminative cognitive processes (OD), and in addition shed some light on how these TD circuits could participate in more complex cognitive processes (i.e., executive functions). We provide experimental proof-of-concept evidence, consistent with findings in previous studies, that supports our hypotheses and validates the methodology for application in larger populations. We consider this to be important for research on various neuropsychiatric conditions and, especially, schizophrenia.
Data availability statement
The original contributions presented in the study are included in the article/Supplementary material, further inquiries can be directed to the corresponding author.
Ethics statement
The studies involving human participants were reviewed and approved by Research Ethics Committee of the University of Navarra. The patients/participants provided their written informed consent to participate in this study.
Author contributions
FO conceived the idea of the experiment and the original hypothesis. MF-S designed the original experimental measurement tool. JG, IA, MF-S, MF, and FO worked on the study design and adapted the measurement tool to MRI. JG and CV-A were involved in the recruitment and initial screening interview of participants. JG led the experiment, under the supervision of and with the help of CV-A and IA. RG-E was responsible for the diagnosis and clinical reporting of structural brain imaging findings. MF-S was responsible for the pre-processing of the fMRI images. MF-S and JG performed the statistical analysis of the results and developed the images. JG wrote the manuscript. IA, CV-A, PM, MF-S, and FO commented, corrected and approved the manuscript. All authors contributed to the article and approved the submitted version.
Funding
This research has been financed by a project of the Carlos III Health Institute. Health Institutions (Project File Number: PI17/00240). Funded by Instituto de Salud Carlos III and co-funded by European Union (ERDF/ESF, “A way to make Europe”).
Acknowledgments
The author thank David Burdon for English proofreading.
Conflict of interest
PM is supported by Clínica Universidad de Navarra and has received research grants from the Ministry of Education (Spain), the Government of Navarra (Spain) and the Spanish Foundation of Psychiatry and Mental Health and AstraZeneca; he is a clinical consultant for MedAvante-ProPhase and has received lecture honoraria from and/or has been a consultant for AB-Biotics, Adept Field Solutions, Guidepoint, Janssen, Novumed, Roland Berger and Scienta.
The remaining authors declare that the research was conducted in the absence of any commercial or financial relationships that could be construed as a potential conflict of interest.
Publisher’s note
All claims expressed in this article are solely those of the authors and do not necessarily represent those of their affiliated organizations, or those of the publisher, the editors and the reviewers. Any product that may be evaluated in this article, or claim that may be made by its manufacturer, is not guaranteed or endorsed by the publisher.
Supplementary material
The Supplementary material for this article can be found online at: https://www.frontiersin.org/articles/10.3389/fpsyg.2023.1110972/full#supplementary-material
Abbreviations
fMRI, functional magnetic resonance imaging; ISI, inter-stimulus interval; TD, time discrimination; OD, oddball detection.
References
Alexiou, K., Zamenopoulos, T., Johnson, J. H., and Gilbert, S. J. (2009). Exploring the neurological basis of design cognition using brain imaging: some preliminary results. Des. Stud. 30, 623–647. doi: 10.1016/J.DESTUD.2009.05.002
Allman, M. J., and Meck, W. H. (2012). Pathophysiological distortions in time perception and timed performance. Brain 135, 656–677. doi: 10.1093/BRAIN/AWR210
Alústiza, I., Garcés, M. S., Solanes, A., Goena, J., Ortuño, M., Molero, P., et al. (2018). Aberrant timing and oddball detection in schizophrenia: findings from a signed differential mapping meta-analysis. Heliyon 4:e01004. doi: 10.1016/j.heliyon.2018.e01004
Alústiza, I., Radua, J., Albajes-Eizagirre, A., Domínguez, M., Aubá, E., and Ortuño, F. (2016). Meta-analysis of functional neuroimaging and cognitive control studies in schizophrenia: preliminary elucidation of a Core dysfunctional timing network. Front. Psychol. 7:192. doi: 10.3389/fpsyg.2016.00192
Alústiza, I., Radua, J., Pla, M., Martin, R., and Ortuño, F. (2017). Meta-analysis of functional magnetic resonance imaging studies of timing and cognitive control in schizophrenia and bipolar disorder: evidence of a primary time deficit. Schizophr. Res. 188, 21–32. doi: 10.1016/j.schres.2017.01.039
Andreasen, N. C., Nopoulos, P., O’Leary, D. S., Miller, D. D., Wassink, T., and Flaum, M. (1999). Defining the phenotype of schizophrenia: cognitive dysmetria and its neural mechanisms. Biol. Psychiatry 46, 908–920. doi: 10.1016/S0006-3223(99)00152-3
Bareš, M., Apps, R., Avanzino, L., Breska, A., D'Angelo, E., Filip, P., et al. (2019). Consensus paper: decoding the contributions of the cerebellum as a time machine. From neurons to clinical applications. Cerebellum 18, 266–286. doi: 10.1007/S12311-018-0979-5
Baudouin, A., Isingrini, M., and Vanneste, S. (2018). Executive functioning and processing speed in age-related differences in time estimation: a comparison of young, old, and very old adults. Aging, Neuropsychology, and Cognition 26, 264–281. doi: 10.1080/13825585.2018.1426715
Bengtsson, S. L., Ehrsson, H. H., Forssberg, H., and Ullén, F. (2005). Effector-independent voluntary timing: behavioural and neuroimaging evidence. Eur. J. Neurosci. 22, 3255–3265. doi: 10.1111/J.1460-9568.2005.04517.X
Bernard, J. A., Peltier, S. J., Benson, B. L., Wiggins, J. L., Jaeggi, S. M., Buschkuehl, M., et al. (2014). Dissociable functional networks of the human dentate nucleus. Cereb. Cortex 24, 2151–2159. doi: 10.1093/CERCOR/BHT065
Brannon, E. M., Libertus, M. E., Meck, W. H., and Woldorff, M. G. (2008). Electrophysiological measures of time processing in infant and adult brains: Weber’s law holds. J. Cogn. Neurosci. 20, 193–203. doi: 10.1162/JOCN.2008.20016
Buhusi, C. V., and Meck, W. H. (2005). What makes us tick? Functional and neural mechanisms of interval timing. Nat. Rev. Neurosci. 6, 755–765. doi: 10.1038/nrn1764
Buzsáki, G., and Llinás, R. (2017). Space and time in the brain. Science 358, 482–485. doi: 10.1126/SCIENCE.AAN8869
Chen, P. Y., le Chen, C., Hsu, Y. C., and Tseng, W. Y. I. (2020). Fluid intelligence is associated with cortical volume and white matter tract integrity within multiple-demand system across adult lifespan. NeuroImage 212:116576. doi: 10.1016/J.NEUROIMAGE.2020.116576
Ciullo, V., Spalletta, G., Caltagirone, C., Jorge, R. E., and Piras, F. (2016). Explicit time deficit in schizophrenia: systematic review and meta-analysis indicate it is primary and not domain specific. Schizophr. Bull. 42, 505–518. doi: 10.1093/SCHBUL/SBV104
Cona, G., Wiener, M., and Scarpazza, C. (2021). From ATOM to GradiATOM: cortical gradients support time and space processing as revealed by a meta-analysis of neuroimaging studies. NeuroImage 224:117407. doi: 10.1016/J.NEUROIMAGE.2020.117407
Cope, T. E., Hughes, L. E., Phillips, H. N., Adams, N. E., Jafarian, A., Nesbitt, D., et al. (2022). Causal evidence for the multiple demand network in change detection: auditory mismatch magnetoencephalography across focal neurodegenerative diseases. J. Neurosci. 42, 3197–3215. doi: 10.1523/JNEUROSCI.1622-21.2022
Coull, J. T., and Droit-Volet, S. (2018). Explicit understanding of duration develops implicitly through action. Trends Cogn. Sci. 22, 923–937. doi: 10.1016/J.TICS.2018.07.011
Coull, J. T., Vidal, F., and Burle, B. (2016). When to act, or not to act: that’s the SMA’s question. Curr. Opin. Behav. Sci. 8, 14–21. doi: 10.1016/J.COBEHA.2016.01.003
Davalos, D., Mioni, G., Grondin, S., and Ortuño, F. (2018). Editorial: time perception and dysfunction: clinical and practical implications. Front. Hum. Neurosci. 12:435. doi: 10.3389/FNHUM.2018.00435/BIBTEX
Davalos, D. B., Rojas, D. C., and Tregellas, J. R. (2011). Temporal processing in schizophrenia: effects of task-difficulty on behavioral discrimination and neuronal responses. Schizophr. Res. 127, 123–130. doi: 10.1016/J.SCHRES.2010.06.020
de Montalembert, M., Tordjman, S., Bonnot, O., and Coulon, N. (2015). Perception temporelle et schizophrénie: approche phénoménologique et neuropsychologique. Encéphale 41, S56–S61. doi: 10.1016/S0013-7006(15)30008-7
de Schotten, M. T., and Forkel, S. J. (2022). The emergent properties of the connected brain. Science 378, 505–510. doi: 10.1126/SCIENCE.ABQ2591
Drayton, L., and Furman, M. (2018). Thy mind, thy brain and time. Trends Neurosci. 41, 641–643. doi: 10.1016/J.TINS.2018.09.001
Eichenbaum, H. (2017). On the integration of space, time, and memory. Neuron 95, 1007–1018. doi: 10.1016/J.NEURON.2017.06.036
Finnerty, G. T., Shadlen, M. N., Jazayeri, M., Nobre, A. C., and Buonomano, D. (2015). Time in cortical circuits. J. Neurosci. 35, 13912–13916. doi: 10.1523/JNEUROSCI.2654-15.2015
Friston, K. J., Holmes, A. P., Worsley, K. J., Poline, J. -P., Frith, C. D., and Frackowiak, R. S. J. (1994). Statistical parametric maps in functional imaging: a general linear approach. Hum. Brain Mapp. 2, 189–210. doi: 10.1002/HBM.460020402
Garcés, M. S., Alústiza, I., Albajes-Eizagirre, A., Goena, J., Molero, P., Radua, J., et al. (2021). An fMRI study using a combined task of interval discrimination and oddball could reveal common brain circuits of cognitive change. Front. Psych. 12:786113. doi: 10.3389/FPSYT.2021.786113/FULL
Gheorghiu, E., and Erkelens, C. J. (2005). Differences in perceived depth for temporally correlated and uncorrelated dynamic random-dot stereograms. Vis. Res. 45, 1603–1614. doi: 10.1016/J.VISRES.2004.12.005
Gibbon, J., Church, R. M., and Meck, W. H. (1984). Scalar timing in memory. Ann. N. Y. Acad. Sci. 423, 52–77. doi: 10.1111/J.1749-6632.1984.TB23417.X
Gili, T., Ciullo, V., and Spalletta, G. (2018). Metastable states of multiscale brain networks are keys to crack the timing problem. Front. Comput. Neurosci. 12:75. doi: 10.3389/FNCOM.2018.00075/BIBTEX
Goldman, R. I., Wei, C. Y., Philiastides, M. G., Gerson, A. D., Friedman, D., Brown, T. R., et al. (2009). Single-trial discrimination for integrating simultaneous EEG and fMRI: identifying cortical areas contributing to trial-to-trial variability in the auditory oddball task. NeuroImage 47, 136–147. doi: 10.1016/J.NEUROIMAGE.2009.03.062
Gómez, J., Jesús Marín-Méndez, J., Molero, P., Atakan, Z., and Ortuño, F. (2014). Time perception networks and cognition in schizophrenia: A review and a proposal. Psychiatry. Res. 220, 737–744. doi: 10.1016/j.psychres.2014.07.048
Hay, L., Duffy, A. H. B., Gilbert, S. J., and Grealy, M. A. (2022). Functional magnetic resonance imaging (fMRI) in design studies: methodological considerations, challenges, and recommendations. Des. Stud. 78:101078. doi: 10.1016/J.DESTUD.2021.101078
Head, D., Rodrigue, K. M., Kennedy, K. M., and Raz, N. (2008). Neuroanatomical and cognitive mediators of age-related differences in episodic memory. Neuropsychology 22, 491–507. doi: 10.1037/0894-4105.22.4.491
Hertrich, I., Dietrich, S., Blum, C., and Ackermann, H. (2021). The role of the dorsolateral prefrontal cortex for speech and language processing. Front. Hum. Neurosci. 15:217. doi: 10.3389/FNHUM.2021.645209/BIBTEX
Justen, C., and Herbert, C. (2018). The spatio-temporal dynamics of deviance and target detection in the passive and active auditory oddball paradigm: a sLORETA study. BMC Neurosci. 19:25. doi: 10.1186/s12868-018-0422-3
Kamp, T., Sorger, B., Benjamins, C., Hausfeld, L., and Goebel, R. (2018). The prestimulus default mode network state predicts cognitive task performance levels on a mental rotation task. Brain Behav. 8:e01034. doi: 10.1002/BRB3.1034
Kendig, C. E. (2015). What is proof of concept research and how does it generate epistemic and ethical categories for future scientific practice? Sci. Eng. Ethics. 22, 735–753. doi: 10.1007/S11948-015-9654-0
Kochunov, P., Coyle, T. R., Rowland, L. M., Jahanshad, N., Thompson, P. M., Kelly, S., et al. (2017). Association of white matter with core cognitive deficits in patients with schizophrenia. JAMA Psychiat. 74, 958–966. doi: 10.1001/JAMAPSYCHIATRY.2017.2228
Kochunov, P., Hong, L. E., Dennis, E. L., Morey, R. A., Tate, D. F., Wilde, E. A., et al. (2022). ENIGMA-DTI: translating reproducible white matter deficits into personalized vulnerability metrics in cross-diagnostic psychiatric research. Hum. Brain Mapp. 43, 194–206. doi: 10.1002/HBM.24998
Koizumi, A., Lau, H., Shimada, Y., and Kondo, H. M. (2018). The effects of neurochemical balance in the anterior cingulate cortex and dorsolateral prefrontal cortex on volitional control under irrelevant distraction. Conscious. Cogn. 59, 104–111. doi: 10.1016/J.CONCOG.2018.01.001
Kononowicz, T. W., Rijn, H.van, and Meck, W. H. (2018). Timing and time perception. Stevens’ Handbook of Experimental Psychology and Cognitive Neuroscience, J. Wixted and S. Ghetti, John Wiley & Sons, Inc, Hoboken, 1–38.
Koziol, L. F., Budding, D., Andreasen, N., D’Arrigo, S., Bulgheroni, S., Imamizu, H., et al. (2014). Consensus paper: the cerebellum’s role in movement and cognition. Cerebellum 13, 151–177. doi: 10.1007/S12311-013-0511-X/FIGURES/8
Lad, S. S., Hurley, R. A., and Taber, K. H. (2020). Temporal processing: neural correlates and clinical relevance. J. Neuropsychiatry Clin. Neurosci. 32, 104–108. doi: 10.1176/APPI.NEUROPSYCH.19120342/ASSET/IMAGES/LARGE/APPI.NEUROPSYCH.19120342F3.JPEG
Lewandowska, M., Piatkowska-Janko, E., Bogorodzki, P., Wolak, T., and Szelag, E. (2010). Changes in fMRI BOLD response to increasing and decreasing task difficulty during auditory perception of temporal order. Neurobiol. Learn. Mem. 94, 382–391. doi: 10.1016/J.NLM.2010.08.005
Livesey, A. C., Wall, M. B., and Smith, A. T. (2007). Time perception: manipulation of task difficulty dissociates clock functions from other cognitive demands. Neuropsychologia 45, 321–331. doi: 10.1016/J.NEUROPSYCHOLOGIA.2006.06.033
Lošák, J., Hüttlová, J., Lipová, P., Mareček, R., Bareš, M., Filip, P., et al. (2016). Predictive motor timing and the cerebellar vermis in schizophrenia: an fMRI study. Schizophr. Bull. 42, 1517–1527. doi: 10.1093/schbul/sbw065
Matthews, W. J., and Meck, W. H. (2016). Temporal cognition: connecting subjective time to perception, attention, and memory. Psychol. Bull. 142, 865–907. doi: 10.1037/BUL0000045
McDonald, J. J., Störmer, V. S., Martinez, A., Feng, W., and Hillyard, S. A. (2013). Salient sounds activate human visual cortex automatically. J. Neurosci. 33, 9194–9201. doi: 10.1523/JNEUROSCI.5902-12.2013
McTeague, L. M., Goodkind, M. S., and Etkin, A. (2016). Transdiagnostic impairment of cognitive control in mental illness. J. Psychiatr. Res. 83, 37–46. doi: 10.1016/J.JPSYCHIRES.2016.08.001
McTeague, L. M., Huemer, J., Carreon, D. M., Jiang, Y., Eickhoff, S. B., and Etkin, A. (2017). Identification of common neural circuit disruptions in cognitive control across psychiatric disorders. Am. J. Psychiatry 174, 676–685. doi: 10.1176/APPI.AJP.2017.16040400
Menon, V. (2015). “Salience network” in Brain Mapping: An Encyclopedic Reference. ed. A. W. Toga, vol. 2 (Cambridge, MA: Academic Press), 597–611.
Menon, V., and Uddin, L. Q. (2010). Saliency, switching, attention and control: a network model of insula function. Brain Struct. Funct. 214, 655–667. doi: 10.1007/S00429-010-0262-0/FIGURES/6
Merchant, H., Harrington, D. L., and Meck, W. H. (2013). Neural basis of the perception and estimation of time. Annu. Rev. Neurosci. 36, 313–336. doi: 10.1146/ANNUREV-NEURO-062012-170349
Mioni, G., Cardullo, S., Ciavarelli, A., and Stablum, F.. (2021). Age-related changes in time discrimination: The involvement of inhibition, working memory and speed of processing. Current Psychology 40, 2462–2471. doi: 10.1007/S12144-019-00170-8/FIGURES/2
Moore, M., Maclin, E. L., Iordan, A. D., Katsumi, Y., Larsen, R. J., Bagshaw, A. P., et al. (2021). Proof-of-concept evidence for trimodal simultaneous investigation of human brain function. Hum. Brain Mapp. 42, 4102–4121. doi: 10.1002/HBM.25541
Nakajima, Y., Matsuda, M., Ueda, K., and Remijn, G. B. (2018). Temporal resolution needed for auditory communication: measurement with mosaic speech. Front. Hum. Neurosci. 12:149. doi: 10.3389/FNHUM.2018.00149/BIBTEX
Nani, A., Manuello, J., Liloia, D., Duca, S., Costa, T., and Cauda, F. (2019). The neural correlates of time: a meta-analysis of neuroimaging studies. J. Cogn. Neurosci. 31, 1796–1826. doi: 10.1162/JOCN_A_01459
Navon, D. (1978). Perception of misoriented words and letter strings. Can. J. Psychol. 32, 129–140. doi: 10.1037/H0081681
Niendam, T. A., Laird, A. R., Ray, K. L., Dean, Y. M., Glahn, D. C., and Carter, C. S. (2012). Meta-analytic evidence for a superordinate cognitive control network subserving diverse executive functions. Cogn. Affect. Behav. Neurosci. 12, 241–268. doi: 10.3758/S13415-011-0083-5
Nobre, A. C., and van Ede, F. (2017). Anticipated moments: temporal structure in attention. Nat. Rev. Neurosci. 19, 34–48. doi: 10.1038/nrn.2017.141
Ortuño, F., and Alústiza, I. (2014). Aristotle got it right again! Med. Hypotheses 83, 509–510. doi: 10.1016/J.MEHY.2014.07.014
Ortuño, F., Guillén-Grima, F., López-García, P., Gómez, J., and Pla, J. (2011). Functional neural networks of time perception: challenge and opportunity for schizophrenia research. Schizophr. Res. 125, 129–135. doi: 10.1016/j.schres.2010.10.003
Ortuño, F. M., Lopez, P., Ojeda, N., and Cervera, S. (2005). Dysfunctional supplementary motor area implication during attention and time estimation tasks in schizophrenia: a PET-O15 water study. NeuroImage 24, 575–579. doi: 10.1016/J.NEUROIMAGE.2004.09.010
Parker, D. A., Trotti, R. L., McDowell, J. E., Keedy, S. K., Hill, S. K., Gershon, E. S., et al. (2021). Auditory oddball responses across the schizophrenia-bipolar spectrum and their relationship to cognitive and clinical features. Am. J. Psychiatry. 178, 952–964. doi: 10.1176/APPI.AJP.2021.20071043
Penny, W. D., and Holmes, A. J. (2003). “Random-effects analysis” in Human brain function. eds. R. Frackowiak, F. Karl, F. Christopher, D. Raymond, P. Cathy, and Z. Semir, et al. (Cambridge, MA: Academic Press), 1144.
Perbal, S., Deweer, B., Pillon, B., Vidailhet, M., Dubois, B., and Pouthas, V. (2005). Effects of internal clock and memory disorders on duration reproductions and duration productions in patients with Parkinson’s disease. Brain Cogn. 58, 35–48. doi: 10.1016/j.bandc.2005.02.003
Pessoa, L. (2022). The entangled brain: How perception, cognition, and emotion are woven together. The MIT Press.
Petter, E. A., Lusk, N. A., Hesslow, G., and Meck, W. H. (2016). Interactive roles of the cerebellum and striatum in sub-second and supra-second timing: support for an initiation, continuation, adjustment, and termination (ICAT) model of temporal processing. Neurosci. Biobehav. Rev. 71, 739–755. doi: 10.1016/J.NEUBIOREV.2016.10.015
Pinheiro, A. P., Johnson, J. F., Amorim, M., Roberto, M., Schwartze, M., Kotz, S. A., et al. (2021). The cerebellum links to positive symptoms of psychosis: a systematic review and meta-analysis. Schizophr. Bull. Open 2:sgab039. doi: 10.1093/SCHIZBULLOPEN/SGAB039
Pinheiro, A. P., Schwartze, M., Gutierrez, F., and Kotz, S. A. (2019). When temporal prediction errs: ERP responses to delayed action-feedback onset. Neuropsychologia 134:107200. doi: 10.1016/J.NEUROPSYCHOLOGIA.2019.107200
Piras, F., Piras, F., Ciullo, V., Danese, E., Caltagirone, C., and Spalletta, G. (2014). Time dysperception perspective for acquired brain injury. Front. Neurol. 4:217. doi: 10.3389/FNEUR.2013.00217/BIBTEX
Polich, J. (1986). Normal variation of P300 from auditory stimuli. Electroencephalogr. Clin. Neurophysiol. 65, 236–240. doi: 10.1016/0168-5597(86)90059-6
Polti, I., Martin, B., and van Wassenhove, V. (2018). The effect of attention and working memory on the estimation of elapsed time. Sci. Rep. 8:6690. doi: 10.1038/S41598-018-25119-Y
Pouthas, V., and Perbal, S. (2004). Time perception depends on accurate clock mechanisms as well as unimpaired attention and memory processes. Acta. Neurobiol. Exp. (Wars) 64, 367–385. Available at: https://www.ane.pl/pdf/6434.pdf
Radua, J., del Pozo, N. O., Gómez, J., Guillen-Grima, F., and Ortuño, F. (2014). Meta-analysis of functional neuroimaging studies indicates that an increase of cognitive difficulty during executive tasks engages brain regions associated with time perception. Neuropsychologia 58, 14–22. doi: 10.1016/j.neuropsychologia.2014.03.016
Roy, M., Grondin, S., and Roy, M. A. (2012). Time perception disorders are related to working memory impairment in schizophrenia. Psychiatry Res. 200, 159–166. doi: 10.1016/J.PSYCHRES.2012.06.008
Sadaghiani, S., Hesselmann, G., and Kleinschmidt, A. (2009). Distributed and antagonistic contributions of ongoing activity fluctuations to auditory stimulus detection. J. Neurosci. 29, 13410–13417. doi: 10.1523/JNEUROSCI.2592-09.2009
Sato, M. (2022). The timing of visual speech modulates auditory neural processing. Brain Lang. 235:105196. doi: 10.1016/J.BANDL.2022.105196
Squires, N. K., Squires, K. C., and Hillyard, S. A. (1975). Two varieties of long-latency positive waves evoked by unpredictable auditory stimuli in man. Electroencephalogr. Clin. Neurophysiol. 38, 387–401. doi: 10.1016/0013-4694(75)90263-1
Stevens, M. C., Kiehl, K. A., Pearlson, G., and Calhoun, V. D. (2007). Functional neural circuits for mental timekeeping. Hum. Brain Mapp. 28, 394–408. doi: 10.1002/HBM.20285
Stevens, A. A., Skudlarski, P., Gatenby, J. C., and Gore, J. C. (2000). Event-related fMRI of auditory and visual oddball tasks. Magn. Reson. Imaging 18, 495–502. doi: 10.1016/S0730-725X(00)00128-4
Todd, J., Howard, Z., Auksztulewicz, R., and Salisbury, D. (2022). Computational modeling of oddball sequence processing exposes common and differential auditory network changes in first-episode schizophrenia-Spectrum disorders and schizophrenia. Schizophr. Bull. 49, 407–416. doi: 10.1093/SCHBUL/SBAC153
Tonoyan, Y., Fornaciai, M., Parsons, B., and Bueti, D. (2022). Subjective time is predicted by local and early visual processing. NeuroImage 264:119707. doi: 10.1016/J.NEUROIMAGE.2022.119707
Tregellas, J. R., Davalos, D. B., and Rojas, D. C. (2006). Effect of task difficulty on the functional anatomy of temporal processing. NeuroImage 32, 307–315. doi: 10.1016/J.NEUROIMAGE.2006.02.036
Wiener, M., Turkeltaub, P., and Coslett, H. B. (2010). The image of time: a voxel-wise meta-analysis. NeuroImage 49, 1728–1740. doi: 10.1016/J.NEUROIMAGE.2009.09.064
Wise, A., and Barnett-Cowan, M. (2018). Perceived simultaneity and temporal order of audiovisual events following concussion. Front. Hum. Neurosci. 12:139. doi: 10.3389/FNHUM.2018.00139/BIBTEX
Keywords: time discrimination, time perception, change detection, oddball paradigm, salience, cognition, cognitive control, fMRI
Citation: Goena J, Alústiza I, Vidal-Adroher C, Garcés MS, Fernández M, Molero P, García-Eulate R, Fernández-Seara M and Ortuño F (2023) Time discrimination and change detection could share a common brain network: findings of a task-based fMRI study. Front. Psychol. 14:1110972. doi: 10.3389/fpsyg.2023.1110972
Edited by:
Mingming Zhang, Liaoning Normal University, ChinaReviewed by:
Valentina Ciullo, Santa Lucia Foundation (IRCCS), ItalySatoshi Iwase, Aichi Medical University, Japan
Copyright © 2023 Goena, Alústiza, Vidal-Adroher, Garcés, Fernández, Molero, García-Eulate, Fernández-Seara and Ortuño. This is an open-access article distributed under the terms of the Creative Commons Attribution License (CC BY). The use, distribution or reproduction in other forums is permitted, provided the original author(s) and the copyright owner(s) are credited and that the original publication in this journal is cited, in accordance with accepted academic practice. No use, distribution or reproduction is permitted which does not comply with these terms.
*Correspondence: Irene Alústiza, aWxhbHVzdGl6YUB1bmF2LmVz
†These authors share senior authorship